Correlation and regression analysis in assessing the relationship between water indicators: a brief description of long-term measurement data from biosensors
DOI:
https://doi.org/10.15587/2706-5448.2025.323730Keywords:
water indicator, measurement system, multi-sensors system, correlation analysis, testing, internet of things, biosensorsAbstract
The object of the study is the method for assessing the relationship between the results of long-term observations of water parameters obtained by the method of combined measurements by a biosensor system. The biosensor system is designed for the combined measurement of five water parameters based on physical value sensors. In the paper, the problem under consideration quite fully levels out a significant limitation of the known solutions designed for the simultaneous measurement of three or four water parameters. Existing approaches in their structure combine less than five biosensors-sensors, which significantly limits the simultaneous measurement of five water parameters.
One of the main and principal results of the paper is the development of a statistical model for assessing the relationship between the combined measurements of five water parameters. It was revealed that among the five measured parameters, the most influential predictor for acidity, conductivity, turbidity and oxidation-reduction potential is water temperature. The established significant and non-random relationship between the parameters is mainly associated with the effect of temperature on the physical processes occurring with an increase and decrease in water temperature depending on the observation time. These estimates demonstrate a higher, statistically significant relationship between the measurement information data. This is achieved by implementing the method of aggregate measurement of water parameters: temperature, acidity, turbidity, conductivity, oxidation-reduction potential.
The efficiency of the statistical model is confirmed by calculating the correlation coefficient based on the Pearson method and the coefficients of determination and reliability of the model. The regression model can be used in practice in developing new or improving known measuring systems and control devices to increase the reliability and effectiveness of water quality control.
Supporting Agency
- This research has been/was/is funded by the Committee of Science of the Ministry of Science and Higher Education of the Republic of Kazakhstan (BR24993051 Development of an intelligent city system based on IoT and data analysis).
References
