Development of computational method for matched filtration with analytical profile of the blurred digital image
DOI:
https://doi.org/10.15587/1729-4061.2022.265309Keywords:
matched filter, transfer function, OLS evaluation, Gaussian, image processingAbstract
A computational method for matched filtration with analytical profile of the blurred digital image of the investigated objects on digital frames has been developed. Such «blurred» objects can be the result of an involuntary shift of a fixed camera, an incorrect choice of the mode of guiding the telescope (diurnal or object tracking) or a failure of the diurnal tracking.
This computational method is based on the analytical selection of the typical form of the object’s image, as well as on the choice of special parameters for the transfer function of the matched filter for the blurred digital image, which makes it possible to evaluate the required parameters of the blurred digital image.
In addition, determining the number of Gaussians of the object’s image makes it possible to perform the most accurate assessment of the initial approximation of the parameters of their shape. Thus, matched filtration makes it possible to highlight the investigated objects with a blurred image of a typical shape against the background of substrate noise. Using the computational method of matched filtration makes it possible to improve the segmentation of images of reference objects on the frame and reduce the number of false detections.
The developed computational method for matched filtration with analytical profile of the blurred digital image of the investigated objects on the frames was tested in practice as part of the research of the CoLiTec project. It was implemented in the intraframe processing unit of the Lemur software for the operational automated detection of new and observation of known objects with a weak brightness. Owing to the Lemur software using and the proposed computational method introduced into it, more than 500,000 measurements of the various investigated objects were successfully processed and identified.
References
- David, P. S., Miller, P. L. (2014). Defending Against Asteroids and Comets. Handbook of Cosmic Hazards and Planetary Defense. Springer. doi: https://doi.org/10.1007/978-3-319-03952-7_59
- Mykhailova, L., Savanevych, V., Sokovikova, N., Bezkrovniy, M., Khlamov, S., Pogorelov, A. (2014). Method of maximum likelihood estimation of compact group objects location on CCD-frame. Eastern-European Journal of Enterprise Technologies, 5 (4 (71)), 16–22. doi: https://doi.org/10.15587/1729-4061.2014.28028
- Savanevych, V. E., Khlamov, S. V., Akhmetov, V. S., Briukhovetskyi, A. B., Vlasenko, V. P., Dikov, E. N. et. al. (2022). CoLiTecVS software for the automated reduction of photometric observations in CCD-frames. Astronomy and Computing,40, 100605. doi: https://doi.org/10.1016/j.ascom.2022.100605
- Cavuoti, S., Brescia, M., Longo, G. (2012). Data mining and knowledge discovery resources for astronomy in the Web 2.0 age. SPIE Astronomical Telescopes and Instrumentation, Software and Cyberinfrastructure for Astronomy II, 8451. doi: https://doi.org/10.1117/12.925321
- Vavilova, I., Pakuliak, L., Babyk, I., Elyiv, A., Dobrycheva, D., Melnyk, O. (2020). Surveys, Catalogues, Databases, and Archives of Astronomical Data. Knowledge Discovery in Big Data from Astronomy and Earth Observation. Elsevier, 57–102. doi: https://doi.org/10.1016/B978-0-12-819154-5.00015-1
- Zhang, Y., Zhao, Y., Cui, C. (2002). Data mining and knowledge discovery in database of astronomy. Progress in Astronomy, 20 (4).
- Khlamov, S., Savanevych, V. (2020). Big Astronomical Datasets and Discovery of New Celestial Bodies in the Solar System in Automated Mode by the CoLiTec Software. Knowledge Discovery in Big Data from Astronomy and Earth Observation, 331–345. doi: https://doi.org/10.1016/b978-0-12-819154-5.00030-8
- Khlamov, S., Savanevych, V., Briukhovetskyi, O., Oryshych, S. (2016). Development of computational method for detection of the object’s near-zero apparent motion on the series of ccd-frames. Eastern-European Journal of Enterprise Technologies, 2 (9 (80)), 41–48. doi: https://doi.org/10.15587/1729-4061.2016.65999
- Smith, G. E. (2010). The invention and early history of the CCD. Reviews of modern physics, 82 (3), 2307–2312. doi: https://doi.org/10.1103/RevModPhys.82.2307
- Kuz'min, S. Z. (2000). Tsifrovaya radiolokatsiya. Vvedenie v teoriyu. Kyiv: Izdatel'stvo KvіTs, 428.
