Development of mathematical models to support decision-making regarding the functioning of critical infrastructure in the industry of energy supply
DOI:
https://doi.org/10.15587/2706-5448.2023.293205Keywords:
critical infrastructure, mathematical models, decision-support system, threats, critical situationAbstract
The object of research is the energy supply company and the processes of generation and supply of electric energy. The paper examines the problems of building mathematical models for forecasting the operation of a critical infrastructure object in the conditions of a changing security environment, characterized by unpredictability, the presence of uncertainties of various types, the appearance of new threats, their combinations, changes in the form, duration, nature of their influence. In the work, the main attention is paid to the study of the functioning of critical infrastructure in the field of energy supply. Based on the study of the functioning of the energy company system, methods of dealing with uncertainties at the stage of data preparation for analysis and during the preliminary construction of models are presented, in particular, statistical and probabilistic approaches, modeling of the studied processes, alternative methods of estimating model parameters, etc. The complexity of preparing the input data set is related to the fact that it is necessary to ensure the representativeness and variability of the data sets, given that a significant number of factors must be included in the model according to the requirements of regulatory documents, which can lead to multicollinearity of the input variables. The paper proposes an analytical toolkit based on the use of mathematical models and their combinations, intended for use in specialized decision support systems. In the course of the research, a number of numerical experiments were conducted, in which the proposed methodology was worked out on the materials of the enterprise – the object of the critical infrastructure of the energy sector. SAS Energy Forecasting software was used to build the models. The best forecasting results are obtained using generalized linear models (GLM), in particular the GLM model in the form of ARIMAX (a moving average autoregressive model that includes an integrated trend component and external regressors). The proposals presented in the work will allow to increase the efficiency of the functioning of the energy sector, including the determination of the goals, tasks and benchmarks of its operation in regular mode for certain periods of time, as well as in the field of development of universal and special mechanisms for ensuring stability in the mode of response to threats and critical situations.
References
- Pro krytychnu infrastrukturu (2021). Zakon Ukrainy No. 1882-IX. 16.11.2021. Available at: https://zakon.rada.gov.ua/laws/show/1882-20#Text
- Na Zakarpatti zapustyly Sytuatsiino-analitychnyi tsentr (2022). Available at: https://carpathia.gov.ua/news/na-zakarpatti-zapustili-situacijno-analitichnij-centr
- Sait Ministerstva tsyfrovoi transformatsii Ukrainy. Available at: https://thedigital.gov.ua
- Informatsiino-analitychna systema «SOTA». Available at: https://www.rnbo.gov.ua/ua/COTA.html
- United Nations Office for Disaster Risk Reduction (UNDRR). Available at: https://www.undrr.org/#
- Centrefor Research on the Epidemiology of Disasters (CRED). Available at: https://www.cred.be
- The International Disasters Database (EM-DAT). Available at: https://www.emdat.be
- «Ievropeiskyi dosvid rozbudovy systemy zakhystu krytychnoi infrastruktury: uroky dlia Ukrainy». Analitychna zapyska (2013). Available at: https://niss.gov.ua/doslidzhennya/nacionalna-bezpeka/evropeyskiy-dosvid-rozbudovi-sistemi-zakhistu-kritichnoi
- Stiikist zdiisnennia zhyttievo vazhlyvykh funktsii: uzahalnennia dosvidu reahuvannia Ukrainy na ruinuvannia enerhetychnoi infrastruktury (2023). Available at: https://niss.gov.ua/doslidzhennya/natsionalna-bezpeka/stiykist-zdiysnennya-zhyttyevo-vazhlyvykh-funktsiy-uzahalnennya
- Pederson, P., Dudenhoeffer, D., Hartley, S., Permann, M. (2006). Critical In frastructure Interdependency Modeling (CIMS). National Laboratory Idaho Falls. Idaho, 126. Available at: http://cip.management.dal.ca/publications/Critical%20Infrastructure%20Interdependency%20Modeling.pdf
- High-level Advisory Bodyon Artificial Intelligence. United Nations. Available at: https://www.un.org/en/ai-advisory-body
- Shevchenko, A. I., Kondratenko, Yu. P., Bilokobylskyi, O. V., Lande, D. V., Vakulenko, M. O., Mintser, O. P. et al. (2022). Stratehiia rozvytku shtuchnoho intelektu v Ukraini na 2022–2030 rr. Artificial Intelligence, 1, 75–157. Available at: https://www.slyusar.kiev.ua/AI_2022-1-1_ua.pdf
- SAS EnergyForecasting. Available at: https://www.sas.com/en_us/software/energy-forecasting.html
- Kaptein, M., Vanden, H. E. (2022). Statistics fo rData Scientists. Cham: Springer, 321. doi: https://doi.org/10.1007/978-3-030-10531-0
- Fomby, T. (2008). The Unobservable Components Model. Southern Methodist University Dallas, 42. Available at: https://s2.smu.edu/tfomby/eco5375/data/Unobservable%20Components%20Models/The%20Unobservable%20Components%20Model.pdf
- Blaine, B. (2023). Introductory Applied Statistics. Cham: Springer, 190. doi: https://doi.org/10.1007/978-3-031-27741-2
- Kokonendji, C. C., Bonat, W. H., Abid, R. (2020). Tweedie regression models and its geometric sums for (semi-) continuous data. WIREs Computational Statistics, 13 (1). doi: https://doi.org/10.1002/wics.1496
- Terentiev, O. M. (2021). Modeli, metody ta informatsiini tekhnolohii prohnozuvannia neliniinykh nestatsionarnykh protsesiv v umovakh nevyznachenosti. Kyiv, 469. Available at: https://itgip.org/wp-content/uploads/2021/04/dis.pdf
- Hernández, L., Baladrón, C., Aguiar, J., Calavia, L., Carro, B., Sánchez-Esguevillas, A. et al. (2014). Artificial Neural Network for Short-Term Load Forecasting in Distribution Systems. Energies, 7 (3), 1576–1598. doi: https://doi.org/10.3390/en7031576
- Borra, S., Dey, N., Bhattacharyya, S., Bouhlel, M. S. (Eds.) (2019). Intelligent decision support systems. Berlin, Boston: DeGruyter, 183. doi: https://doi.org/10.1515/9783110621105
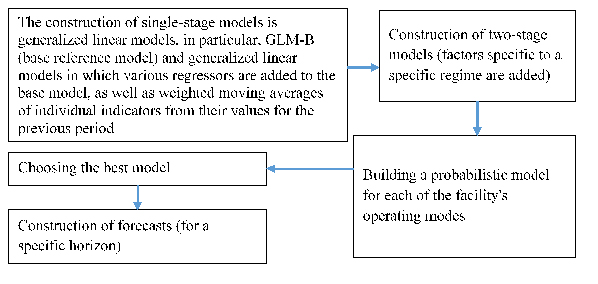
Downloads
Published
How to Cite
Issue
Section
License
Copyright (c) 2023 Oleksandr Terentiev, Tetyana Prosyankina-Zharova, Valerii Diakon, Roman Manuilenco

This work is licensed under a Creative Commons Attribution 4.0 International License.
The consolidation and conditions for the transfer of copyright (identification of authorship) is carried out in the License Agreement. In particular, the authors reserve the right to the authorship of their manuscript and transfer the first publication of this work to the journal under the terms of the Creative Commons CC BY license. At the same time, they have the right to conclude on their own additional agreements concerning the non-exclusive distribution of the work in the form in which it was published by this journal, but provided that the link to the first publication of the article in this journal is preserved.