Розробка апаратно-програмного рішення для виявлення об'єктів складних форм у відеопотоці
DOI:
https://doi.org/10.15587/2706-5448.2024.319799Ключові слова:
комп’ютерний зір, одноплатний комп’ютер, система ініціалізації, подвійна перевірка, алгоритми обробки відеоАнотація
Об’єктом дослідження є процес діагностики об’єктів складних форм у відеопотоці. У роботі досліджується прикладна проблема створення апаратно-програмного рішення для виявлення об’єктів складних форм у відеопотоці. У якості апаратного забезпечення використано одноплатні комп’ютери Raspberry Pi моделей 4 та 5 з додатковими модулями UPS HAT (D) та акумуляторами 21700, що забезпечують роботу при відсутності електропостачання. До одноплатних комп’ютерів підключалися Serial Camera Interface камери й вебкамерами Full HD 1080р для вивчення ефективних способів обробки відео за кількома досліджуваними архітектурами обробки відео. Розглянуто вісім архітектур обробки відео на основі методів Oriented Features from Accelerated Segment Test and Rotated Binary Robust Independent Elementary Features та Scale-Invariant Feature Transform. Кожна архітектура обробки відео піддавалася тестуванню за допомогою однохвилинного відео, де визначалася її середня продуктивність. Обмеженнями обробки відео був регіон інтересу розмірами 400x300 пікселів та наявність обмеженої кількості еталонних зображень. Для автоматизації запуску програм на одноплатних комп’ютерах використовувалася система ініціалізації systemd.
Розглянуто відомі алгоритми обробки відео та запропоновано модифікацію алгоритму шляхом використання подвійної перевірки наявності об’єкта у відеопотоці. Реалізовано апаратно-програмне рішення, що складається з одноплатного комп’ютера з підключеними до нього зовнішніми камерами та створено програмне забезпечення виявлення об’єктів складних форм у відеопотоці. Рішення є корисним як допоміжний інструмент для виявлення об’єктів складних форм у відеопотоці на робототехнічних платформах, у промисловості, побуті, навчальному процесі, при ремонті електронних модулів. Практичне значення дослідження полягає в тому, що набули подальшого розвитку архітектури обробки об’єктів складних форм. Вони передбачають здійснення подвійної перевірки наявності об’єкта у відеопотоці, що збільшує час обробки одного кадру, а з іншої сторони – підвищує ефективність детекції об’єктів на основі лише одного еталонного фото.
Посилання
- Guo, J., Shao, M., Chen, X., Yang, Y., Sun, E. (2024). Research on night-time vehicle target detection based on improved KSC-YOLO V5. Signal, Image and Video Processing, 19 (1). https://doi.org/10.1007/s11760-024-03576-5
- Krasnobayev, V., Yanko, A., Fil, I. (2024). Fault-Tolerant Operation of an Integer Data Processing System. Mathematical Modeling and Simulation of Systems. Springer, 172–185. https://doi.org/10.1007/978-3-031-67348-1_13
