Розробка прототипу мобільного робота з виявлення пожежі та автоматичного пожежогасіння на основі згорткової нейронної мережі
DOI:
https://doi.org/10.15587/2706-5448.2022.269861Ключові слова:
мобільний робот, кероване глибинне навчання, згорточна нейронна мережа (CNN), обробка зображень, трансферне навчанняАнотація
Об’єктом дослідження є прототип мобільного робота для виявлення пожежі та автоматичного пожежогасіння на основі згорткової нейронної мережі. За останні кілька десятиліть пожежі вважаються однією з найсерйозніших катастроф, які трапляються в багатьох місцях по всьому світу. Великі пожежі завдають шкоди будівлям, інфраструктурі та майну. Вони призводить до людських втрат і приносять людям великих збитків. Таким чином, вогонь становить для нас велику загрозу, він також надзвичайно небезпечний для пожежників. Пожежу можуть спричинити такі матеріали, як гума та хімічні продукти. Іншими джерелами пожежі є короткі замикання електрообладнання та несправності в електромережах. Крім того, причиною пожежі можуть бути проблеми з перегріванням і перевантаженням. Всі ці причини призводять до поганих наслідків, коли на такі проблеми не реагують негайно. Поява технології комп’ютерного зору відіграла таку значну роль у житті людини. Сфера штучного інтелекту покращила ефективність і поведінку робототехніки понад очікування. Втручання штучного інтелекту змусило робототехніку діяти розумно. З цієї причини в цій роботі представлено мобільного робота, заснованого на глибокому навчанні, для виявлення джерела пожежі та визначення його координатної позиції, а потім автоматичного руху до цілі та гасіння пожежі. Алгоритми глибокого навчання є ефективними для програм виявлення об’єктів. Модель CNN є одним із найпоширеніших алгоритмів глибокого навчання, який використовувався в дослідженні для виявлення пожежі. Через недостатню кількість наборів даних і великі зусилля, необхідні для створення моделі з нуля. MobileNet V2 – це одна з моделей CNN, що підтримує метод трансферного навчання. Після навчання моделі та її тестування на 20 % використаних наборів даних точність класифікації досягла 98,01 %. Повторюваність руху робота була реалізована та протестована, що дало середню похибку 0,648 см.
Спонсор дослідження
- Presentation of research in the form of publication through financial support in the form of a grant from SUES (Support to Ukrainian Editorial Staff).
Посилання
- Brushlinsky, N. N., Ahrens, M., Sokolov, S. V., Wagner, P. (2016). World fire statistics. Cent. fire statistics., 10.
- Woodrow, B. (2012). Fire as Vulnerability: The Value Added from Adopting a Vulnerability Approach. World Fire Stat. Bull. Valéria Pacella.
- Pastor, E., Zárate, L., Planas, E., Arnaldos, J. (2003). Mathematical models and calculation systems for the study of wildland fire behaviour. Progress in Energy and Combustion Science, 29 (2), 139–153. doi: https://doi.org/10.1016/s0360-1285(03)00017-0
- Masellis, M., Ferrara, M. M., Gunn, S. W. A. (1999). Fire disaster and burn disaster: Planning and management. Annals of Burns and Fire Disasters, 12, 67–76.
- Jamesdaniel, S., Elhage, K. G., Rosati, R., Ghosh, S., Arnetz, B., Blessman, J. (2019). Tinnitus and Self-Perceived Hearing Handicap in Firefighters: A Cross-Sectional Study. International Journal of Environmental Research and Public Health, 16 (20), 3958. doi: https://doi.org/10.3390/ijerph16203958
- Tedim, F., Leone, V., McCaffrey, S., McGee, T. K., Coughlan, M., Correia, F. J. M., Magalhães, C. G. (2020). Safety enhancement in extreme wildfire events. Extreme Wildfire Events and Disasters. Elsevier, 91–115. doi: https://doi.org/10.1016/b978-0-12-815721-3.00005-9
- Heydari, A., Ostadtaghizadeh, A., Ardalan, A., Ebadi, A., Mohammadfam, I., Khorasani-Zavareh, D. (2022). Exploring the criteria and factors affecting firefighters’ resilience: A qualitative study. Chinese Journal of Traumatology, 25 (2), 107–114. doi: https://doi.org/10.1016/j.cjtee.2021.06.001
- Yoon, J.-H., Kim, Y.-K., Kim, K. S., Ahn, Y.-S. (2016). Characteristics of Workplace Injuries among Nineteen Thousand Korean Firefighters. Journal of Korean Medical Science, 31 (10), 1546. doi: https://doi.org/10.3346/jkms.2016.31.10.1546
- Khoon, T. N., Sebastian, P., Saman, A. B. S. (2012). Autonomous Fire Fighting Mobile Platform. Procedia Engineering, 41, 1145–1153. doi: https://doi.org/10.1016/j.proeng.2012.07.294
- Kim, J.-H., Starr, J. W., Lattimer, B. Y. (2014). Firefighting Robot Stereo Infrared Vision and Radar Sensor Fusion for Imaging through Smoke. Fire Technology, 51 (4), 823–845. doi: https://doi.org/10.1007/s10694-014-0413-6
- Sivakumar, E. V., Manoj, P., Pumithadevi, S., Sylvia, S. S., Thangaraj, M. (2016). Voice Controlled Intellegent Fire Extinguisher Robot. International Journal for Science and Advance Research in Technology, 2, 65–67.
