Developing a prototype of fire detection and automatic extinguisher mobile robot based on convolutional neural network
DOI:
https://doi.org/10.15587/2706-5448.2022.269861Keywords:
mobile robot, supervised deep learning, convolutional neural network (CNN), image processing, transfer learningAbstract
The object of research is a prototype of fire detection and automatic extinguisher mobile robot based on convolutional neural network. Within the recent few decades, fires are considered as one of the most serious disaster that occurs in many places around the world. The severity of fire incidents causes damages to buildings, infrastructures and properties. Resulting losses of human’s life and costs them a lot of losses. Thus, fire poses a great threat to us significantly; it is extremely dangerous for fire fighters. Fires can be resulted by materials such as rubber and chemical products. Other sources of fire are the short circuits on electrical devices and faults in power circuits. Additionally, overheating and overloading problems can be the cause of fire incidents. All these reasons lead to bad consequences when there is no immediate response to such problems. The advent of computer vision technology has played such a significant role for human life. Artificial intelligence field has improved the efficiency and behaviors of robotics beyond expectations. The interference of artificial intelligence made robotics act intelligently. For this reason, in this paper we presented a mobile robot based on deep learning to detect the fire source and determines its coordinate position then automatically moves toward the target and extinguish fire. Deep learning algorithms are the efficient ones for object detection applications. CNN model is one of the most common deep learning algorithms which have been used in the study for the fire detection. Due to the insufficient amount of datasets and large efforts required to build model from scratch. MobileNet V2 is one of the CNN models that support transfer learning technique. After training the model and testing it on 20 % of the used datasets the classification accuracy achieved up to 98.01 %. The motion repeatability of the robot has been implemented and tested resulting mean error 0.648 cm.
Supporting Agency
- Presentation of research in the form of publication through financial support in the form of a grant from SUES (Support to Ukrainian Editorial Staff).
References
- Brushlinsky, N. N., Ahrens, M., Sokolov, S. V., Wagner, P. (2016). World fire statistics. Cent. fire statistics., 10.
- Woodrow, B. (2012). Fire as Vulnerability: The Value Added from Adopting a Vulnerability Approach. World Fire Stat. Bull. Valéria Pacella.
- Pastor, E., Zárate, L., Planas, E., Arnaldos, J. (2003). Mathematical models and calculation systems for the study of wildland fire behaviour. Progress in Energy and Combustion Science, 29 (2), 139–153. doi: https://doi.org/10.1016/s0360-1285(03)00017-0
- Masellis, M., Ferrara, M. M., Gunn, S. W. A. (1999). Fire disaster and burn disaster: Planning and management. Annals of Burns and Fire Disasters, 12, 67–76.
- Jamesdaniel, S., Elhage, K. G., Rosati, R., Ghosh, S., Arnetz, B., Blessman, J. (2019). Tinnitus and Self-Perceived Hearing Handicap in Firefighters: A Cross-Sectional Study. International Journal of Environmental Research and Public Health, 16 (20), 3958. doi: https://doi.org/10.3390/ijerph16203958
- Tedim, F., Leone, V., McCaffrey, S., McGee, T. K., Coughlan, M., Correia, F. J. M., Magalhães, C. G. (2020). Safety enhancement in extreme wildfire events. Extreme Wildfire Events and Disasters. Elsevier, 91–115. doi: https://doi.org/10.1016/b978-0-12-815721-3.00005-9
- Heydari, A., Ostadtaghizadeh, A., Ardalan, A., Ebadi, A., Mohammadfam, I., Khorasani-Zavareh, D. (2022). Exploring the criteria and factors affecting firefighters’ resilience: A qualitative study. Chinese Journal of Traumatology, 25 (2), 107–114. doi: https://doi.org/10.1016/j.cjtee.2021.06.001
- Yoon, J.-H., Kim, Y.-K., Kim, K. S., Ahn, Y.-S. (2016). Characteristics of Workplace Injuries among Nineteen Thousand Korean Firefighters. Journal of Korean Medical Science, 31 (10), 1546. doi: https://doi.org/10.3346/jkms.2016.31.10.1546
- Khoon, T. N., Sebastian, P., Saman, A. B. S. (2012). Autonomous Fire Fighting Mobile Platform. Procedia Engineering, 41, 1145–1153. doi: https://doi.org/10.1016/j.proeng.2012.07.294
- Kim, J.-H., Starr, J. W., Lattimer, B. Y. (2014). Firefighting Robot Stereo Infrared Vision and Radar Sensor Fusion for Imaging through Smoke. Fire Technology, 51 (4), 823–845. doi: https://doi.org/10.1007/s10694-014-0413-6
- Sivakumar, E. V., Manoj, P., Pumithadevi, S., Sylvia, S. S., Thangaraj, M. (2016). Voice Controlled Intellegent Fire Extinguisher Robot. International Journal for Science and Advance Research in Technology, 2, 65–67.