- Tariq, M. O., Siddiq, A., Irshad, H., Aman, M., Khan, M. S. (2021). An Open Source Water Quality Measurement System for Remote Areas. The 1st International Conference on Energy, Power and Environment, 50. https://doi.org/10.3390/engproc2021012050
- Zhao, Y., Yu, T., Hu, B., Zhang, Z., Liu, Y., Liu, X. et al. (2022). Retrieval of Water Quality Parameters Based on Near-Surface Remote Sensing and Machine Learning Algorithm. Remote Sensing, 14 (21), 5305. https://doi.org/10.3390/rs14215305
- Wood, R., Tembele, W., Hema, A., Somé, A., Kinganda-Lusamaki, E., Basilubo, C. et al. (2024). Implementation of the WHO core components of an infection prevention and control programme in two sub-saharan African acute health-care facilities: a mixed methods study. Antimicrobial Resistance & Infection Control, 13 (1). https://doi.org/10.1186/s13756-023-01358-1
- Mathur, R., Sharma, M. K., Loganathan, K., Abbas, M., Hussain, S., Kataria, G. et al. (2024). Modeling of two-stage anaerobic onsite wastewater sanitation system to predict effluent soluble chemical oxygen demand through machine learning. Scientific Reports, 14 (1). https://doi.org/10.1038/s41598-023-50805-x
- Wang, Z., Duan, L., Shuai, D., Qiu, T. (2024). Research on water environmental indicators prediction method based on EEMD decomposition with CNN-BiLSTM. Scientific Reports, 14 (1). https://doi.org/10.1038/s41598-024-51936-5
- Shah, M. I., Javed, M. F., Abunama, T. (2020). Proposed formulation of surface water quality and modelling using gene expression, machine learning, and regression techniques. Environmental Science and Pollution Research, 28 (11), 13202–13220. https://doi.org/10.1007/s11356-020-11490-9
- de Camargo, E. T., Spanhol, F. A., Slongo, J. S., da Silva, M. V. R., Pazinato, J., de Lima Lobo, A. V. et al. (2023). Low-Cost Water Quality Sensors for IoT: A Systematic Review. Sensors, 23 (9), 4424. https://doi.org/10.3390/s23094424
- Essamlali, I., Nhaila, H., El Khaili, M. (2024). Advances in machine learning and IoT for water quality monitoring: A comprehensive review. Heliyon, 10 (6), e27920. https://doi.org/10.1016/j.heliyon.2024.e27920
- Abbas Fadel, A., Ibrahim Shujaa, M. (2020). Water Quality Monitoring System Based on IOT Platform. IOP Conference Series: Materials Science and Engineering, 928 (3), 032054. https://doi.org/10.1088/1757-899x/928/3/032054
- Bogdan, R., Paliuc, C., Crisan-Vida, M., Nimara, S., Barmayoun, D. (2023). Low-Cost Internet-of-Things Water-Quality Monitoring System for Rural Areas. Sensors, 23 (8), 3919. https://doi.org/10.3390/s23083919