- Sonal, M., Bharat, M., Saraswati, S., Bansude, V. U. (2017). Fire Fighting Robot. International Research Journal of Engineering and Technology, 4, 136–138.
- Vijayalakshmi, B., Vasanth, A., Kumar, S. V., Raj, S. N. (2017). Autonomous Fire Fighting Robot With Self Power Management. International Journal of Scientific Engineering and Research, 8, 234–237.
- Sucuoglu, H. S., Bogrekci, I., Demircioglu, P. (2018). Development of Mobile Robot with Sensor Fusion Fire Detection Unit. IFAC-PapersOnLine, 51 (30), 430–435. doi: https://doi.org/10.1016/j.ifacol.2018.11.324
- Aliff, M., Samsiah, N., Yusof, M., Zainal, A. (2019). Development of Fire Fighting Robot (QRob). International Journal of Advanced Computer Science and Applications, 10 (1). doi: https://doi.org/10.14569/ijacsa.2019.0100118
- Chandra, P. S., Revathi, V., Sireesha, A., Kumar, N. S. (2019). Development of DTMF Centred Remotely Located Fire Extinguishing Robot. International Journal of Innovative Technology and Exploring Engineering, 9 (1), 39–43. doi: https://doi.org/10.35940/ijitee.a3902.119119
- Dhiman, A., Shah, N., Adhikari, P., Kumbhar, S., Dhanjal, I., Mehendale, N. (2020). Fire Fighter Robot with Deep Learning and Machine Vision. SSRN Electronic Journal. doi: https://doi.org/10.2139/ssrn.3633609
- Alzubaidi, L., Zhang, J., Humaidi, A. J., Al-Dujaili, A., Duan, Y., Al-Shamma, O. et al. (2021). Review of deep learning: concepts, CNN architectures, challenges, applications, future directions. Journal of Big Data, 8 (1). doi: https://doi.org/10.1186/s40537-021-00444-8
- Liu, H., Lang, B. (2019). Machine Learning and Deep Learning Methods for Intrusion Detection Systems: A Survey. Applied Sciences, 9 (20), 4396. doi: https://doi.org/10.3390/app9204396
- Najafabadi, M. M., Villanustre, F., Khoshgoftaar, T. M., Seliya, N., Wald, R., Muharemagic, E. (2015). Deep learning applications and challenges in big data analytics. Journal of Big Data, 2 (1). doi: https://doi.org/10.1186/s40537-014-0007-7
- Shen, C. (2018). A Transdisciplinary Review of Deep Learning Research and Its Relevance for Water Resources Scientists. Water Resources Research, 54 (11), 8558–8593. doi: https://doi.org/10.1029/2018wr022643
- Atitallah, S. B., Driss, M., Boulila, W., Ghézala, H. B. (2020). Leveraging Deep Learning and IoT big data analytics to support the smart cities development: Review and future directions. Computer Science Review, 38, 100303. doi: https://doi.org/10.1016/j.cosrev.2020.100303
- Wang, W., Liang, D., Chen, Q., Iwamoto, Y., Han, X.-H., Zhang, Q. et al. (2019). Medical Image Classification Using Deep Learning. Deep Learning in Healthcare. Springer, 33–51. doi: https://doi.org/10.1007/978-3-030-32606-7_3
- Papernot, N., McDaniel, P., Jha, S., Fredrikson, M., Celik, Z. B., Swami, A. (2016). The Limitations of Deep Learning in Adversarial Settings. 2016 IEEE European Symposium on Security and Privacy (EuroS&P), 372–387. doi: https://doi.org/10.1109/eurosp.2016.36
- Shorten, C., Khoshgoftaar, T. M., Furht, B. (2021). Deep Learning applications for COVID-19. Journal of Big Data, 8 (1). doi: https://doi.org/10.1186/s40537-020-00392-9
- Udendhran, R., Balamurugan, M., Suresh, A., Varatharajan, R. (2020). Enhancing image processing architecture using deep learning for embedded vision systems. Microprocessors and Microsystems, 76, 103094. doi: https://doi.org/10.1016/j.micpro.2020.103094
- Peng, X., Zhang, X., Li, Y., Liu, B. (2020). Research on image feature extraction and retrieval algorithms based on convolutional neural network. Journal of Visual Communication and Image Representation, 69, 102705. doi: https://doi.org/10.1016/j.jvcir.2019.102705
- Ramprasath, M., Anand, M. V., Hariharan, S. (2018). Image classification using convolutional neural networks. International Journal of Pure and Applied Mathematics, 119 (17), 1307–1319.
