Models of adaptive integration of weighted interval data in tasks of predictive expert assessment
DOI:
https://doi.org/10.15587/1729-4061.2022.265782Keywords:
predictive assessment, complexing, alternative data, interval analysis, confidence probabilityAbstract
The problem of improving the methodological and algorithmic support of the complexing process by developing models of adaptive redundant complexing of weighted interval data has been solved. The object of this study is the process of complexing interval data obtained from several independent sources; the subject is the algebraic methods of excessive complexing of weighted interval data. The relevance of the task is due to the severity of the problem of consolidating homogeneous data in order to obtain more accurate and relevant information about the object or process under study. Models have been developed that, unlike the existing ones, make it possible a posteriori to take into account the accuracy of experts at the preliminary stage of expert evaluation. A single analytical form of the model for processing weighted interval and point estimates with the possibility of structural and parametric tuning is proposed. It allows one to increase the degree of automation of processing expert assessments under conditions of interval uncertainty. Recommendations for the practical application of the proposed models have been formulated. Options for parametric configuration of preference functions were indicated depending on the characteristics of weighted interval estimates. The commonality of the limiting cases of the proposed models with previously known ones is proved. The example shows the shift of the integrated assessment to the side of more accurate assessments at the previous stage of source assessment. The adaptability of the proposed models is illustrated. At the same time, a slight, on average, about 10 %, expansion of the complexed interval relative to the primary ones was registered. The built models and algorithms can be used in automated expert systems, as well as in cascade models of information processing and compression.
References
- Preis, T., Moat, H. S., Stanley, H. E. (2013). Quantifying Trading Behavior in Financial Markets Using Google Trends. Scientific Reports, 3 (1). doi: https://doi.org/10.1038/srep01684
- Nasseri, A. A., Tucker, A., de Cesare, S. (2015). Quantifying StockTwits semantic terms’ trading behavior in financial markets: An effective application of decision tree algorithms. Expert Systems with Applications, 42 (23), 9192–9210. doi: https://doi.org/10.1016/j.eswa.2015.08.008
- Kolanovic, M., Krishnamachari, R. T. (2017). Big data and AI strategies: Machine learning and alternative data approach to investing. Global Quantitative & Derivatives Strategy. Available at: https://cpb-us-e2.wpmucdn.com/faculty.sites.uci.edu/dist/2/51/files/2018/05/JPM-2017-MachineLearningInvestments.pdf
- Cohen, L., Malloy, C., Nguyen, Q. (2020). Lazy Prices. The Journal of Finance, 75 (3), 1371–1415. doi: https://doi.org/10.1111/jofi.12885
- Fleder, M., Shah, D. (2020). Forecasting with Alternative Data. ACM SIGMETRICS Performance Evaluation Review, 48 (1), 23–24. doi: https://doi.org/10.1145/3410048.3410062
- Charoenwong, B., Kwan, A. (2021). Alternative Data, Big Data, and Applications to Finance. Blockchain Technologies, 35–105. doi: https://doi.org/10.1007/978-981-33-6137-9_2
- Bellstam, G., Bhagat, S., Cookson, J. A. (2021). A Text-Based Analysis of Corporate Innovation. Management Science, 67 (7), 4004–4031. doi: https://doi.org/10.1287/mnsc.2020.3682