- Krasnobayev, V., Yanko, A., Kovalchuk, D., Fil, I. (2024). Synthesis of a Mathematical Model of a Fault-Tolerant Real-Time Computer System Operating in Non-positional Arithmetic in Residual Classes. Mathematical Modeling and Simulation of Systems. Springer, 186–199. https://doi.org/10.1007/978-3-031-67348-1_14
- Krasnobayev, V., Yanko, A., Hlushko, A., Kruk, O., Kruk, O., Gakh, V. (2023). Cyberspace protection system based on the data comparison method. Economic and cyber security. Kharkiv: PC TECHNOLOGY CENTER, 3–29. https://doi.org/10.15587/978-617-7319-98-5.ch1
- Pushkarenko, Y., Zaslavskyi, V. (2024). Research on the state of areas in Ukraine affected by military actions based on remote sensing data and deep learning architectures. Radioelectronic and Computer Systems, 2024 (2), 5–18. https://doi.org/10.32620/reks.2024.2.01
- Ultralytics YOLO Docs. Available at: https://docs.ultralytics.com
- Liu, W., Anguelov, D., Erhan, D., Szegedy, C., Reed, S., Fu, C.-Y., Berg, A.; C. Leibe, B., Matas, J., Sebe, N., Welling, M. (Eds.) (2016). SSD: Single Shot MultiBox Detector. Computer Vision – ECCV 2016. Cham: Springer, 21–37. https://doi.org/10.1007/978-3-319-46448-0_2
- Svistun, L., Glushko, А., Shtepenko, K. (2018). Organizational Aspects of Development Projects Implementation at the Real Estate Market in Ukraine. International Journal of Engineering & Technology, 7 (3.2), 447. https://doi.org/10.14419/ijet.v7i3.2.14569
- Ran, Y., Xu, X. (2020). Point cloud registration method based on SIFT and geometry feature. Optik, 203, 163902. https://doi.org/10.1016/j.ijleo.2019.163902
- Singh, P. K., Jana, B., Datta, K. (2022). Robust data hiding scheme through distinct keypoint selection exploiting modified Bilateral-Laplacian SIFT with encoding pipeline. Displays, 74, 102268. https://doi.org/10.1016/j.displa.2022.102268
- Sun, Y. (2024). Exploration on Data Collection and Analysis System Based on Integrated SIFT Algorithm. Procedia Computer Science, 243, 388–395. https://doi.org/10.1016/j.procs.2024.09.048
- Yu, Q., Ni, D., Jiang, Y., Yan, Y., An, J., Sun, T. (2021). Universal SAR and optical image registration via a novel SIFT framework based on nonlinear diffusion and a polar spatial-frequency descriptor. ISPRS Journal of Photogrammetry and Remote Sensing, 171, 1–17. https://doi.org/10.1016/j.isprsjprs.2020.10.019
- Li, Y., Li, Q., Liu, Y., Xie, W. (2019). A spatial-spectral SIFT for hyperspectral image matching and classification. Pattern Recognition Letters, 127, 18–26. https://doi.org/10.1016/j.patrec.2018.08.032
- Pavithra, S., Shreyashwini, N. K., Bhavana, H. S., Nikhitha, G., Kavitha, T. (2023). Hand-Drawn Electronic Component Recognition Using ORB. Procedia Computer Science, 218, 504–513. https://doi.org/10.1016/j.procs.2023.01.032