- Sonal, M., Bharat, M., Saraswati, S., Bansude, V. U. (2017). Fire Fighting Robot. International Research Journal of Engineering and Technology, 4, 136–138.
- Vijayalakshmi, B., Vasanth, A., Kumar, S. V., Raj, S. N. (2017). Autonomous Fire Fighting Robot With Self Power Management. International Journal of Scientific Engineering and Research, 8, 234–237.
- Sucuoglu, H. S., Bogrekci, I., Demircioglu, P. (2018). Development of Mobile Robot with Sensor Fusion Fire Detection Unit. IFAC-PapersOnLine, 51 (30), 430–435. doi: https://doi.org/10.1016/j.ifacol.2018.11.324
- Aliff, M., Samsiah, N., Yusof, M., Zainal, A. (2019). Development of Fire Fighting Robot (QRob). International Journal of Advanced Computer Science and Applications, 10 (1). doi: https://doi.org/10.14569/ijacsa.2019.0100118
- Chandra, P. S., Revathi, V., Sireesha, A., Kumar, N. S. (2019). Development of DTMF Centred Remotely Located Fire Extinguishing Robot. International Journal of Innovative Technology and Exploring Engineering, 9 (1), 39–43. doi: https://doi.org/10.35940/ijitee.a3902.119119
- Dhiman, A., Shah, N., Adhikari, P., Kumbhar, S., Dhanjal, I., Mehendale, N. (2020). Fire Fighter Robot with Deep Learning and Machine Vision. SSRN Electronic Journal. doi: https://doi.org/10.2139/ssrn.3633609
- Alzubaidi, L., Zhang, J., Humaidi, A. J., Al-Dujaili, A., Duan, Y., Al-Shamma, O. et al. (2021). Review of deep learning: concepts, CNN architectures, challenges, applications, future directions. Journal of Big Data, 8 (1). doi: https://doi.org/10.1186/s40537-021-00444-8
- Liu, H., Lang, B. (2019). Machine Learning and Deep Learning Methods for Intrusion Detection Systems: A Survey. Applied Sciences, 9 (20), 4396. doi: https://doi.org/10.3390/app9204396
- Najafabadi, M. M., Villanustre, F., Khoshgoftaar, T. M., Seliya, N., Wald, R., Muharemagic, E. (2015). Deep learning applications and challenges in big data analytics. Journal of Big Data, 2 (1). doi: https://doi.org/10.1186/s40537-014-0007-7
- Shen, C. (2018). A Transdisciplinary Review of Deep Learning Research and Its Relevance for Water Resources Scientists. Water Resources Research, 54 (11), 8558–8593. doi: https://doi.org/10.1029/2018wr022643
- Atitallah, S. B., Driss, M., Boulila, W., Ghézala, H. B. (2020). Leveraging Deep Learning and IoT big data analytics to support the smart cities development: Review and future directions. Computer Science Review, 38, 100303. doi: https://doi.org/10.1016/j.cosrev.2020.100303
- Wang, W., Liang, D., Chen, Q., Iwamoto, Y., Han, X.-H., Zhang, Q. et al. (2019). Medical Image Classification Using Deep Learning. Deep Learning in Healthcare. Springer, 33–51. doi: https://doi.org/10.1007/978-3-030-32606-7_3
- Papernot, N., McDaniel, P., Jha, S., Fredrikson, M., Celik, Z. B., Swami, A. (2016). The Limitations of Deep Learning in Adversarial Settings. 2016 IEEE European Symposium on Security and Privacy (EuroS&P), 372–387. doi: https://doi.org/10.1109/eurosp.2016.36
- Shorten, C., Khoshgoftaar, T. M., Furht, B. (2021). Deep Learning applications for COVID-19. Journal of Big Data, 8 (1). doi: https://doi.org/10.1186/s40537-020-00392-9
- Udendhran, R., Balamurugan, M., Suresh, A., Varatharajan, R. (2020). Enhancing image processing architecture using deep learning for embedded vision systems. Microprocessors and Microsystems, 76, 103094. doi: https://doi.org/10.1016/j.micpro.2020.103094
- Peng, X., Zhang, X., Li, Y., Liu, B. (2020). Research on image feature extraction and retrieval algorithms based on convolutional neural network. Journal of Visual Communication and Image Representation, 69, 102705. doi: https://doi.org/10.1016/j.jvcir.2019.102705
- Ramprasath, M., Anand, M. V., Hariharan, S. (2018). Image classification using convolutional neural networks. International Journal of Pure and Applied Mathematics, 119 (17), 1307–1319.
