Exploring an LSTM-SARIMA routine for core inflation forecasting
DOI:
https://doi.org/10.15587/2706-5448.2024.301209Keywords:
dynamic time warping, clustering, K-Means, recurrent neural network, machine learning, core inflationAbstract
The object of the research is the Core Inflation Forecasting. The paper investigates the performance of the novel model routine in the exercise of the Core Inflation Forecasting. It aggregates 300+ components into 6 by the similarity of their dynamics using an updated DTW algorithm fine-tuned for monthly time series and the K-Means algorithm for grouping. Then the SARIMA model extracts linear and seasonal components, which is followed by an LSTM model that captures non-linearities and interdependencies. It solves the problem of high-quality inflation forecasting using a disaggregated dataset. While standard and traditional econometric techniques are focused on the limited sets of data that consists just a couple of variables, proposed methodology is able to capture richer part of the volatility comprising more information. The model is compared with a huge pool of other models, simple ones like Random Walk and SARIMA, to ML models like XGBoost, Random Forest and simple LSTM. While all Data Science model shows decent performance, the DTW+K-Means+SARIMA+LSTM routine gives the best RMSE over 1-month ahead and 2-month ahead forecasts, which proves the high quality of the proposed forecasting model and solves the key problem of the paper. It is explained by the model's capability to capture both linear/seasonal patterns from the data using SARIMA part as long as it non-linear and interdependent using LSTM approach. Models are fitted for the case of Ukraine as long as they’ve been estimated on the corresponding data and may be actively used for further inflation forecasting.
References
- Krukovets, D. (2023). Updated DTW+K-Means approach with LSTM and ARIMA-type models for Core Inflation forecasting. Bulletin of Taras Shevchenko National University of Kyiv. Series: Physics and Mathematics, 2, 214–225. doi: https://doi.org/10.17721/1812-5409.2023/2.38
- Huwiler, M., Kaufmann, D. (2013). Combining disaggregate forecasts for inflation: The SNB’s ARIMA model. Swiss National Bank Economic Studies, 7.
- Mondal, P., Shit, L., Goswami, S. (2014). Study of Effectiveness of Time Series Modeling (Arima) in Forecasting Stock Prices. International Journal of Computer Science, Engineering and Applications, 4 (2), 13–29. doi: https://doi.org/10.5121/ijcsea.2014.4202
- Anggraeni, W., Andri, K. B., Sumaryanto, Mahananto, F. (2017). The Performance of ARIMAX Model and Vector Autoregressive (VAR) Model in Forecasting Strategic Commodity Price in Indonesia. Procedia Computer Science, 124, 189–196. doi: https://doi.org/10.1016/j.procs.2017.12.146
- Medeiros, M. C., Vasconcelos, G. F. R., Veiga, Á., Zilberman, E. (2019). Forecasting Inflation in a Data-Rich Environment: The Benefits of Machine Learning Methods. Journal of Business & Economic Statistics, 39 (1), 98–119. doi: https://doi.org/10.1080/07350015.2019.1637745
- Profatska, N. (2021). Standard quality report state statistical observation «changes in prices (tariffs) for consumer goods (services)» 2.06.01.01. State Statistics Service of Ukraine, 1–11.
- Krukovets, D., Verchenko, O. (2019). Short-Run Forecasting of Core Inflation in Ukraine: a Combined ARMA Approach. Visnyk of the National Bank of Ukraine, 248, 11–20. doi: https://doi.org/10.26531/vnbu2019.248.02
- Shapovalenko, N. (2021). A Suite of Models for CPI Forecasting. Visnyk of the National Bank of Ukraine, 252, 4–36. doi: https://doi.org/10.26531/vnbu2021.252.01
- Almosova, A., Andresen, N. (2019). Nonlinear Inflation Forecasting with Recurrent Neural Networks. European Central Bank (ECB), 1–45.
