Розробка методу прогнозування небезпечних траєкторій руху суден в умовах невизначеності дій судноводія
DOI:
https://doi.org/10.15587/2706-5448.2024.313523Ключові слова:
маневрування у стиснених водах, аварійні ситуації, надійність роботи, оптимізація процесів керування, кермове управління, модуль автоматичного керуванняАнотація
Об’єктом дослідження є процеси автоматизації в морській навігації для забезпечення безпеки руху суден шляхом прогнозування їх траєкторій в умовах складної акваторії, таких як вузкості, протоки та порти. У дослідженні було застосовано шість ключових етапів для створення комплексного методу кластеризації та прогнозування траєкторій суден на основі даних ECDIS.
На першому етапі було побудовано траєкторії руху суден відповідно до категорій ризику, використовуючи алгоритми LCSS та DTW для порівняння запланованих і фактичних траєкторій. Це дозволило точно ідентифікувати відхилення від курсу та визначити потенційно небезпечні ділянки траєкторії. Другий етап реалізував кластеризацію за допомогою алгоритмів DBSCAN та GMM. DBSCAN використовувався для ідентифікації щільності точок у просторі, а GMM забезпечував моделювання ймовірностей кластерів, що дозволило краще визначити зони ризику. Третій етап застосував алгоритм стискання Douglas-Peucker для зменшення кількості точок у траєкторіях, що дозволило зберегти ключові характеристики та оптимізувати обробку даних. На четвертому етапі було проведено оцінку стабільності руху суден за допомогою перетворення Фур’є, що дозволило виявити високочастотні коливання, які можуть свідчити про нестабільність руху, спричинену змінами курсу або швидкості. П’ятий етап включав нечітку кластеризацію траєкторій за допомогою моделі Гауссових змішувань (GMM), що дозволило моделювати ймовірності небезпечних траєкторій з урахуванням невизначеності навігаційних параметрів. На завершальному етапі було використано багатошарову нейронну мережу (MLP) для прогнозування майбутніх точок траєкторій суден. Модель показала високу точність у прогнозуванні координат судна, що дало змогу своєчасно коригувати траєкторії.
Експериментальні результати показали, що розроблений метод підвищив точність прогнозування траєкторій суден до 72–81 %, а також значно зменшив кінцеву похибку, що забезпечує ефективне управління ризиками під час складної навігації.
Посилання
- Nosov, P., Koretsky, O., Zinchenko, S., Prokopchuk, Y., Gritsuk, I., Sokol, I., Kyrychenko, K. (2023). Devising an approach to safety management of vessel control through the identification of navigator’s state. Eastern-European Journal of Enterprise Technologies, 4 (3 (124)), 19–32. https://doi.org/10.15587/1729-4061.2023.286156
- Ponomaryova, V., Nosov, P., Ben, A., Popovych, I., Prokopchuk, Y., Mamenko, P. et al. (2024). Devising an approach for the automated restoration of shipmaster’s navigational qualification parameters under risk conditions. Eastern-European Journal of Enterprise Technologies, 1 (3 (127)), 6–26. https://doi.org/10.15587/1729-4061.2024.296955
- Hrytsuk, I., Nosov, P., Ponomarova, V., Diahyleva, O. (2023). Reduction of navigation risks by using fuzzy logic to automate control processes under uncertainty. Nauka i Tekhnika Sohodni, 6 (20), 8–22. https://doi.org/10.52058/2786-6025-2023-6(20)-8-22
- Liang, M., Liu, W., Gao, R., Xiao, Z., Zhang, X., Wang, H. (2024). A Survey of Distance-Based Vessel Trajectory Clustering: Data Pre-processing, Methodologies, Applications, and Experimental Evaluation. https://doi.org/10.48550/arXiv.2407.11084
- Ma, L., Shi, G., Li, W., Jiang, D. (2023). A Direction-Preserved Vessel Trajectory Compression Algorithm Based on Open Window. Journal of Marine Science and Engineering, 11 (12), 2362. https://doi.org/10.3390/jmse11122362
- Alam, M. M., Spadon, G., Etemad, M., Torgo, L., Milios, E. (2024). Enhancing short-term vessel trajectory prediction with clustering for heterogeneous and multi-modal movement patterns. Ocean Engineering, 308, 118303. https://doi.org/10.1016/j.oceaneng.2024.118303
