RETRACTED ARTICLE: Exploring ML-based classification system for digital learning platforms: a mapping technique of massive open online courses
Keywords:
digital learning systems, massive open online courses (MOOCs), Bloom’s taxonomy, machine learning, training softwareAbstract
The object of research is massive open online courses. One of the most problematic areas in online learning is how to improve the quality assurance of digital learning systems. Analysis and classification of massive open online courses is a difficult task, given the variability of massive open online courses structures, contents, designs, platforms, providers, and learner profiles. To overcome this challenge, this study aims to propose an automatic and large-scale machine learning based classification system for massive open online courses according to their learning objectives by making use of the six cognitive levels of Bloom’s taxonomy. During the course of the research, it is shown that analyzing learning objectives associated with modules and programs can further enhance the quality of digital learning system. As a result of the research, a representation and a detailed analysis of the dataset for experimentation with the different models are provided. Further research can focus on the privacy implications of the current control on developments of artificial intelligence taking into account creativity, and innovation which can hardly be performed by machines.
References
- Sebbaq, H., Faddouli, N. E. E. (2021). MOOCs semantic interoperability: Towards unified and pedagogically enriched model for building a linked data repository. International Conference on Digital Technologies and Applications. Springer, 621–631. doi: https://doi.org/10.1007/978-3-030-73882-2_56
- Devlin, J., Chang, M. W., Lee, K., Toutanova, K. (2018). Bert: Pre-training of deep bidirectional transformers for language understanding. arXiv preprint arXiv:1810.04805. doi: https://doi.org/10.48550/arXiv.1810.04805
- Abduljabbar, D. A., Omar, N. (2015). Exam questions classification based on Bloom’s taxonomy cognitive level using classifiers combination. Journal of Theoretical and Applied information Technology, 78 (3), 447–455. Available at: http://www.jatit.org/volumes/Vol78No3/15Vol78No3.pdf
- Davis, D., Seaton, D., Hauff, C., Houben, G. J. (2018). Toward large-scale learning design: Categorizing course designs in service of supporting learning outcomes. Proceedings of the Fifth Annual ACM Conference on Learning at Scale, 1–10. doi: https://doi.org/10.1145/3231644.3231663
- Kopp, M., Lackner, E. (2014). Do MOOCs need a special instructional design? Proceedings of Sixth international Conference on Education and New Learning. EDULEARN14. Barcelona, 7138–7147. Available at: https://library.iated.org/view/KOPP2014DOM
- Molenda, M. (2003). In search of the elusive ADDIE model. Performance Improvement, 42 (5), 34–36. doi: https://doi.org/10.1002/pfi.4930420508
- Conole, G. (2014). The 7Cs of learning design: A new approach to rethinking design practice. Proceedings of the Ninth international Conference on Networked Learning. Edinburgh, 502–509. Available at: https://www.lancaster.ac.uk/fss/organisations/netlc/past/nlc2014/abstracts/pdf/conole.pdf
- Osman, A., Yahya, A. A. (2016). Classifications of exam questions using linguistically-motivated features: A case study based on Bloom’s taxonomy. Available at: https://www.researchgate.net/publication/298286164_Classifications_of_Exam_Questions_Using_Linguistically-Motivated_Features_A_Case_Study_Based_on_Blooms_Taxonomy
