Assessing the potential of artificial intelligence and machine learning for thermal management in electronic devices
DOI:
https://doi.org/10.15587/2706-5448.2025.323117Keywords:
artificial intelligence (AI), machine learning (ML), thermal management, semiconductor thermal dissipation, predictive modelling, energy-efficient computingAbstract
The object of this study is the potential of artificial intelligence (AI) and machine learning (ML) techniques for thermal management in electronic devices. One of the most problematic aspects identified is the challenge of ensuring performance, reliability, and energy efficiency across diverse systems, including semiconductors, data centers, and consumer electronics. In the course of the research, the Preferred Reporting Items for Systematic Reviews and Meta-Analyses (PRISMA) methodology was used to systematically analyze 150 studies. These studies employed various approaches, such as predictive modeling, optimization algorithms, and real-time control systems.
Our findings indicate that AI-driven thermal management can reduce energy consumption by up to 81.81 %, depending on the cooling method and optimization. Reinforcement learning-based HVAC control achieves 17.4 % energy savings, while ML-driven power management in manycore systems reduces energy use by 30 % and lowers peak chip temperatures by 17 °C. Neural network-based thermal forecasting achieves <1 % error, improving prediction accuracy. Additionally, LSTM models for thermal prognosis achieve a 3.45 % relative prediction error, outperforming traditional regression methods.
These results highlight the potential of AI in optimizing thermal behavior across data centers, smart buildings, and manycore chip architectures. Key limitations were also identified, including limited data availability, challenges in model interpretability, and integration with legacy systems. The study provides a roadmap for scalable AI-driven thermal management. Emerging trends such as physics-informed ML models and the integration of cooling technologies promise innovation. Compared to conventional methods, these advancements deliver clear benefits in sustainability and adaptability.
References
- Wang, Y., Gao, Q., Wang, G., Lu, P., Zhao, M., Bao, W. (2018). A review on research status and key technologies of battery thermal management and its enhanced safety. International Journal of Energy Research, 42 (13), 4008–4033. https://doi.org/10.1002/er.4158
- Fan, S., Duan, F. (2020). A review of two-phase submerged boiling in thermal management of electronic cooling. International Journal of Heat and Mass Transfer, 150, 119324. https://doi.org/10.1016/j.ijheatmasstransfer.2020.119324
- Feng, C.-P., Chen, L.-B., Tian, G.-L., Wan, S.-S., Bai, L., Bao, R.-Y. et al. (2019). Multifunctional Thermal Management Materials with Excellent Heat Dissipation and Generation Capability for Future Electronics. ACS Applied Materials & Interfaces, 11 (20), 18739–18745. https://doi.org/10.1021/acsami.9b03885
- Zhang, N. (2024). Diagnosis and Prevention of Overheating Failures in Mechanical Equipment Based on Numerical Analysis of Temperature and Thermal Stress Fields. International Journal of Heat and Technology, 42 (2), 466–474. https://doi.org/10.18280/ijht.420212
- Hu, R., Liu, Y., Shin, S., Huang, S., Ren, X., Shu, W. et al. (2020). Emerging Materials and Strategies for Personal Thermal Management. Advanced Energy Materials, 10 (17). https://doi.org/10.1002/aenm.201903921
- Khosla, R., Miranda, N. D., Trotter, P. A., Mazzone, A., Renaldi, R., McElroy, C. et al. (2020). Cooling for sustainable development. Nature Sustainability, 4 (3), 201–208. https://doi.org/10.1038/s41893-020-00627-w
- Syu, J.-H., Lin, J. C.-W., Srivastava, G., Yu, K. (2023). A Comprehensive Survey on Artificial Intelligence Empowered Edge Computing on Consumer Electronics. IEEE Transactions on Consumer Electronics, 69 (4), 1023–1034. https://doi.org/10.1109/tce.2023.3318150
- Nazir, K., Memon, S. A., Saurbayeva, A. (2024). A novel framework for developing a machine learning-based forecasting model using multi-stage sensitivity analysis to predict the energy consumption of PCM-integrated building. Applied Energy, 376, 124180. https://doi.org/10.1016/j.apenergy.2024.124180
- Mathew, J., Krishnan, S. (2021). A Review on Transient Thermal Management of Electronic Devices. Journal of Electronic Packaging, 144 (1). https://doi.org/10.1115/1.4050002
- He, Z., Yan, Y., Zhang, Z. (2021). Thermal management and temperature uniformity enhancement of electronic devices by micro heat sinks: A review. Energy, 216, 119223. https://doi.org/10.1016/j.energy.2020.119223
- Kucova, T., Prauzek, M., Konecny, J., Andriukaitis, D., Zilys, M., Martinek, R. (2023). Thermoelectric energy harvesting for internet of things devices using machine learning: A review. CAAI Transactions on Intelligence Technology, 8 (3), 680–700. https://doi.org/10.1049/cit2.12259
- Chuttar, A., Thyagarajan, A., Banerjee, D. (2021). Leveraging Machine Learning (Artificial Neural Networks) for Enhancing Performance and Reliability of Thermal Energy Storage Platforms Utilizing Phase Change Materials. Journal of Energy Resources Technology, 144 (2). https://doi.org/10.1115/1.4051048
- Tang, X., Guo, Q., Li, M., Wei, C., Pan, Z., Wang, Y. (2021). Performance analysis on liquid-cooled battery thermal management for electric vehicles based on machine learning. Journal of Power Sources, 494, 229727. https://doi.org/10.1016/j.jpowsour.2021.229727