- Romanenkov, Y., Vartanian, V. (2016). Formation of prognostic software support for strategic decision-making in an organization. Eastern-European Journal of Enterprise Technologies, 2 (9 (80)), 25–34. doi: https://doi.org/10.15587/1729-4061.2016.66306
- Вuravtsev, A. V. (2017). Grey management analysis. Perspectives of Science & Education, 5 (29), 74–79. Available at: https://cyberleninka.ru/article/n/seryy-upravlencheskiy-analiz
- Chan, J. W. K., Tong, T. K. L. (2007). Multi-criteria material selections and end-of-life product strategy: Grey relational analysis approach. Materials & Design, 28 (5), 1539–1546. doi: https://doi.org/10.1016/j.matdes.2006.02.016
- Shostak, I. V., Danova, M. A., Romanenkov, Yu. A. (2015). Informatsionnaya tekhnologiya podderzhki prinyatiya ekspertnykh resheniy v natsional'nykh Forsayt-issledovaniyakh. Komunalne hospodarstvo mist, 123, 58–67. Available at: https://www.researchgate.net/publication/315664668_Informacionnaa_tehnologia_podderzki_prinatia_ekspertnyh_resenij_v_nacionalnyh_forsajt-issledovaniah
- Durrant-Whyte, H., Henderson, T. C. (2008). Multisensor Data Fusion. Springer Handbook of Robotics, 585–610. doi: https://doi.org/10.1007/978-3-540-30301-5_26
- Hoover, A., Olsen, B. D. (1999). A real-time occupancy map from multiple video streams. Proceedings 1999 IEEE International Conference on Robotics and Automation (Cat. No.99CH36288C). doi: https://doi.org/10.1109/robot.1999.770442
- Smelyakov, K., Sandrkin, D., Ruban, I., Vitalii, M., Romanenkov, Y. (2018). Search by Image. New Search Engine Service Model. 2018 International Scientific-Practical Conference Problems of Infocommunications. Science and Technology (PIC S&T). doi: https://doi.org/10.1109/infocommst.2018.8632117
- Vartanyan, V. M., Cheranovskiy, O. V., Al Daheri Ali Mohamed (2011). Evaluation of basic model robust stability of unmanned aerial vehicles. Aviatsionno-kosmicheskaya tekhnika i tekhnologiya, 6, 44–51. Available at: http://nbuv.gov.ua/UJRN/aktit_2011_6_9
- Ruban, I., Khudov, H., Khudov, V., Khizhnyak, I., Makoveichuk, O. (2017). Segmentation of the images obtained from onboard optoelectronic surveillance systems by the evolutionary method. Eastern-European Journal of Enterprise Technologies, 5 (9 (89)), 49–57. doi: https://doi.org/10.15587/1729-4061.2017.109904
- Abdelgawad, A., Bayoumi, M. (2012). Resource-Aware Data Fusion Algorithms for Wireless Sensor Networks. Lecture Notes in Electrical Engineering. doi: https://doi.org/10.1007/978-1-4614-1350-9
- Khaleghi, B., Khamis, A., Karray, F. O., Razavi, S. N. (2013). Multisensor data fusion: A review of the state-of-the-art. Information Fusion, 14 (1), 28–44. doi: https://doi.org/10.1016/j.inffus.2011.08.001
- Sineglazov, V. M., Chumachenko, E. I., Gorbatyuk, V. S. (2012). Metod resheniya zadachi prognozirovaniya na osnove kompleksirovaniya otsenok. Induktyvne modeliuvannia skladnykh system, 4, 214–223.
- Bidyuk, P. I., Gasanov, A. S., Vavilov, S. E. (2013). Analiz kachestva otsenok prognozov s ispol'zovaniem metoda kompleksirovaniya. Systemni doslidzhennia ta informatsiyni tekhnolohiyi, 4, 7–16. Available at: http://nbuv.gov.ua/UJRN/sdtit_2013_4_3
- Vasil'ev, A. A. (2015). Obedinenie prognozov ekonomicheskikh pokazateley na osnove bives-otsenki s vesovoy funktsiey Kh'yubera. Aktual'nye problemy gumanitarnykh i estestvennykh nauk, 10-4, 44–47. Available at: https://cyberleninka.ru/article/n/obedinenie-prognozov-ekonomicheskih-pokazateley-na-osnove-bives-otsenki-s-vesovoy-funktsiey-hyubera
- Molev, M. D., Zanina, I. A., Stuzhenko, N. I. (2013). Sintez prognoznoy informatsii v praktike otsenki ekologo-ekonomicheskogo razvitiya regiona. Inzhenerniy vestnik Dona, 4, 59. Available at: https://cyberleninka.ru/article/n/cintez-prognoznoy-informatsii-v-praktike-otsenki-ekologo-ekonomicheskogo-razvitiya-regiona