- Belmessaoud, N. M., Bentoutou, Y., Chikr El-Mezouar, M. (2022). FPGA implementation of feature detection and matching using ORB. Microprocessors and Microsystems, 94, 104666. https://doi.org/10.1016/j.micpro.2022.104666
- Sharif, H., Hölzel, M. (2017). A comparison of prefilters in ORB-based object detection. Pattern Recognition Letters, 93, 154–161. https://doi.org/10.1016/j.patrec.2016.11.007
- A, V., Cholin, A. S., Bhat, A. D., Murthy, K. N. B., Natarajan, S. (2018). An Efficient ORB based Face Recognition framework for Human-Robot Interaction. Procedia Computer Science, 133, 913–923. https://doi.org/10.1016/j.procs.2018.07.095
- Taranco, R., Arnau, J.-M., González, A. (2023). LOCATOR: Low-power ORB accelerator for autonomous cars. Journal of Parallel and Distributed Computing, 174, 32–45. https://doi.org/10.1016/j.jpdc.2022.12.005
- Wu, T., Hung, I.-K., Xu, H., Yang, L., Wang, Y., Fang, L., Lou, X. (2022). An Optimized SIFT-OCT Algorithm for Stitching Aerial Images of a Loblolly Pine Plantation. Forests, 13 (9), 1475. https://doi.org/10.3390/f13091475
- Khudov, H., Hridasov, I., Khizhnyak, I., Yuzova, I., Solomonenko, Y. (2024). Segmentation of image from a first-person-view unmanned aerial vehicle based on a simple ant algorithm. Information and Controlling System, 4 (9 (130)), 44–55. https://doi.org/10.15587/1729-4061.2024.310372
- Rebrov, V., Lukin, V. (2023). Post-processing of compressed noisy images by BM3D filter. Radioelectronic and Computer Systems, 4, 100–111. https://doi.org/10.32620/reks.2023.4.09
- Bilozerskyi, V., Dergachov, K., Krasnov, L., Zymovin, A., Popov, A. (2023). New method for video stream brightness stabilization: algorithms and performance evaluation. Radioelectronic and Computer Systems, 3, 125–135. https://doi.org/10.32620/reks.2023.3.10
- Shefer, O., Laktionov, O., Pents, V., Hlushko, A., Kuchuk, N. (2024). Practical principles of integrating artificial intelligence into the technology of regional security predicting. Advanced Information Systems, 8 (1), 86–93. https://doi.org/10.20998/2522-9052.2024.1.11
- Polshchykov, K., Zdorenko, Y., Masesov, M. (2015). Neuro-fuzzy system for prediction of telecommunication channel load. 2015 Second International Scientific-Practical Conference Problems of Infocommunications Science and Technology (PIC S&T). Kharkiv, 33–34. https://doi.org/10.1109/infocommst.2015.7357261
- Onyshchenko, S., Haitan, O., Yanko, A., Zdorenko, Yu., Rudenko, O. (2024). Method for detection of the modified DDoS cyber attacks on a web resource of an Information and Telecommunication Network based on the use of intelligent systems. Proceedings of the Modern Data Science Technologies Workshop (MoDaST 2024). Lviv, 219–235. Available at: https://ceur-ws.org/Vol-3723/paper12.pdf
