Analysis of body parts of boxers during punching using modern technologies
DOI:
https://doi.org/10.15391/ed.2024-1.06Keywords:
boxing, competitive activity, impact, modern technologies, detection, modeling, body partsAbstract
Purpose: to evaluate the effectiveness of using modern computer vision technologies to determine the body parts of boxers during a punch. Material and methods. During the research, the following methods were used: analysis of scientific and methodological literature and Internet resources, analysis of videos and images using machine learning technologies (in particular, computer vision technologies), as well as the application of mathematical statistics methods. The final boxing match in the weight category up to 91 kg at the Olympic Games (Tokyo, 2021) was chosen for analysis. The total number of images for analysis was 1025. In the study, YOLO machine learning models were used to detect the persons who were in the images and MediaPipe to determine the body parts of each of the athletes. Results: as part of a scientific study of detecting the YOLO pattern during a boxing match, fairly high results were obtained. The first athlete in a red uniform was detected with high accuracy - he was not detected on only 1,4% of images; the second athlete in the blue uniform was detected in 98,7 % of all images. The probability of correctly identifying the athlete by the model was 89,5 % and 91,2 %, respectively, for the first and second athlete. Analysis of the results indicates that the MediaPipe model has limitations in identifying certain body parts of athletes during martial arts competitions. In particular, the percentage of images in which body parts could not be identified varies from 21,7 % to 31,7 %. The overall picture shows that the model successfully identifies key body parts such as shoulders, elbows, wrists, palms, and fingers, with a probability of results ranging from 61,5 % to 74,5 %. But visual direct analysis shows certain problems with determining the movements of athletes. Conclusions. An analysis of the results of the use of modern computer vision technologies to determine the body parts of boxers during striking in competitive activities was carried out. The results indicate the high efficiency and accuracy of the YOLO model in the task of detecting athletes during sports events. But at the same time, opposite data were obtained using the MediaPipe model to determine the body parts of athletes. Visual direct analysis shows certain problems with determining the movements of athletes. The general trend is that in the conditions of boxing competitions, the MediaPipe model may face challenges related to the specifics of this sport and require further optimizations to achieve the highest accuracy and reliability in the identification of boxers' body parts. But at the same time, the integration of computer vision technologies into sports events opens up new opportunities for objective analysis and improvement of the technical skills of martial artists.
References
Архипов, О.А., & Питомець, О.П. (2016). Якісний біомеханічний аналіз техніки бігу на короткі, середні та довгі дистанції. Науковий часопис НПУ імені М.П. Драгоманова, 3 (70), 285-288.
Гамалій В. (2020). Біомеханічні аспекти раціоналізації процесу навчання рухів у процесі технічної підготовки спортсменів. Теорія і методика фізичного виховання і спорту, 28(2), 36-41. DOI:10.32652/tmfvs.2020.2.36-41
Камаєв, О.І., Тропін, Ю.М., & Арнаут, В.Ю. (2019). Біомеханічний аналіз виконання технічних дій у спортивній боротьбі. Проблеми і перспективи розвитку спортивних ігор і єдиноборств у вищих навчальних закладах, 1, 32-35.
Коробейніков, Г., Воронцов, А., Костюченко, В., & Григоренко, О. (2020). Аналіз змагальної діяльності збірної команди України з греко-римської боротьби на чемпіонатах Європи 2019–2020 років. Теорія і методика фізичного виховання і спорту, (4), 27-33 DOI:10.32652/tmfvs.2020.4.27-33
Латишев М.В., Поляничко О.М., Вольський Д.С., Лахтадир О.В., & Рибак Л.О. (2022). Аналіз попередніх досягнень призерів чемпіонату світу 2022 року з вільної боротьби. Єдиноборства, 4(26), 44–53 DOI:10.15391/ed.2022-4.05
Латишев, М., Рибак, Л., Штанагей, Д., Вольський, Д., & Омельчук, М. (2023). Сучасні технології для визначення ланок тіла борців. Єдиноборства, (4 (30)), 49-58. DOI:10.15391/ed.2023-4.05
Латишев, М.В., Квасниця, О.М., Спесивих, О.О., & Квасниця, І.М. (2019). Прогнозування: методи, критерії та спортивний результат. Спортивний вісник Придніпров’я, 1, 39-47 DOI:10.32540/2071-1476-2019-1-039
Подригало, Л.В., & Володченко, О.А. (2016). Порівняльний аналіз біомеханічних аспектів кік-боксу та інших одноборств. Вісник Чернігівського національного педагогічного університету. Серія: Педагогічні науки. Фізичне виховання та спорт, 139 (1), 145-149.
