Evaluation of the efficiency and accuracy of the system for collecting and processing EMG signals obtained using a bracelet
DOI:
https://doi.org/10.15587/2706-5448.2024.306428Keywords:
bracelet, electromyography, bionic prosthesis, data acquisition system, signal processing, machine learning algorithmsAbstract
The object of research is a bracelet that uses the electromyography (EMG) method to control a bionic prosthesis. In the conditions of the development of modern biomedical technologies and robotics, such a system becomes key to improving the quality of life of people with disabilities, providing efficient and accurate control of prostheses. The problem addressed in the research is the development and analysis of a bionic prosthesis control system using a bracelet using the EMG method. The main focus is on the optimization of data collection and processing processes, as well as the development of machine learning algorithms for gesture recognition in order to improve the accuracy and efficiency of prosthetic control.
The essence of the obtained results is the development and testing of a new bionic prosthesis control system that uses EMG signals obtained with the help of a bracelet. The study showed that the classifier based on the support vector method outperforms other algorithms such as neural networks and decision trees, achieving an average accuracy of 90 %. The obtained data were successfully filtered and subjected to feature extraction, which allowed to create effective gesture recognition algorithms. The system was tested in real time, which confirmed its high accuracy and efficiency.
The proposed system includes an innovative bracelet for collecting EMG data, which are then processed and analyzed using modern machine learning algorithms. The innovativeness of the proposed approach lies not only in the high accuracy of gesture recognition, but also in the possibility of adapting the system to different types of bionic prostheses and operating conditions. This is achieved by using a classifier based on the support vector method, which demonstrates significantly higher accuracy compared to other algorithms such as neural networks and decision trees. The test results show an average accuracy of 92.5 %, which confirms the high efficiency of the system.
The use of this system involves the intensive use of EMG sensors, which allows more accurate determination of the user's intentions regarding the control of the prosthesis. This, in turn, contributes to the improvement of the quality of life of users, providing them with greater functionality and convenience in the use of bionic prostheses.
References
- Hye, N. M., Hany, U., Chakravarty, S., Akter, L., Ahmed, I. (2023). Artificial Intelligence for sEMG-Based Muscular Movement Recognition for Hand Prosthesis. IEEE Access, 11, 38850–38863. doi: https://doi.org/10.1109/access.2023.3267674
- Noce, E., Dellacasa Bellingegni, A., Ciancio, A. L., Sacchetti, R., Davalli, A., Guglielmelli, E., Zollo, L. (2019). EMG and ENG-envelope pattern recognition for prosthetic hand control. Journal of Neuroscience Methods, 311, 38–46. doi: https://doi.org/10.1016/j.jneumeth.2018.10.004
- Beyrouthy, T., Al Kork, S. K., Korbane, J. A., Abdulmonem, A. (2016). EEG Mind controlled Smart Prosthetic Arm. 2016 IEEE International Conference on Emerging Technologies and Innovative Business Practices for the Transformation of Societies (EmergiTech). doi: https://doi.org/10.1109/emergitech.2016.7737375
- Shahsavari, H., Matourypour, P., Ghiyasvandian, S., Ghorbani, A., Bakhshi, F., Mahmoudi, M., Golestannejad, M. (2020). Upper limb amputation; Care needs for reintegration to life: An integrative review. International Journal of Orthopaedic and Trauma Nursing, 38, 100773. doi: https://doi.org/10.1016/j.ijotn.2020.100773
- D’Anna, E., Valle, G., Mazzoni, A., Strauss, I., Iberite, F., Patton, J. et al. (2019). A closed-loop hand prosthesis with simultaneous intraneural tactile and position feedback. Science Robotics, 4 (27). doi: https://doi.org/10.1126/scirobotics.aau8892
- Zhang, Yang, Qian, Zhang. (2019). Real-Time Surface EMG Pattern Recognition for Hand Gestures Based on an Artificial Neural Network. Sensors, 19 (14), 3170. doi: https://doi.org/10.3390/s19143170
- Bi, L., Feleke, A., Guan, C. (2019). A review on EMG-based motor intention prediction of continuous human upper limb motion for human-robot collaboration. Biomedical Signal Processing and Control, 51, 113–127. doi: https://doi.org/10.1016/j.bspc.2019.02.011
- Zhang, Y., Xiao, R., Harrison, C. (2016). Advancing Hand Gesture Recognition with High Resolution Electrical Impedance Tomography. Proceedings of the 29th Annual Symposium on User Interface Software and Technology. Tokyo, 843–850. doi: https://doi.org/10.1145/2984511.2984574
- Maat, B., Smit, G., Plettenburg, D., Breedveld, P. (2018). Passive prosthetic hands and tools. Prosthetics & Orthotics International, 42 (1), 66–74. doi: https://doi.org/10.1177/0309364617691622
- Shi, W.-T., Lyu, Z.-J., Tang, S.-T., Chia, T.-L., Yang, C.-Y. (2018). A bionic hand controlled by hand gesture recognition based on surface EMG signals: A preliminary study. Biocybernetics and Biomedical Engineering, 38 (1), 126–135. doi: https://doi.org/10.1016/j.bbe.2017.11.001
- Unanyan, N. N., Belov, A. A. (2020). A Real-Time Fail-Safe Algorithm for Decoding of Myoelectric Signals to Control a Prosthetic Arm. 2020 21th International Carpathian Control Conference (ICCC). doi: https://doi.org/10.1109/iccc49264.2020.9257287
- Abdhul, A. A., Subramani, D., Ganesan, J., Subramaniam, S., Dharani, K. G. (2020). Design and Development of EMG Based Prosthetic Arm. 2020 6th International Conference on Advanced Computing and Communication Systems (ICACCS). doi: https://doi.org/10.1109/icaccs48705.2020.9074206
- Prakash, A., Sahi, A. K., Sharma, N., Sharma, S. (2020). Force myography controlled multifunctional hand prosthesis for upper-limb amputees. Biomedical Signal Processing and Control, 62, 102122. doi: https://doi.org/10.1016/j.bspc.2020.102122
- Li, X., Fu, J., Xiong, L., Shi, Y., Davoodi, R., Li, Y. (2015). Identification of finger force and motion from forearm surface electromyography. Proceedings of the IEEE International Conference on Multisensor Fusion and Integration for Intelligent Systems (MFI). San Diego, 316–321. doi: https://doi.org/10.1109/mfi.2015.7295827
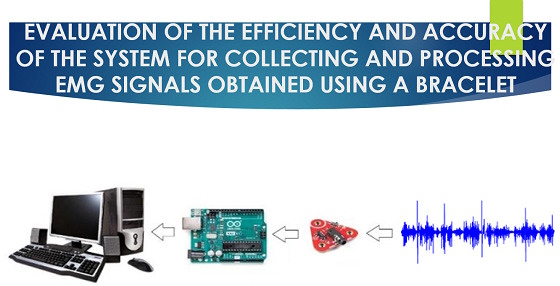
Downloads
Published
How to Cite
Issue
Section
License
Copyright (c) 2024 Ruslan Bilyi

This work is licensed under a Creative Commons Attribution 4.0 International License.
The consolidation and conditions for the transfer of copyright (identification of authorship) is carried out in the License Agreement. In particular, the authors reserve the right to the authorship of their manuscript and transfer the first publication of this work to the journal under the terms of the Creative Commons CC BY license. At the same time, they have the right to conclude on their own additional agreements concerning the non-exclusive distribution of the work in the form in which it was published by this journal, but provided that the link to the first publication of the article in this journal is preserved.