The improvement of the intelligent decision support system for forecasting non-linear non-stationary processes
DOI:
https://doi.org/10.15587/2706-5448.2023.286516Keywords:
forecasting, non-linear non-stationary processes, decision support system, data uncertainty, system analysis principlesAbstract
The paper is focused on solving the modern scientific and applied problem related to development and practical use in Decision Support Systems (DSS) of information technologies directed towards forecasting of non-linear non-stationary processes (NNP) that take place in economy and finances as well as in many other areas of activities. Thus, object of study are non-linear non-stationary processes taking place in economy and financial sphere.
The basic problem of the study is development of new mathematical models and methods of analysis and forecasting non-linear non-stationary processes in economy and finances, improvement of information decision support technologies that would help to enhance quality of forecast estimates and respective decisions in conditions of uncertainties and risk. The methods given in the paper are used for automating the process of intellectual data analysis that describe the processes under study and automatizing model constructing procedures.
As a result of the study performed the information technology was developed to be used in DSS based upon system analysis principles, taking into consideration possible data uncertainties, regression and intellectual data analysis. The technology provides for constructing adequate models of the process under study and computing high quality forecast estimates. The particular feature of the approach proposed is that it provides for high quality of experimental results due to taking into consideration special features of non-linear non-stationary processes that take place in various spheres of activities and their evolution is influenced by many specific factors.
The use of the technology proposed in decision support systems of enterprises, state governmental organs, and local self-government will create basis for effective solving the tasks of governing development of non-linear non-stationary processes that take place in many spheres of activities. The approaches proposed in the paper can be used in practice as separately as well as parts of existing information systems at enterprises and organizations.
References
- De Gooijer, J. G. (2017). Elements of Nonlinear Time Series Analysis and Forecasting. Cham: Springer, 618. doi: https://doi.org/10.1007/978-3-319-43252-6
- Amendola, A., Francq, C. (2009). Concepts of and tools for Nonlinear Time‐Series Modelling. Handbook of Computational Econometrics. Muenchen: MPRA, 377–427. doi: https://doi.org/10.1002/9780470748916.ch10
- Chai, S., Du, M., Chen, X., Chu, W. (2020). A Hybrid Forecasting Model for Nonstationary and Nonlinear Time Series in the Stochastic Process of CO2 Emission Trading Price Fluctuation. Mathematical Problems in Engineering, 2020, 1–13. doi: https://doi.org/10.1155/2020/8978504
- Moffat, I. U., Akpan, E. A. (2019). Selection of Heteroscedastic Models: A Time Series Forecasting Approach. Applied Mathematics, 10 (5), 333–348. doi: https://doi.org/10.4236/am.2019.105024
- Gustafsson, O. (2020). Some contributions to heteroscedastic time series analysis and computational aspects of Bayesian VARs. Stockholm University.
- Stepchenko, A., Chizhov, J., Aleksejeva, L., Tolujew, J. (2017). Nonlinear, Non-stationary and Seasonal Time Series Forecasting Using Different Methods Coupled with Data Preprocessing. Procedia Computer Science, 104, 578–585. doi: https://doi.org/10.1016/j.procs.2017.01.175
- Ruxanda, G., Opincariu, S., Ionescu, S. (2019). Modelling non-stationary financial time series with input warped Student T-Processes. Romanian Journal of Economic Forecasting, XXII (3), 51–61.
- Dahlhaus, R. (1997). Fitting time series models to nonstationary processes. The Annals of Statistics, 25 (1). doi: https://doi.org/10.1214/aos/1034276620
- Tjøstheim, D. (2020). Some notes on nonlinear cointegration: A partial review with some novel perspectives. Econometric Reviews, 39 (7), 655–673. doi: https://doi.org/10.1080/07474938.2020.1771900
- Wen, J., Li, Y., Wang, J., Sun, W. (2022). Nonstationary Process Monitoring Based on Cointegration Theory and Multiple Order Moments. Processes, 10 (1), 169. doi: https://doi.org/10.3390/pr10010169
- Zeevi, A., Meir, R., Adler, R. J. (1999). Non-Linear Models for Time Series Using Mixtures of Autoregressive Models.
