Recent advances in computational drug discovery for therapy against coronavirus SARS-CoV-2
DOI:
https://doi.org/10.15587/2519-4852.2023.290318Keywords:
human coronavirus, QSAR, molecular docking, virtual screening, machine learning, molecular dynamics, structure-based drug design, Mpro and PLpro proteases, CADD, SARS-CоV-2Abstract
Despite essential experimental efforts focused on studying severe acute respiratory syndrome coronavirus 2 (SARS-CoV-2), computational chemistry methods are promising complementary tools in combating coronavirus disease 2019 (COVID-19). The present review aims to provide readers with the recent progress and advances in computational approaches currently used to streamline drug discovery and development in the context of COVID-19. Our review is dual purpose. It is intended (a) to familiarize the readership with the general concept of QSAR, in silico screening, molecular docking and molecular dynamics (MD) simulations and (b) to provide key examples of the recent applications of these computational tools in discovering novel therapeutic agents against COVID-19. We outline how structure- and ligand-based drug design can accelerate the structural elucidation of pharmacological drug targeting and the discovery of preclinical drug candidate molecules. Several examples of MD computational studies demonstrate how atomistic MD simulations can facilitate our understanding of the molecular basis of drug actions and biological mechanisms of virus inhibition in atomic detail. Finally, the short- and long-term perspectives in computational drug discovery are discussed.
The aim of this study is to summarize the last three years' progress and advances in computational approaches currently used to streamline the drug discovery and development process in the context of COVID-19.
Materials and methods. The literature overview of QSAR, in silico screening, machine learning, molecular docking and molecular dynamics (MD) simulations is given in the context of COVID-19. The literature search was performed using online databases, such as Scopus, Web of Science, PDB-protein databank, and PubMed, focusing on the following keywords - human coronavirus, QSAR, molecular docking, virtual screening, machine learning, molecular dynamics, Mpro and PLpro proteases, SARS-CоV-2, respectively.
Results. The review familiarizes the readership with the general concept of QSAR, in silico screening, machine learning, molecular docking and MD simulations and provides key examples of the recent applications of these computational tools in discovering novel therapeutic agents against COVID-19.
Conclusions. New insight into the recent progress and achievements in computer-guided drug discovery for therapeutic agents against SARS-CoV-2 is provided
Supporting Agency
- Grant No. 42/0062 (2021.01/0062) “Molecular design, synthesis and screening of new potential antiviral pharmaceutical ingredients for the treatment of infectious diseases COVID-19” from the National Research Foundation of Ukraine.
References
- Vincenzi, M., Mercurio, F. A., Leone, M. (2023). Looking for SARS-CoV-2 Therapeutics Through Computational Approaches. Current Medicinal Chemistry, 30 (28), 3158–3214. doi: https://doi.org/10.2174/0929867329666221004104430
- Muratov, E. N., Amaro, R., Andrade, C. H., Brown, N., Ekins, S., Fourches, D. et al. (2021). A critical overview of computational approaches employed for COVID-19 drug discovery. Chemical Society Reviews, 50 (16), 9121–9151. doi: https://doi.org/10.1039/d0cs01065k
- Middha, S. K., Usha, T., Sukhralia, S., Pareek, C., Yadav, R., Agnihotri, R. et al.; Parihar, A., Khan, R., Kumar, A., Kaushik, A. K., Gohel, H. (Eds.) (2022). Prediction of drug-target interaction – a helping hand in drug repurposing. Computational Approaches for Novel Therapeutic and Diagnostic Designing to Mitigate SARS-CoV-2 Infection. Academic Press, 519–536. doi: https://doi.org/10.1016/b978-0-323-91172-6.00006-6
- Nandi, S., Nayak, B. S., Khede, M. K., Saxena, A. K. (2022). Repurposing of Chemotherapeutics to Combat COVID-19. Current Topics in Medicinal Chemistry, 22 (32), 2660–2694. doi: https://doi.org/10.2174/1568026623666221130142517
- Yadav, M., Dhagat, S., Eswari, J. S. (2020). Emerging strategies on in silico drug development against COVID-19: challenges and opportunities. European Journal of Pharmaceutical Sciences, 155, 105522. doi: https://doi.org/10.1016/j.ejps.2020.105522
- Gao, K., Wang, R., Chen, J., Cheng, L., Frishcosy, J., Huzumi, Y. et al. (2022). Methodology-Centered Review of Molecular Modeling, Simulation, and Prediction of SARS-CoV-2. Chemical Reviews, 122 (13), 11287–11368. doi: https://doi.org/10.1021/acs.chemrev.1c00965
- Kumar, S., Kovalenko, S., Bhardwaj, S., Sethi, A., Gorobets, N. Yu., Desenko, S. M. et al. (2022). Drug repurposing against SARS-CoV-2 using computational approaches. Drug Discovery Today, 27 (7), 2015–2027. doi: https://doi.org/10.1016/j.drudis.2022.02.004
- Sadybekov, A. V., Katritch, V. (2023). Computational approaches streamlining drug discovery. Nature, 616 (7958), 673–685. doi: https://doi.org/10.1038/s41586-023-05905-z
- Shah, A., Jain, M.; Rudrapal, M., Egbuna, C. (Eds.) (2022). Limitations and future challenges of computer-aided drug design methods. Computer Aided Drug Design (CADD): From Ligand-Based Methods to Structure-Based Approaches. Elsevier, 283–297. doi: https://doi.org/10.1016/b978-0-323-90608-1.00006-x
- Chen, L., Fan, Z., Chang, J., Yang, R., Hou, H., Guo, H. et al. (2023). Sequence-based drug design as a concept in computational drug design. Nature Communications, 14 (1). doi: https://doi.org/10.1038/s41467-023-39856-w
- Bon, M., Bilsland, A., Bower, J., McAulay, K. (2022). Fragment‐based drug discovery –the importance of high‐quality molecule libraries. Molecular Oncology, 16 (21), 3761–3777. doi: https://doi.org/10.1002/1878-0261.13277
- Green, H., Koes, D. R., Durrant, J. D. (2021). DeepFrag: a deep convolutional neural network for fragment-based lead optimization. Chemical Science, 12 (23), 8036–8047. doi: https://doi.org/10.1039/d1sc00163a
- Shi, X.-X., Wang, Z.-Z., Wang, F., Hao, G.-F., Yang, G.-F. (2023). ACFIS 2.0: an improved web-server for fragment-based drug discovery via a dynamic screening strategy. Nucleic Acids Research, 51 (W1), W25–W32. doi: https://doi.org/10.1093/nar/gkad348
- Gurung, A. B., Ali, M. A., Lee, J., Farah, M. A., Al-Anazi, K. M. (2021). An Updated Review of Computer-Aided Drug Design and Its Application to COVID-19. BioMed Research International, 2021, 1–18. doi: https://doi.org/10.1155/2021/8853056
- Bajad, N. G., Rayala, S., Gutti, G., Sharma, A., Singh, M., Kumar, A., Singh, S. K. (2021). Systematic review on role of structure based drug design (SBDD) in the identification of anti-viral leads against SARS-Cov-2. Current Research in Pharmacology and Drug Discovery, 2, 100026. doi: https://doi.org/10.1016/j.crphar.2021.100026
- Zhang, S., Krumberger, M., Morris, M. A., Parrocha, C. M. T., Kreutzer, A. G., Nowick, J. S. (2021). Structure-based drug design of an inhibitor of the SARS-CoV-2 (COVID-19) main protease using free software: A tutorial for students and scientists. European Journal of Medicinal Chemistry, 218, 113390. doi: https://doi.org/10.1016/j.ejmech.2021.113390