Шандригось, В.І. (2018a). Cистемно-історичний аналіз досягнень країн-учасниць в змаганнях з вільної боротьби на Олімпійських Іграх (1904-2016 рр.). Єдиноборства, 1 (7), 89-97.
Шандригось, В.І. (2018b). Використання інформаційних технологій в теорії і практиці спортивних єдиноборств. Науково-методичні основи використання інформаційних технологій в галузі фізичної культури та спорту, 25(2), 137-41.
Шандригось, В.І., Блажейко, А.І., & Латишев, М.В. (2022). Стан і перспективи розвитку вільної боротьби в Україні. Єдиноборства, №2(24), 96–116 DOI:10.15391/ed.2022-2.09
Штанагей, Д.В., Коробейніков, Г.В., Колумбет, О.М., & Дудорова, Л.Ю. (2021). The study of the dynamic characteristics of hand movements of female boxers with different types of functional asymmetry. Науковий часопис Національного педагогічного університету імені МП Драгоманова, (7 (138)), 16-21 DOI 10.31392/NPU-nc.series15.2021.7(138).03
Cook, G., Burton, L., Hoogenboom, B.J., & Voight, M. (2014). Functional movement screening: The use of fundamental movements as an assessment of function‐part 1. International journal of sports physical therapy, 9(3), 396.
Groos, D., Ramampiaro, H., & Ihlen, E.A. (2021). EfficientPose: Scalable single-person pose estimation. Applied intelligence, 51, 2518-2533 DOI:10.1007/s10489-020-01918-7
Jiang, P., Ergu, D., Liu, F., Cai, Y., & Ma, B. (2022). A Review of Yolo algorithm developments. Procedia Computer Science, 199, 1066-1073 DOI:10.1016/j.procs.2022.01.135
Latyshev, M., Shandrygos, V., Tropin, Y., Polianychko, O., Deineko, A., Lakhtadyr, O., & Mozoliuk, O. (2021). Age distribution of wrestlers participating in the world championships. Acta Kinesiologica, 15 (1): 138-143 DOI:10.51371/issn.1840-2976.2021.15.1.17
Latyshev, M., Tropin, Y., Podrigalo, L., & Boychenko, N. (2022). Analysis of the Relative Age Effect in Elite Wrestlers. Ido movement for culture. Journal of Martial Arts Anthropology, (22 (3)), 28-32 DOI:10.14589/ido.22.3.5
Pardos, A., Tziomaka, M., Menychtas, A. & Maglogiannis, I. (2022). Automated Posture Analysis for the Assessment of Sports Exercises. In Proceedings of the 12th Hellenic Conference on Artificial Intelligence, 1-9 DOI:10.1145/3549737.3549784
Shandrygos, V.I., Blazheyko, A.I., Latyshev, N.V., Tropyn, Y.N., Boychenko, N.V., & Myroshnychenho, Y.S. (2022). Analysis of the performances of the national team of Ukraine in women’s wrestling at official competitions (1992–2021): second message. Rehabilitation & Recreation, 10, 170-183. DOI:10.32782/2522-1795.2022.10.22
Shandrygos, V.I., Boychenko, N.V., Tropyn, Y.N., & Latyshev, N.V. (2023). Influence of functional asymmetry on performance of technical actions at freestyle wrestlers. Martial artse, 1(27), 110-122. DOI:10.15391/ed.2023-1.10
Tropin, Y., Podrigalo, L., Boychenko, N., Podrihalo, O., Volodchenko, O., Volskyi, D., & Roztorhui, M. (2023). Analyzing predictive approaches in martial arts research. Pedagogy of Physical Culture and Sports, 27(4), 321–330. DOI:10.15561/26649837.2023.0408
Tropin, Y., Romanenko, V., Cynarski, W., Boychenko, N., & Kovalenko, J. (2022). Model characteristics of competitive activity of MMA mixed martial arts athletes of different weight categories. Slobozhanskyi Herald of Science and Sport, 2(26), 41-46. DOI:10.15391/snsv.2022-2
Wang, J., Qiu, K., Peng, H., Fu, J., & Zhu, J. (2019). AI coach: Deep human pose estimation and analysis for personalized athletic training assistance. In Proceedings of the 27th ACM international conference on multimedia, 374-382 DOI:10.1145/3343031.3350910
Youtube. Men's Boxing Super Heavyweight +91kg Final Tokyo Access Mode: – [Electronic resource]. – Access mode https://www.youtube.com/watch?v=ms80nCeFYds (date of application 01.11.2023).