- Kingma, D. P., Ba, J. (2014). Adam: A method for stochastic optimization. arXiv preprint arXiv:1412.6980. doi: https://doi.org/10.48550/arXiv.1412.6980
- Major, C. H., Blackmon, S. J. (2016). Massive Open Online Courses: Variations on a New Instructional Form. New Directions for Institutional Research, 2015 (167), 11–25. doi: https://doi.org/10.1002/ir.20151
- Das, S., Das Mandal, S. K., Basu, A. (2020). Identification of Cognitive Learning Complexity of Assessment Questions Using Multi-class Text Classification. Contemporary Educational Technology, 12 (2), ep275. doi: https://doi.org/10.30935/cedtech/8341
- Haris, S. S., Omar, N. (2012). A rule-based approach in Bloom’s taxonomy question classification through natural language processing. Seventh international Conference on Computing and Convergence Technology. CCCT, 410–414. Available at: https://ieeexplore.ieee.org/abstract/document/6530368
- Conole, G. (2016). MOOCs as disruptive technologies: strategies for enhancing the learner experience and quality of MOOCs. Revista de Educación a Distancia (RED), 50. doi: https://doi.org/10.6018/red/50/2
- González-Carvajal, S., Garrido-Merchán, E. C. (2020). Comparing BERT against traditional machine learning text classification. arXiv preprint arXiv:2005.13012. doi: https://doi.org/10.48550/arXiv.2005.13012
- Merrill, M. D. (2012). First principles of instruction. John Wiley & Sons. Available at: https://digitalcommons.usu.edu/usufaculty_monographs/100/
- Margaryan, A., Bianco, M., Littlejohn, A. (2015). Instructional quality of Massive Open Online Courses (MOOCs). Computers & Education, 80, 77–83. doi: https://doi.org/10.1016/j.compedu.2014.08.005
- Grandini, M., Bagli, E., Visani, G. (2020). Metrics for multi-class classification: An overview. arXiv preprint arXiv:2008.05756. doi: https://doi.org/10.48550/arXiv.2008.05756
- Pardos, Z. A., Schneider, E. (2013). AiED 2013 workshops proceedings. Vol. 1. Available at: http://people.cs.pitt.edu/~falakmasir/docs/AiED2013.pdf
- Quintana, R. M., Tan, Y. (2019). Characterizing MOOC Pedagogies: Exploring Tools and Methods for Learning Designers and Researchers. Online Learning, 23 (4), 62–84. doi: https://doi.org/10.24059/olj.v23i4.2084
- Vaswani, A., Shazeer, N., Parmar, N., Uszkoreit, J., Jones, L., Gomez, A. N. et al. (2017). Attention is all you need. 31st Conference on Neural information Processing Systems. NiPS 2017. Long Beach, 5998–6008. doi: https://doi.org/10.48550/arXiv.1706.03762
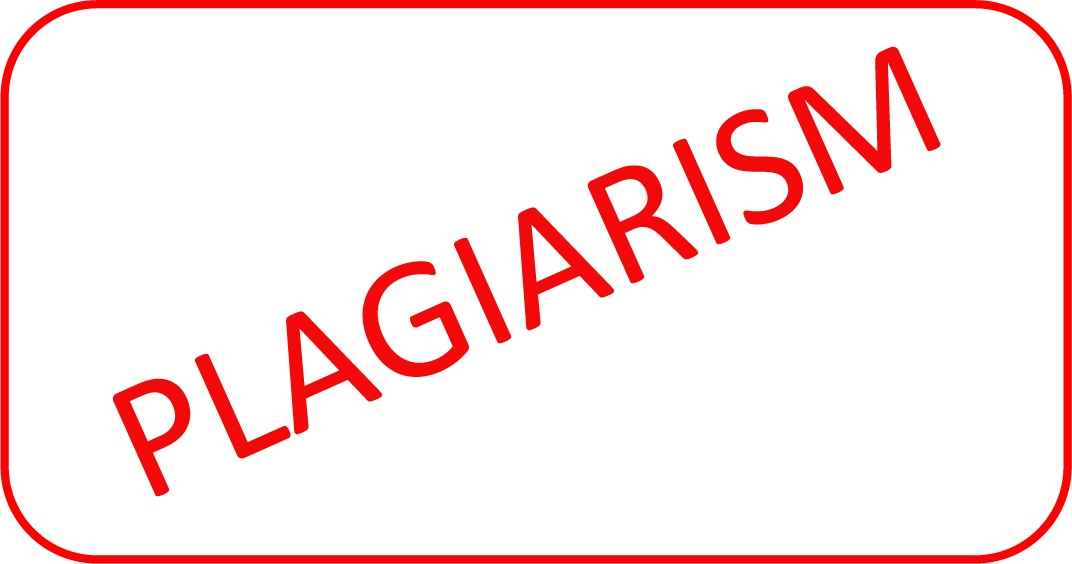
Downloads
Published
How to Cite
Issue
Section
License
Copyright (c) 2022 Ayse Kok Arslan

This work is licensed under a Creative Commons Attribution 4.0 International License.
The consolidation and conditions for the transfer of copyright (identification of authorship) is carried out in the License Agreement. In particular, the authors reserve the right to the authorship of their manuscript and transfer the first publication of this work to the journal under the terms of the Creative Commons CC BY license. At the same time, they have the right to conclude on their own additional agreements concerning the non-exclusive distribution of the work in the form in which it was published by this journal, but provided that the link to the first publication of the article in this journal is preserved.