- Varela‐Rial, A., Majewski, M., De Fabritiis, G. (2021). Structure based virtual screening: Fast and slow. WIREs Computational Molecular Science, 12 (2). doi: https://doi.org/10.1002/wcms.1544
- Chen, W., Liu, X., Zhang, S., Chen, S. (2023). Artificial intelligence for drug discovery: Resources, methods, and applications. Molecular Therapy – Nucleic Acids, 31, 691–702. doi: https://doi.org/10.1016/j.omtn.2023.02.019
- Nasser, M., Yusof, U. K., Salim, N. (2023). Deep Learning Based Methods for Molecular Similarity Searching: A Systematic Review. Processes, 11 (5). doi: https://doi.org/10.3390/pr11051340
- Amaro, R. E., Mulholland, A. J. (2018). Multiscale methods in drug design bridge chemical and biological complexity in the search for cures. Nature Reviews Chemistry, 2 (4). doi: https://doi.org/10.1038/s41570-018-0148
- Amin, Sk. A., Banerjee, S., Singh, S., Qureshi, I. A., Gayen, S., Jha, T. (2021). First structure–activity relationship analysis of SARS-CoV-2 virus main protease (Mpro) inhibitors: an endeavor on COVID-19 drug discovery. Molecular Diversity, 25 (3), 1827–1838. doi: https://doi.org/10.1007/s11030-020-10166-3
- Luo, D., Tong, J.-B., Zhang, X., Xiao, X.-C., Bian, S. (2022). Computational strategies towards developing novel SARS-CoV-2 Mpro inhibitors against COVID-19. Journal of Molecular Structure, 1247, 131378. doi: https://doi.org/10.1016/j.molstruc.2021.131378
- Golbraikh, A., Shen, M., Xiao, Z., Xiao, Y.-D., Lee, K.-H., Tropsha, A. (2003). Rational selection of training and test sets for the development of validated QSAR models. Journal of Computer-Aided Molecular Design, 17 (2/4), 241–253. doi: https://doi.org/10.1023/a:1025386326946
- Golbraikh, A., Tropsha, A. (2000). Predictive QSAR modeling based on diversity sampling of experimental datasets for the training and test set selection. Molecular Diversity, 5 (4), 231–243. doi: https://doi.org/10.1023/a:102137210868
- Tetko, I. V., Gasteiger, J., Todeschini, R., Mauri, A., Livingstone, D., Ertl, P. et al. (2005). Virtual Computational Chemistry Laboratory – Design and Description. Journal of Computer-Aided Molecular Design, 19 (6), 453–463. doi: https://doi.org/10.1007/s10822-005-8694-y
- Yap, C. W. (2011). PaDEL-descriptor: An open source software to calculate molecular descriptors and fingerprints. Journal of Computational Chemistry, 32 (7), 1466–1474. doi: https://doi.org/10.1002/jcc.21707
- Todeschini, R., Consonni, V. (2000). Handbook of Molecular Descriptors. Weinheim: Wiley-VCH. doi: https://doi.org/10.1002/9783527613106
- Devillers, J., Balaban, A. T. (1999). Topological Indices and Related Descriptors in QSAR and QSPR. London: CRC Press, 811. doi: https://doi.org/10.1201/9781482296945
- Malinowski, E. R. (2002). Factor Analysis in Chemistry. Wiley-VCH, 432.
- Devillers, J. (1996). Genetic Algorithms in Molecular Modeling: Academic Press. doi: https://doi.org/10.1016/b978-0-12-213810-2.x5000-2
- Solnon, C., Jussien, N. (2013). Ant Colony Optimization and Constraint Programming. John Wiley & Sons. doi: https://doi.org/10.1002/9781118557563
- Breiman, L. (2001). Random Forests. Machine Learning, 45, 5–32. doi: https://doi.org/10.1023/a:1010933404324
- Tibshirani, R. (1996). Regression Shrinkage and Selection Via the Lasso. Journal of the Royal Statistical Society: Series B (Methodological), 58 (1), 267–288. doi: https://doi.org/10.1111/j.2517-6161.1996.tb02080.x
- Hesterberg, T., Choi, N. H., Meier, L., Fraley, C. (2008). Least angle and ℓ1 penalized regression: A review. Statistics Surveys, 2, 61–93. doi: https://doi.org/10.1214/08-ss035
- Zhao, P., Yu, B. (2007). Stagewise Lasso. Journal of Machine Learning Research, 8, 2701–2726.
- Hastie, T., Tibshirani, R. (2004). Efficient quadratic regularization for expression arrays. Biostatistics, 5 (3), 329–340. doi: https://doi.org/10.1093/biostatistics/kxh010
- Roy, K., Kar, S., Das, R. N. (2015). A Primer on QSAR/QSPR Modeling: Fundamental Concepts. Cham: Springer International Publishing. doi: https://doi.org/10.1007/978-3-319-17281-1
- Khaldan, A., Bouamrane, S., En-Nahli, F., El-mernissi, R., El khatabi, K., Hmamouchi, R. et al. (2021). Prediction of potential inhibitors of SARS-CoV-2 using 3D-QSAR, molecular docking modeling and ADMET properties. Heliyon, 7 (3), e06603. doi: https://doi.org/10.1016/j.heliyon.2021.e06603
- Jayakumar, J., Ebanesar, A., Gautam, S. (2023). Predictive analysis, diagnosis of COVID-19 through computational screening and validation with spectro photometrical approach. Toxicology and Environmental Health Sciences, 15 (2), 109–117. doi: https://doi.org/10.1007/s13530-023-00172-x
- Beger, R. D., Young, J. F., Fang, H. (2004). Discriminant Function Analyses of Liver-Specific Carcinogens. Journal of Chemical Information and Computer Sciences, 44 (3), 1107–1110. doi: https://doi.org/10.1021/ci0342829
- Hosmer, D. W. J., Lemeshow, S. (2000). Applied Logistic Regression. John Wiley & Sons. doi: https://doi.org/10.1002/0471722146
- Xu, Y., Zomer, S., Brereton, R. G. (2006). Support Vector Machines: A Recent Method for Classification in Chemometrics. Critical Reviews in Analytical Chemistry, 36 (3-4), 177–188. doi: https://doi.org/10.1080/10408340600969486
- Herbrich, R. (2001). Learning Kernel Classifiers Theory and Algorithms. Cambridge: The MIT Press. doi: https://doi.org/10.7551/mitpress/4170.001.0001
- Müller, K.-R., Rätsch, G., Sonnenburg, S., Mika, S., Grimm, M., Heinrich, N. (2005). Classifying ‘Drug-likeness' with Kernel-Based Learning Methods. Journal of Chemical Information and Modeling, 45 (2), 249–253. doi: https://doi.org/10.1021/ci049737o
- Rokach, L., Maimon, O. (2014). Data Mining with Decision Trees. Vol. 81. World Scientific, 328. doi: https://doi.org/10.1142/9097
- Santana, M. V. S., Silva-Jr, F. P.; Parihar, A., Khan, R., Kumar, A., Kaushik, A. K., Gohel, H. (Eds.) (2022). Artificial intelligence methods to repurpose and discover new drugs to fight the Coronavirus disease-2019 pandemic. Computational Approaches for Novel Therapeutic and Diagnostic Designing to Mitigate SARS-CoV-2 Infection. Academic Press, 537–557. doi: https://doi.org/10.1016/b978-0-323-91172-6.00016-9
- Krishnamurthy, A., Goel, P.; Parihar, A., Khan, R., Kumar, A., Kaushik, A. K., Gohel, H. (Eds.) (2022). Artificial intelligence-based drug screening and drug repositioning tools and their application in the present scenario. Computational Approaches for Novel Therapeutic and Diagnostic Designing to Mitigate SARS-CoV-2 Infection. Academic Press, 379–398. doi: https://doi.org/10.1016/b978-0-323-91172-6.00010-8
- Murcia-Soler, M., Pérez-Giménez, F., García-March, F. J., Salabert-Salvador, M. T., Díaz-Villanueva, W., Castro-Bleda, M. J., Villanueva-Pareja, A. (2004). Artificial Neural Networks and Linear Discriminant Analysis: A Valuable Combination in the Selection of New Antibacterial Compounds. Journal of Chemical Information and Computer Sciences, 44 (3), 1031–1041. doi: https://doi.org/10.1021/ci030340e