- Romanenkov, Yu. A., Vartanyan, V. M., Revenko, D. S. (2014). Kompleksirovanie prognoznykh otsenok v sisteme monitoringa pokazateley sostoyaniya biznes-protsessa. Systemy upravlinnia, navihatsiyi ta zviazku, 2 (30). Available at: https://www.researchgate.net/publication/315693915_Kompleksirovanie_prognoznyh_ocenok_v_sisteme_monitoringa_pokazatelej_sostoania_biznes-processa
- Kovalenko, I., Shved, A. (2018). Development of a technology of structuring group expert judgments under various types of uncertainty. Eastern-European Journal of Enterprise Technologies, 3 (4 (93)), 60–68. doi: https://doi.org/10.15587/1729-4061.2018.133299
- Sternin, M. Yu., Shepelev, G. I. (2009). Sravnenie poliinterval'nykh otsenok v metode OIO. Intelligent Support of Decision Making. International book series «Information science & computing, 83–88. Available at: http://foibg.com/ibs_isc/ibs-10/ibs-10-p11.pdf
- Shepelev, G., Sternin, M. (2014). Evaluating Expected Effectiveness of Interval Alternatives. International Journal “Information Theories and Applications”, 21 (3), 263–274. Available at: http://www.foibg.com/ijita/vol21/ijita21-03-p06.pdf
- Baohua Li, Yunmin Zhu, Rong Li, X. (2002). Fault-tolerant interval estimation fusion by Dempster-Shafer theory. Proceedings of the Fifth International Conference on Information Fusion. FUSION 2002. (IEEE Cat.No.02EX5997). doi: https://doi.org/10.1109/icif.2002.1021010
- Liu, H., Wang, B., Liu, Z., Zhou, Y. (2009). Data Fusion Based on Interval Dempster-Shafer Theory for Emitter Platform Identification. 2009 International Conference on Information Engineering and Computer Science. doi: https://doi.org/10.1109/iciecs.2009.5364227
- Liang, H., Li, C.-C., Dong, Y., Jiang, Y. (2016). The fusion process of interval opinions based on the dynamic bounded confidence. Information Fusion, 29, 112–119. doi: https://doi.org/10.1016/j.inffus.2015.08.010
- Zhu, Y., Li, B. (2006). Optimal interval estimation fusion based on sensor interval estimates with confidence degrees. Automatica, 42 (1), 101–108. doi: https://doi.org/10.1016/j.automatica.2005.07.008
- Ramalingam, S., Maheswari, U. (2016). A fuzzy interval valued fusion technique for multi-modal 3D face recognition. 2016 IEEE International Carnahan Conference on Security Technology (ICCST). doi: https://doi.org/10.1109/ccst.2016.7815709
- Carlson, R. J. (2013). Voter compatibility in interval societies. HMC Senior Theses. Available at: https://scholarship.claremont.edu/cgi/viewcontent.cgi?article=1044&context=hmc_theses
- Collett, M. A., Cox, M. G., Esward, T. J., Harris, P. M., Sousa, J. A. (2007). Aggregating measurement data influenced by common effects. Metrologia, 44 (5), 308–318. doi: https://doi.org/10.1088/0026-1394/44/5/007
- Duta, M., Henry, M. (2005). The fusion of redundant SEVA measurements. IEEE Transactions on Control Systems Technology, 13 (2), 173–184. doi: https://doi.org/10.1109/tcst.2004.840448
- Dopazo, E., Martínez-Céspedes, M. L. (2017). Rank aggregation methods dealing with ordinal uncertain preferences. Expert Systems with Applications, 78, 103–109. doi: https://doi.org/10.1016/j.eswa.2017.01.051
- Voschinin, A. P. (2002). Interval'niy analiz dannykh: razvitie i perspektivy. Zavodskaya Laboratoriya, 68 (1), 118–126.
- Orlov, A. I. (2013). Osnovnye idei statistiki interval'nykh dannykh. Nauchniy zhurnal KubGAU, 94, 55–70. Available at: https://cyberleninka.ru/article/n/osnovnye-idei-statistiki-intervalnyh-dannyh
- Alefel'd, G., Khertsberger, Yu. (1987). Vvedenie v interval'nye vychisleniya. Moscow: Mir, 360.