- Putrada, A. G., Abdurohman, M., Perdana, D., Nuha, H. H. (2022). Machine Learning Methods in Smart Lighting Toward Achieving User Comfort: A Survey. IEEE Access, 10, 45137–45178. https://doi.org/10.1109/access.2022.3169765
- Rane, N., Choudhary, S., Rane, J. (2023). Enhancing thermal comfort through leading-edge design, monitoring, and optimization technologies: A review. Sustainable and Clean Buildings, 1 (1), 123–146. https://doi.org/10.2139/ssrn.4642529
- Panduman, Y. Y. F., Funabiki, N., Fajrianti, E. D., Fang, S., Sukaridhoto, S. (2024). A Survey of AI Techniques in IoT Applications with Use Case Investigations in the Smart Environmental Monitoring and Analytics in Real-Time IoT Platform. Information, 15 (3), 153. https://doi.org/10.3390/info15030153
- Khan, S. A., Eze, C., Dong, K., Shahid, A. R., Patil, M. S., Ahmad, S. et al. (2022). Design of a new optimized U-shaped lightweight liquid-cooled battery thermal management system for electric vehicles: A machine learning approach. International Communications in Heat and Mass Transfer, 136, 106209. https://doi.org/10.1016/j.icheatmasstransfer.2022.106209
- Zhou, Y., Zheng, S., Zhang, G. (2020). A review on cooling performance enhancement for phase change materials integrated systems – flexible design and smart control with machine learning applications. Building and Environment, 174, 106786. https://doi.org/10.1016/j.buildenv.2020.106786
- Rabienataj Darzi, A. A., Mousavi, S. M., Razbin, M., Li, M. (2024). Utilizing neural networks and genetic algorithms in AI-assisted CFD for optimizing PCM-based thermal energy storage units with extended surfaces. Thermal Science and Engineering Progress, 54, 102795. https://doi.org/10.1016/j.tsep.2024.102795
- Xin, X., Zhang, Z., Zhou, Y., Liu, Y., Wang, D., Nan, S. (2024). A comprehensive review of predictive control strategies in heating, ventilation, and air-conditioning (HVAC): Model-free VS model. Journal of Building Engineering, 94, 110013. https://doi.org/10.1016/j.jobe.2024.110013
- Suryawanshi, H. A. (2024). Predictions of thermal behavior of power electronics components with neural networks. [PhD thesis; Technische Hochschule Ingolstadt].
- Pei, Q., Chen, S., Zhang, Q., Zhu, X., Liu, F., Jia, Z. et al. (2022). CoolEdge: hotspot-relievable warm water cooling for energy-efficient edge datacenters. Proceedings of the 27th ACM International Conference on Architectural Support for Programming Languages and Operating Systems, 814–829. https://doi.org/10.1145/3503222.3507713
- Antal, M., Cioara, T., Anghel, I., Gorzenski, R., Januszewski, R., Oleksiak, A. et al. (2019). Reuse of Data Center Waste Heat in Nearby Neighborhoods: A Neural Networks-Based Prediction Model. Energies, 12 (5), 814. https://doi.org/10.3390/en12050814
- Sohel Murshed, S. M., Nieto de Castro, C. A. (2017). A critical review of traditional and emerging techniques and fluids for electronics cooling. Renewable and Sustainable Energy Reviews, 78, 821–833. https://doi.org/10.1016/j.rser.2017.04.112
- Chakraborty, S., Shukla, D., Kumar Panigrahi, P. (2024). A review on coolant selection for thermal management of electronics and implementation of multiple-criteria decision-making approach. Applied Thermal Engineering, 245, 122807. https://doi.org/10.1016/j.applthermaleng.2024.122807
- Schreiber, T., Netsch, C., Eschweiler, S., Wang, T., Storek, T., Baranski, M., Müller, D. (2021). Application of data-driven methods for energy system modelling demonstrated on an adaptive cooling supply system. Energy, 230, 120894. https://doi.org/10.1016/j.energy.2021.120894
- Guo, C. Y., Muhieldeen, M. W., Teng, K. H., Ang, C. K., Lim, W. H. (2024). A novel thermal management system for lithium-ion battery modules combining indirect liquid-cooling with forced air-cooling: Deep learning approach. Journal of Energy Storage, 94, 112434. https://doi.org/10.1016/j.est.2024.112434
- Pagani, S., Manoj, P. D. S., Jantsch, A., Henkel, J. (2020). Machine Learning for Power, Energy, and Thermal Management on Multicore Processors: A Survey. IEEE Transactions on Computer-Aided Design of Integrated Circuits and Systems, 39 (1), 101–116. https://doi.org/10.1109/tcad.2018.2878168
- Ilager, S., Buyya, R. (2021). Energy and thermal-aware resource management of cloud data centres: A taxonomy and future directions. arXiv preprint arXiv:2107.02342
- He, Z., Guo, W., Zhang, P. (2022). Performance prediction, optimal design and operational control of thermal energy storage using artificial intelligence methods. Renewable and Sustainable Energy Reviews, 156, 111977. https://doi.org/10.1016/j.rser.2021.111977
- Chuttar, A., Banerjee, D. (2021). Machine Learning (ML) Based Thermal Management for Cooling of Electronics Chips by Utilizing Thermal Energy Storage (TES) in Packaging That Leverages Phase Change Materials (PCM). Electronics, 10 (22), 2785. https://doi.org/10.3390/electronics10222785
- Amiraski, M., Werner, D., Hankin, A., Sebot, J., Vaidyanathan, K., Hempstead, M. (2023). Boreas: A Cost-Effective Mitigation Method for Advanced Hotspots using Machine Learning and Hardware Telemetry. 2023 IEEE International Symposium on Performance Analysis of Systems and Software (ISPASS), 295–305. https://doi.org/10.1109/ispass57527.2023.00036
