Удосконалення інтелектуальних систем підтримки прийняття рішень для прогнозування нелінійних нестаціонарних процесів
DOI:
https://doi.org/10.15587/2706-5448.2023.286516Ключові слова:
прогнозування, нелінійні нестаціонарні процеси, система підтримки прийняття рішень, невизначеність даних, принципи системного аналізуАнотація
Робота присвячена перспективній науково-прикладній задачі розроблення та використання у системах підтримки прийняття рішень інформаційних технологій прогнозування нелінійних нестаціонарних процесів (ННП), що мають місце в економіці та фінансах, а також у багатьох інших галузях діяльності. Таким чином, об’єктом дослідження є нелінійні нестаціонарні процеси, що мають місце в економіці та фінансовій сфері.
Основним напрямком дослідження є створення нових математичних моделей та методів аналізу та прогнозування нелінійних нестаціонарних процесів в економіці та фінансах, удосконалення інформаційних технологій підтримки прийняття рішень, що дозволить підвищити якість оцінок прогнозів та відповідних рішень в умовах невизначеності та ризику. Методи, що представлені в роботі, призначені для автоматизації процесу інтелектуального аналізу даних, що описують досліджувані процеси, та автоматизації процедур побудови моделей.
В результаті виконаних досліджень запропоновано інформаційну технологію, призначену для використання у системах підтримки прийняття рішень, побудованих на основі системного підходу, врахування невизначеностей, регресійного та інтелектуального аналізу даних, що забезпечує побудову адекватних моделей досліджуваних процесів і отримання високоякісних оцінок прогнозів. Особливістю пропонованого підходу, яка дозволяє забезпечувати якість отримуваних результатів є те, що в ній враховано особливості нелінійних нестаціонарних процесів, що мають місце у різних сферах діяльності, адже вони мають особливості розвитку та протікають під впливом багатьох специфічних факторів.
Використання такої технології в системах підтримки прийняття рішень як підприємств, так і органів державного управління та місцевого самоврядування створюватиме підґрунтя для вирішення завдань управління розвитком досліджуваних нелінійних нестаціонарних процесів, що протікають у багатьох сферах діяльності. Підходи, пропоновані в роботі, можуть бути використані на практиці як окремо, так і в складі існуючих інформаційних систем підприємств, установ, організацій.
Посилання
- De Gooijer, J. G. (2017). Elements of Nonlinear Time Series Analysis and Forecasting. Cham: Springer, 618. doi: https://doi.org/10.1007/978-3-319-43252-6
- Amendola, A., Francq, C. (2009). Concepts of and tools for Nonlinear Time‐Series Modelling. Handbook of Computational Econometrics. Muenchen: MPRA, 377–427. doi: https://doi.org/10.1002/9780470748916.ch10
- Chai, S., Du, M., Chen, X., Chu, W. (2020). A Hybrid Forecasting Model for Nonstationary and Nonlinear Time Series in the Stochastic Process of CO2 Emission Trading Price Fluctuation. Mathematical Problems in Engineering, 2020, 1–13. doi: https://doi.org/10.1155/2020/8978504
- Moffat, I. U., Akpan, E. A. (2019). Selection of Heteroscedastic Models: A Time Series Forecasting Approach. Applied Mathematics, 10 (5), 333–348. doi: https://doi.org/10.4236/am.2019.105024
- Gustafsson, O. (2020). Some contributions to heteroscedastic time series analysis and computational aspects of Bayesian VARs. Stockholm University.
- Stepchenko, A., Chizhov, J., Aleksejeva, L., Tolujew, J. (2017). Nonlinear, Non-stationary and Seasonal Time Series Forecasting Using Different Methods Coupled with Data Preprocessing. Procedia Computer Science, 104, 578–585. doi: https://doi.org/10.1016/j.procs.2017.01.175
- Ruxanda, G., Opincariu, S., Ionescu, S. (2019). Modelling non-stationary financial time series with input warped Student T-Processes. Romanian Journal of Economic Forecasting, XXII (3), 51–61.
- Dahlhaus, R. (1997). Fitting time series models to nonstationary processes. The Annals of Statistics, 25 (1). doi: https://doi.org/10.1214/aos/1034276620
- Tjøstheim, D. (2020). Some notes on nonlinear cointegration: A partial review with some novel perspectives. Econometric Reviews, 39 (7), 655–673. doi: https://doi.org/10.1080/07474938.2020.1771900
- Wen, J., Li, Y., Wang, J., Sun, W. (2022). Nonstationary Process Monitoring Based on Cointegration Theory and Multiple Order Moments. Processes, 10 (1), 169. doi: https://doi.org/10.3390/pr10010169
- Zeevi, A., Meir, R., Adler, R. J. (1999). Non-Linear Models for Time Series Using Mixtures of Autoregressive Models.