- Gabrielsson, R. B., Carlsson, G. (2019). Exposition and Interpretation of the Topology of Neural Networks. Paper presented at the 2019 18th IEEE International Conference On Machine Learning And Applications (ICMLA). doi: https://doi.org/10.1109/icmla.2019.00180
- Skinnider, M. A., Stacey, R. G., Wishart, D. S., Foster, L. J. (2021). Chemical language models enable navigation in sparsely populated chemical space. Nature Machine Intelligence, 3 (9), 759–770. doi: https://doi.org/10.1038/s42256-021-00368-1
- Tay, D. W. P., Yeo, N. Z. X., Adaikkappan, K., Lim, Y. H., Ang, S. J. (2023). 67 million natural product-like compound database generated via molecular language processing. Scientific Data, 10 (1). doi: https://doi.org/10.1038/s41597-023-02207-x
- Chen, H., Bajorath, J. (2023). Designing highly potent compounds using a chemical language model. Scientific Reports, 13 (1). doi: https://doi.org/10.1038/s41598-023-34683-x
- Bilodeau, C., Jin, W., Jaakkola, T., Barzilay, R., Jensen, K. F. (2022). Generative models for molecular discovery: Recent advances and challenges. WIREs Computational Molecular Science, 12 (5). doi: https://doi.org/10.1002/wcms.1608
- Pyzer-Knapp, E., Laino, T., Grisafi, A., Wilkins, D., Willatt, M., Ceriotti, M. et al. (2019). Machine Learning in Chemistry: Data-Driven Algorithms, Learning Systems, and Predictions. American Chemical Society. doi: https://doi.org/10.1021/bk-2019-1326
- Varmuza, K., Filzmoser, P. (2009). Introduction to Multivariate Statistical Analysis in Chemometrics. Boca Raton: CRC Press, 336. doi: https://doi.org/10.1201/9781420059496
- Pen, L. Z., Xian Xian, K., Yew, C. F., Hau, O. S., Sumari, P., Abualigah, L. et al. (2023). Classification Applications with Deep Learning and Machine Learning Technologies. Cham: Springer International Publishing. doi: https://doi.org/10.1007/978-3-031-17576-3
- Veerasamy, R., Rajak, H., Jain, A. K., Sivadasan, S., Varghese, C., K., A. R. (2011). Validation of QSAR Models-Strategies and Importance. International Journal of Drug Design and Discovery, 2 (3), 511–518.
- Alexander, D. L. J., Tropsha, A., Winkler, D. A. (2015). Beware of R2: Simple, Unambiguous Assessment of the Prediction Accuracy of QSAR and QSPR Models. Journal of Chemical Information and Modeling, 55 (7), 1316–1322. doi: https://doi.org/10.1021/acs.jcim.5b00206
- Consonni, V., Ballabio, D., Todeschini, R. (2009). Comments on the Definition of the Q2 Parameter for QSAR Validation. Journal of Chemical Information and Modeling, 49 (7), 1669–1678. doi: https://doi.org/10.1021/ci900115y
- Liu, H., Cocea, M. (2017). Semi-random partitioning of data into training and test sets in granular computing context. Granular Computing, 2 (4), 357–386. doi: https://doi.org/10.1007/s41066-017-0049-2
- Joseph, V. R. (2022). Optimal ratio for data splitting. Statistical Analysis and Data Mining: The ASA Data Science Journal, 15 (4), 531–538. doi: https://doi.org/10.1002/sam.11583
- Arlot, S., Celisse, A. (2010). A survey of cross-validation procedures for model selection. Statistics Surveys, 4, 40–79. doi: https://doi.org/10.1214/09-ss054
- Espinosa, G., Arenas, A., Giralt, F. (2001). Fundamentals of Molecular Similarity. Boston: Springer US.
- Mestres, J., Rohrer, D. C., Maggiora, G. M. (1997). MIMIC: A molecular-field matching program. Exploiting applicability of molecular similarity approaches. Journal of Computational Chemistry, 18 (7), 934–954. doi: https://doi.org/10.1002/(sici)1096-987x(199705)18:7<934::aid-jcc6>3.0.co;2-s
- Klebe, G. (Ed.) (2007). Virtual Screening: An Alternative or Complement to High Throughput Screening? Kluwer Academic Publishers.
- Bajusz, D., Rácz, A., Héberger, K.; Chackalamannil, S., Rotella, D., Ward, S. E. (Eds.) (2017). Chemical Data Formats, Fingerprints, and Other Molecular Descriptions for Database Analysis and Searching. Comprehensive Medicinal Chemistry III. Oxford: Elsevier, 329–378. doi: https://doi.org/10.1016/b978-0-12-409547-2.12345-5
- Capecchi, A., Probst, D., Reymond, J.-L. (2020). One molecular fingerprint to rule them all: drugs, biomolecules, and the metabolome. Journal of Cheminformatics, 12 (1). doi: https://doi.org/10.1186/s13321-020-00445-4
- Willett, P. (2006). Similarity-based virtual screening using 2D fingerprints. Drug Discovery Today, 11 (23-24), 1046–1053. doi: https://doi.org/10.1016/j.drudis.2006.10.005
- Scior, T., Bender, A., Tresadern, G., Medina-Franco, J. L., Martínez-Mayorga, K., Langer, T. et al. (2012). Recognizing Pitfalls in Virtual Screening: A Critical Review. Journal of Chemical Information and Modeling, 52 (4), 867–881. doi: https://doi.org/10.1021/ci200528d
- Landrum, G. (2020). RDKit: Open-source cheminformatics. Available at: https://www.rdkit.org/
- Alves, V. M., Bobrowski, T., Melo‐Filho, C. C., Korn, D., Auerbach, S., Schmitt, C. et al. (2020). QSAR Modeling of SARS‐CoV Mpro Inhibitors Identifies Sufugolix, Cenicriviroc, Proglumetacin, and other Drugs as Candidates for Repurposing against SARS‐CoV‐2. Molecular Informatics, 40 (1). doi: https://doi.org/10.1002/minf.202000113
- Morgan, H. L. (1965). The Generation of a Unique Machine Description for Chemical Structures-A Technique Developed at Chemical Abstracts Service. Journal of Chemical Documentation, 5 (2), 107–113. doi: https://doi.org/10.1021/c160017a018
- Willett, P. (2003). Similarity-based approaches to virtual screening. Biochemical Society Transactions, 31 (3), 603–606. doi: https://doi.org/10.1042/bst0310603
- Raymond, J. W., Willett, P. (2002). Effectiveness of graph-based and fingerprint-based similarity measures for virtual screening of 2D chemical structure databases. Journal of Computer-Aided Molecular Design, 16 (1), 59–71. doi: https://doi.org/10.1023/a:1016387816342
- Gossen, J., Albani, S., Hanke, A., Joseph, B. P., Bergh, C., Kuzikov, M. et al. (2021). A Blueprint for High Affinity SARS-CoV-2 Mpro Inhibitors from Activity-Based Compound Library Screening Guided by Analysis of Protein Dynamics. ACS Pharmacology & Translational Science, 4 (3), 1079–1095. doi: https://doi.org/10.1021/acsptsci.0c00215
- Nikolova, N., Jaworska, J. (2003). Approaches to Measure Chemical Similarity – a Review. QSAR & Combinatorial Science, 22 (9-10), 1006–1026. Portico. doi: https://doi.org/10.1002/qsar.200330831
- Stumpfe, D., Ripphausen, P., Bajorath, J. (2012). Virtual compound screening in drug discovery. Future Medicinal Chemistry, 4 (5), 593–602. doi: https://doi.org/10.4155/fmc.12.19
- Giordano, D., Biancaniello, C., Argenio, M. A., Facchiano, A. (2022). Drug Design by Pharmacophore and Virtual Screening Approach. Pharmaceuticals, 15 (5), 646. doi: https://doi.org/10.3390/ph15050646
- Glaab, E., Manoharan, G. B., Abankwa, D. (2021). Pharmacophore Model for SARS-CoV-2 3CLpro Small-Molecule Inhibitors and in Vitro Experimental Validation of Computationally Screened Inhibitors. Journal of Chemical Information and Modeling, 61 (8), 4082–4096. doi: https://doi.org/10.1021/acs.jcim.1c00258
- Wermuth, C. G., Ganellin, C. R., Lindberg, P., Mitscher, L. A. (1998). Glossary of terms used in medicinal chemistry (IUPAC Recommendations 1998). Pure and Applied Chemistry, 70 (5), 1129–1143. doi: https://doi.org/10.1351/pac199870051129
- Molecular Operating Environment (MOE). (2023). 2022.02 Chemical Computing Group ULC. Montreal.