- Zhang, R., Chen, X., Ye, L., Yu, W., Zhang, B., Liu, J. (2024). Predicting Vessel Trajectories Using ASTGCN with StemGNN-Derived Correlation Matrix. Applied Sciences, 14 (10), 4104. https://doi.org/10.3390/app14104104
- Yang, X., Han, Z., Zhang, Y., Liu, H., Liu, S., Ai, W., Liu, J. (2024). VEPO-S2S: A VEssel Portrait Oriented Trajectory Prediction Model Based on S2S Framework. Applied Sciences, 14 (14), 6344. https://doi.org/10.3390/app14146344
- Luo, S., Zeng, W., Sun, B. (2023). Contrastive Learning for Graph-Based Vessel Trajectory Similarity Computation. Journal of Marine Science and Engineering, 11 (9), 1840. https://doi.org/10.3390/jmse11091840
- Zhu, Q., Xi, Y., Hu, S., Chen, Y. (2023). Exploring the behavior feature of complex trajectories of ships with Fourier transform processing: a case from fishing vessels. Frontiers in Marine Science, 10. https://doi.org/10.3389/fmars.2023.1271930
- Li, Y., Yu, Q., Yang, Z. (2024). Vessel Trajectory Prediction for Enhanced Maritime Navigation Safety: A Novel Hybrid Methodology. Journal of Marine Science and Engineering, 12 (8), 1351. https://doi.org/10.3390/jmse12081351
- Zhang, X., Zhou, S. (2023). Vessel Trajectory Data Compression Algorithm considering Critical Region Identification. Journal of Advanced Transportation, 2023, 1–18. https://doi.org/10.1155/2023/8831371
- Donandt, K., Söffker, D. (2024). Incorporating Navigation Context into Inland Vessel Trajectory Prediction: A Gaussian Mixture Model and Transformer Approach. https://doi.org/10.48550/arXiv.2406.02344
- Yang, C.-H., Wu, C.-H., Shao, J.-C., Wang, Y.-C., Hsieh, C.-M. (2022). AIS-Based Intelligent Vessel Trajectory Prediction Using Bi-LSTM. IEEE Access, 10, 24302–24315. https://doi.org/10.1109/access.2022.3154812
- Aguilar-Ibanez, C., Suarez-Castanon, M. S., García-Canseco, E., Rubio, J. de J., Barron-Fernandez, R., Martinez, J. C. (2024). Trajectory Tracking Control of an Autonomous Vessel in the Presence of Unknown Dynamics and Disturbances. Mathematics, 12 (14), 2239. https://doi.org/10.3390/math12142239
- Li, J. H., Kang, H., Kim, M. G., Lee, M. J., Cho, G. R. (2022). Asymptotic Trajectory Tracking of Underactuated Non-minimum Phase Marine Vessels. IFAC-PapersOnLine, 55 (31), 281–286. https://doi.org/10.1016/j.ifacol.2022.10.443
- Oka Widyantara, I. M., Noven Hartawan, I. P., Ngurah Eka Karyawati, A. A. I., Er, N. I., Artana, K. B. (2023). Automatic identification system-based trajectory clustering framework to identify vessel movement pattern. IAES International Journal of Artificial Intelligence (IJ-AI), 12 (1), 1. https://doi.org/10.11591/ijai.v12.i1.pp1-11
- Xu, Y., Zhang, J., Ren, Y., Zeng, Y., Yuan, J., Liu, Z., Wang, L., Ou, D. (2022). Improved Vessel Trajectory Prediction Model Based on Stacked-BiGRUs. Security and Communication Networks, 2022, 1–17. https://doi.org/10.1155/2022/8696558
- Cahapin, E. L., Malabag, B. A., Santiago Jr., C. S., Reyes, J. L., Legaspi, G. S., Adrales, K. L. (2023). Clustering of students admission data using k-means, hierarchical, and DBSCAN algorithms. Bulletin of Electrical Engineering and Informatics, 12 (6), 3647–3656. https://doi.org/10.11591/eei.v12i6.4849
- Yang, L., Liu, J., Wang, Y. (2024). Application of adaptive douglas-peucker with acceleration algorithm in ship trajectory compression. 2024 IEEE Conference on Artificial Intelligence (CAI), 206–209. https://doi.org/10.1109/cai59869.2024.00045
- Sun, B., Wu, X., Chen, X., Zou, Z., Li, Q., Ren, B. (2024). Parameter Estimation of Sub-/Super-synchronous Oscillation Based on Interpolated All-phase Fast Fourier Transform with Optimized Window Function. Journal of Modern Power Systems and Clean Energy, 12 (4), 1031–1041. https://doi.org/10.35833/mpce.2023.000179
- Asri, S. D. (2024). Underwater Image Segmentation with the GMM (Gaussian Mixture Model) Algorithm. JSAI (Journal Scientific and Applied Informatics), 7 (2), 253–257. https://doi.org/10.36085/jsai.v7i2.6418
- Sambasiva Rao, R. (2014). Mathematical Neural Network (MaNN) Models Part VI: Single-layer perceptron [SLP] and Multi-layer perceptron [MLP] Neural networks in ChEM- Lab. Journal of Applicable Chemistry, 3, 2209–2311.
