Method of diagnosing some diseases of the neuro-muscular system and features of data processing in software
DOI:
https://doi.org/10.15587/2706-5448.2023.273848Keywords:
skeletal muscle, diagnostics of the neuromuscular system, modeling, evoked potentials, electrical stimulation, algorithm, softwareAbstract
Electromyostimulation is a method of restorative treatment based on electrical stimulation of nerves and muscles. The electric current, which is used in electrical stimulation to obtain induced muscle contractions, is characterized by a large number of different parameters. However, not every possible option of electrical stimulation is highly effective. To solve the task of diagnosing some diseases of the neuromuscular system, it is important to organize the software by analyzing the parameters of the evoked potentials. Therefore, the object of research is the processes of skeletal muscle contraction under the influence of natural electrical pulses of the nervous system or under the influence of external electrical stimulation. The subject of research is models describing the processes in muscles during contraction and methods of data processing. In the course of the study, such research methods as mathematical modeling methods and methods of processing medical and biological data were used.
The paper examines the experimental strength-duration dependence of skeletal muscle and obtained mathematical models for the normal state of the neuromuscular apparatus and different degrees of denervation. On the basis of electrodiagnosis of a patient with impaired motor functions, the dynamics of changes in the patient's condition and the effectiveness of treatment were traced. Based on the results of the study, an analysis of the parameters of the evoked potentials of the stimulation electromyogram during adaptive electrostimulation was carried out in order to control its effectiveness or establish a diagnosis in some diseases of the neuromuscular system. This made it possible to develop a method for correcting errors in the interpretation of one of the quality parameters and increase the reliability of the diagnosis. The obtained results can be used in the improvement of technical devices for electrostimulation therapy, as well as control of the effectiveness of rehabilitation procedures.
References
- Bochkezanian, V., Newton, R. U., Trajano, G. S., Vieira, A., Pulverenti, T. S., Blazevich, A. J. (2018). Effect of tendon vibration during wide-pulse neuromuscular electrical stimulation (NMES) on muscle force production in people with spinal cord injury (SCI). BMC Neurology, 18 (1). doi: https://doi.org/10.1186/s12883-018-1020-9
- Bekhet, A. H., Bochkezanian, V., Saab, I. M., Gorgey, A. S. (2019). The Effects of Electrical Stimulation Parameters in Managing Spasticity After Spinal Cord Injury. American Journal of Physical Medicine & Rehabilitation, 98 (6), 484–499. doi: https://doi.org/10.1097/phm.0000000000001064
- Bochkezanian, V., Newton, R. U., Trajano, G. S., Blazevich, A. J. (2018). Effects of Neuromuscular Electrical Stimulation in People with Spinal Cord Injury. Medicine & Science in Sports & Exercise, 50 (9), 1733–1739. doi: https://doi.org/10.1249/mss.0000000000001637
- Yeroshenko, O., Prasol, I., Suknov, M. (2022). Modeling of electrostimulation characteristics to determine the optimal amplitude of current stimuli. Radioelectronic and computer systems, 2, 191–199. doi: https://doi.org/10.32620/reks.2022.2.15
- Yeroshenko, O., Prasol, I. (2022). Simulation of the electrical signal of the muscles to obtain the electromiosignal spectrum. Technology Audit and Production Reserves, 2 (2 (64)), 38–43. doi: https://doi.org/10.15587/2706-5448.2022.254566
- Pascual-Valdunciel, A., Rajagopal, A., Pons, J. L., Delp, S. (2022). Non-invasive electrical stimulation of peripheral nerves for the management of tremor. Journal of the Neurological Sciences, 435, 120195. doi: https://doi.org/10.1016/j.jns.2022.120195
- Vysotskа, О., Georgiyants, M., Pecherska, A., Porvan, A., Boguslavska, N. (2018). Information technology for choosing the corrective facilities under stress impact on the biological object. Radioelectronic and Computer Systems, 3, 34–48. doi: https://doi.org/10.32620/reks.2018.3.05
- Dudar, T. V., Titarenko, O. V., Nekos, A. N., Vysotska, O. V., Porvan, A. P. (2021). Some aspects of environmental hazard due to uranium mining in Ukraine. Journal of Geology, Geography and Geoecology, 30 (1), 34–42. doi: https://doi.org/10.15421/112104
- Vуsotska, O., Nosov, K., Hnoevyi, I., Porvan, A., Rysovana, L., Dovnar, A. et al. (2022). Image processing procedure for remote recording of the Gambusia sp. introduced into a water for anti-malaria. Technology Audit and Production Reserves, 1 (2 (63)), 14–18. doi: https://doi.org/10.15587/2706-5448.2022.252297
- Liashenko, O., Barkovska O., Al-Atroshi, C., Datsok, O., Liashenko, S. (2019). Model of the Work of the Neurocontroller to Control Fuzzy Data from the Sensors of the Climate Control Subsystem «Smart House». International Journal of Advanced Trends in Computer Science and Engineering, 8 (1.2), 70–74.