- Okulu, D., Selimefendigil, F., Öztop, H. F. (2022). Review on nanofluids and machine learning applications for thermoelectric energy conversion in renewable energy systems. Engineering Analysis with Boundary Elements, 144, 221–261. https://doi.org/10.1016/j.enganabound.2022.08.004
- Blad, C., Bøgh, S., Kallesøe, C. S. (2022). Data-driven Offline Reinforcement Learning for HVAC-systems. Energy, 261, 125290. https://doi.org/10.1016/j.energy.2022.125290
- Correa-Jullian, C., Cardemil, J. M., López Droguett, E., Behzad, M. (2020). Assessment of Deep Learning techniques for Prognosis of solar thermal systems. Renewable Energy, 145, 2178–2191. https://doi.org/10.1016/j.renene.2019.07.100
- Patel, N., Krishnamurthy, P., Amrouch, H., Henkel, J., Shamouilian, M., Karri, R., Khorrami, F. (2022). Towards a New Thermal Monitoring Based Framework for Embedded CPS Device Security. IEEE Transactions on Dependable and Secure Computing, 19 (1), 524–536. https://doi.org/10.1109/tdsc.2020.2973959
- Zhang, Q., Meng, Z., Hong, X., Zhan, Y., Liu, J., Dong, J. et al. (2021). A survey on data center cooling systems: Technology, power consumption modeling and control strategy optimization. Journal of Systems Architecture, 119, 102253. https://doi.org/10.1016/j.sysarc.2021.102253
- Chen, W.-Y., Shi, X.-L., Zou, J., Chen, Z.-G. (2022). Thermoelectric coolers for on-chip thermal management: Materials, design, and optimization. Materials Science and Engineering: R: Reports, 151, 100700. https://doi.org/10.1016/j.mser.2022.100700
- Habibi Khalaj, A., Halgamuge, S. K. (2017). A Review on efficient thermal management of air- and liquid-cooled data centers: From chip to the cooling system. Applied Energy, 205, 1165–1188. https://doi.org/10.1016/j.apenergy.2017.08.037
- Hannan, M. A., Lipu, M. S. H., Ker, P. J., Begum, R. A., Agelidis, V. G., Blaabjerg, F. (2019). Power electronics contribution to renewable energy conversion addressing emission reduction: Applications, issues, and recommendations. Applied Energy, 251, 113404. https://doi.org/10.1016/j.apenergy.2019.113404
- Alzoubi, Y. I., Mishra, A. (2024). Green artificial intelligence initiatives: Potentials and challenges. Journal of Cleaner Production, 468, 143090. https://doi.org/10.1016/j.jclepro.2024.143090
- Yahia, H. S., Zeebaree, S. R. M., Sadeeq, M. A. M., Salim, N. O. M., Kak, S. F., AL-Zebari, A. et al. (2021). Comprehensive Survey for Cloud Computing Based Nature-Inspired Algorithms Optimization Scheduling. Asian Journal of Research in Computer Science, 8 (2), 1–16. https://doi.org/10.9734/ajrcos/2021/v8i230195
- Benoit-Cattin, T., Velasco-Montero, D., Fernández-Berni, J. (2020). Impact of Thermal Throttling on Long-Term Visual Inference in a CPU-Based Edge Device. Electronics, 9 (12), 2106. https://doi.org/10.3390/electronics9122106
- Dhameliya, N. (2022). Power Electronics Innovations: Improving Efficiency and Sustainability in Energy Systems. Asia Pacific Journal of Energy and Environment, 9 (2), 71–80. https://doi.org/10.18034/apjee.v9i2.752
- Iranfar, A., Terraneo, F., Csordas, G., Zapater, M., Fornaciari, W., Atienza, D. (2020). Dynamic Thermal Management with Proactive Fan Speed Control Through Reinforcement Learning. 2020 Design, Automation & Test in Europe Conference & Exhibition (DATE), 418–423. https://doi.org/10.23919/date48585.2020.9116510
- Zhang, X., Wu, Z., Sun, Q., Gu, W., Zheng, S., Zhao, J. (2024). Application and progress of artificial intelligence technology in the field of distribution network voltage Control: A review. Renewable and Sustainable Energy Reviews, 192, 114282. https://doi.org/10.1016/j.rser.2024.114282
- Devasenan, M., Madhavan, S. (2024). Thermal intelligence: exploring AI’s role in optimizing thermal systems – a review. Interactions, 245 (1). https://doi.org/10.1007/s10751-024-02122-6
- Zhuang, D., Gan, V. J. L., Duygu Tekler, Z., Chong, A., Tian, S., Shi, X. (2023). Data-driven predictive control for smart HVAC system in IoT-integrated buildings with time-series forecasting and reinforcement learning. Applied Energy, 338, 120936. https://doi.org/10.1016/j.apenergy.2023.120936
- Shaban, W. M., Kabeel, A. E., El Hadi Attia, M., Talaat, F. M. (2024). Optimizing photovoltaic thermal solar systems efficiency through advanced artificial intelligence driven thermal management techniques. Applied Thermal Engineering, 247, 123029. https://doi.org/10.1016/j.applthermaleng.2024.123029
- Jalasri, M., Panchal, S. M., Mahalingam, K., Venkatasubramanian, R., Hemalatha, R., Boopathi, S. (2024). AI-Powered Smart Energy Management for Optimizing Energy Efficiency in High-Performance Computing Systems. Future of Digital Technology and AI in Social Sectors. IGI Global, 329–366. https://doi.org/10.4018/979-8-3693-5533-6.ch012
- Zhang, K., Guliani, A., Ogrenci-Memik, S., Memik, G., Yoshii, K., Sankaran, R., Beckman, P. (2018). Machine Learning-Based Temperature Prediction for Runtime Thermal Management Across System Components. IEEE Transactions on Parallel and Distributed Systems, 29 (2), 405–419. https://doi.org/10.1109/tpds.2017.2732951
- Han, Z., Zhao, J., Leung, H., Ma, K. F., Wang, W. (2021). A Review of Deep Learning Models for Time Series Prediction. IEEE Sensors Journal, 21 (6), 7833–7848. https://doi.org/10.1109/jsen.2019.2923982
- Krivoguz, D., Ioshpa, A., Chernyi, S., Zhilenkov, A., Kustov, A., Zinchenko, A. (2024). Enhancing long-term air temperature forecasting with deep learning architectures. Journal of Robotics and Control, 5 (3), 706–716.