- Chan, N., Wang, Q. (2015). Nonlinear regressions with nonstationary time series. Journal of Econometrics, 185 (1), 182–195. doi: https://doi.org/10.1016/j.jeconom.2014.04.025
- Halva, H., Hyvarinen, A. (2020). Hidden Markov nonlinear ICA: unsupervised learning from nonstationary time series. 36th Conference on Uncertainty in Artificial Intelligence (UAI), 124, 939–948.
- Liu, T., Chen, S., Li, K., Gan, S., Harris, C. J. (2023). Adaptive Multioutput Gradient RBF Tracker for Nonlinear and Nonstationary Regression. IEEE Transactions on Cybernetics, 1–14. doi: https://doi.org/10.1109/tcyb.2023.3235155
- Sichko, T., Neskorodieva, T., Rymar, P. (2022). Methods and models of decision-making in uncertain conditions. Computer Systems and Information Technologies, 3, 47–51. doi: https://doi.org/10.31891/csit-2021-5-6
- Sarukhan, J., Whyte, A. (Eds.) (2005). Recognizing Uncertainties in Evaluating Responses. Whyte Ecosystems and Human Well-being: Policy Responses. Washington, Covelo, London: Island press, 266. Available at: http://pdf.wri.org/ecosystems_human_wellbeing.pdf
- Rounsevell, M. D. A., Arneth, A., Brown, C., Cheung, W. W. L., Gimenez, O., Holman, I. et al. (2021). Identifying uncertainties in scenarios and models of socio-ecological systems in support of decision-making. One Earth, 4 (7), 967–985. doi: https://doi.org/10.1016/j.oneear.2021.06.003
- Dewulf, A., Biesbroek, R. (2018). Nine lives of uncertainty in decision-making: strategies for dealing with uncertainty in environmental governance. Policy and Society, 37 (4), 441–458. doi: https://doi.org/10.1080/14494035.2018.1504484
- Holsapple, C. W., Winston, A. B. (1996). Decision Support Systems. Saint Paul: West Publishing Company, 850.
- Turban, E., Aronson, J. E. (2001). Decision Support Systems. New Jersey: Prentice Hall, 865.
- Islam, A. M. A., Vatn, J. (2023). Condition-based multi-component maintenance decision support under degradation uncertainties. International Journal of System Assurance Engineering and Management. doi: https://doi.org/10.1007/s13198-023-01900-9
- Bidiuk, P., Tymoshchuk, O., Kovalenko, A., Korshevniuk, L. (2022). Systemy i metody pidtrymky pryiniattia rishen. Kyiv: KPI im. Ihoria Sikorskoho, 610.
- Al Eid, A. A., Yavuz, U. (2022). The Effect of Using Decision Support Systems Applications and Business Intelligence Systems in Making Strategic Decisions: A Field Study in the City of Gaziantep. Global Journal of Economics and Business, 12 (2), 256–273. doi: https://doi.org/10.31559/gjeb2022.12.2.8
- Wang, J., Yu, C., Zhang, J. (2019). Constructing the regional intelligent economic decision support system based on fuzzy C-mean clustering algorithm. Soft Computing, 24 (11), 7989–7997. doi: https://doi.org/10.1007/s00500-019-04091-3
- Borra, S., Dey, N., Bhattacharyya, S., Bouhlel, M. S. (Eds.) (2019). Intelligent decision support systems. Intelligent Decision Support Systems: Applications in Signal Processing. Berlin, Boston: De Gruyter, 183. doi: https://doi.org/10.1515/9783110621105
- Zghurovskyi, M. Z., Bidiuk, P. I., Terentiiev, O. M., Prosiankina-Zharova, T. I. (2015). Baiiesivski merezhi v systemakh pidtrymky pryiniattia rishen. Kyiv: TOV «Vydavnyche pidpryiemstvo «Edelveis», 300.