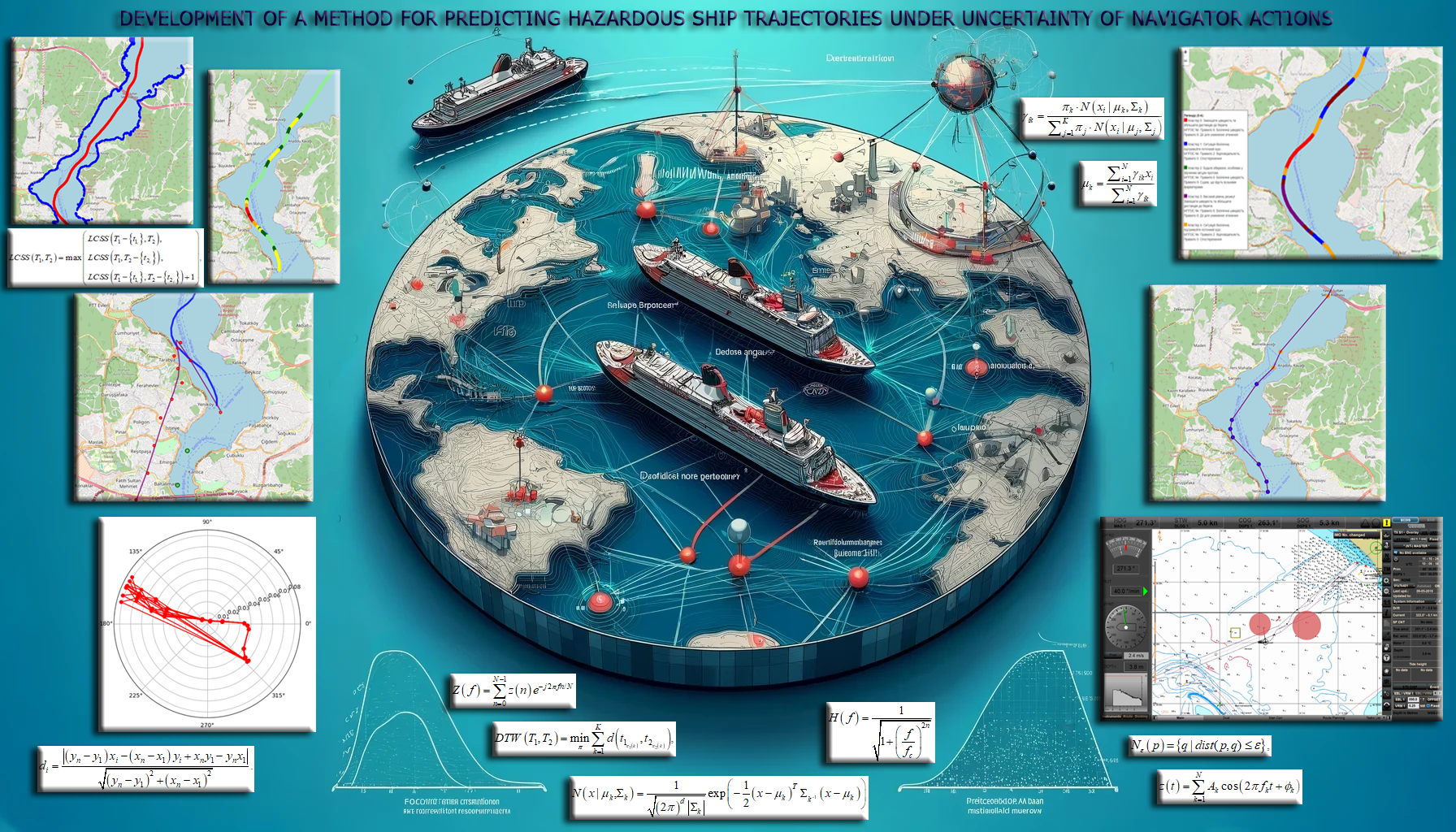
##submission.downloads##
Опубліковано
Як цитувати
Номер
Розділ
Ліцензія
Авторське право (c) 2024 Pavlo Nosov, Victoria Ponomaryova

Ця робота ліцензується відповідно до Creative Commons Attribution 4.0 International License.
Закріплення та умови передачі авторських прав (ідентифікація авторства) здійснюється у Ліцензійному договорі. Зокрема, автори залишають за собою право на авторство свого рукопису та передають журналу право першої публікації цієї роботи на умовах ліцензії Creative Commons CC BY. При цьому вони мають право укладати самостійно додаткові угоди, що стосуються неексклюзивного поширення роботи у тому вигляді, в якому вона була опублікована цим журналом, але за умови збереження посилання на першу публікацію статті в цьому журналі.