- Ghahramani, A., Galicia, P., Lehrer, D., Varghese, Z., Wang, Z., Pandit, Y. (2020). Artificial Intelligence for Efficient Thermal Comfort Systems: Requirements, Current Applications and Future Directions. Frontiers in Built Environment, 6. https://doi.org/10.3389/fbuil.2020.00049
- Zou, F., Yen, G. G., Tang, L., Wang, C. (2021). A reinforcement learning approach for dynamic multi-objective optimization. Information Sciences, 546, 815–834. https://doi.org/10.1016/j.ins.2020.08.101
- Du, Y., Zhou, Z., Yang, X., Yang, X., Wang, C., Liu, J., Yuan, J. (2023). Dynamic thermal environment management technologies for data center: A review. Renewable and Sustainable Energy Reviews, 187, 113761. https://doi.org/10.1016/j.rser.2023.113761
- Arshad, R., Zahoor, S., Shah, M. A., Wahid, A., Yu, H. (2017). Green IoT: An Investigation on Energy Saving Practices for 2020 and Beyond. IEEE Access, 5, 15667–15681. https://doi.org/10.1109/access.2017.2686092
- Halhoul Merabet, G., Essaaidi, M., Ben Haddou, M., Qolomany, B., Qadir, J., Anan, M. et al. (2021). Intelligent building control systems for thermal comfort and energy-efficiency: A systematic review of artificial intelligence-assisted techniques. Renewable and Sustainable Energy Reviews, 144, 110969. https://doi.org/10.1016/j.rser.2021.110969
- Emara, R. A. M. O. (2024). Artificial Intelligence Based Controller for a Temperature Control System. [PhD thesis; Faculty of Engineering, The British University in Egypt].
- Cox, S. J., Kim, D., Cho, H., Mago, P. (2019). Real time optimal control of district cooling system with thermal energy storage using neural networks. Applied Energy, 238, 466–480. https://doi.org/10.1016/j.apenergy.2019.01.093
- Gill, S. S., Xu, M., Ottaviani, C., Patros, P., Bahsoon, R., Shaghaghi, A. et al. (2022). AI for next generation computing: Emerging trends and future directions. Internet of Things, 19, 100514. https://doi.org/10.1016/j.iot.2022.100514
- Datta, S., Chakraborty, W., Radosavljevic, M. (2022). Toward attojoule switching energy in logic transistors. Science, 378 (6621), 733–740. https://doi.org/10.1126/science.ade7656
- Bahru, R., Hamzah, A. A., Mohamed, M. A. (2020). Thermal management of wearable and implantable electronic healthcare devices: Perspective and measurement approach. International Journal of Energy Research, 45 (2), 1517–1534. https://doi.org/10.1002/er.6031
- Mallick, S., Gayen, D. (2023). Thermal behaviour and thermal runaway propagation in lithium-ion battery systems – A critical review. Journal of Energy Storage, 62, 106894. https://doi.org/10.1016/j.est.2023.106894
- Zhao, J., Feng, X., Tran, M.-K., Fowler, M., Ouyang, M., Burke, A. F. (2024). Battery safety: Fault diagnosis from laboratory to real world. Journal of Power Sources, 598, 234111. https://doi.org/10.1016/j.jpowsour.2024.234111
- Hanafi, A., Moawed, M., Abdellatif, O. (2024). Advancing Sustainable Energy Management: A Comprehensive Review of Artificial Intelligence Techniques in Building. Engineering Research Journal (Shoubra), 53 (2), 26–46. https://doi.org/10.21608/erjsh.2023.226854.1196
- Tong, X. C. (2010). Development and Application of Advanced Thermal Management Materials. Advanced Materials for Thermal Management of Electronic Packaging, 527–593. https://doi.org/10.1007/978-1-4419-7759-5_12
- Van Quang, T., Doan, D. T., Yun, G. Y. (2024). Recent advances and effectiveness of machine learning models for fluid dynamics in the built environment. International Journal of Modelling and Simulation, 1–27. https://doi.org/10.1080/02286203.2024.2371682
- Hu, G., Prasianakis, N., Churakov, S. V., Pfingsten, W. (2024). Performance analysis of data-driven and physics-informed machine learning methods for thermal-hydraulic processes in Full-scale Emplacement experiment. Applied Thermal Engineering, 245, 122836. https://doi.org/10.1016/j.applthermaleng.2024.122836
- Li, Z., Luo, H., Jiang, Y., Liu, H., Xu, L., Cao, K. et al. (2024). Comprehensive review and future prospects on chip-scale thermal management: Core of data center’s thermal management. Applied Thermal Engineering, 251, 123612. https://doi.org/10.1016/j.applthermaleng.2024.123612
- Wang, J., Zhao, T. (2024). Medium spatiotemporal characteristics based global optimization method for energy efficiency trade-off issue in variable flow rate HVAC system. Applied Thermal Engineering, 247, 123132. https://doi.org/10.1016/j.applthermaleng.2024.123132
- Chatterjee, A., Khovalyg, D. (2023). Dynamic indoor thermal environment using Reinforcement Learning-based controls: Opportunities and challenges. Building and Environment, 244, 110766. https://doi.org/10.1016/j.buildenv.2023.110766
- Boinapalli, N. R. (2020). Digital transformation in us industries: Ai as a catalyst for sustainable growth. NEXG AI Review of America, 1 (1), 70–84.