- Longo, L., Riccaboni, M., Rungi, A. (2022). A neural network ensemble approach for GDP forecasting. Journal of Economic Dynamics and Control, 134, 104278. doi: https://doi.org/10.1016/j.jedc.2021.104278
- Siami-Namini, S., Tavakoli, N., Siami Namin, A. (2018). A Comparison of ARIMA and LSTM in Forecasting Time Series. 2018 17th IEEE International Conference on Machine Learning and Applications (ICMLA). doi: https://doi.org/10.1109/icmla.2018.00227
- Hyndman, R. J., Khandakar, Y. (2008). Automatic Time Series Forecasting: The Forecast Package for R. Journal of Statistical Software, 27 (3). doi: https://doi.org/10.18637/jss.v027.i03
- Fan, G.-F., Zhang, L.-Z., Yu, M., Hong, W.-C., Dong, S.-Q. (2022). Applications of random forest in multivariable response surface for short-term load forecasting. International Journal of Electrical Power & Energy Systems, 139, 108073. doi: https://doi.org/10.1016/j.ijepes.2022.108073
- Kumar, M., Thenmozhi, M. (2014). Forecasting stock index returns using ARIMA-SVM, ARIMA-ANN, and ARIMA-random forest hybrid models. International Journal of Banking, Accounting and Finance, 5 (3), 284. doi: https://doi.org/10.1504/ijbaaf.2014.064307
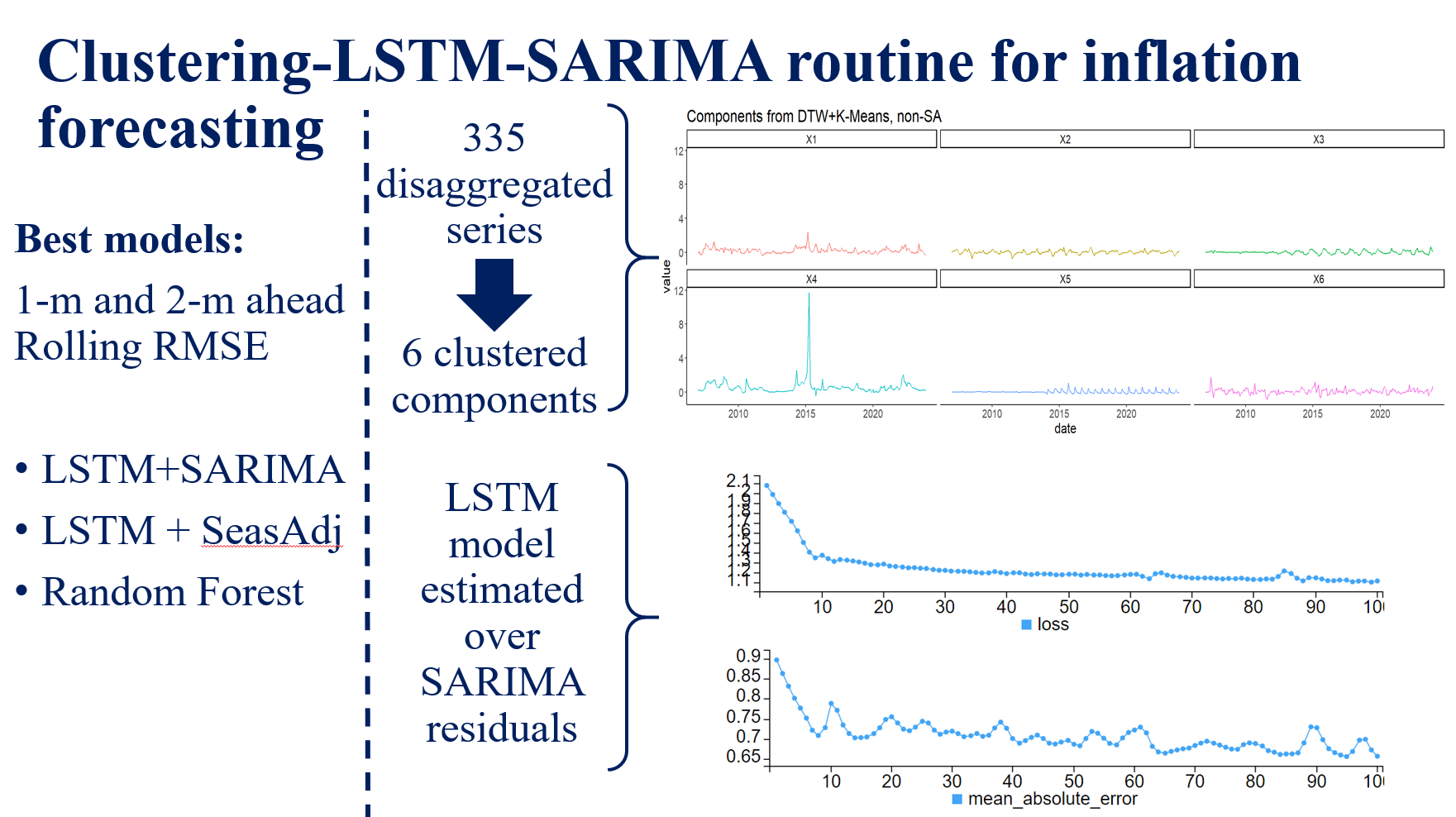
Downloads
Published
How to Cite
Issue
Section
License
Copyright (c) 2024 Dmytro Krukovets

This work is licensed under a Creative Commons Attribution 4.0 International License.
The consolidation and conditions for the transfer of copyright (identification of authorship) is carried out in the License Agreement. In particular, the authors reserve the right to the authorship of their manuscript and transfer the first publication of this work to the journal under the terms of the Creative Commons CC BY license. At the same time, they have the right to conclude on their own additional agreements concerning the non-exclusive distribution of the work in the form in which it was published by this journal, but provided that the link to the first publication of the article in this journal is preserved.