- Liang, G., Hong, H., Xie, W., Zheng, L. (2018). Combining Convolutional Neural Network With Recursive Neural Network for Blood Cell Image Classification. IEEE Access, 6, 36188–36197. doi: https://doi.org/10.1109/access.2018.2846685
- Albawi, S., Mohammed, T. A., Al-Zawi, S. (2017). Understanding of a convolutional neural network. 2017 International Conference on Engineering and Technology (ICET). doi: https://doi.org/10.1109/icengtechnol.2017.8308186
- Ajit, A., Acharya, K., Samanta, A. (2020). A Review of Convolutional Neural Networks. 2020 International Conference on Emerging Trends in Information Technology and Engineering (Ic-ETITE). doi: https://doi.org/10.1109/ic-etite47903.2020.049
- Shaheen, F., Verma, B., Asafuddoula, Md. (2016). Impact of Automatic Feature Extraction in Deep Learning Architecture. 2016 International Conference on Digital Image Computing: Techniques and Applications (DICTA). doi: https://doi.org/10.1109/dicta.2016.7797053
- Elangovan, P., Nath, M. K. (2020). Glaucoma assessment from color fundus images using convolutional neural network. International Journal of Imaging Systems and Technology, 31 (2), 955–971. doi: https://doi.org/10.1002/ima.22494
- Elkhayati, M., Elkettani, Y. (2022). UnCNN: A New Directed CNN Model for Isolated Arabic Handwritten Characters Recognition. Arabian Journal for Science and Engineering, 47 (8), 10667–10688. doi: https://doi.org/10.1007/s13369-022-06652-5
- Li, Z., Ren, K., Jiang, X., Li, B., Zhang, H., Li, D. (2022). Domain generalization using pretrained models without fine-tuning. doi: https://doi.org/10.48550/arXiv.2203.04600
- Mehrotra, R., Ansari, M. A., Agrawal, R., Anand, R. S. (2020). A Transfer Learning approach for AI-based classification of brain tumors. Machine Learning with Applications, 2, 100003. doi: https://doi.org/10.1016/j.mlwa.2020.100003
