Digital identification and pattern recognition capabilities using machine learning methods, navigation systems, and video surveillance
DOI:
https://doi.org/10.15587/2706-5448.2024.297044Keywords:
unmanned vehicle, convolutional neural networks, pattern recognition, machine learning, navigation, planning, video surveillanceAbstract
The objects of the study are unmanned vehicles and branches of the bridge of the city of Kyiv (Ukraine), which connects the Great Ring Road, Zhytomyr Highway and Peremogy Avenue. The built routes were analyzed using the technology of recognition of road signs, people and vehicles. The important problem of this research is to analyze the possibilities of detecting obstacles by an unmanned vehicle using pattern recognition, which combines the methods of machine communication, navigation and real-time video surveillance.
Based on the study, the results of detecting and avoiding obstacles on the road, where a study was conducted to investigate the main reasons that can cause time delays (traffic jams, weather conditions, accidents). The results of planning and navigation are obtained to determine the appropriate road route, which allows detecting and eliminating obstacles on the road, as well as building a map plan of the route in advance using online map services (Google Maps). It is shown that recognition of road signs (based on the classification using a road sign map consisting of 7 categories), people and vehicles minimizes the occurrence of road accidents, traffic jams and time delays. To recognize the images of road signs, people and vehicles, we studied the road sections connecting to the branched bridge.
Thus, the authors have reviewed and analyzed the digital capabilities of pattern identification and recognition using machine learning methods, navigation and video surveillance systems, where the safety of vehicles with detection of road signs and obstacles on the way is of great importance. The results obtained can complement the possibilities of using unmanned vehicles to avoid obstacles and road accidents based on a trained pattern recognition system. This system, using convolutional neural networks and video surveillance navigation systems, will be able to provide the driver and the people around it with safe driving conditions.
References
- Rezwan, S., Choi, W. (2022). Artificial Intelligence Approaches for UAV Navigation: Recent Advances and Future Challenges. IEEE Access, 10, 26320–26339. doi: https://doi.org/10.1109/access.2022.3157626
- Ni, J., Chen, Y., Chen, Y., Zhu, J., Ali, D., Cao, W. (2020). A Survey on Theories and Applications for Self-Driving Cars Based on Deep Learning Methods. Applied Sciences, 10 (8), 2749. doi: https://doi.org/10.3390/app10082749
- Kamble, S. J., Kounte, M. R. (2020). Machine Learning Approach on Traffic Congestion Monitoring System in Internet of Vehicles. Procedia Computer Science, 171, 2235–2241. doi: https://doi.org/10.1016/j.procs.2020.04.241
- Bachute, M. R., Subhedar, J. M. (2021). Autonomous Driving Architectures: Insights of Machine Learning and Deep Learning Algorithms. Machine Learning with Applications, 6, 100164. doi: https://doi.org/10.1016/j.mlwa.2021.100164
- Ali, K. S., Abid, N. M. (2021). The Importance of Google Maps for Traffic in Calculating the Level of Service for the Road and Traffic Delay. IOP Conference Series: Materials Science and Engineering, 1076 (1), 012015. doi: https://doi.org/10.1088/1757-899x/1076/1/012015
- Gupta, A., Anpalagan, A., Guan, L., Khwaja, A. S. (2021). Deep learning for object detection and scene perception in self-driving cars: Survey, challenges, and open issues. Array, 10, 100057. doi: https://doi.org/10.1016/j.array.2021.100057
- Benito-Picazo, J., Domínguez, E., Palomo, E. J., López-Rubio, E. (2020). Deep learning-based video surveillance system managed by low cost hardware and panoramic cameras. Integrated Computer-Aided Engineering, 27 (4), 373–387. doi: https://doi.org/10.3233/ica-200632
- Duarte, F. (2019). Self-driving cars: A city perspective. Science Robotics, 4 (28). doi: https://doi.org/10.1126/scirobotics.aav9843
- Hasan, N., Anzum, T., Jahan, N. (2021). Traffic sign recognition system (TSRS): SVM and convolutional neural network. Inventive Communication and Computational Technologies: Proceedings of ICICCT 2020. Springer, 69–79.
- Holovatsky, I. V., Kornaha, Yа. I. (2019). Intellectual system of recognition of road elements. Scientific Notes of Taurida National V. I. Vernadsky University. Series: Technical Sciences, 6 (1), 47–50. doi: https://doi.org/10.32838/2663-5941/2019.6-1/09
- Yan, C., Jiang, H., Zhang, B., Coenen, F. (2015). Recognizing driver inattention by convolutional neural networks. 2015 8th International Congress on Image and Signal Processing (CISP), 680–685. doi: https://doi.org/10.1109/cisp.2015.7407964
- Derrow-Pinion, A., She, J., Wong, D., Lange, O., Hester, T., Perez, L. et al. (2021). ETA Prediction with Graph Neural Networks in Google Maps. Proceedings of the 30th ACM International Conference on Information & Knowledge Management, 3767–3776. doi: https://doi.org/10.1145/3459637.3481916
- Badrloo, S., Varshosaz, M., Pirasteh, S., Li, J. (2022). Image-Based Obstacle Detection Methods for the Safe Navigation of Unmanned Vehicles: A Review. Remote Sensing, 14 (15), 3824. doi: https://doi.org/10.3390/rs14153824
- O’Mahony, N., Campbell, S., Krpalkova, L., Riordan, D., Walsh, J., Murphy, A., Ryan, C. (2018). Deep Learning for Visual Navigation of Unmanned Ground Vehicles : A review. 2018 29th Irish Signals and Systems Conference (ISSC). doi: https://doi.org/10.1109/issc.2018.8585381
- Konovalov, O. Yu. (2021). Rozrobka pidsystemy rozpiznavannia dorozhnikh znakiv avtomobilnoho avtopilota. Kharkiv, 82.