- Khan, O., Parvez, M., Seraj, M., Yahya, Z., Devarajan, Y., Nagappan, B. (2024). Optimising building heat load prediction using advanced control strategies and Artificial Intelligence for HVAC system. Thermal Science and Engineering Progress, 49, 102484. https://doi.org/10.1016/j.tsep.2024.102484
- Olabi, A. G., Abdelghafar, A. A., Maghrabie, H. M., Sayed, E. T., Rezk, H., Radi, M. A. et al. (2023). Application of artificial intelligence for prediction, optimization, and control of thermal energy storage systems. Thermal Science and Engineering Progress, 39, 101730. https://doi.org/10.1016/j.tsep.2023.101730
- Dinakarrao, S. M. P., Joseph, A., Haridass, A., Shafique, M., Henkel, J., Homayoun, H. (2019). Application and Thermal-reliability-aware Reinforcement Learning Based Multi-core Power Management. ACM Journal on Emerging Technologies in Computing Systems, 15 (4), 1–19. https://doi.org/10.1145/3323055
- Shahid, A., Plaum, F., Korõtko, T., Rosin, A. (2024). AI Technologies and Their Applications in Small-Scale Electric Power Systems. IEEE Access, 12, 109984–110001. https://doi.org/10.1109/access.2024.3440067
- Ahmad, T., Chen, H., Huang, R., Yabin, G., Wang, J., Shair, J. et al. (2018). Supervised based machine learning models for short, medium and long-term energy prediction in distinct building environment. Energy, 158, 17–32. https://doi.org/10.1016/j.energy.2018.05.169
- Wang, Z., Hong, T., Piette, M. A. (2020). Building thermal load prediction through shallow machine learning and deep learning. Applied Energy, 263, 114683. https://doi.org/10.1016/j.apenergy.2020.114683
- Hundi, P., Shahsavari, R. (2020). Comparative studies among machine learning models for performance estimation and health monitoring of thermal power plants. Applied Energy, 265, 114775. https://doi.org/10.1016/j.apenergy.2020.114775
- Xiao, P., Ni, Z., Liu, D., Hu, Z. (2021). A power and thermal-aware virtual machine management framework based on machine learning. Cluster Computing, 24 (3), 2231–2248. https://doi.org/10.1007/s10586-020-03228-6
- Lin, W., Wu, G., Wang, X., Li, K. (2020). An Artificial Neural Network Approach to Power Consumption Model Construction for Servers in Cloud Data Centers. IEEE Transactions on Sustainable Computing, 5 (3), 329–340. https://doi.org/10.1109/tsusc.2019.2910129
- Chen, K.-C., Liao, Y.-H., Chen, C.-T., Wang, L.-Q. (2023). Adaptive Machine Learning-Based Proactive Thermal Management for NoC Systems. IEEE Transactions on Very Large Scale Integration (VLSI) Systems, 31 (8), 1114–1127. https://doi.org/10.1109/tvlsi.2023.3282969
- Patel, K., Mehta, N., Oza, P., Thaker, J., Bhise, A. (2024). Revolutionizing Data Centre Sustainability: The Role of Machine Learning in Energy Efficiency. 2024 IEEE International Conference on Interdisciplinary Approaches in Technology and Management for Social Innovation (IATMSI), 1–6. https://doi.org/10.1109/iatmsi60426.2024.10503107
- Erhan, L., Ndubuaku, M., Di Mauro, M., Song, W., Chen, M., Fortino, G., Bagdasar, O., Liotta, A. (2021). Smart anomaly detection in sensor systems: A multi-perspective review. Information Fusion, 67, 64–79. https://doi.org/10.1016/j.inffus.2020.10.001
- Fan, C., Xiao, F., Li, Z., Wang, J. (2018). Unsupervised data analytics in mining big building operational data for energy efficiency enhancement: A review. Energy and Buildings, 159, 296–308. https://doi.org/10.1016/j.enbuild.2017.11.008
- Bueno, A. M., Mendes da Luz, I., Niza, I. L., Broday, E. E. (2023). Hierarchical and K-means clustering to assess thermal dissatisfaction and productivity in university classrooms. Building and Environment, 233, 110097. https://doi.org/10.1016/j.buildenv.2023.110097
- Surucu, O., Gadsden, S. A., Yawney, J. (2023). Condition Monitoring using Machine Learning: A Review of Theory, Applications, and Recent Advances. Expert Systems with Applications, 221, 119738. https://doi.org/10.1016/j.eswa.2023.119738
- Schneider, T., Bedrikow, A. B., Stahl, K. (2024). Enhanced prediction of thermomechanical systems using machine learning, PCA, and finite element simulation. Advanced Modeling and Simulation in Engineering Sciences, 11 (1). https://doi.org/10.1186/s40323-024-00268-0
- Zhou, X., Du, H., Xue, S., Ma, Z. (2024). Recent advances in data mining and machine learning for enhanced building energy management. Energy, 307, 132636. https://doi.org/10.1016/j.energy.2024.132636
- Zhu, S., Lu, W., Feng, Y., Sun, C. (2024). Energy Optimization for Building Energy Management with Thermal Storage: A Multi-Agent Deep Reinforcement Learning Approach. 2024 43rd Chinese Control Conference (CCC), 2805–2812. https://doi.org/10.23919/ccc63176.2024.10661808
- Zhang, Z., Lam, K. P. (2018). Practical implementation and evaluation of deep reinforcement learning control for a radiant heating system. Proceedings of the 5th Conference on Systems for Built Environments, 148–157. https://doi.org/10.1145/3276774.3276775
- Ortiz, Y., Arévalo, P., Peña, D., Jurado, F. (2024). Recent Advances in Thermal Management Strategies for Lithium-Ion Batteries: A Comprehensive Review. Batteries, 10 (3), 83. https://doi.org/10.3390/batteries10030083
- Vamvakas, D., Michailidis, P., Korkas, C., Kosmatopoulos, E. (2023). Review and Evaluation of Reinforcement Learning Frameworks on Smart Grid Applications. Energies, 16 (14), 5326. https://doi.org/10.3390/en16145326