- Klette, R. (2014). Concise computer vision. An Introduction into Theory and Algorithms. Springer, 429. doi: https://doi.org/10.1007/978-1-4471-6320-6
- Minaee, S., Boykov, Y. Y., Porikli, F., Plaza, A. J., Kehtarnavaz, N., Terzopoulos, D. (2021). Image Segmentation Using Deep Learning: A Survey. IEEE Transactions on Pattern Analysis and Machine Intelligence. doi: https://doi.org/10.1109/tpami.2021.3059968
- Akhmetov, V., Khlamov, S., Tabakova, I., Hernandez, W., Hipolito, J. I. N., Fedorov, P. (2019). New approach for pixelization of big astronomical data for machine vision purpose. IEEE 28th International Symposium on Industrial Electronics (ISIE). doi: https://doi.org/10.1109/isie.2019.8781270
- Bishop, C. M. (2013). Model-based machine learning. Philosophical Transactions of the Royal Society A: Mathematical, Physical and Engineering Sciences, 371 (1984), 20120222. doi: https://doi.org/10.1098/rsta.2012.0222
- Dadkhah, M., Lyashenko, V. V., Deineko, Z. V., Shamshirband, S., Jazi, M. D. (2019). Methodology of wavelet analysis in research of dynamics of phishing attacks. International Journal of Advanced Intelligence Paradigms, 12 (3/4), 220. doi: https://doi.org/10.1504/ijaip.2019.098561
- Steger, C., Ulrich, M., Wiedemann, C. (2018). Machine vision algorithms and applications. John Wiley & Sons, 516.
- Kobzar', A. I. (2006). Prikladnaya matematicheskaya statistika. Dlya inzhenerov i nauchnykh rabotnikov. Moscow: FIZMATLI, 816.
- Burger, W., Burge, M. (2009). Principles of digital image processing: core algorithms. Springer, 332. doi: https://doi.org/10.1007/978-1-84800-195-4
- Gonzalez, R., Woods, R. (2018). Digital image processing. Pearson.
- Rubin, B. (2015). Encyclopedia of Mathematics and its Application. Introduction to Radon transforms. With Elements of Fractional Calculus and Harmonic Analysis. Cambridge University Press, 596.
- Wang, J., Cai, D., Wen, Y. (2011). Comparison of matched filter and dechirp processing used in Linear Frequency Modulation. 2011 IEEE 2nd International Conference on Computing, Control and Industrial Engineering. doi: https://doi.org/10.1109/ccieng.2011.6008069
- Jorgensen, B. (1982). Statistical properties of the generalized inverse Gaussian distribution. Springer, 188. doi: https://doi.org/10.1007/978-1-4612-5698-4
- Lemur software. Available at: https://www.colitec.space
- Kashuba, S., Tsvetkov, M., Bazyey, N., Isaeva, E., Golovnia, V. (2018). The Simeiz plate collection of the ODESSA astronomical observatory. 11th Bulgarian-Serbian Astronomical Conference, 207–216. Available at: https://www.researchgate.net/publication/331386063_THE_SIMEIZ_PLATE_COLLECTION_OF_THE_ODESSA_ASTRONOMICAL_OBSERVATORY
- Molotov, I., Agapov, V., Kouprianov, V. et. al. (2009). ISON worldwide scientific optical network. Fifth European Conference on Space Debris. Available at: https://www.researchgate.net/publication/234251876_ISON_Worldwide_Scientific_Optical_Network
- Mingmuang, Y., Tummuangpak, P., Asanok, K., Jaroenjittichai, P. (2019). The mass distribution and the rotation curve of the Milky Way Galaxy using NARIT 4.5 m small radio telescope and the 2.3 m Onsala radio telescope. Journal of Physics: Conference Series, 1380 (1), 012028. doi: https://doi.org/10.1088/1742-6596/1380/1/012028
- Rao, K. R., Kim, D. N., Hwang, J.-J. (2010). Fast Fourier Transform - Algorithms and Applications. Springer, 426. doi: https://doi.org/10.1007/978-1-4020-6629-0
- Haynal, S., Haynal, H. (2011). Generating and searching families of FFT algorithms. Journal on Satisfiability, Boolean Modeling and Computation, 7 (4), 145–187. doi: https://doi.org/10.3233/sat190084
- Ivanov, M. T., Sergienko, A. B., Ushakov, V. N. (2021). Radiotekhnicheskie tsepi i signaly. Sankt-Peterburg: Piter, 336.
- Belov, L. A. (2021). Radioelektronika. Formirovanie stabil'nykh chastot i signalov. Moscow: Izdatel'stvo Yurayt, 268.
- Soyfer, V. A. (Ed.) (2003). Metody komp'yuternoy obrabotki izobrazheniy. Moscow: Fizmatlit, 784.
- Le, D.-H., Pham, C.-K., Nguyen, T. T. T., Bui, T. T. (2012). Parameter extraction and optimization using Levenberg-Marquardt algorithm. 2012 Fourth International Conference on Communications and Electronics (ICCE). doi: https://doi.org/10.1109/cce.2012.6315945
- Sergienko, A. B. (2011). Tsifrovaya obrabotka signalov. Sankt-Peterburg: BKhV-Peterburg, 768.