Zhang, W., Liu, Z., Zhou, L., Leung, H., & Chan, A. B. (2017). Martial arts, dancing and sports dataset: A challenging stereo and multi-view dataset for 3D human pose estimation. Image and Vision Computing, 61, 22-39 DOI:10.1016/j.imavis.2017.02.002
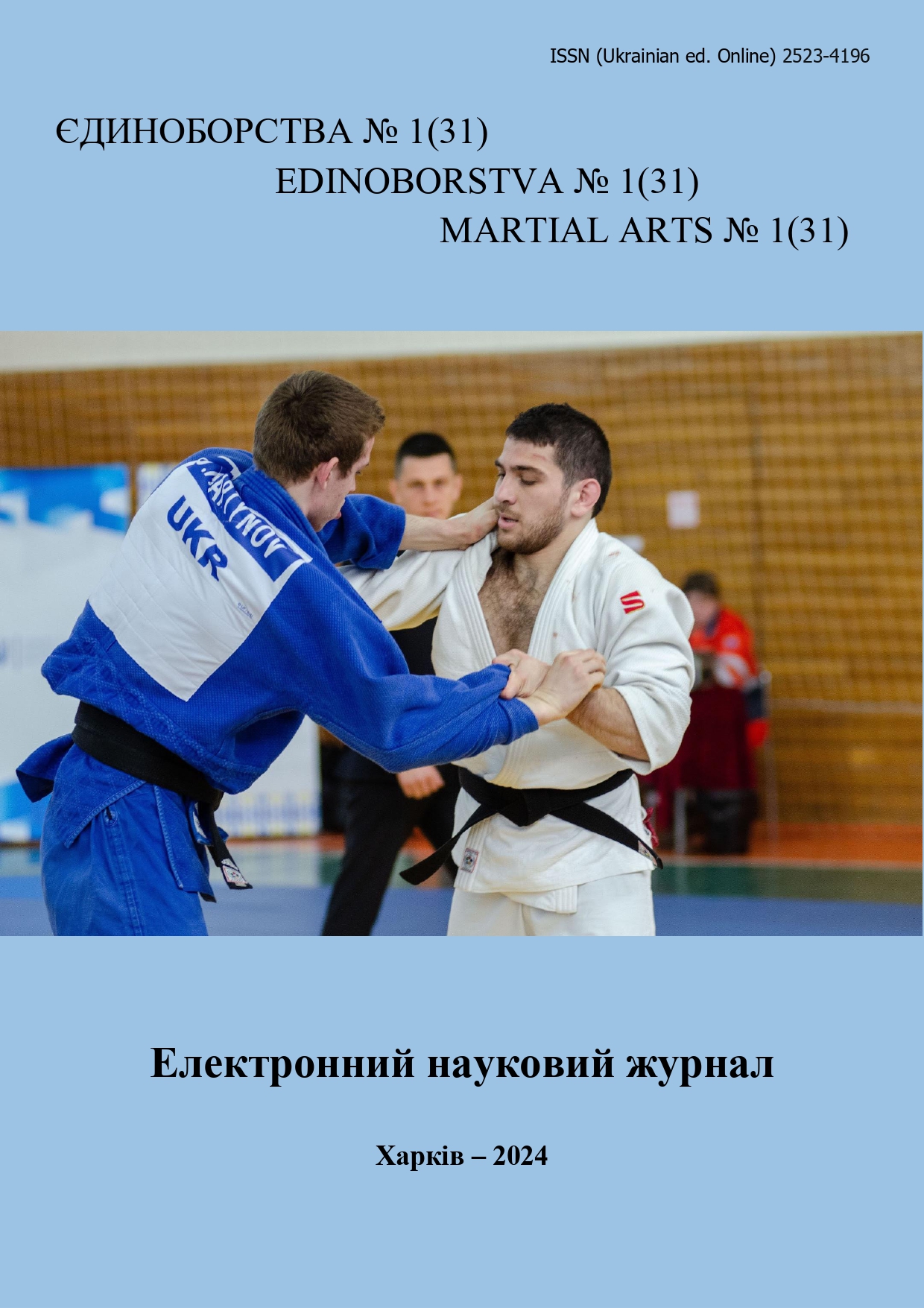