- Liang, G., Hong, H., Xie, W., Zheng, L. (2018). Combining Convolutional Neural Network With Recursive Neural Network for Blood Cell Image Classification. IEEE Access, 6, 36188–36197. doi: https://doi.org/10.1109/access.2018.2846685
- Albawi, S., Mohammed, T. A., Al-Zawi, S. (2017). Understanding of a convolutional neural network. 2017 International Conference on Engineering and Technology (ICET). doi: https://doi.org/10.1109/icengtechnol.2017.8308186
- Ajit, A., Acharya, K., Samanta, A. (2020). A Review of Convolutional Neural Networks. 2020 International Conference on Emerging Trends in Information Technology and Engineering (Ic-ETITE). doi: https://doi.org/10.1109/ic-etite47903.2020.049
- Shaheen, F., Verma, B., Asafuddoula, Md. (2016). Impact of Automatic Feature Extraction in Deep Learning Architecture. 2016 International Conference on Digital Image Computing: Techniques and Applications (DICTA). doi: https://doi.org/10.1109/dicta.2016.7797053
- Elangovan, P., Nath, M. K. (2020). Glaucoma assessment from color fundus images using convolutional neural network. International Journal of Imaging Systems and Technology, 31 (2), 955–971. doi: https://doi.org/10.1002/ima.22494
- Elkhayati, M., Elkettani, Y. (2022). UnCNN: A New Directed CNN Model for Isolated Arabic Handwritten Characters Recognition. Arabian Journal for Science and Engineering, 47 (8), 10667–10688. doi: https://doi.org/10.1007/s13369-022-06652-5
- Li, Z., Ren, K., Jiang, X., Li, B., Zhang, H., Li, D. (2022). Domain generalization using pretrained models without fine-tuning. doi: https://doi.org/10.48550/arXiv.2203.04600
