Development of a process complexity index of low pressure die casting for early product design evaluation
DOI:
https://doi.org/10.15587/1729-4061.2022.264984Keywords:
Design analysis, LPDC, process complexity indexAbstract
The design of a product is key for the manufacturing industry to compete in the current era. Failure to plan a product design means losing in the market and falling behind competitors. One way to comprehensively evaluate one design is by analyzing its complexity. Complexity analyzes not only clear view parameters such as geometry and process time but also the whole design parameters, including its production process. This paper develops a process complexity index of low pressure die casting. A casting process is one unique process that depends on the melting and solidification of material in a die. A complexity analysis of low pressure die casting is yet to be done. Three different cylinder heads fabricated with low pressure die casting were used in the case study with the product’s types of 3SZ, 1TR, and 2TR. A process complexity analysis is performed based on the LPDC process’s physical and non physical parameters. The physical parameters are fixtures, tools, gauges, and machines. The non physical parameters are determined from the features and specifications of the low pressure die casting subprocess: setting, filling, solidification, and handling. The analysis successfully defines the complexity of each product, with 1TR having an index of 7.08, 2TR being 6.93, and 3SZ being 5.14. This developed complexity index can be utilized for early product design and cost estimation evaluation
References
- Tao, F., Qi, Q., Liu, A., & Kusiak, A. (2018). Data-driven smart manufacturing. Journal of Manufacturing Systems, 48, 157–169.
- Phuyal, S., Bista, D., & Bista, R. (2020). Challenges, opportunities and future directions of smart manufacturing: a state of art review. Sustainable Futures, 2, 100023.
- Zhong, R. Y., Xu, X., Klotz, E., & Newman, S. T. (2017). Intelligent manufacturing in the context of industry 4.0: a review. Engineering, 3(5), 616–630.
- Zhou, J., Li, P., Zhou, Y., Wang, B., Zang, J., & Meng, L. (2018). Toward new-generation intelligent manufacturing. Engineering, 4(1), 11–20.
- Budiono, H. D. S., Kiswanto, G., & Soemardi, T. P. (2014). Method and model development for manufacturing cost estimation during the early design phase related to the complexity of the machining processes. International Journal of Technology, 2, 183–192.
- Budiono, H. D. S., & Hadiwardoyo, F. A. (2021). Development of Product Complexity Index in 3D Models Using a Hybrid Feature Recognition Method with Rule-Based and Graph-Based Methods. Eastern-European Journal of Enterprise Technologies, 3(1), 111.
- Budiono, H. D. S., Nurcahyo, R., & Habiburrahman, M. (2021). Relationship between manufacturing complexity, strategy, and performance of manufacturing industries in Indonesia. Heliyon, 7(6), e07225.
- Shehab, E. M., & Abdalla, H. S. (2001). An integrated prototype system for cost-effective design. Concurrent Engineering, 9(4), 243–256.
- Asiedu, Y., & Gu, P. (1998). Product life cycle cost analysis: state of the art review. International Journal of Production Research, 36(4), 883–908.
- Dewhurst, P., & Boothroyd, G. (1988). Early cost estimating in product design. Journal of Manufacturing Systems, 7(3), 183–191.
- Kalpakjian, S. (2013). Manufacturing Engineering and Technology, McGraw Hill 7th edition.
- Niazi, A., Dai, J. S., Balabani, S., & Seneviratne, L. (2006). Product cost estimation: Technique classification and methodology review.
- Yoo, S., & Kang, N. (2021). Explainable artificial intelligence for manufacturing cost estimation and machining feature visualization. Expert Systems with Applications, 183, 115430.
- Bodendorf, F., Merkl, P., & Franke, J. (2021). Intelligent cost estimation by machine learning in supply management: A structured literature review. Computers & Industrial Engineering, 160, 107601.
- Kadir, A. Z. A., Yusof, Y., & Wahab, M. S. (2020). Additive manufacturing cost estimation models—a classification review. The International Journal of Advanced Manufacturing Technology, 107(9), 4033–4053.
- Roy, R., Souchoroukov, P., & Shehab, E. (2011). Detailed cost estimating in the automotive industry: Data and information requirements. International Journal of Production Economics, 133(2), 694–707.
- ElMaraghy, W. H., & Urbanic, R. J. (2003). Modelling of manufacturing systems complexity. CIRP Annals, 52(1), 363–366.