- Chan, N., Wang, Q. (2015). Nonlinear regressions with nonstationary time series. Journal of Econometrics, 185 (1), 182–195. doi: https://doi.org/10.1016/j.jeconom.2014.04.025
- Halva, H., Hyvarinen, A. (2020). Hidden Markov nonlinear ICA: unsupervised learning from nonstationary time series. 36th Conference on Uncertainty in Artificial Intelligence (UAI), 124, 939–948.
- Liu, T., Chen, S., Li, K., Gan, S., Harris, C. J. (2023). Adaptive Multioutput Gradient RBF Tracker for Nonlinear and Nonstationary Regression. IEEE Transactions on Cybernetics, 1–14. doi: https://doi.org/10.1109/tcyb.2023.3235155
- Sichko, T., Neskorodieva, T., Rymar, P. (2022). Methods and models of decision-making in uncertain conditions. Computer Systems and Information Technologies, 3, 47–51. doi: https://doi.org/10.31891/csit-2021-5-6
- Sarukhan, J., Whyte, A. (Eds.) (2005). Recognizing Uncertainties in Evaluating Responses. Whyte Ecosystems and Human Well-being: Policy Responses. Washington, Covelo, London: Island press, 266. Available at: http://pdf.wri.org/ecosystems_human_wellbeing.pdf
- Rounsevell, M. D. A., Arneth, A., Brown, C., Cheung, W. W. L., Gimenez, O., Holman, I. et al. (2021). Identifying uncertainties in scenarios and models of socio-ecological systems in support of decision-making. One Earth, 4 (7), 967–985. doi: https://doi.org/10.1016/j.oneear.2021.06.003
- Dewulf, A., Biesbroek, R. (2018). Nine lives of uncertainty in decision-making: strategies for dealing with uncertainty in environmental governance. Policy and Society, 37 (4), 441–458. doi: https://doi.org/10.1080/14494035.2018.1504484
- Holsapple, C. W., Winston, A. B. (1996). Decision Support Systems. Saint Paul: West Publishing Company, 850.
- Turban, E., Aronson, J. E. (2001). Decision Support Systems. New Jersey: Prentice Hall, 865.
- Islam, A. M. A., Vatn, J. (2023). Condition-based multi-component maintenance decision support under degradation uncertainties. International Journal of System Assurance Engineering and Management. doi: https://doi.org/10.1007/s13198-023-01900-9
- Bidiuk, P., Tymoshchuk, O., Kovalenko, A., Korshevniuk, L. (2022). Systemy i metody pidtrymky pryiniattia rishen. Kyiv: KPI im. Ihoria Sikorskoho, 610.
- Al Eid, A. A., Yavuz, U. (2022). The Effect of Using Decision Support Systems Applications and Business Intelligence Systems in Making Strategic Decisions: A Field Study in the City of Gaziantep. Global Journal of Economics and Business, 12 (2), 256–273. doi: https://doi.org/10.31559/gjeb2022.12.2.8
- Wang, J., Yu, C., Zhang, J. (2019). Constructing the regional intelligent economic decision support system based on fuzzy C-mean clustering algorithm. Soft Computing, 24 (11), 7989–7997. doi: https://doi.org/10.1007/s00500-019-04091-3
- Borra, S., Dey, N., Bhattacharyya, S., Bouhlel, M. S. (Eds.) (2019). Intelligent decision support systems. Intelligent Decision Support Systems: Applications in Signal Processing. Berlin, Boston: De Gruyter, 183. doi: https://doi.org/10.1515/9783110621105
- Zghurovskyi, M. Z., Bidiuk, P. I., Terentiiev, O. M., Prosiankina-Zharova, T. I. (2015). Baiiesivski merezhi v systemakh pidtrymky pryiniattia rishen. Kyiv: TOV «Vydavnyche pidpryiemstvo «Edelveis», 300.