- Khlamov, S., Savanevych, V., Briukhovetskyi, O., Pohorelov, A., Vlasenko, V., Dikov, E. (2018). CoLiTec Software for the Astronomical Data Sets Processing. 2018 IEEE Second International Conference on Data Stream Mining & Processing (DSMP). doi: https://doi.org/10.1109/dsmp.2018.8478504
- Khlamov, S., Savanevych, V., Briukhovetskyi, O., Tabakova, I., Trunova, T. (2022). Data Mining of the Astronomical Images by the CoLiTec Software. CEUR Workshop Proceedings, 3171, 1043–1055. Available at: http://ceur-ws.org/Vol-3171/paper75.pdf
- Akhmetov, V., Khlamov, S., Khramtsov, V., Dmytrenko, A. (2019). Astrometric Reduction of the Wide-Field Images. Advances in Intelligent Systems and Computing, 896–909. doi: https://doi.org/10.1007/978-3-030-33695-0_58
- Akhmetov, V., Khlamov, S., Dmytrenko, A. (2018). Fast Coordinate Cross-Match Tool for Large Astronomical Catalogue. Advances in Intelligent Systems and Computing III, 3–16. doi: https://doi.org/10.1007/978-3-030-01069-0_1
- Akhmetov, V., Khlamov, S., Savanevych, V., Dikov, E. (2019). Cloud Computing Analysis of Indian ASAT Test on March 27, 2019. 2019 IEEE International Scientific-Practical Conference Problems of Infocommunications, Science and Technology (PIC S&T). doi: https://doi.org/10.1109/picst47496.2019.9061243
- Orbital height profile of MICROSAT-R. Available at: https://heavens-above.com/OrbitHeight.aspx?satid=43947&startMJD=58484.0
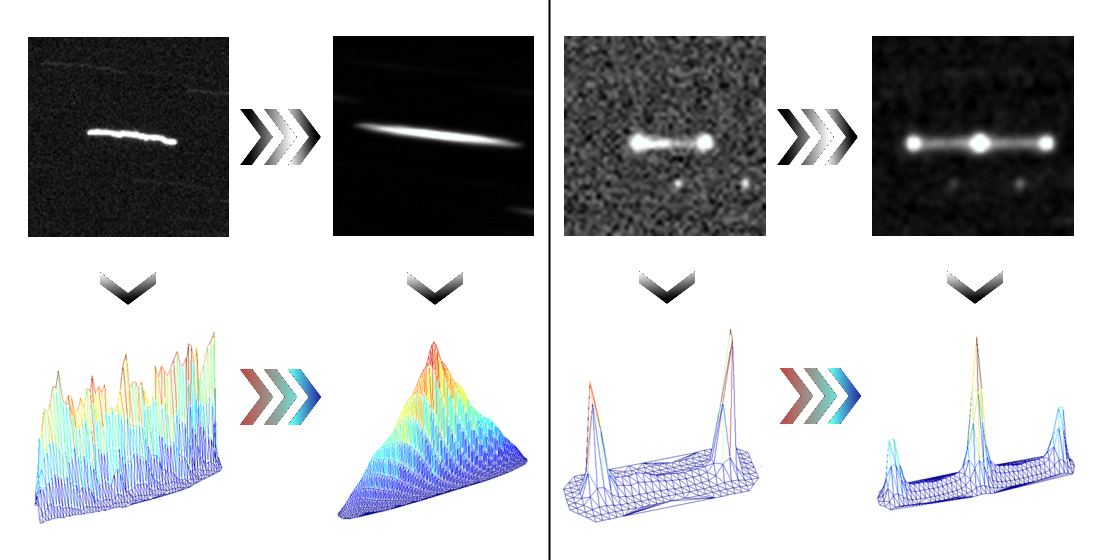
Downloads
Published
How to Cite
Issue
Section
License
Copyright (c) 2022 Sergii Khlamov, Vladimir Vlasenko, Vadym Savanevych, Oleksandr Briukhovetskyi, Tetiana Trunova, Victor Chelombitko, Iryna Tabakova

This work is licensed under a Creative Commons Attribution 4.0 International License.
The consolidation and conditions for the transfer of copyright (identification of authorship) is carried out in the License Agreement. In particular, the authors reserve the right to the authorship of their manuscript and transfer the first publication of this work to the journal under the terms of the Creative Commons CC BY license. At the same time, they have the right to conclude on their own additional agreements concerning the non-exclusive distribution of the work in the form in which it was published by this journal, but provided that the link to the first publication of the article in this journal is preserved.
A license agreement is a document in which the author warrants that he/she owns all copyright for the work (manuscript, article, etc.).
The authors, signing the License Agreement with TECHNOLOGY CENTER PC, have all rights to the further use of their work, provided that they link to our edition in which the work was published.
According to the terms of the License Agreement, the Publisher TECHNOLOGY CENTER PC does not take away your copyrights and receives permission from the authors to use and dissemination of the publication through the world's scientific resources (own electronic resources, scientometric databases, repositories, libraries, etc.).
In the absence of a signed License Agreement or in the absence of this agreement of identifiers allowing to identify the identity of the author, the editors have no right to work with the manuscript.
It is important to remember that there is another type of agreement between authors and publishers – when copyright is transferred from the authors to the publisher. In this case, the authors lose ownership of their work and may not use it in any way.