- Mehrotra, R., Ansari, M. A., Agrawal, R., Anand, R. S. (2020). A Transfer Learning approach for AI-based classification of brain tumors. Machine Learning with Applications, 2, 100003. doi: https://doi.org/10.1016/j.mlwa.2020.100003
- Yao, Y., Doretto, G. (2010). Boosting for transfer learning with multiple sources. 2010 IEEE Computer Society Conference on Computer Vision and Pattern Recognition, 1855–1862. doi: https://doi.org/10.1109/cvpr.2010.5539857
- Bouhamed, O., Ghazzai, H., Besbes, H., Massoud, Y. (2020). Autonomous UAV Navigation: A DDPG-Based Deep Reinforcement Learning Approach. 2020 IEEE International Symposium on Circuits and Systems (ISCAS). doi: https://doi.org/10.1109/iscas45731.2020.9181245
- Howard, A. G., Zhu, M., Chen, B., Kalenichenko, D., Wang, W., Weyand, T. et al. (2017). Mobilenets: Efficient convolutional neural networks for mobile vision applications. doi: https://doi.org/10.48550/arXiv.1704.04861
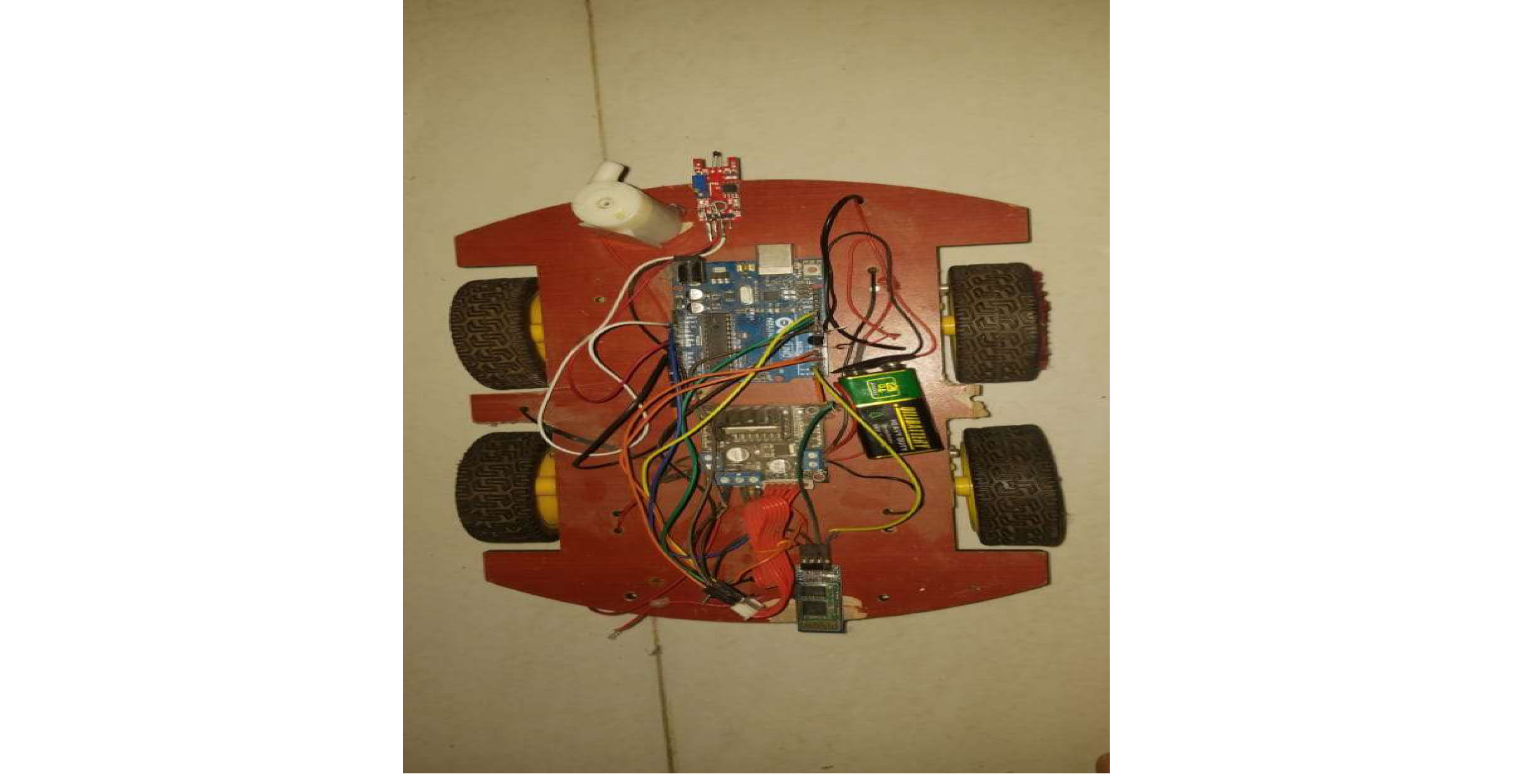
##submission.downloads##
Опубліковано
Як цитувати
Номер
Розділ
Ліцензія
Авторське право (c) 2022 Amin Saif, Gamal Muneer, Yusuf Abdulrahman, Hareth Abdulbaqi, Aiman Abdullah, Abdullah Ali, Abduljalil Derhim

Ця робота ліцензується відповідно до Creative Commons Attribution 4.0 International License.
Закріплення та умови передачі авторських прав (ідентифікація авторства) здійснюється у Ліцензійному договорі. Зокрема, автори залишають за собою право на авторство свого рукопису та передають журналу право першої публікації цієї роботи на умовах ліцензії Creative Commons CC BY. При цьому вони мають право укладати самостійно додаткові угоди, що стосуються неексклюзивного поширення роботи у тому вигляді, в якому вона була опублікована цим журналом, але за умови збереження посилання на першу публікацію статті в цьому журналі.