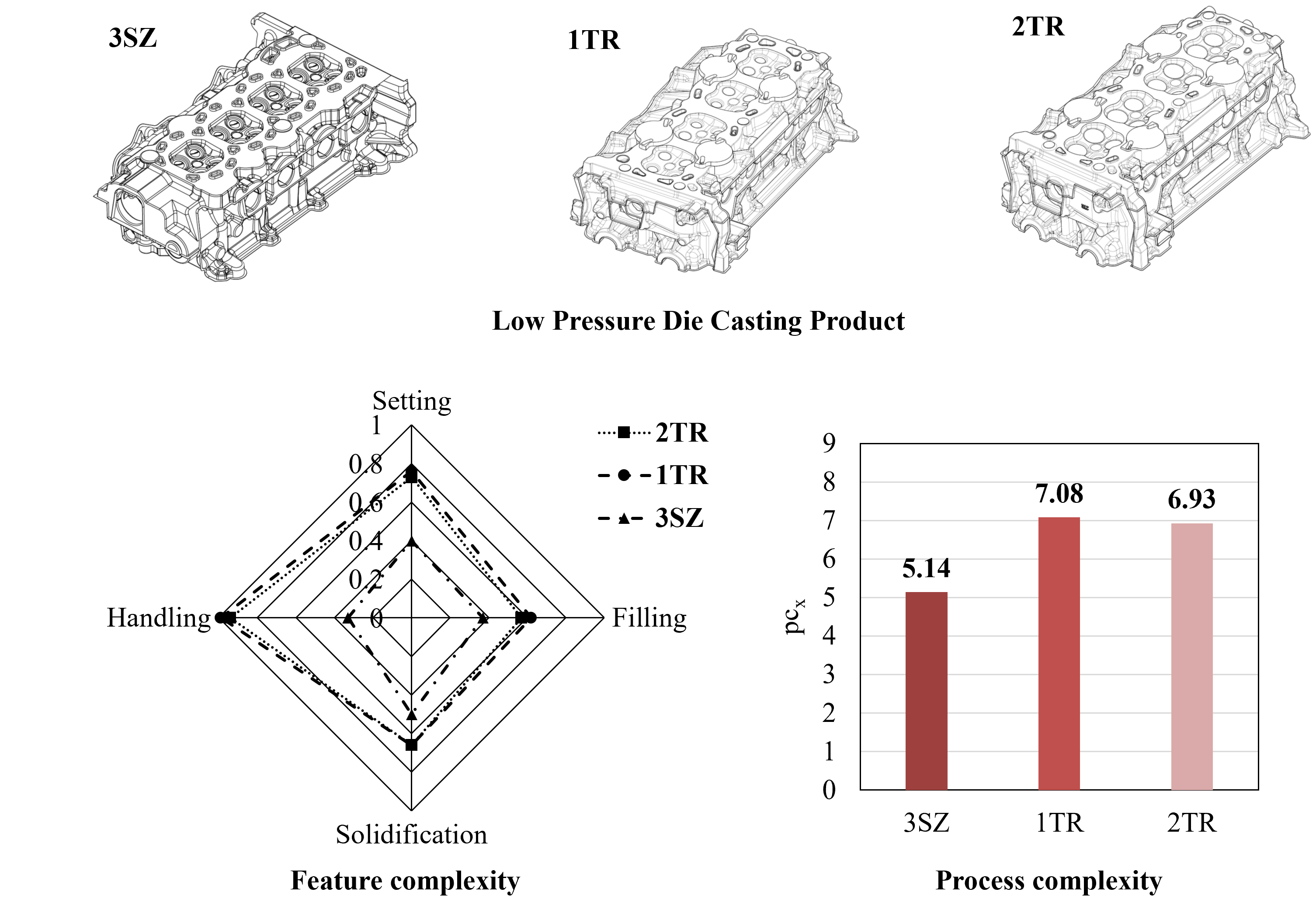
Downloads
Published
How to Cite
Issue
Section
License
Copyright (c) 2022 Hendri Dwi Saptioratri Budiono, Dian Nurdian, Mohammad Akita Indianto, Henky Suskito Nugroho

This work is licensed under a Creative Commons Attribution 4.0 International License.
The consolidation and conditions for the transfer of copyright (identification of authorship) is carried out in the License Agreement. In particular, the authors reserve the right to the authorship of their manuscript and transfer the first publication of this work to the journal under the terms of the Creative Commons CC BY license. At the same time, they have the right to conclude on their own additional agreements concerning the non-exclusive distribution of the work in the form in which it was published by this journal, but provided that the link to the first publication of the article in this journal is preserved.
A license agreement is a document in which the author warrants that he/she owns all copyright for the work (manuscript, article, etc.).
The authors, signing the License Agreement with TECHNOLOGY CENTER PC, have all rights to the further use of their work, provided that they link to our edition in which the work was published.
According to the terms of the License Agreement, the Publisher TECHNOLOGY CENTER PC does not take away your copyrights and receives permission from the authors to use and dissemination of the publication through the world's scientific resources (own electronic resources, scientometric databases, repositories, libraries, etc.).
In the absence of a signed License Agreement or in the absence of this agreement of identifiers allowing to identify the identity of the author, the editors have no right to work with the manuscript.
It is important to remember that there is another type of agreement between authors and publishers – when copyright is transferred from the authors to the publisher. In this case, the authors lose ownership of their work and may not use it in any way.