- Wolber, G., Langer, T. (2004). LigandScout: 3-D Pharmacophores Derived from Protein-Bound Ligands and Their Use as Virtual Screening Filters. Journal of Chemical Information and Modeling, 45 (1), 160–169. doi: https://doi.org/10.1021/ci049885e
- Brooijmans, N., Kuntz, I. D. (2003). Molecular Recognition and Docking Algorithms. Annual Review of Biophysics and Biomolecular Structure, 32 (1), 335–373. doi: https://doi.org/10.1146/annurev.biophys.32.110601.142532
- Weiner, S. J., Kollman, P. A., Nguyen, D. T., Case, D. A. (1986). An all atom force field for simulations of proteins and nucleic acids. Journal of Computational Chemistry, 7 (2), 230–252. doi: https://doi.org/10.1002/jcc.540070216
- Gohlke, H., Hendlich, M., Klebe, G. (2000). Knowledge-based scoring function to predict protein-ligand interactions. Journal of Molecular Biology, 295 (2), 337–356. doi: https://doi.org/10.1006/jmbi.1999.3371
- Eldridge, M. D., Murray, C. W., Auton, T. R., Paolini, G. V., Mee, R. P. (1997). Empirical scoring functions: I. The development of a fast empirical scoring function to estimate the binding affinity of ligands in receptor complexes. Journal of Computer-Aided Molecular Design, 11 (5), 425–445. doi: https://doi.org/10.1023/a:1007996124545
- Alex, A., Finn, P. (1997). Fast and accurate predictions of relative binding energies. Journal of Molecular Structure: THEOCHEM, 398-399, 551–554. doi: https://doi.org/10.1016/s0166-1280(97)00031-6
- Ballester, P. J., Mitchell, J. B. O. (2010). A machine learning approach to predicting protein–ligand binding affinity with applications to molecular docking. Bioinformatics, 26 (9), 1169–1175. doi: https://doi.org/10.1093/bioinformatics/btq112
- Guedes, I. A., Barreto, A. M. S., Marinho, D., Krempser, E., Kuenemann, M. A., Sperandio, O. et al. (2021). New machine learning and physics-based scoring functions for drug discovery. Scientific Reports, 11 (1). doi: https://doi.org/10.1038/s41598-021-82410-1
- Charifson, P. S., Corkery, J. J., Murcko, M. A., Walters, W. P. (1999). Consensus Scoring: A Method for Obtaining Improved Hit Rates from Docking Databases of Three-Dimensional Structures into Proteins. Journal of Medicinal Chemistry, 42 (25), 5100–5109. doi: https://doi.org/10.1021/jm990352k
- Kumar, T. D. A. (2022). Drug Design. A Conceptual Overview. Abingdon: CRC Press. doi: https://doi.org/10.1201/9781003298755
- Kitchen, D. B., Decornez, H., Furr, J. R., Bajorath, J. (2004). Docking and scoring in virtual screening for drug discovery: methods and applications. Nature Reviews Drug Discovery, 3 (11), 935–949. doi: https://doi.org/10.1038/nrd1549
- Warren, G. L., Andrews, C. W., Capelli, A.-M., Clarke, B., LaLonde, J., Lambert, M. H. et al. (2005). A Critical Assessment of Docking Programs and Scoring Functions. Journal of Medicinal Chemistry, 49 (20), 5912–5931. doi: https://doi.org/10.1021/jm050362n
- Stahl, M., Rarey, M. (2001). Detailed Analysis of Scoring Functions for Virtual Screening. Journal of Medicinal Chemistry, 44 (7), 1035–1042. doi: https://doi.org/10.1021/jm0003992
- Zhu, H., Zhou, R., Cao, D., Tang, J., Li, M. (2023). A pharmacophore-guided deep learning approach for bioactive molecular generation. Nature Communications, 14 (1). doi: https://doi.org/10.1038/s41467-023-41454-9
- Mazanetz, M., Law, R., Whittaker, M. (2013). Hit and Lead Identification from Fragments. De novo Molecular Design. Weinheim: Wiley-VCH Verlag GmbH & Co.KGaA, 143–200. doi: https://doi.org/10.1002/9783527677016.ch6
- Wetzel, S., Klein, K., Renner, S., Rauh, D., Oprea, T. I., Mutzel, P., Waldmann, H. (2009). Interactive exploration of chemical space with Scaffold Hunter. Nature Chemical Biology, 5 (8), 581–583. doi: https://doi.org/10.1038/nchembio.187
- Langevin, M., Minoux, H., Levesque, M., Bianciotto, M. (2020). Scaffold-Constrained Molecular Generation. Journal of Chemical Information and Modeling, 60 (12), 5637–5646. doi: https://doi.org/10.1021/acs.jcim.0c01015
- Scott, O. B., Edith Chan, A. W. (2020). ScaffoldGraph: an open-source library for the generation and analysis of molecular scaffold networks and scaffold trees. Bioinformatics, 36 (12), 3930–3931. doi: https://doi.org/10.1093/bioinformatics/btaa219
- Schaub, J., Zander, J., Zielesny, A., Steinbeck, C. (2022). Scaffold Generator: a Java library implementing molecular scaffold functionalities in the Chemistry Development Kit (CDK). Journal of Cheminformatics, 14 (1). doi: https://doi.org/10.1186/s13321-022-00656-x
- Buckle, D. R., Erhardt, P. W., Ganellin, C. R., Kobayashi, T., Perun, T. J., Proudfoot, J., Senn-Bilfinger, J.; Desai, M. C. (Ed.) (2013). Glossary of Terms Used in Medicinal Chemistry Part II (IUPAC Recommendations 2013). Annual Reports in Medicinal Chemistry. Academic Press, 48, 387–418. doi: https://doi.org/10.1016/b978-0-12-417150-3.00024-7
- Hessler, G., Baringhaus, K.-H. (2010). The scaffold hopping potential of pharmacophores. Drug Discovery Today: Technologies, 7 (4), e263–e269. doi: https://doi.org/10.1016/j.ddtec.2010.09.001
- Brown, N. (Ed.) (2013). Scaffold Hopping in Medicinal Chemistry. Wiley-VCH. doi: https://doi.org/10.1002/9783527665143
- Forli, S., Huey, R., Pique, M. E., Sanner, M. F., Goodsell, D. S., Olson, A. J. (2016). Computational protein–ligand docking and virtual drug screening with the AutoDock suite. Nature Protocols, 11 (5), 905–919. doi: https://doi.org/10.1038/nprot.2016.051