- Kalmykov, S. A., Shokin, Yu. I., Yuldashev, Z. Kh. (1986). Metody interval'nogo analiza. Novosibirsk: Nauka, 223. Available at: https://hi.b-ok.xyz/book/445084/5ae6b7
- Shokin, Yu. I. (1981). Interval'niy analiz. Novosibirsk: Nauka, 112. Available at: https://read.in.ua/book156021/
- Shariy, S. P. (2016). Konechnomerniy interval'niy analiz. Novosibirsk: XYZ, 606.
- Hansen, E. R. (1993). Computing zeros of functions using generalized interval arithmetic. Interval Computations, 3, 3–27. Available at: https://interval.louisiana.edu/reliable-computing-journal/1993/interval-computations-1993-3-pp-003-028.pdf
- Moore, R. E. (1979). Methods and Applications of Interval Analysis. Philadelphia: SIAM, 125. doi: https://doi.org/10.1137/1.9781611970906
- Kaucher, E. (1980). Interval analysis in the extended interval spase IR. Computing Suppl, 2, 33–49. Available at: http://www.math.bas.bg/~epopova/Kaucher-80-CS_33-49.pdf
- Bardachev, Yu. N., Kryuchkovskiy, V. V., Malomuzh, T. V. (2010). Metodologicheskaya predpochtitel'nost' interval'nykh ekspertnykh otsenok pri prinyatii resheniy v usloviyakh neopredelennosti. Visnyk Kharkivskoho natsionalnoho universytetu imeni V. N. Karazina. Seriya: Matematychne modeliuvannia. Informatsiyni tekhnolohiyi. Avtomatyzovani systemy upravlinnia, 890, 18–28. Available at: http://mia.univer.kharkov.ua/13/30045.pdf
- Romanenkov, Y., Danova, M., Kashcheyeva, V., Bugaienko, O., Volk, M., Karminska-Bielobrova, M., Lobach, O. (2018). Complexification methods of interval forecast estimates in the problems on shortterm prediction. Eastern-European Journal of Enterprise Technologies, 3 (3 (93)), 50–58. doi: https://doi.org/10.15587/1729-4061.2018.131939
- Podruzhko, A. A., Podruzhko, A. S., Kiritsev, P. N. (2009). Interval'nye metody resheniya zadach kalibrovki i klassifikatsii. Trudy Instituta sistemnogo analiza Rossiyskoy Akademii nauk. Dinamika neodnorodnykh sistem, 44, 173–186.
- Kochkarov, R. A. (2015). Interval'nye zadachi na predfraktal'nykh grafakh. Novye informatsionnye tekhnologii v avtomatizirovannykh sistemakh, 18, 255–264. Available at: https://cyberleninka.ru/article/n/intervalnye-zadachi-na-predfraktalnyh-grafah
- Marzullo, K. (1990). Tolerating failures of continuous-valued sensors. ACM Transactions on Computer Systems, 8 (4), 284–304. doi: https://doi.org/10.1145/128733.128735
- Muravyov, S. V., Khudonogova, L. I., Emelyanova, E. Y. (2018). Interval data fusion with preference aggregation. Measurement, 116, 621–630. doi: https://doi.org/10.1016/j.measurement.2017.08.045
- Khudonogova, L. I., Muravyov, S. V. (2021). Interval Data Fusion with Preference Aggregation for Balancing Measurement Accuracy and Energy Consumption in WSN. Wireless Personal Communications, 118 (4), 2399–2421. doi: https://doi.org/10.1007/s11277-021-08132-9