- Shi, Z., Zheng, R., Zhao, J., Shen, R., Gu, L., Liu, Y. et al. (2024). Towards various occupants with different thermal comfort requirements: A deep reinforcement learning approach combined with a dynamic PMV model for HVAC control in buildings. Energy Conversion and Management, 320, 118995. https://doi.org/10.1016/j.enconman.2024.118995
- Ran, Y., Hu, H., Wen, Y., Zhou, X. (2023). Optimizing Energy Efficiency for Data Center via Parameterized Deep Reinforcement Learning. IEEE Transactions on Services Computing, 16 (2), 1310–1323. https://doi.org/10.1109/tsc.2022.3184835
- Chang, C.-W., Dinh, N. T. (2019). Classification of machine learning frameworks for data-driven thermal fluid models. International Journal of Thermal Sciences, 135, 559–579. https://doi.org/10.1016/j.ijthermalsci.2018.09.002
- Li, A., Weng, J., Yuen, A. C. Y., Wang, W., Liu, H., Lee, E. W. M. et al. (2023). Machine learning assisted advanced battery thermal management system: A state-of-the-art review. Journal of Energy Storage, 60, 106688. https://doi.org/10.1016/j.est.2023.106688
- Ababei, C., Moghaddam, M. G. (2019). A Survey of Prediction and Classification Techniques in Multicore Processor Systems. IEEE Transactions on Parallel and Distributed Systems, 30 (5), 1184–1200. https://doi.org/10.1109/tpds.2018.2878699
- Kirchgässner, W., Wallscheid, O., Böcker, J. (2023). Thermal neural networks: Lumped-parameter thermal modeling with state-space machine learning. Engineering Applications of Artificial Intelligence, 117, 105537. https://doi.org/10.1016/j.engappai.2022.105537
- Aparna, R., Idicula, S. M. (2022). Spatio-Temporal Data Clustering using Deep Learning: A Review. 2022 IEEE International Conference on Evolving and Adaptive Intelligent Systems (EAIS), 1–10. https://doi.org/10.1109/eais51927.2022.9787701
- Werner, D., Juretus, K., Savidis, I., Hempstead, M. (2018). Machine Learning on the Thermal Side-Channel: Analysis of Accelerator-Rich Architectures. 2018 IEEE 36th International Conference on Computer Design (ICCD), 83–91. https://doi.org/10.1109/iccd.2018.00022
- Ukiwe, E. K., Adeshina, S. A., Jacob, T., Adetokun, B. B. (2024). Deep learning model for detection of hotspots using infrared thermographic images of electrical installations. Journal of Electrical Systems and Information Technology, 11 (1). https://doi.org/10.1186/s43067-024-00148-y
- Zhao, X., Zhao, Y., Hu, S., Wang, H., Zhang, Y., Ming, W. (2023). Progress in Active Infrared Imaging for Defect Detection in the Renewable and Electronic Industries. Sensors, 23 (21), 8780. https://doi.org/10.3390/s23218780
- King, M., Woo, S. I., Yune, C.-Y. (2024). Utilizing a CNN-RNN machine learning approach for forecasting time-series outlet fluid temperature monitoring by long-term operation of BHEs system. Geothermics, 122, 103082. https://doi.org/10.1016/j.geothermics.2024.103082
- Deepak, G., Parthiban, M., Nath, Srigitha, S., Sulaiman Alfurhood, B., Mouleswararao, B., Ravi Kishore, V. (2024). Ai-enhanced thermal modeling for integrated process-product-system optimization in zero-defect manufacturing chains. Thermal Science and Engineering Progress, 55, 102945. https://doi.org/10.1016/j.tsep.2024.102945
- Chike, O. G., Ahmad, N., Faiz Wan Ali, W. F. (2024). Neural network prediction of thermal field spatiotemporal evolution during additive manufacturing: an overview. The International Journal of Advanced Manufacturing Technology, 134 (5-6), 2107–2128. https://doi.org/10.1007/s00170-024-14256-6
- Szymanik, B., Psuj, G., Hashemi, M., Lopato, P. (2021). Detection and Identification of Defects in 3D-Printed Dielectric Structures via Thermographic Inspection and Deep Neural Networks. Materials, 14 (15), 4168. https://doi.org/10.3390/ma14154168
- Wu, S.-H., Tariq, U., Joy, R., Mahmood, M. A., Malik, A. W., Liou, F. (2024). A Robust Recurrent Neural Networks-Based Surrogate Model for Thermal History and Melt Pool Characteristics in Directed Energy Deposition. Materials, 17 (17), 4363. https://doi.org/10.3390/ma17174363
- Eivazi, H., Guastoni, L., Schlatter, P., Azizpour, H., Vinuesa, R. (2021). Recurrent neural networks and Koopman-based frameworks for temporal predictions in a low-order model of turbulence. International Journal of Heat and Fluid Flow, 90, 108816. https://doi.org/10.1016/j.ijheatfluidflow.2021.108816
- Drgoňa, J., Tuor, A. R., Chandan, V., Vrabie, D. L. (2021). Physics-constrained deep learning of multi-zone building thermal dynamics. Energy and Buildings, 243, 110992. https://doi.org/10.1016/j.enbuild.2021.110992
- Cheng, Y., Huang, Y., Pang, B., Zhang, W. (2018). ThermalNet: A deep reinforcement learning-based combustion optimization system for coal-fired boiler. Engineering Applications of Artificial Intelligence, 74, 303–311. https://doi.org/10.1016/j.engappai.2018.07.003
- Akhsham, M., Dousti, M. J., Safari, S. (2025). Neural Network-Based Control of Forced-Convection and Thermoelectric Coolers. IEEE Transactions on Computer-Aided Design of Integrated Circuits and Systems, 44 (2), 582–591. https://doi.org/10.1109/tcad.2024.3438689
- Li, M., Dai, L., Hu, Y. (2022). Machine Learning for Harnessing Thermal Energy: From Materials Discovery to System Optimization. ACS Energy Letters, 7 (10), 3204–3226. https://doi.org/10.1021/acsenergylett.2c01836
- Zhang, B., Hu, W., Cao, D., Huang, Q., Chen, Z., Blaabjerg, F. (2019). Deep reinforcement learning–based approach for optimizing energy conversion in integrated electrical and heating system with renewable energy. Energy Conversion and Management, 202, 112199. https://doi.org/10.1016/j.enconman.2019.112199