- Goodsell, D. S., Morris, G. M., Olson, A. J. (1996). Automated docking of flexible ligands: Applications of AutoDock. Journal of Molecular Recognition, 9 (1), 1–5. doi: https://doi.org/10.1002/(sici)1099-1352(199601)9:1<1::aid-jmr241>3.0.co;2-6
- Trott, O., Olson, A. J. (2009). AutoDock Vina: Improving the speed and accuracy of docking with a new scoring function, efficient optimization, and multithreading. Journal of Computational Chemistry, 31 (2), 455–461. doi: https://doi.org/10.1002/jcc.21334
- Eberhardt, J., Santos-Martins, D., Tillack, A. F., Forli, S. (2021). AutoDock Vina 1.2.0: New Docking Methods, Expanded Force Field, and Python Bindings. Journal of Chemical Information and Modeling, 61 (8), 3891–3898. doi: https://doi.org/10.1021/acs.jcim.1c00203
- Jones, G., Willett, P., Glen, R. C., Leach, A. R., Taylor, R. (1997). Development and validation of a genetic algorithm for flexible docking. Journal of Molecular Biology, 267 (3), 727–748. doi: https://doi.org/10.1006/jmbi.1996.0897
- Grosdidier, A., Zoete, V., Michielin, O. (2011). SwissDock, a protein-small molecule docking web service based on EADock DSS. Nucleic Acids Research, 39, W270–W277. doi: https://doi.org/10.1093/nar/gkr366
- Kramer, B., Rarey, M., Lengauer, T. (1999). Evaluation of the FLEXX incremental construction algorithm for protein-ligand docking. Proteins: Structure, Function, and Genetics, 37 (2), 228–241. doi: https://doi.org/10.1002/(sici)1097-0134(19991101)37:2<228::aid-prot8>3.0.co;2-8
- Saeedi, S., Rahmati, A., Chavoshpour-Natanzi, Z. (2022). Synthesis of pyrazolo[5′,1′:2,3]imidazo[1,5-c]quinazolin-6(5H)-ones and molecular docking study of their affinity against the COVID-19 main protease. RSC Advances, 12 (30), 19579–19589. doi: https://doi.org/10.1039/d2ra03179e
- Amendola, G., Ettari, R., Previti, S., Di Chio, C., Messere, A., Di Maro, S. et al. (2021). Lead Discovery of SARS-CoV-2 Main Protease Inhibitors through Covalent Docking-Based Virtual Screening. Journal of Chemical Information and Modeling, 61 (4), 2062–2073. doi: https://doi.org/10.1021/acs.jcim.1c00184
- Porto, V. A., Porto, R. S. (2022). In silico Studies of Novel Synthetic Compounds as Potential Drugs to Inhibit Coronavirus (SARS-CoV-2): A Systematic Review. Biointerface Research in Applied Chemistry, 12 (4), 4293–4306. doi: https://doi.org/10.33263/briac124.42934306
- Hou, N., Shuai, L., Zhang, L., Xie, X., Tang, K., Zhu, Y. et al. (2023). Development of Highly Potent Noncovalent Inhibitors of SARS-CoV-2 3CLpro. ACS Central Science, 9 (2), 217–227. doi: https://doi.org/10.1021/acscentsci.2c01359
- Evseeva, L. V., Ivanov, V. V., Karpina, V. R., Kovalenko, S. S., Kuznetsov, I. E., Langer, T. et al. (2020). The virtual screening application for searching potential antiviral agents to treat COVID-19 disease. Journal of Organic and Pharmaceutical Chemistry, 18 (2 (70)), 3–15. doi: https://doi.org/10.24959/ophcj.20.200019
- Kulkarni, S. A., Nagarajan, S. K., Ramesh, V., Palaniyandi, V., Selvam, S. P., Madhavan, T. (2020). Computational evaluation of major components from plant essential oils as potent inhibitors of SARS-CoV-2 spike protein. Journal of Molecular Structure, 1221, 128823. doi: https://doi.org/10.1016/j.molstruc.2020.128823
- Ahmad, M., Dwivedy, A., Mariadasse, R., Tiwari, S., Kar, D., Jeyakanthan, J., Biswal, B. K. (2020). Prediction of Small Molecule Inhibitors Targeting the Severe Acute Respiratory Syndrome Coronavirus-2 RNA-dependent RNA Polymerase. ACS Omega, 5 (29), 18356–18366. doi: https://doi.org/10.1021/acsomega.0c02096
- Lohachova, K. O., Sviatenko, A. S., Kyrychenko, A., Ivanov, V. V., Langer, T., Kovalenko, S. M., Kalugin, O. N. (2023). Computer-aided drug design of novel nirmatrelvir analogs inhibiting main protease of Coronavirus SARS-CoV-2. Journal of Applied Pharmaceutical Science, 13. In press
- Tejera, E., Munteanu, C. R., López-Cortés, A., Cabrera-Andrade, A., Pérez-Castillo, Y. (2020). Drugs Repurposing Using QSAR, Docking and Molecular Dynamics for Possible Inhibitors of the SARS-CoV-2 Mpro Protease. Molecules, 25 (21), 5172. doi: https://doi.org/10.3390/molecules25215172
- Pathak, N., Chen, Y.-T., Hsu, Y.-C., Hsu, N.-Y., Kuo, C.-J., Tsai, H. P. (2020). Uncovering Flexible Active Site Conformations of SARS-CoV-2 3CL Proteases through Protease Pharmacophore Clusters and COVID-19 Drug Repurposing. ACS Nano, 15 (1), 857–872. doi: https://doi.org/10.1021/acsnano.0c07383
- Zhang, C.-H., Stone, E. A., Deshmukh, M., Ippolito, J. A., Ghahremanpour, M. M., Tirado-Rives, J. et al. (2021). Potent Noncovalent Inhibitors of the Main Protease of SARS-CoV-2 from Molecular Sculpting of the Drug Perampanel Guided by Free Energy Perturbation Calculations. ACS Central Science, 7 (3), 467–475. doi: https://doi.org/10.1021/acscentsci.1c00039
- Salo-Ahen, O. M. H., Alanko, I., Bhadane, R., Bonvin, A. M. J. J., Honorato, R. V., Hossain, S. et al. (2020). Molecular Dynamics Simulations in Drug Discovery and Pharmaceutical Development. Processes, 9 (1), 71. doi: https://doi.org/10.3390/pr9010071
- Allen, M. P., Tildesley, D. J. (2017). Computer Simulation of Liquids: Second Edition. Oxford: Oxford University Press. doi: https://doi.org/10.1093/oso/9780198803195.001.0001
- Christen, M., Hünenberger, P. H., Bakowies, D., Baron, R., Bürgi, R., Geerke, D. P. et al. (2005). The GROMOS software for biomolecular simulation: GROMOS05. Journal of Computational Chemistry, 26 (16), 1719–1751. doi: https://doi.org/10.1002/jcc.20303