- Yao, Y., Doretto, G. (2010). Boosting for transfer learning with multiple sources. 2010 IEEE Computer Society Conference on Computer Vision and Pattern Recognition, 1855–1862. doi: https://doi.org/10.1109/cvpr.2010.5539857
- Bouhamed, O., Ghazzai, H., Besbes, H., Massoud, Y. (2020). Autonomous UAV Navigation: A DDPG-Based Deep Reinforcement Learning Approach. 2020 IEEE International Symposium on Circuits and Systems (ISCAS). doi: https://doi.org/10.1109/iscas45731.2020.9181245
- Howard, A. G., Zhu, M., Chen, B., Kalenichenko, D., Wang, W., Weyand, T. et al. (2017). Mobilenets: Efficient convolutional neural networks for mobile vision applications. doi: https://doi.org/10.48550/arXiv.1704.04861
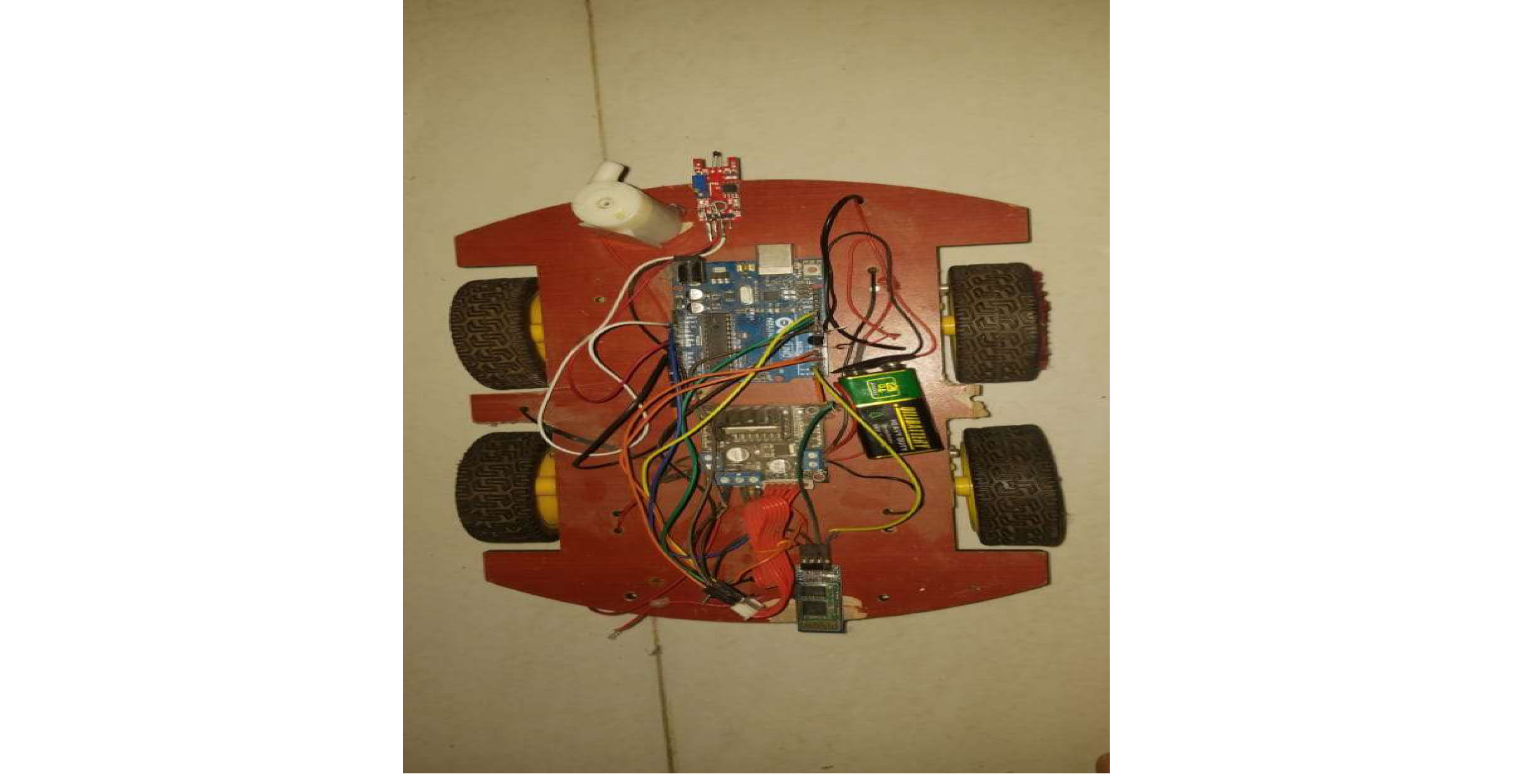
Downloads
Published
How to Cite
Issue
Section
License
Copyright (c) 2022 Amin Saif, Gamal Muneer, Yusuf Abdulrahman, Hareth Abdulbaqi, Aiman Abdullah, Abdullah Ali, Abduljalil Derhim

This work is licensed under a Creative Commons Attribution 4.0 International License.
The consolidation and conditions for the transfer of copyright (identification of authorship) is carried out in the License Agreement. In particular, the authors reserve the right to the authorship of their manuscript and transfer the first publication of this work to the journal under the terms of the Creative Commons CC BY license. At the same time, they have the right to conclude on their own additional agreements concerning the non-exclusive distribution of the work in the form in which it was published by this journal, but provided that the link to the first publication of the article in this journal is preserved.