- Ashayeri, M., Abbasabadi, N. (2024). A Hybrid Physics‐Based Machine Learning Approach for Integrated Energy and Exposure Modeling. Artificial Intelligence in Performance‐Driven Design, 57–79. https://doi.org/10.1002/9781394172092.ch3
- Anwar, A., Talha, M., Hameed, A. (2023). Application of Artificial Intelligence in Thermal Management and Wavelet Analysis: A Comprehensive Review. https://doi.org/10.31219/osf.io/d2vn3
- Ali, S. A., Habib, K., Younas, M., Rahman, S., Das, L., Rubbi, F. et al. (2024). Advancements in Thermal Energy Storage: A Review of Material Innovations and Strategic Approaches for Phase Change Materials. Energy & Fuels, 38 (20), 19336–19392. https://doi.org/10.1021/acs.energyfuels.4c03634
- Sadeghi, G. (2022). Energy storage on demand: Thermal energy storage development, materials, design, and integration challenges. Energy Storage Materials, 46, 192–222. https://doi.org/10.1016/j.ensm.2022.01.017
- Chekifi, T., Boukraa, M., Benmoussa, A. (2024). Artificial Intelligence for Thermal Energy Storage Enhancement: A Comprehensive Review. Journal of Energy Resources Technology, 146 (6). https://doi.org/10.1115/1.4065197
- Bandhu, D., Khadir, M. D., Kaushik, A., Sharma, S., Ali, H. A., Jain, A. (2023). Innovative Approaches to Thermal Management in Next-Generation Electronics. E3S Web of Conferences, 430, 01139. https://doi.org/10.1051/e3sconf/202343001139
- Lodhi, S. K., Hussain, H. K., Hussain, I. (2024). Using AI to Increase Heat Exchanger Efficiency: An Extensive Analysis of Innovations and Uses. International Journal of Multidisciplinary Sciences and Arts, 3 (4), 1–14. https://doi.org/10.47709/ijmdsa.v3i4.4617
- Sabegh, M. (2022). Deep Reinforcement Learning and Model Predictive Control Approaches for the Scheduled Operation of Domestic Refrigerators. [PhD thesis; University of Lincoln].
- Ghalkhani, M., Habibi, S. (2022). Review of the Li-Ion Battery, Thermal Management, and AI-Based Battery Management System for EV Application. Energies, 16 (1), 185. https://doi.org/10.3390/en16010185
- Nasiri, M., Hadim, H. (2024). Advances in battery thermal management: Current landscape and future directions. Renewable and Sustainable Energy Reviews, 200, 114611. https://doi.org/10.1016/j.rser.2024.114611
- Cao, K., Li, Z., Luo, H., Jiang, Y., Liu, H., Xu, L. et al. (2024). Comprehensive review and future prospects of multi-level fan control strategies in data centers for joint optimization of thermal management systems. Journal of Building Engineering, 94, 110021. https://doi.org/10.1016/j.jobe.2024.110021
- Nadjahi, C., Louahlia, H., Lemasson, S. (2018). A review of thermal management and innovative cooling strategies for data center. Sustainable Computing: Informatics and Systems, 19, 14–28. https://doi.org/10.1016/j.suscom.2018.05.002
- Weinstein, J. (2023). Semiconductors and the calculation of the balance of power. Available at: https://knowledge.uchicago.edu/nanna/record/6118/files/Semiconductors%20and%20the%20Calculation%20of%20the%20Balance%20of%20Power.pdf?withWatermark=0&withMetadata=0®isterDownload=1&version=1
- Hosseinimotlagh, S., Enright, D., Shelton, C. R., Kim, H. (2021). Data-Driven Structured Thermal Modeling for COTS Multi-core Processors. 2021 IEEE Real-Time Systems Symposium (RTSS), 201–213. https://doi.org/10.1109/rtss52674.2021.00028
- Hirtz, T., Tian, H., Shahzad, S., Wu, F., Yang, Y., Ren, T.-L. (2024). Deep reinforcement learning framework for end-to-end semiconductor process control. Neural Computing and Applications, 36 (20), 12443–12460. https://doi.org/10.1007/s00521-024-09710-1
- Radamson, H. H., Zhu, H., Wu, Z., He, X., Lin, H., Liu, J. et al. (2020). State of the Art and Future Perspectives in Advanced CMOS Technology. Nanomaterials, 10 (8), 1555. https://doi.org/10.3390/nano10081555
- Fassi, Y., Heiries, V., Boutet, J., Boisseau, S. (2024). Toward Physics-Informed Machine-Learning-Based Predictive Maintenance for Power Converters – A Review. IEEE Transactions on Power Electronics, 39 (2), 2692–2720. https://doi.org/10.1109/tpel.2023.3328438
- Buffa, S., Fouladfar, M. H., Franchini, G., Lozano Gabarre, I., Andrés Chicote, M. (2021). Advanced Control and Fault Detection Strategies for District Heating and Cooling Systems – A Review. Applied Sciences, 11 (1), 455. https://doi.org/10.3390/app11010455
- Zhang, Y., Zhao, Y., Dai, S., Nie, B., Ma, H., Li, J. et al. (2022). Cooling technologies for data centres and telecommunication base stations – A comprehensive review. Journal of Cleaner Production, 334, 130280. https://doi.org/10.1016/j.jclepro.2021.130280
- Suryadevara, S. (2021). Energy-proportional computing: Innovations in data center efficiency and performance optimization. International Journal, 44–64.
- Li, Y., Wen, Y., Tao, D., Guan, K. (2020). Transforming Cooling Optimization for Green Data Center via Deep Reinforcement Learning. IEEE Transactions on Cybernetics, 50 (5), 2002–2013. https://doi.org/10.1109/tcyb.2019.2927410
- Biswas, P., Rashid, A., Biswas, A., Nasim, M. A. A., Chakraborty, S., Gupta, K. D., George, R. (2024). AI-driven approaches for optimizing power consumption: a comprehensive survey. Discover Artificial Intelligence, 4 (1). https://doi.org/10.1007/s44163-024-00211-7
- Velayutham, A. (2019). Ai-driven storage optimization for sustainable cloud data centers: Reducing energy consumption through predictive analytics, dynamic storage scaling, and proactive resource allocation. Sage Science Review of Applied Machine Learning, 2 (2), 57–71.