- Van Der Spoel, D., Lindahl, E., Hess, B., Groenhof, G., Mark, A. E., Berendsen, H. J. C. (2005). GROMACS: Fast, flexible, and free. Journal of Computational Chemistry, 26 (16), 1701–1718. doi: https://doi.org/10.1002/jcc.20291
- Kalé, L., Skeel, R., Bhandarkar, M., Brunner, R., Gursoy, A., Krawetz, N. et al. (1999). NAMD2: Greater Scalability for Parallel Molecular Dynamics. Journal of Computational Physics, 151 (1), 283–312. doi: https://doi.org/10.1006/jcph.1999.6201
- Brooks, B. R., Bruccoleri, R. E., Olafson, B. D., States, D. J., Swaminathan, S., Karplus, M. (1983). CHARMM: A program for macromolecular energy, minimization, and dynamics calculations. Journal of Computational Chemistry, 4 (2), 187–217. doi: https://doi.org/10.1002/jcc.540040211
- Case, D. A., Cheatham, T. E., Darden, T., Gohlke, H., Luo, R., Merz, K. M. et al. (2005). The Amber biomolecular simulation programs. Journal of Computational Chemistry, 26 (16), 1668–1688. doi: https://doi.org/10.1002/jcc.20290
- Thompson, A. P., Aktulga, H. M., Berger, R., Bolintineanu, D. S., Brown, W. M., Crozier, P. S. et al. (2022). LAMMPS – a flexible simulation tool for particle-based materials modeling at the atomic, meso, and continuum scales. Computer Physics Communications, 271, 108171. doi: https://doi.org/10.1016/j.cpc.2021.108171
- Smith, W., Yong, C. W., Rodger, P. M. (2002). DL_POLY: Application to molecular simulation. Molecular Simulation, 28 (5), 385–471. doi: https://doi.org/10.1080/08927020290018769
- Van Der Spoel, D., Lindahl, E., Hess, B., van Buuren, A. R., Apol, E., Meulenhoff, P. J. et al. (2010). Gromacs User Manual version 4.5.4. Available at: www.gromacs.org
- Verkhivker, G. M., Agajanian, S., Oztas, D. Y., Gupta, G. (2021). Atomistic Simulations and In Silico Mutational Profiling of Protein Stability and Binding in the SARS-CoV-2 Spike Protein Complexes with Nanobodies: Molecular Determinants of Mutational Escape Mechanisms. ACS Omega, 6 (40), 26354–26371. doi: https://doi.org/10.1021/acsomega.1c03558
- Domingo, M., Faraudo, J. (2023). Effect of surfactants on SARS-CoV-2: Molecular dynamics simulations. The Journal of Chemical Physics, 158 (11). doi: https://doi.org/10.1063/5.0135251
- Samanta, P. N., Majumdar, D., Leszczynski, J. (2023). Elucidating Atomistic Insight into the Dynamical Responses of the SARS-CoV-2 Main Protease for the Binding of Remdesivir Analogues: Leveraging Molecular Mechanics To Decode the Inhibition Mechanism. Journal of Chemical Information and Modeling, 63 (11), 3404–3422. doi: https://doi.org/10.1021/acs.jcim.3c00105
- Rashid, H. ur, Ahmad, N., Abdalla, M., Khan, K., Martines, M. A. U., Shabana, S. (2022). Molecular docking and dynamic simulations of Cefixime, Etoposide and Nebrodenside A against the pathogenic proteins of SARS-CoV-2. Journal of Molecular Structure, 1247, 131296. doi: https://doi.org/10.1016/j.molstruc.2021.131296
- Muhseen, Z. T., Hameed, A. R., Al-Hasani, H. M. H., Tahir ul Qamar, M., Li, G. (2020). Promising terpenes as SARS-CoV-2 spike receptor-binding domain (RBD) attachment inhibitors to the human ACE2 receptor: Integrated computational approach. Journal of Molecular Liquids, 320, 114493. doi: https://doi.org/10.1016/j.molliq.2020.114493
- Wakchaure, P. D., Ghosh, S., Ganguly, B. (2020). Revealing the Inhibition Mechanism of RNA-Dependent RNA Polymerase (RdRp) of SARS-CoV-2 by Remdesivir and Nucleotide Analogues: A Molecular Dynamics Simulation Study. The Journal of Physical Chemistry B, 124 (47), 10641–10652. doi: https://doi.org/10.1021/acs.jpcb.0c06747
- Byléhn, F., Menéndez, C. A., Perez-Lemus, G. R., Alvarado, W., de Pablo, J. J. (2021). Modeling the Binding Mechanism of Remdesivir, Favilavir, and Ribavirin to SARS-CoV-2 RNA-Dependent RNA Polymerase. ACS Central Science, 7 (1), 164–174. doi: https://doi.org/10.1021/acscentsci.0c01242
- Ghorbani, M., Brooks, B. R., Klauda, J. B. (2020). Critical Sequence Hotspots for Binding of Novel Coronavirus to Angiotensin Converter Enzyme as Evaluated by Molecular Simulations. The Journal of Physical Chemistry B, 124 (45), 10034–10047. doi: https://doi.org/10.1021/acs.jpcb.0c05994
- Carli, M., Sormani, G., Rodriguez, A., Laio, A. (2020). Candidate Binding Sites for Allosteric Inhibition of the SARS-CoV-2 Main Protease from the Analysis of Large-Scale Molecular Dynamics Simulations. The Journal of Physical Chemistry Letters, 12 (1), 65–72. doi: https://doi.org/10.1021/acs.jpclett.0c03182
- Sakkiah, S., Guo, W., Pan, B., Ji, Z., Yavas, G., Azevedo, M. et al. (2021). Elucidating Interactions Between SARS-CoV-2 Trimeric Spike Protein and ACE2 Using Homology Modeling and Molecular Dynamics Simulations. Frontiers in Chemistry, 8, 622632. doi: https://doi.org/10.3389/fchem.2020.622632
- Sheik Amamuddy, O., Verkhivker, G. M., Tastan Bishop, Ö. (2020). Impact of Early Pandemic Stage Mutations on Molecular Dynamics of SARS-CoV-2 Mpro. Journal of Chemical Information and Modeling, 60 (10), 5080–5102. doi: https://doi.org/10.1021/acs.jcim.0c00634
- Rajagopal, K., Varakumar, P., Aparna, B., Byran, G., Jupudi, S. (2020). Identification of some novel oxazine substituted 9-anilinoacridines as SARS-CoV-2 inhibitors for COVID-19 by molecular docking, free energy calculation and molecular dynamics studies. Journal of Biomolecular Structure and Dynamics, 39 (15), 5551–5562. doi: https://doi.org/10.1080/07391102.2020.1798285
- Yoshino, R., Yasuo, N., Sekijima, M. (2020). Identification of key interactions between SARS-CoV-2 main protease and inhibitor drug candidates. Scientific Reports, 10 (1), 12493. doi: https://doi.org/10.1038/s41598-020-69337-9
