Synergistic prediction of penetration rate in Boukhadhra mining using regression, design of experiments, fuzzy logic, and artificial neural networks
DOI:
https://doi.org/10.15587/2706-5448.2024.309965Keywords:
drilling, mining, rate of penetration, design of experiments, fuzzy logic, artificial neural networkAbstract
The comparative analysis of predictive methodologies highlights the original contribution of this study in optimizing the prediction of Rate of Penetration (ROP) in mining drilling operations. The emphasis on employing advanced Artificial Neural Networks (ANN), fuzzy logic, and linear regression models provides new insights into enhancing predictive accuracy and operational efficiency in mining practices. This study aims to quantify the effects of three pivotal drilling parameters: compressive strength, rotational pressure, and thrust pressure on the rate of penetration, a critical performance metric in mining drilling operations. Additionally, the study seeks to develop and evaluate advanced predictive methodologies for predicting ROP. The effects of compressive strength, rotational pressure, and thrust pressure on the rate of penetration were investigated through a Design of Experiments (DOE) approach. Initially, the main effects and two-way interactions among these variables were identified using DOE. Subsequently, three predictive methodologies: linear regression, fuzzy logic, and artificial neural networks, were developed and evaluated to predict ROP based on the identified factors. The evaluation of predictive methodologies revealed that the ANN model demonstrated superior accuracy in predicting the ROP, achieving over 95 % accuracy. Additionally, the fuzzy logic model provided effective handling of nonlinearities in the data, while the linear regression model offered initial insights into the relationships between the variables. The application of advanced predictive methodologies: artificial neural networks, fuzzy logic, and linear regression to optimize the prediction of rate of penetration in mining drilling operations offers precise insights into drilling parameter interactions, enhancing operational efficiency and supporting informed decision making in mining practices.
References
- Anemangely, M., Ramezanzadeh, A., Tokhmechi, B., Molaghab, A., Mohammadian, A. (2018). Drilling rate prediction from petrophysical logs and mud logging data using an optimized multilayer perceptron neural network. Journal of Geophysics and Engineering, 15 (4), 1146–1159. https://doi.org/10.1088/1742-2140/aaac5d
- Hegde, C., Millwater, H., Pyrcz, M., Daigle, H., Gray, K. (2019). Rate of penetration (ROP) optimization in drilling with vibration control. Journal of Natural Gas Science and Engineering, 67, 71–81. https://doi.org/10.1016/j.jngse.2019.04.017
- Shi, X., Liu, G., Gong, X., Zhang, J., Wang, J., Zhang, H. (2016). An Efficient Approach for Real-Time Prediction of Rate of Penetration in Offshore Drilling. Mathematical Problems in Engineering, 2016, 1–13. https://doi.org/10.1155/2016/3575380
- Nascimento, A., Tamas Kutas, D., Elmgerbi, A., Thonhauser, G., Hugo Mathias, M. (2015). Mathematical Modeling Applied to Drilling Engineering: An Application of Bourgoyne and Young ROP Model to a Presalt Case Study. Mathematical Problems in Engineering, 2015, 1–9. https://doi.org/10.1155/2015/631290
- Najjarpour, M., Jalalifar, H., Norouzi-Apourvari, S. (2021). A review on half a century of experience in rate of penetration management: Application of analytical, semi-analytical and empirical models. Advances in Geo-Energy Research, 5 (3), 252–273. https://doi.org/10.46690/ager.2021.03.03
- Seifabad, M. C., Ehteshami, P. (2013). Estimating the drilling rate in Ahvaz oil field. Journal of Petroleum Exploration and Production Technology, 3 (3), 169–173. https://doi.org/10.1007/s13202-013-0060-3
- Bezminabadi, S. N., Ramezanzadeh, A., Esmaeil Jalali, S.-M., Tokhmechi, B., Roustaei, A. (2017). Effect of Rock Properties on ROP Modeling Using Statistical and Intelligent Methods: A Case Study of an Oil Well in Southwest of Iran. Archives of Mining Sciences, 62 (1), 131–144. https://doi.org/10.1515/amsc-2017-0010
- Alsubaih, A., Albadran, F., Alkanaani, N. (2018). Mechanical specific energy and statistical techniques to maximizing the drilling rates for production section of Mishrif wells in Southern Iraq fields. SPE/IADC Middle East Drilling Technology Conference and Exhibition. Abu Dhabi: Society of Petroleum Engineers. https://doi.org/10.2118/189354-ms
- Rais, K., Kara, M., Hadji, R. H., Gadri, L., Khochmen, L. (2017). Original approach for the drilling process op-timization in open cast mines; Case study of kef essenoun open pit mine northeast of algeria. Mining Science, 24, 147–159.