- Kanagaraj, N., Hicks, D., Goyal, A., Tiwari, S., Singh, G. (2021). Deep learning using computer vision in self driving cars for lane and traffic sign detection. International Journal of System Assurance Engineering and Management, 12 (6), 1011–1025. doi: https://doi.org/10.1007/s13198-021-01127-6
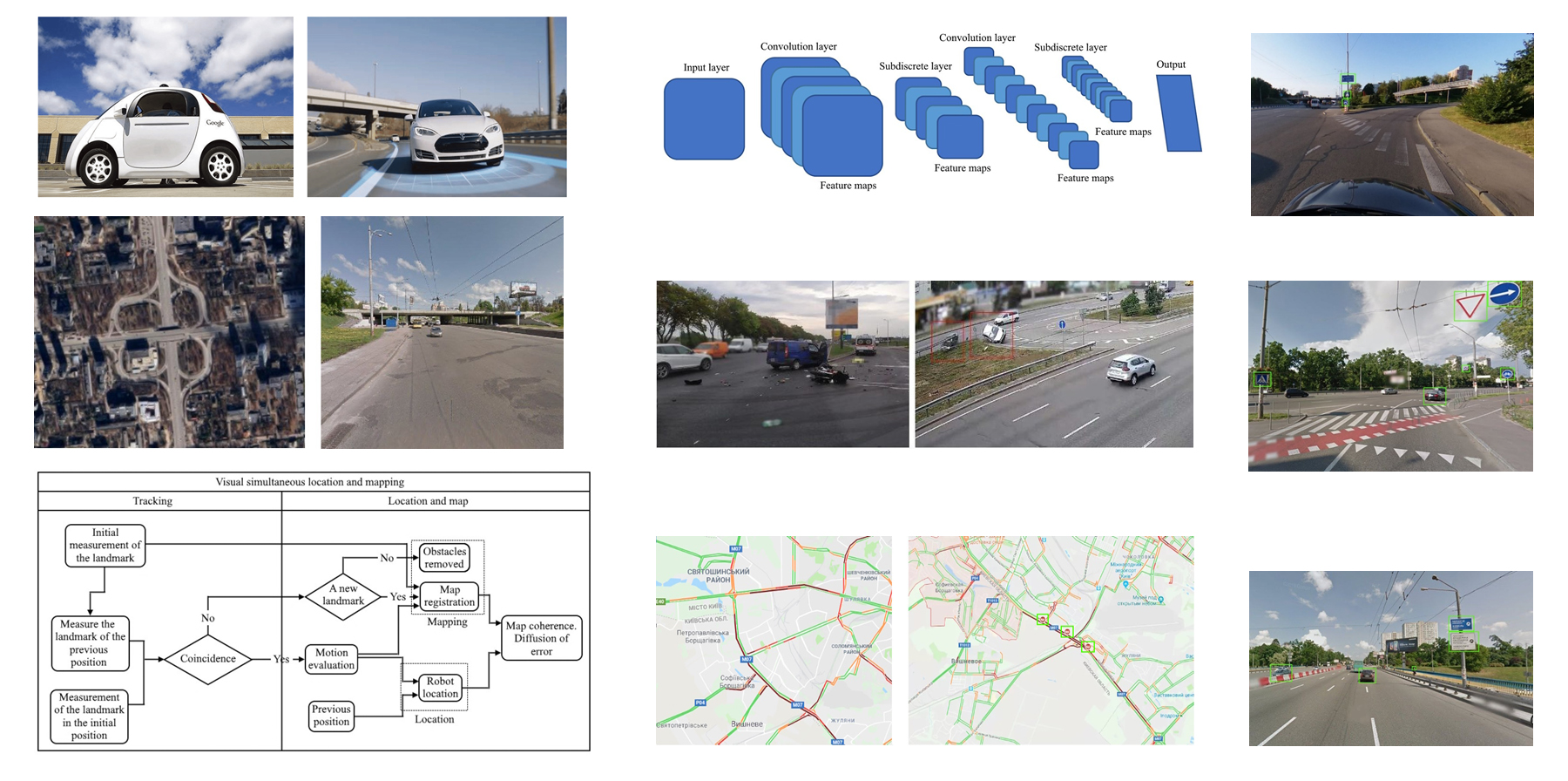
Downloads
Published
How to Cite
Issue
Section
License
Copyright (c) 2024 Olena Marchenko, Oleksandr Viunenko, Ihor Nechai

This work is licensed under a Creative Commons Attribution 4.0 International License.
The consolidation and conditions for the transfer of copyright (identification of authorship) is carried out in the License Agreement. In particular, the authors reserve the right to the authorship of their manuscript and transfer the first publication of this work to the journal under the terms of the Creative Commons CC BY license. At the same time, they have the right to conclude on their own additional agreements concerning the non-exclusive distribution of the work in the form in which it was published by this journal, but provided that the link to the first publication of the article in this journal is preserved.