- Sang, P., Tian, S.-H., Meng, Z.-H., Yang, L.-Q. (2020). Anti-HIV drug repurposing against SARS-CoV-2. RSC Advances, 10 (27), 15775–15783. doi: https://doi.org/10.1039/d0ra01899f
- Mukherjee, S., Dasgupta, S., Adhikary, T., Adhikari, U., Panja, S. S. (2020). Structural insight to hydroxychloroquine-3C-like proteinase complexation from SARS-CoV-2: inhibitor modelling study through molecular docking and MD-simulation study. Journal of Biomolecular Structure and Dynamics, 39 (18), 7322–7334. doi: https://doi.org/10.1080/07391102.2020.1804458
- Grottesi, A., Bešker, N., Emerson, A., Manelfi, C., Beccari, A. R., Frigerio, F. et al. (2020). Computational Studies of SARS-CoV-2 3CLpro: Insights from MD Simulations. International Journal of Molecular Sciences, 21 (15), 5346. doi: https://doi.org/10.3390/ijms21155346
- Kumar, D., Kumari, K., Jayaraj, A., Kumar, V., Kumar, R. V., Dass, S. K. et al. (2020). Understanding the binding affinity of noscapines with protease of SARS-CoV-2 for COVID-19 using MD simulations at different temperatures. Journal of Biomolecular Structure and Dynamics, 39 (7), 2659–2672. doi: https://doi.org/10.1080/07391102.2020.1752310
- Al-Khafaji, K., AL-Duhaidahawi, D., Taskin Tok, T. (2021). Using integrated computational approaches to identify safe and rapid treatment for SARS-CoV-2. Journal of Biomolecular Structure and Dynamics, 39 (9), 3387–3395. doi: https://doi.org/10.1080/07391102.2020.1764392
- Muhammad, I. A., Muangchoo, K., Muhammad, A., Ajingi, Y. S., Muhammad, I. Y., Umar, I. D., Muhammad, A. B. (2020). A Computational Study to Identify Potential Inhibitors of SARS-CoV-2 Main Protease (Mpro) from Eucalyptus Active Compounds. Computation, 8 (3), 79. doi: https://doi.org/10.3390/computation8030079
- Maurya, A. K., Mishra, N. (2020). In silico validation of coumarin derivatives as potential inhibitors against Main Protease, NSP10/NSP16-Methyltransferase, Phosphatase and Endoribonuclease of SARS CoV-2. Journal of Biomolecular Structure and Dynamics, 39 (18), 7306–7321. doi: https://doi.org/10.1080/07391102.2020.1808075
- Alamri, M. A., Tahir ul Qamar, M., Mirza, M. U., Bhadane, R., Alqahtani, S. M., Muneer, I. et al. (2020). Pharmacoinformatics and molecular dynamics simulation studies reveal potential covalent and FDA-approved inhibitors of SARS-CoV-2 main protease 3CLpro. Journal of Biomolecular Structure and Dynamics, 39 (13), 4936–4948. doi: https://doi.org/10.1080/07391102.2020.1782768
- Stoddard, S. V., Stoddard, S. D., Oelkers, B. K., Fitts, K., Whalum, K., Whalum, K. et al. (2020). Optimization Rules for SARS-CoV-2 Mpro Antivirals: Ensemble Docking and Exploration of the Coronavirus Protease Active Site. Viruses, 12 (9), 942. doi: https://doi.org/10.3390/v12090942
- Rout, J., Swain, B. C., Tripathy, U. (2020). In silicoinvestigation of spice molecules as potent inhibitor of SARS-CoV-2. Journal of Biomolecular Structure and Dynamics, 40 (2), 860–874. doi: https://doi.org/10.1080/07391102.2020.1819879
- Umesh, Kundu, D., Selvaraj, C., Singh, S. K., Dubey, V. K. (2021). Identification of new anti-nCoV drug chemical compounds from Indian spices exploiting SARS-CoV-2 main protease as target. Journal of Biomolecular Structure and Dynamics, 39 (9), 3428–3434. doi: https://doi.org/10.1080/07391102.2020.1763202
- El Hassab, M. A., Shoun, A. A., Al-Rashood, S. T., Al-Warhi, T., Eldehna, W. M. (2020). Identification of a New Potential SARS-COV-2 RNA-Dependent RNA Polymerase Inhibitor via Combining Fragment-Based Drug Design, Docking, Molecular Dynamics, and MM-PBSA Calculations. Frontiers in Chemistry, 8, 584894. doi: https://doi.org/10.3389/fchem.2020.584894
- Gangadevi, S., Badavath, V. N., Thakur, A., Yin, N., De Jonghe, S., Acevedo, O. et al. (2021). Kobophenol A Inhibits Binding of Host ACE2 Receptor with Spike RBD Domain of SARS-CoV-2, a Lead Compound for Blocking COVID-19. The Journal of Physical Chemistry Letters, 12 (7), 1793–1802. doi: https://doi.org/10.1021/acs.jpclett.0c03119
- Liang, J., Karagiannis, C., Pitsillou, E., Darmawan, K. K., Ng, K., Hung, A., Karagiannis, T. C. (2020). Site mapping and small molecule blind docking reveal a possible target site on the SARS-CoV-2 main protease dimer interface. Computational Biology and Chemistry, 89, 107372. doi: https://doi.org/10.1016/j.compbiolchem.2020.107372
- Khan, M. A., Meena, S., Alam, Md. A., Ghosh, S. (2020). A solvent sensitive coumarin derivative coupled with gold nanoparticles as selective fluorescent sensor for Pb2+ ions in real samples. Spectrochimica Acta Part A: Molecular and Biomolecular Spectroscopy, 243, 118810. doi: https://doi.org/10.1016/j.saa.2020.118810
- Kumar, K. A., Sharma, M., Dalal, V., Singh, V., Tomar, S., Kumar, P. (2021). Multifunctional inhibitors of SARS-CoV-2 by MM/PBSA, essential dynamics, and molecular dynamic investigations. Journal of Molecular Graphics and Modelling, 107, 107969. doi: https://doi.org/10.1016/j.jmgm.2021.107969
- Eltayb, W. A., Abdalla, M., Rabie, A. M. (2023). Novel Investigational Anti-SARS-CoV-2 Agent Ensitrelvir “S-217622”: A Very Promising Potential Universal Broad-Spectrum Antiviral at the Therapeutic Frontline of Coronavirus Species. ACS Omega, 8 (6), 5234–5246. doi: https://doi.org/10.1021/acsomega.2c03881
- King, E., Aitchison, E., Li, H., Luo, R. (2021). Recent Developments in Free Energy Calculations for Drug Discovery. Frontiers in Molecular Biosciences, 8, 712085. doi: https://doi.org/10.3389/fmolb.2021.712085