- Kricak, L., Negovanovic, M., Mitrovic, S., Miljanovic, I., Nuric, S., Nuric, A. (2015). Development of a fuzzy model for predicting the penetration rate of tricone rotary blasthole drilling in open pit mines. Journal of the Southern African Institute of Mining and Metallurgy, 115 (11), 1065–1071. https://doi.org/10.17159/2411-9717/2015/v115n11a11
- Alarifi, S., AlNuaim, S., Abdulraheem, A. (2015). Productivity Index Prediction for Oil Horizontal Wells Using Different Artificial Intelligence Techniques. All Days. https://doi.org/10.2118/172729-ms
- Bai, Y., Zhuang, H., Wang, D. (2006). Fundamentals of fuzzy logic control – fuzzy sets, fuzzy rules and defuzzifications. Advanced Fuzzy Logic Technologies in Industrial Applications, XXV. Springer, 17–36. https://doi.org/10.1007/978-1-84628-469-4_2
- Ghasemi, E., Ataei, M., Hashemolhosseini, H. (2012). Development of a fuzzy model for predicting ground vibration caused by rock blasting in surface mining. Journal of Vibration and Control, 19 (5), 755–770. https://doi.org/10.1177/1077546312437002
- Tahmasebi, P., Hezarkhani, A. (2012). A hybrid neural networks-fuzzy logic-genetic algorithm for grade estimation. Computers & Geosciences, 42, 18–27. https://doi.org/10.1016/j.cageo.2012.02.004
- Anifowose, F., Abdulraheem, A. (2011). Fuzzy logic-driven and SVM-driven hybrid computational intelligence models applied to oil and gas reservoir characterization. Journal of Natural Gas Science and Engineering, 3 (3), 505–517. https://doi.org/10.1016/j.jngse.2011.05.002
- Hemphill, T., Bern, P. A., Rojas, J., Ravi, K. (2007). Field Validation of Drillpipe Rotation Effects on Equivalent Circulating Density. Proceedings of the SPE Annual Technical Conference and Exhibition, Society of Petroleum Engineers (SPE). https://doi.org/10.2118/110470-ms
- Hinton, G. E., Osindero, S., Teh, Y.-W. (2006). A Fast Learning Algorithm for Deep Belief Nets. Neural Computation, 18 (7), 1527–1554. https://doi.org/10.1162/neco.2006.18.7.1527
- Liew, S. S., Khalil-Hani, M., Bakhteri, R. (2016). An optimized second order stochastic learning algorithm for neural network training. Neurocomputing, 186, 74–89. https://doi.org/10.1016/j.neucom.2015.12.076
- Arabjamaloei, R., Shadizadeh, S. (2011). Modeling and Optimizing Rate of Penetration Using Intelligent Systems in an Iranian Southern Oil Field (Ahwaz Oil Field). Petroleum Science and Technology, 29 (16), 1637–1648. https://doi.org/10.1080/10916460902882818
- Shi, X., Liu, G., Gong, X., Zhang, J., Wang, J., Zhang, H. (2016). An Efficient Approach for Real-Time Prediction of Rate of Penetration in Offshore Drilling. Mathematical Problems in Engineering, 2016, 1–13. https://doi.org/10.1155/2016/3575380
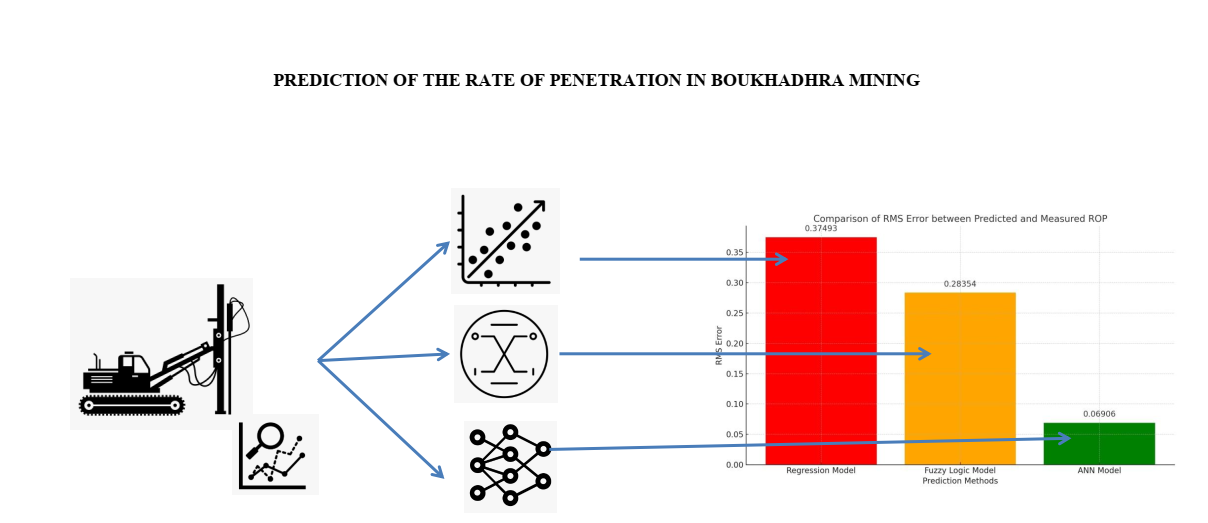
Downloads
Published
How to Cite
Issue
Section
License
Copyright (c) 2024 Mohamed Mebarkia, Asma Abdelmalek, Zoubir Aoulmi, Messaoud Louafi, Abdelhak Tabet, Aissa Benselhoub

This work is licensed under a Creative Commons Attribution 4.0 International License.
The consolidation and conditions for the transfer of copyright (identification of authorship) is carried out in the License Agreement. In particular, the authors reserve the right to the authorship of their manuscript and transfer the first publication of this work to the journal under the terms of the Creative Commons CC BY license. At the same time, they have the right to conclude on their own additional agreements concerning the non-exclusive distribution of the work in the form in which it was published by this journal, but provided that the link to the first publication of the article in this journal is preserved.