- Levin, V. I. (2004). Uporyadochenie intervalov i zadachi optimizatsii s interval'nymi parametrami. Kibernetika i sistemnyy analiz, 3, 14–24. Available at: http://ec.lib.vntu.edu.ua/DocDescription?doc_id=112446
- Levin, V. I. (2002). Intervals comparison and optimisation under the conditions of uncertainty. Vestnik Tambovskogo universiteta. Seriya: Estestvennye i tekhnicheskie nauki, 7 (3), 383–389. Available at: https://cyberleninka.ru/article/n/sravnenie-intervalov-i-optimizatsiya-v-usloviyah-neopredelennosti
- Romanenkov, Yu., Kosenko, V., Lobach, O., Grinchenko, E., Grinchenko, M. (2019). The Method for Ranking Quasi-Optimal Alternatives in Interval Game Models Against Nature. Proceedings of the 3rd International Conference on Computational Linguistics and Intelligent Systems (COLINS-2019). Available at: https://www.researchgate.net/publication/333488747_The_Method_for_Ranking_Quasi-Optimal_Alternatives_in_Interval_Game_Models_Against_Nature
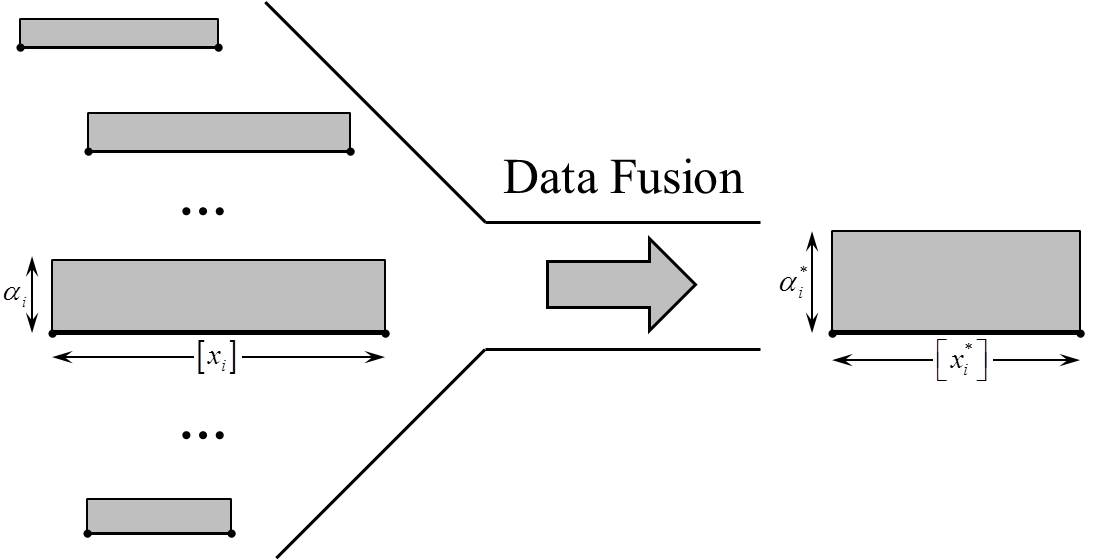
Downloads
Published
How to Cite
Issue
Section
License
Copyright (c) 2022 Igor Ruban, Hennadii Horenskyi, Yuri Romanenkov, Daniil Revenko

This work is licensed under a Creative Commons Attribution 4.0 International License.
The consolidation and conditions for the transfer of copyright (identification of authorship) is carried out in the License Agreement. In particular, the authors reserve the right to the authorship of their manuscript and transfer the first publication of this work to the journal under the terms of the Creative Commons CC BY license. At the same time, they have the right to conclude on their own additional agreements concerning the non-exclusive distribution of the work in the form in which it was published by this journal, but provided that the link to the first publication of the article in this journal is preserved.
A license agreement is a document in which the author warrants that he/she owns all copyright for the work (manuscript, article, etc.).
The authors, signing the License Agreement with TECHNOLOGY CENTER PC, have all rights to the further use of their work, provided that they link to our edition in which the work was published.
According to the terms of the License Agreement, the Publisher TECHNOLOGY CENTER PC does not take away your copyrights and receives permission from the authors to use and dissemination of the publication through the world's scientific resources (own electronic resources, scientometric databases, repositories, libraries, etc.).
In the absence of a signed License Agreement or in the absence of this agreement of identifiers allowing to identify the identity of the author, the editors have no right to work with the manuscript.
It is important to remember that there is another type of agreement between authors and publishers – when copyright is transferred from the authors to the publisher. In this case, the authors lose ownership of their work and may not use it in any way.