- Rublee, E., Rabaud, V., Konolige, K., Bradski, G. (2011). ORB: An efficient alternative to SIFT or SURF. 2011 International Conference on Computer Vision, 2564–2571. https://doi.org/10.1109/iccv.2011.6126544
- Lowe, D. G. (1999). Object recognition from local scale-invariant features. Proceedings of the Seventh IEEE International Conference on Computer Vision, 1150–1157. https://doi.org/10.1109/iccv.1999.790410
- Muja, M., Lowe, D. G. (2009). Fast approximate nearest neighbors with automatic algorithm configuration. International Conference on Computer Vision Theory and Application (VISSAPP), 331–340. https://doi.org/10.5220/0001787803310340
- Lowe, D. G. (2004). Distinctive Image Features from Scale-Invariant Keypoints. International Journal of Computer Vision, 60 (2), 91–110. https://doi.org/10.1023/b:visi.0000029664.99615.94
- Visual Studio Code. Available at: https://code.visualstudio.com/
- Männel, W. (2023). The ultimate guide on using systemd to autostart scripts on the Raspberry Pi. TheDigitalPictureFrame.com. Available at: https://www.thedigitalpictureframe.com/ultimate-guide-systemd-autostart-scripts-raspberry-pi/
- Laktionov, O., Yanko, A., Pedchenko, N. (2024). Identification of air targets using a hybrid clustering algorithm. Eastern-European Journal of Enterprise Technologies, 5 (4 (131)), 89–95. https://doi.org/10.15587/1729-4061.2024.314289
- Fedorenko, G., Fesenko, H., Kharchenko, V. (2022). Analysis of methods and development of the concept of guaranteed detection and recognition of explosive objects. Innovative Technologies and Scientific Solutions for Industries, 4 (22), 20–31. https://doi.org/10.30837/itssi.2022.22.020
- Sun, Y., Fesenko, H., Kharchenko, V., Zhong, L., Kliushnikov, I., Illiashenko, O., Morozova, O., Sachenko, A. (2022). UAV and IoT-Based Systems for the Monitoring of Industrial Facilities Using Digital Twins: Methodology, Reliability Models, and Application. Sensors, 22 (17), 6444. https://doi.org/10.3390/s22176444
- MultiWii. MultiWii flight controller source code and documentation. Available at: https://github.com/multiwii
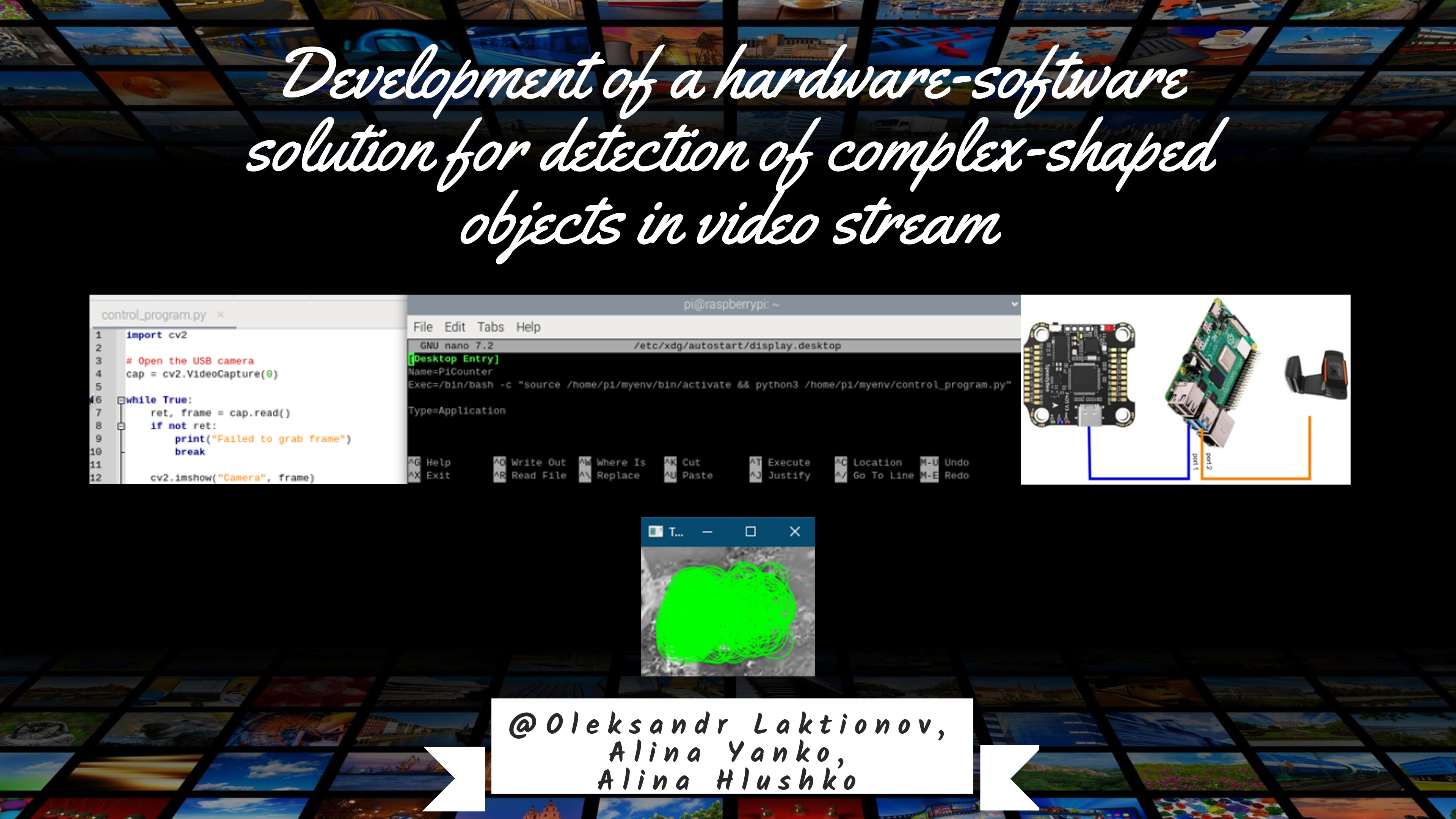
##submission.downloads##
Опубліковано
Як цитувати
Номер
Розділ
Ліцензія
Авторське право (c) 2024 Oleksandr Laktionov, Alina Yanko, Alina Hlushko

Ця робота ліцензується відповідно до Creative Commons Attribution 4.0 International License.
Закріплення та умови передачі авторських прав (ідентифікація авторства) здійснюється у Ліцензійному договорі. Зокрема, автори залишають за собою право на авторство свого рукопису та передають журналу право першої публікації цієї роботи на умовах ліцензії Creative Commons CC BY. При цьому вони мають право укладати самостійно додаткові угоди, що стосуються неексклюзивного поширення роботи у тому вигляді, в якому вона була опублікована цим журналом, але за умови збереження посилання на першу публікацію статті в цьому журналі.