- Chumachenko, D., Yakovlev, S. (2021). Intelligent system of epidemic situation monitoring and control. CEUR Workshop Proceedingsthis link is disabled, 2870, 46–55.
- Jung, J., Lee, D.-W., Son, Y. K., Kim, B. S., Shin, H. C. (2021). Volitional EMG Estimation Method during Functional Electrical Stimulation by Dual-Channel Surface EMGs. Sensors, 21 (23), 8015–8032. doi: https://doi.org/10.3390/s21238015
- Dideriksen, J. L., Laine, C. M., Dosen, S., Muceli, S., Rocon, E., Pons, J. L. et al. (2017). Electrical Stimulation of Afferent Pathways for the Suppression of Pathological Tremor. Frontiers in Neuroscience, 11. doi: https://doi.org/10.3389/fnins.2017.00178
- Kim, J., Wichmann, T., Inan, O. T., DeWeerth, S. P. (2022). Analyzing the Effects of Parameters for Tremor Modulation via Phase-Locked Electrical Stimulation on a Peripheral Nerve. Journal of Personalized Medicine, 12 (1), 76–91. doi: https://doi.org/10.3390/jpm12010076
- Prasol, I. V., Yeroshenko, О. A. (2022). Modeling the electrical stimulation intensity dependence on stimulus frequency. Radiotekhnika, 209, 192–199. doi: https://doi.org/10.30837/rt.2022.2.209.19
- Gobbo, M., Maffiuletti, N. A., Orizio, C., Minetto, M. A. (2014). Muscle motor point identification is essential for optimizing neuromuscular electrical stimulation use. Journal of NeuroEngineering and Rehabilitation, 11 (1). doi: https://doi.org/10.1186/1743-0003-11-17
- Gorgey, A. S., Mahoney, E., Kendall, T., Dudley, G. A. (2006). Effects of neuromuscular electrical stimulation parameters on specific tension. European Journal of Applied Physiology, 97 (6), 737–744. doi: https://doi.org/10.1007/s00421-006-0232-7