- Jia, T., Wang, C., Tian, Z., Wang, B., Tian, F. (2022). Design of Digital and Intelligent Financial Decision Support System Based on Artificial Intelligence. Computational Intelligence and Neuroscience, 2022, 1–7. doi: https://doi.org/10.1155/2022/1962937
- Jung, D., Tran Tuan, V., Quoc Tran, D., Park, M., Park, S. (2020). Conceptual Framework of an Intelligent Decision Support System for Smart City Disaster Management. Applied Sciences, 10 (2), 666. doi: https://doi.org/10.3390/app10020666
- Zhai, Z., Martínez, J. F., Beltran, V., Martínez, N. L. (2020). Decision support systems for agriculture 4.0: Survey and challenges. Computers and Electronics in Agriculture, 170, 105256. doi: https://doi.org/10.1016/j.compag.2020.105256
- Yazan, D. M., van Capelleveen, G., Fraccascia, L. (2022). Decision-Support Tools for Smart Transition to Circular Economy. Smart Industry – Better Management, 151–169. doi: https://doi.org/10.1108/s1877-636120220000028010
- Alasiri, M. M., Salameh, A. A. (2020). The impact of business intelligence (BI) and decision support systems (DSS): exploratory study. International Journal of Management, 11 (5), 1001–1016.
- Wojcik, W., Smolarz, A. (Eds.) (2017). Information Technology in Medical Diagnostics. London: CRC Press/Balkema, 209. doi: https://doi.org/10.1201/9781315098050
- Le Pontois, S., Jaillot, M. (2021). Activating Creativity in Situations of Uncertainty: The Role of Third Spaces. Journal of Innovation Economics & Management, 36 (3), 33–62. doi: https://doi.org/10.3917/jie.pr1.0102
- Rutkowsky, L. (2005). Metody i Techniki Sztucznej Inteligencji. Warszawa: PWN, 520.
- Sugumaran, V. (2002). Intelligent Support Systems Technology: Knowledge Management. London: IRM Press, 318.
- Semar-Bitah, K., Tarabet, C., Mouzai, M., Chetibi, A. (2019). Scalable Proposed Decision Support System. Proceedings of the 9th International Conference on Information Systems and Technologies. doi: https://doi.org/10.1145/3361570.3361613
- Gembarski, P. C., Plappert, S., Lachmayer, R. (2021). Making design decisions under uncertainties: probabilistic reasoning and robust product design. Journal of Intelligent Information Systems, 57 (3), 563–581. doi: https://doi.org/10.1007/s10844-021-00665-6
- Morris, J., Reilly, J., Paltsev, S., Sokolov, A., Cox, K. (2022). Representing Socio‐Economic Uncertainty in Human System Models. Earth’s Future, 10 (4). doi: https://doi.org/10.1029/2021ef002239
- Cunha, M. da C. (2023). Water and Environmental Systems Management Under Uncertainty: From Scenario Construction to Robust Solutions and Adaptation. Water Resources Management, 37 (6-7), 2271–2285. doi: https://doi.org/10.1007/s11269-023-03429-9
- Pole, A., West, M., Harrison, J. (1994). Applied Bayesian Forecasting and Time Series Analysis. Boca Raton: Chapman&Hall, 408.
- SAS Institute. Available at: https://www.sas.com/ru_ua/home.html
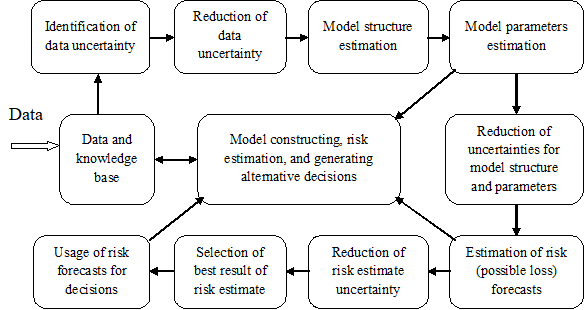
##submission.downloads##
Опубліковано
Як цитувати
Номер
Розділ
Ліцензія
Авторське право (c) 2023 Petro Bidiuk, Tetyana Prosyankina-Zharova, Valerii Diakon, Dmytro Diakon

Ця робота ліцензується відповідно до Creative Commons Attribution 4.0 International License.
Закріплення та умови передачі авторських прав (ідентифікація авторства) здійснюється у Ліцензійному договорі. Зокрема, автори залишають за собою право на авторство свого рукопису та передають журналу право першої публікації цієї роботи на умовах ліцензії Creative Commons CC BY. При цьому вони мають право укладати самостійно додаткові угоди, що стосуються неексклюзивного поширення роботи у тому вигляді, в якому вона була опублікована цим журналом, але за умови збереження посилання на першу публікацію статті в цьому журналі.