- Zara, L., Efrém, N.-L., van Muijlwijk-Koezen, J. E., de Esch, I. J. P., Zarzycka, B. (2021). Progress in Free Energy Perturbation: Options for Evolving Fragments. Drug Discovery Today: Technologies, 40, 36–42. doi: https://doi.org/10.1016/j.ddtec.2021.10.001
- Hansson, T., Marelius, J., Åqvist, J. (1998). Ligand binding affinity prediction by linear interaction energy methods. Journal of Computer-Aided Molecular Design, 12 (1), 27–35. doi: https://doi.org/10.1023/a:1007930623000
- Ngo, S. T., Hung, H. M., Nguyen, M. T. (2016). Fast and accurate determination of the relative binding affinities of small compounds to HIV-1 protease using non-equilibrium work. Journal of Computational Chemistry, 37 (31), 2734–2742. doi: https://doi.org/10.1002/jcc.24502
- Kollman, P. A., Massova, I., Reyes, C., Kuhn, B., Huo, S., Chong, L. et al. (2000). Calculating Structures and Free Energies of Complex Molecules: Combining Molecular Mechanics and Continuum Models. Accounts of Chemical Research, 33 (12), 889–897. doi: https://doi.org/10.1021/ar000033j
- Jarzynski, C. (1997). Equilibrium free-energy differences from nonequilibrium measurements: A master-equation approach. Physical Review E, 56 (5), 5018–5035. doi: https://doi.org/10.1103/physreve.56.5018
- Kollman, P. (1993). Free energy calculations: Applications to chemical and biochemical phenomena. Chemical Reviews, 93 (7), 2395–2417. doi: https://doi.org/10.1021/cr00023a004
- Zwanzig, R. W. (2004). High-Temperature Equation of State by a Perturbation Method. I. Nonpolar Gases. The Journal of Chemical Physics, 22 (8), 1420–1426. doi: https://doi.org/10.1063/1.1740409
- Fu, H., Chen, H., Cai, W., Shao, X., Chipot, C. (2021). BFEE2: Automated, Streamlined, and Accurate Absolute Binding Free-Energy Calculations. Journal of Chemical Information and Modeling, 61 (5), 2116–2123. doi: https://doi.org/10.1021/acs.jcim.1c00269
- Blazhynska, M., Goulard Coderc de Lacam, E., Chen, H., Roux, B., Chipot, C. (2022). Hazardous Shortcuts in Standard Binding Free Energy Calculations. The Journal of Physical Chemistry Letters, 13 (27), 6250–6258. doi: https://doi.org/10.1021/acs.jpclett.2c01490
- Coderc de Lacam, E. G., Blazhynska, M., Chen, H., Gumbart, J. C., Chipot, C. (2022). When the Dust Has Settled: Calculation of Binding Affinities from First Principles for SARS-CoV-2 Variants with Quantitative Accuracy. Journal of Chemical Theory and Computation, 18 (10), 5890–5900. doi: https://doi.org/10.1021/acs.jctc.2c00604
- Gupta, A., Zhou, H.-X. (2020). Profiling SARS-CoV-2 Main Protease (MPRO) Binding to Repurposed Drugs Using Molecular Dynamics Simulations in Classical and Neural Network-Trained Force Fields. ACS Combinatorial Science, 22 (12), 826–832. doi: https://doi.org/10.1021/acscombsci.0c00140
- Ngo, S. T., Nguyen, T. H., Tung, N. T., Mai, B. K. (2022). Insights into the binding and covalent inhibition mechanism of PF-07321332 to SARS-CoV-2 Mpro. RSC Advances, 12 (6), 3729–3737. doi: https://doi.org/10.1039/d1ra08752e
- Zanetti-Polzi, L., Smith, M. D., Chipot, C., Gumbart, J. C., Lynch, D. L., Pavlova, A. et al. (2021). Tuning Proton Transfer Thermodynamics in SARS-CoV-2 Main Protease: Implications for Catalysis and Inhibitor Design. The Journal of Physical Chemistry Letters, 12 (17), 4195–4202. doi: https://doi.org/10.1021/acs.jpclett.1c00425
- Qayed, W. S., Ferreira, R. S., Silva, J. R. A. (2022). In Silico Study towards Repositioning of FDA-Approved Drug Candidates for Anticoronaviral Therapy: Molecular Docking, Molecular Dynamics and Binding Free Energy Calculations. Molecules, 27 (18), 5988. doi: https://doi.org/10.3390/molecules27185988
- Parks, C. D., Gaieb, Z., Chiu, M., Yang, H., Shao, C., Walters, W. P. et al. (2020). D3R grand challenge 4: blind prediction of protein–ligand poses, affinity rankings, and relative binding free energies. Journal of Computer-Aided Molecular Design, 34 (2), 99–119. doi: https://doi.org/10.1007/s10822-020-00289-y
- Gentile, F., Yaacoub, J. C., Gleave, J., Fernandez, M., Ton, A.-T., Ban, F. et al. (2022). Artificial intelligence-enabled virtual screening of ultra-large chemical libraries with deep docking. Nature Protocols, 17 (3), 672–697. doi: https://doi.org/10.1038/s41596-021-00659-2
- Gorgulla, C., Boeszoermenyi, A., Wang, Z.-F., Fischer, P. D., Coote, P. W., Padmanabha Das, K. M. et al. (2020). An open-source drug discovery platform enables ultra-large virtual screens. Nature, 580 (7805), 663–668. doi: https://doi.org/10.1038/s41586-020-2117-z
- Arantes, P. R., Saha, A., Palermo, G. (2020). Fighting COVID-19 Using Molecular Dynamics Simulations. ACS Central Science, 6 (10), 1654–1656. doi: https://doi.org/10.1021/acscentsci.0c01236
- Frye, L., Bhat, S., Akinsanya, K., Abel, R. (2021). From computer-aided drug discovery to computer-driven drug discovery. Drug Discovery Today: Technologies, 39, 111–117. doi: https://doi.org/10.1016/j.ddtec.2021.08.001
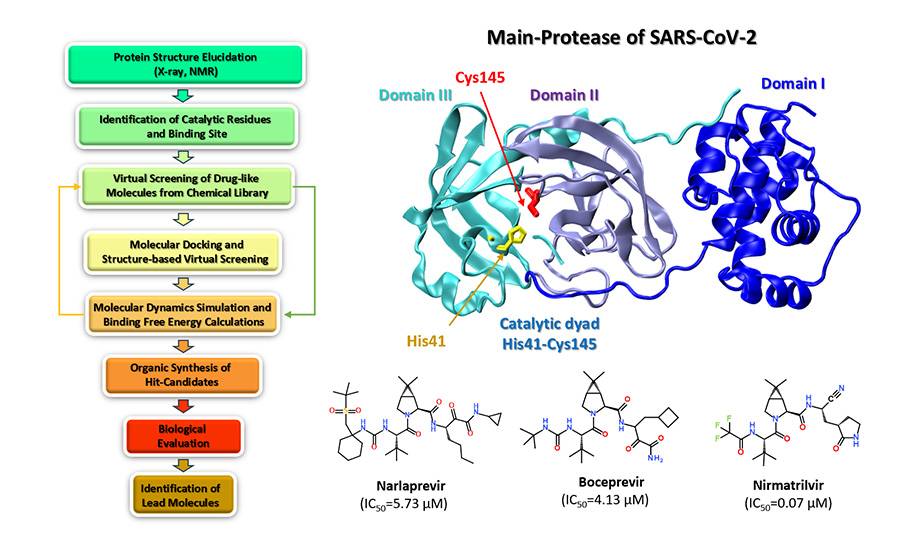
Downloads
Published
How to Cite
Issue
Section
License
Copyright (c) 2023 Volodymyr Ivanov, Kateryna Lohachova, Yaroslav Kolesnik, Anton Zakharov, Larysa Yevsieieva, Alexander Kyrychenko, Thierry Langer, Sergiy M. Kovalenko, Oleg N. Kalugin

This work is licensed under a Creative Commons Attribution 4.0 International License.
Our journal abides by the Creative Commons CC BY copyright rights and permissions for open access journals.