- Jia, T., Wang, C., Tian, Z., Wang, B., Tian, F. (2022). Design of Digital and Intelligent Financial Decision Support System Based on Artificial Intelligence. Computational Intelligence and Neuroscience, 2022, 1–7. doi: https://doi.org/10.1155/2022/1962937
- Jung, D., Tran Tuan, V., Quoc Tran, D., Park, M., Park, S. (2020). Conceptual Framework of an Intelligent Decision Support System for Smart City Disaster Management. Applied Sciences, 10 (2), 666. doi: https://doi.org/10.3390/app10020666
- Zhai, Z., Martínez, J. F., Beltran, V., Martínez, N. L. (2020). Decision support systems for agriculture 4.0: Survey and challenges. Computers and Electronics in Agriculture, 170, 105256. doi: https://doi.org/10.1016/j.compag.2020.105256
- Yazan, D. M., van Capelleveen, G., Fraccascia, L. (2022). Decision-Support Tools for Smart Transition to Circular Economy. Smart Industry – Better Management, 151–169. doi: https://doi.org/10.1108/s1877-636120220000028010
- Alasiri, M. M., Salameh, A. A. (2020). The impact of business intelligence (BI) and decision support systems (DSS): exploratory study. International Journal of Management, 11 (5), 1001–1016.
- Wojcik, W., Smolarz, A. (Eds.) (2017). Information Technology in Medical Diagnostics. London: CRC Press/Balkema, 209. doi: https://doi.org/10.1201/9781315098050
- Le Pontois, S., Jaillot, M. (2021). Activating Creativity in Situations of Uncertainty: The Role of Third Spaces. Journal of Innovation Economics & Management, 36 (3), 33–62. doi: https://doi.org/10.3917/jie.pr1.0102
- Rutkowsky, L. (2005). Metody i Techniki Sztucznej Inteligencji. Warszawa: PWN, 520.
- Sugumaran, V. (2002). Intelligent Support Systems Technology: Knowledge Management. London: IRM Press, 318.
- Semar-Bitah, K., Tarabet, C., Mouzai, M., Chetibi, A. (2019). Scalable Proposed Decision Support System. Proceedings of the 9th International Conference on Information Systems and Technologies. doi: https://doi.org/10.1145/3361570.3361613
- Gembarski, P. C., Plappert, S., Lachmayer, R. (2021). Making design decisions under uncertainties: probabilistic reasoning and robust product design. Journal of Intelligent Information Systems, 57 (3), 563–581. doi: https://doi.org/10.1007/s10844-021-00665-6
- Morris, J., Reilly, J., Paltsev, S., Sokolov, A., Cox, K. (2022). Representing Socio‐Economic Uncertainty in Human System Models. Earth’s Future, 10 (4). doi: https://doi.org/10.1029/2021ef002239
- Cunha, M. da C. (2023). Water and Environmental Systems Management Under Uncertainty: From Scenario Construction to Robust Solutions and Adaptation. Water Resources Management, 37 (6-7), 2271–2285. doi: https://doi.org/10.1007/s11269-023-03429-9
- Pole, A., West, M., Harrison, J. (1994). Applied Bayesian Forecasting and Time Series Analysis. Boca Raton: Chapman&Hall, 408.
- SAS Institute. Available at: https://www.sas.com/ru_ua/home.html
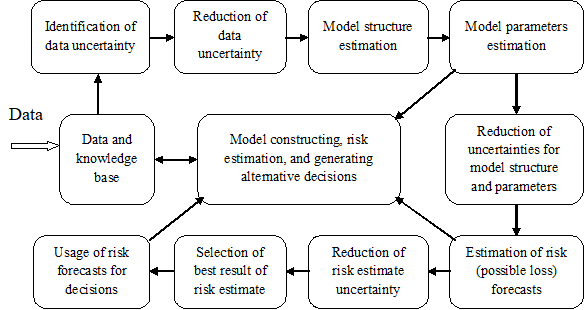
Downloads
Published
How to Cite
Issue
Section
License
Copyright (c) 2023 Petro Bidiuk, Tetyana Prosyankina-Zharova, Valerii Diakon, Dmytro Diakon

This work is licensed under a Creative Commons Attribution 4.0 International License.
The consolidation and conditions for the transfer of copyright (identification of authorship) is carried out in the License Agreement. In particular, the authors reserve the right to the authorship of their manuscript and transfer the first publication of this work to the journal under the terms of the Creative Commons CC BY license. At the same time, they have the right to conclude on their own additional agreements concerning the non-exclusive distribution of the work in the form in which it was published by this journal, but provided that the link to the first publication of the article in this journal is preserved.