Development of methods for identifying the state of various dynamic objects
DOI:
https://doi.org/10.15587/2706-5448.2023.279437Keywords:
heterogeneous dynamic objects, complex technical systems, complex analysis, processing of various types of dataAbstract
Artificial intelligence technologies are actively used to solve both general and highly specialized tasks. In the process of assessing (identifying) the condition of complex and heterogeneous objects, there is a high degree of a priori uncertainty regarding their condition and a small amount of initial data describing them. The trends of armed conflicts of the last decades and the regularities of the development of information systems, convincingly indicate the need to change approaches to the collection of information from various sources and their analysis. There is a constant transformation of the forms of information presentation and the order of storage and access to various types of data. The problem of integrating disparate sources of information collection into a single information space is also not fully resolved.
That is why the issue of improving the efficiency of assessing the state of complex and heterogeneous dynamic objects is an important and urgent issue. The objects of research are heterogeneous dynamic objects. The subject of the research is the identification of the state of heterogeneous dynamic objects. In the research, the method of identifying the state of heterogeneous dynamic objects was developed. The novelty of the proposed method consists in:
‒ taking into account the degree of uncertainty about the state of a heterogeneous dynamic object;
‒ taking into account the degree of data noise as a result of distortion of data characterizing the state of a heterogeneous dynamic object;
‒ reducing computing costs while assessing the state of heterogeneous dynamic objects;
‒ the possibility of performing calculations with source data that are different in nature and units of measurement.
It is advisable to implement the mentioned method in specialized software, which is used to analyze the state of complex technical systems and make decisions.
References
- Shishatckii, A. V., Bashkirov, O. M., Kostina, O. M. (2015). Rozvitok іntegrovanikh sistem zv’iazku ta peredachі danikh dlia potreb Zbroinikh Sil. Ozbroennia ta vіiskova tekhnіka, 1 (5), 35–40.
- Dudnyk, V., Sinenko, Y., Matsyk, M., Demchenko, Y., Zhyvotovskyi, R., Repilo, I. et al. (2020). Development of a method for training artificial neural networks for intelligent decision support systems. Eastern-European Journal of Enterprise Technologies, 3 (2 (105)), 37–47. doi: https://doi.org/10.15587/1729-4061.2020.203301
- Zuiev, P., Zhyvotovskyi, R., Zvieriev, O., Hatsenko, S., Kuprii, V., Nakonechnyi, O. et al. (2020). Development of complex methodology of processing heterogeneous data in intelligent decision support systems. Eastern-European Journal of Enterprise Technologies, 4 (9 (106)), 14–23. doi: https://doi.org/10.15587/1729-4061.2020.208554
- Yeromina, N., Kurban, V., Mykus, S., Peredrii, O., Voloshchenko, O., Kosenko, V. et al. (2021). The Creation of the Database for Mobile Robots Navigation under the Conditions of Flexible Change of Flight Assignment. International Journal of Emerging Technology and Advanced Engineering, 11 (5), 37–44. doi: https://doi.org/10.46338/ijetae0521_05
- Rotshtein, A. P. (1999). Intellektualnye tekhnologii identifikatcii: nechetkie mnozhestva, geneticheskie algoritmy, neironnye seti. Vinnitca: UNIVERSUM, 320.
- Ramaji, I. J., Memari, A. M. (2018). Interpretation of structural analytical models from the coordination view in building information models. Automation in Construction, 90, 117–133. doi: https://doi.org/10.1016/j.autcon.2018.02.025
- Pérez-González, C. J., Colebrook, M., Roda-García, J. L., Rosa-Remedios, C. B. (2019). Developing a data analytics platform to support decision making in emergency and security management. Expert Systems with Applications, 120, 167–184. doi: https://doi.org/10.1016/j.eswa.2018.11.023
- Chen, H. (2018). Evaluation of Personalized Service Level for Library Information Management Based on Fuzzy Analytic Hierarchy Process. Procedia Computer Science, 131, 952–958. doi: https://doi.org/10.1016/j.procs.2018.04.233
- Chan, H. K., Sun, X., Chung, S.-H. (2019). When should fuzzy analytic hierarchy process be used instead of analytic hierarchy process? Decision Support Systems, 125, 113114. doi: https://doi.org/10.1016/j.dss.2019.113114
- Osman, A. M. S. (2019). A novel big data analytics framework for smart cities. Future Generation Computer Systems, 91, 620–633. doi: https://doi.org/10.1016/j.future.2018.06.046
- Gödri, I., Kardos, C., Pfeiffer, A., Váncza, J. (2019). Data analytics-based decision support workflow for high-mix low-volume production systems. CIRP Annals, 68 (1), 471–474. doi: https://doi.org/10.1016/j.cirp.2019.04.001
- Harding, J. L. (2013). Data quality in the integration and analysis of data from multiple sources: some research challenges. The International Archives of the Photogrammetry, Remote Sensing and Spatial Information Sciences, XL-2/W1, 59–63. doi: https://doi.org/10.5194/isprsarchives-xl-2-w1-59-2013
- Kosko, B. (1986). Fuzzy cognitive maps. International Journal of Man-Machine Studies, 24 (1), 65–75. doi: https://doi.org/10.1016/s0020-7373(86)80040-2
- Gorelova, G. V. (2013). Kognitivnyi podkhod k imitatcionnomu modelirovaniiu slozhnykh sistem. Izvestiia IuFU. Tekhnicheskie nauki, 3, 239–250.
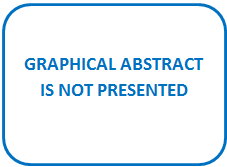
Downloads
Published
How to Cite
Issue
Section
License
Copyright (c) 2023 Oleksii Romanov, Andrii Shyshatskyi, Oleh Shknai, Volodymyr Yashchenok, Tetiana Stasiuk, Oleksandr Trotsko, Nadiia Protas, Hennadii Miahkykh, Vira Velychko, Dmytro Balan

This work is licensed under a Creative Commons Attribution 4.0 International License.
The consolidation and conditions for the transfer of copyright (identification of authorship) is carried out in the License Agreement. In particular, the authors reserve the right to the authorship of their manuscript and transfer the first publication of this work to the journal under the terms of the Creative Commons CC BY license. At the same time, they have the right to conclude on their own additional agreements concerning the non-exclusive distribution of the work in the form in which it was published by this journal, but provided that the link to the first publication of the article in this journal is preserved.