- Dadashi, S., Aghasi, A. (2024). Thermal-aware virtual machine placement approaches: A survey. Journal of Mahani Mathematical Research Center, 13 (2).
- Gu, J. (2023). Characterization and modelling of resource usage and energy consumption in hpc datacenters by machine learnin. [Master Thesis; University of Amsterdam].
- Boppana, V. R. (2023). Data Analytics for Predictive Maintenance in Healthcare Equipment. EPH-International Journal of Business & Management Science, 9 (2), 26–36. https://doi.org/10.53555/eijbms.v10i1.176
- Casado-Vara, R., Vale, Z., Prieto, J., Corchado, J. M. (2018). Fault-Tolerant Temperature Control Algorithm for IoT Networks in Smart Buildings. Energies, 11 (12), 3430. https://doi.org/10.3390/en11123430
- Balali, Y., Chong, A., Busch, A., O’Keefe, S. (2023). Energy modelling and control of building heating and cooling systems with data-driven and hybrid models – A review. Renewable and Sustainable Energy Reviews, 183, 113496. https://doi.org/10.1016/j.rser.2023.113496
- Pattyam, S. P. (2020). Ai in data science for predictive analytics: Techniques for model development, validation, and deployment. Journal of Science & Technology, 1 (1), 511–552.
- Latapy, A., Ferret, Y., Testut, L., Talke, S., Aarup, T., Pons, F., Jan, G., Bradshaw, E., Pouvreau, N. (2022). Data rescue process in the context of sea level reconstructions: An overview of the methodology, lessons learned, up‐to‐date best practices and recommendations. Geoscience Data Journal, 10 (3), 396–425. https://doi.org/10.1002/gdj3.179
- Liang, W., Tadesse, G. A., Ho, D., Fei-Fei, L., Zaharia, M., Zhang, C., Zou, J. (2022). Advances, challenges and opportunities in creating data for trustworthy AI. Nature Machine Intelligence, 4 (8), 669–677. https://doi.org/10.1038/s42256-022-00516-1
- Shah, V., Konda, S. R. (2021). Neural networks and explainable ai: Bridging the gap between models and interpretability. International Journal of Computer Science and Technology, 5 (2), 163–176.
- Chen, Z., Xiao, F., Guo, F., Yan, J. (2023). Interpretable machine learning for building energy management: A state-of-the-art review. Advances in Applied Energy, 9, 100123. https://doi.org/10.1016/j.adapen.2023.100123
- Buah, E. (2022). Artificial intelligence technology acceptance framework for energy systems analysis. Available at: https://lutpub.lut.fi/handle/10024/163686
- Sheriffdeen, K. (2024). Physics-based simulation-assisted machine learning for estimating engineering system failure durations. Available at: https://easychair.org/publications/preprint/nHDK
- Kasaraneni, R. K. (2020). AI-enhanced energy management systems for electric vehicles: Optimizing battery performance and longevity. Journal of Science & Technology, 1 (1), 670–708.
- Modupe, O. T., Otitoola, A. A., Oladapo, O. J., Abiona, O. O., Oyeniran, O. C., Adewusi, A. O., et al. (2024). Reviewing the transformational impact of edge computing on real-time data processing and analytics. Computer Science & IT Research Journal, 5 (3), 693–702. https://doi.org/10.51594/csitrj.v5i3.929
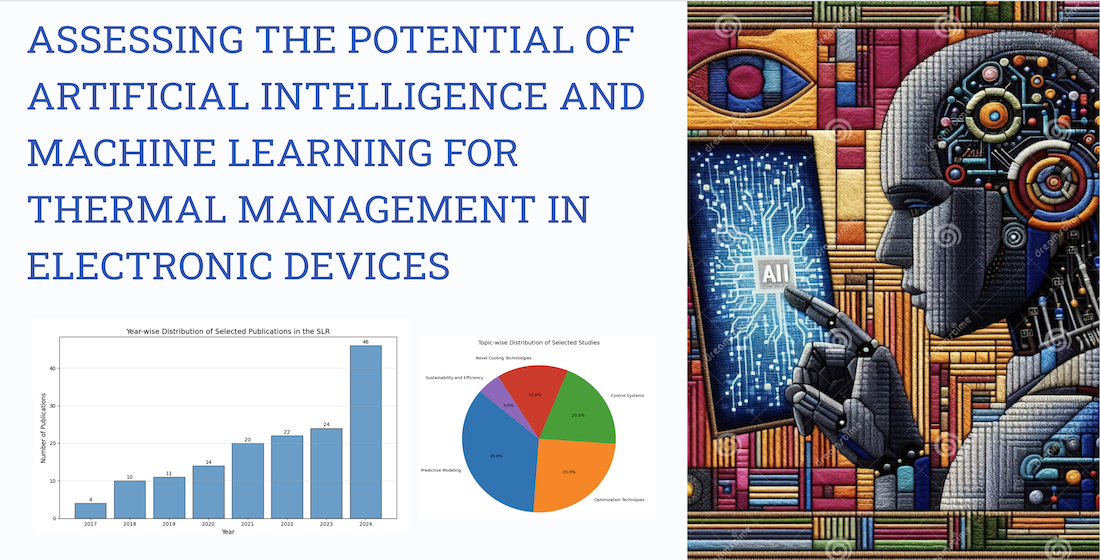
Downloads
Published
How to Cite
Issue
Section
License
Copyright (c) 2025 Oleh Yatskiv, Bohdan Koman

This work is licensed under a Creative Commons Attribution 4.0 International License.
The consolidation and conditions for the transfer of copyright (identification of authorship) is carried out in the License Agreement. In particular, the authors reserve the right to the authorship of their manuscript and transfer the first publication of this work to the journal under the terms of the Creative Commons CC BY license. At the same time, they have the right to conclude on their own additional agreements concerning the non-exclusive distribution of the work in the form in which it was published by this journal, but provided that the link to the first publication of the article in this journal is preserved.