- Dolbow, D. R., Gorgey, A. S., Sutor, T. W., Bochkezanian, V., Musselman, K. (2021). Invasive and Non-Invasive Approaches of Electrical Stimulation to Improve Physical Functioning after Spinal Cord Injury. Journal of Clinical Medicine, 10 (22), 5356. doi: https://doi.org/10.3390/jcm10225356
- Prasol, I., Dovnar, O., Yeroshenko, O. (2022). Method of Diagnostic Parameters Analysis and Software Features. 2022 IEEE 3rd KhPI Week on Advanced Technology (KhPIWeek). doi: https://doi.org/10.1109/khpiweek57572.2022.9916500
- Pascual-Valdunciel, A., Lopo-Martinez, V., Sendra-Arranz, R., Gonzalez-Sanchez, M., Perez-Sanchez, J. R., Grandas, F. et al. (2022). Prediction of Pathological Tremor Signals Using Long Short-Term Memory Neural Networks. IEEE Journal of Biomedical and Health Informatics, 26 (12), 5930–5941. doi: https://doi.org/10.1109/jbhi.2022.3209316
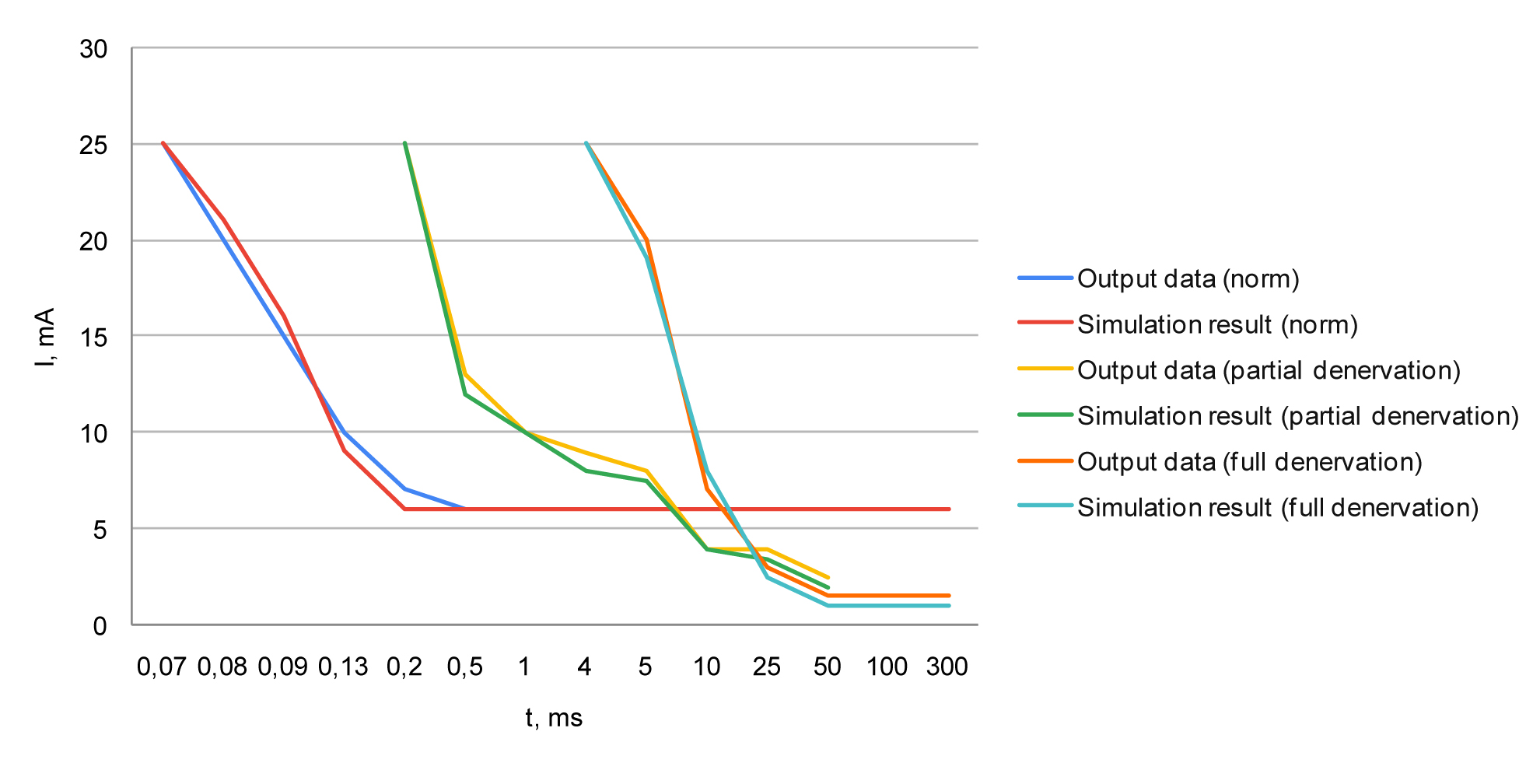
Downloads
Published
How to Cite
Issue
Section
License
Copyright (c) 2023 Igor Prasol, Olexandr Dovnar, Olha Yeroshenko

This work is licensed under a Creative Commons Attribution 4.0 International License.
The consolidation and conditions for the transfer of copyright (identification of authorship) is carried out in the License Agreement. In particular, the authors reserve the right to the authorship of their manuscript and transfer the first publication of this work to the journal under the terms of the Creative Commons CC BY license. At the same time, they have the right to conclude on their own additional agreements concerning the non-exclusive distribution of the work in the form in which it was published by this journal, but provided that the link to the first publication of the article in this journal is preserved.