- Lakshmikantha, V., Hiriyannagowda, A., Manjunath, A., Patted, A., Basavaiah, J., Anthony, A. A. (2021). IoT based smart water quality monitoring system. Global Transitions Proceedings, 2 (2), 181–186. https://doi.org/10.1016/j.gltp.2021.08.062
- Geetha, S., Gouthami, S. (2016). Internet of things enabled real time water quality monitoring system. Smart Water, 2 (1). https://doi.org/10.1186/s40713-017-0005-y
- Chowdury, M. S. U., Emran, T. B., Ghosh, S., Pathak, A., Alam, Mohd, M., Absar, N. et al. (2019). IoT Based Real-time River Water Quality Monitoring System. Procedia Computer Science, 155, 161–168. https://doi.org/10.1016/j.procs.2019.08.025
- Sabari, M., Aswinth, P., Karthik, T., Bharath Kumar, C. (2020). Water Quality Monitoring System Based on IoT. 2020 5th International Conference on Devices, Circuits and Systems (ICDCS), 279–282. https://doi.org/10.1109/icdcs48716.2020.243598
- Altay, Y. A., Lyamin, A. V., Kelemseiit, N. E., Skakov, D. M. (2023). Cascade Notch Filter with a Unity Feedback and Improved Transient Response. 2023 V International Conference on Control in Technical Systems (CTS), 217–220. https://doi.org/10.1109/cts59431.2023.10288775
- Altay, Y. A., Bazarbay, L., Uskenbayeva, R. K., Fedorov, A. V., Kalpeyeva, Z. B. (2024). Water Indicator Measurement and Data Processing From Internet of Things Modules. 2024 International Conference on Electrical Engineering and Photonics (EExPolytech), 253–256. https://doi.org/10.1109/eexpolytech62224.2024.10755886
- Altay, Y. A., Bazarbay, L. (2024). Artificial Intelligence-Assisted Water Quality Model: Long-Term Follow-Up Data. 2024 V International Conference on Neural Networks and Neurotechnologies (NeuroNT), 1–3. https://doi.org/10.1109/neuront62606.2024.10585363
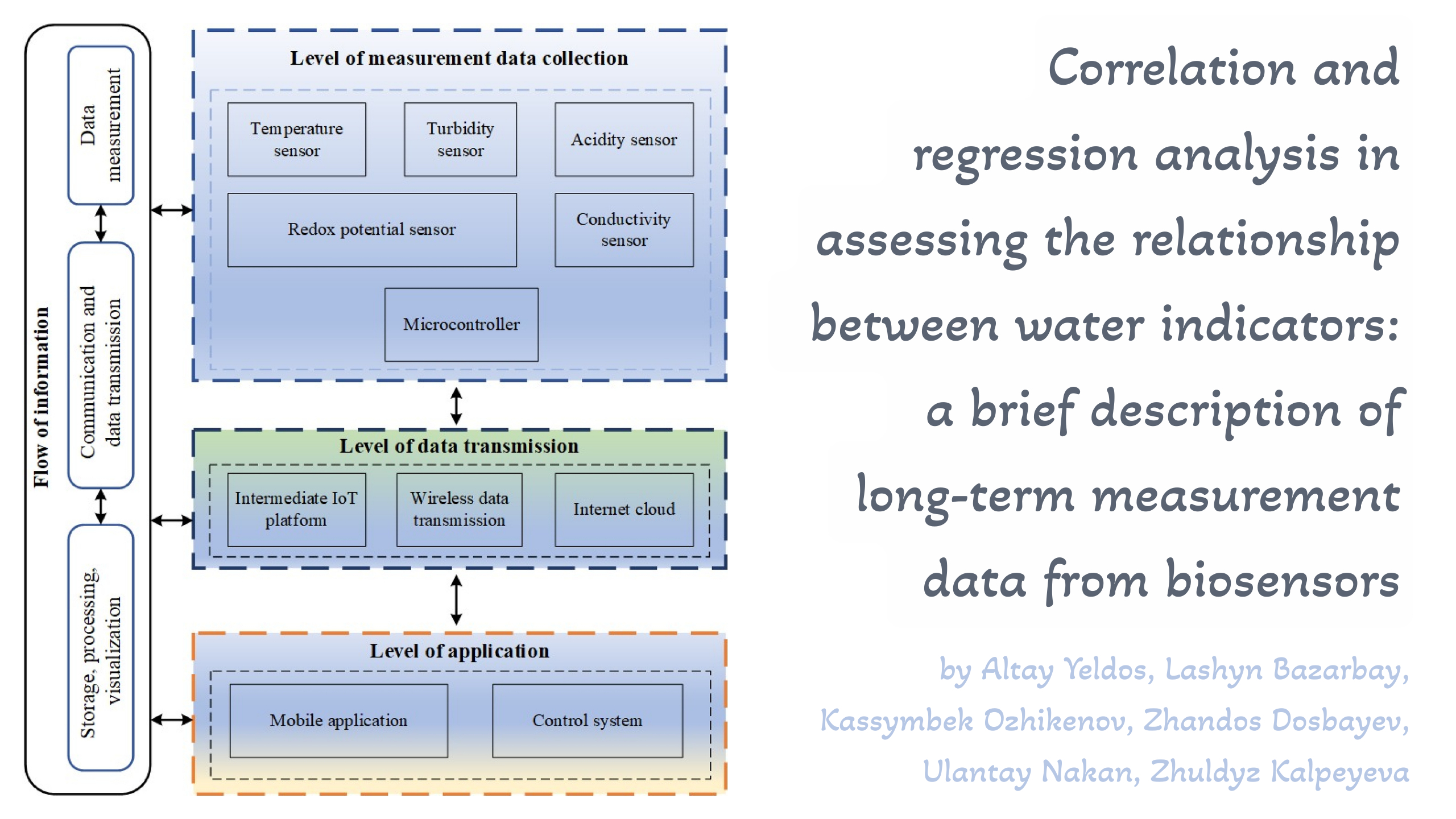
Downloads
Published
How to Cite
Issue
Section
License
Copyright (c) 2025 Altay Yeldos, Lashin Bazarbay, Kassymbek Ozhikenov, Zhandos Dosbayev, Ulantay Nakan, Zhuldyz Kalpeyeva

This work is licensed under a Creative Commons Attribution 4.0 International License.
The consolidation and conditions for the transfer of copyright (identification of authorship) is carried out in the License Agreement. In particular, the authors reserve the right to the authorship of their manuscript and transfer the first publication of this work to the journal under the terms of the Creative Commons CC BY license. At the same time, they have the right to conclude on their own additional agreements concerning the non-exclusive distribution of the work in the form in which it was published by this journal, but provided that the link to the first publication of the article in this journal is preserved.