Building an information analysis system within a corporate information system for combining and structuring organization data (on the example of a university)
DOI:
https://doi.org/10.15587/1729-4061.2022.267893Keywords:
information analysis system, business intelligence systems, production rules, identifying hidden dependencies, PowerBI, data structuring, data analysis, university information systemAbstract
Digitalization of all spheres of life has led to the fact that organizations store a large amount of information in various data sources. The process of strategic decision-making may involve an in-depth analysis of data on many items of the organization's production cycle. However, data collection in this case can take weeks. This is quite a long time for prompt decision-making.
The object of the study is data stored in the corporate information system of the organization, methods of their analysis for making management decisions.
The subject of the study is the automation of work with data within the corporate analytical system, the identification of data analysis patterns, as well as the design of an information analysis system of a university.
The presented information analysis system will solve the problem of consolidating disparate data of corporate information systems, as well as operational data of the organization. This is ensured by the creation of a metadatabase and the formation of an information analysis system add-on using PowerBI technologies. The generally accepted design scheme of the information system was modernized demonstrating the place of the metadatabase within the corporate information system of the university. A model of data analysis based on the formation of production rules for building a decision tree on the example of human resources analysis is presented.
The results of this study can be useful to analysts, executives and senior managers of large organizations in creating an analysis system for the organization's performance
References
- Kopnova, O. L. (2021). Use of the BI systems for organising the information space of the university. International Journal of Business Intelligence and Data Mining, 19 (1), 80. doi: https://doi.org/10.1504/ijbidm.2021.116040
- Mutanov, G., Mamykova, Z., Kopnova, O., Bolatkhan, M. (2020). Applied research of data management in the education system for decision-making on the example of Al-Farabi Kazakh National University. E3S Web of Conferences, 159, 09003. doi: https://doi.org/10.1051/e3sconf/202015909003
- Kolyeva, N., Kopnova, O., Shaporeva, A. (2021). Adaptation Information and analytical system adaptation in the contour of the corporate system of the university. E3S Web of Conferences, 270, 01037. doi: https://doi.org/10.1051/e3sconf/202127001037
- Shaporeva, A., Kopnova, O., Shmigirilova, I., Kukharenko, Y., Aitymova, A. (2022). Development of comprehensive decision support tools in distance learning quality management processes. Eastern-European Journal of Enterprise Technologies, 4 (3 (118)), 43–50. doi: https://doi.org/10.15587/1729-4061.2022.263285
- Aitymova, A., Shaporeva, A., Kopnova, O., Kushumbayev, A., Aitymov, Z. (2022). Development and modeling of combined components of the information environment. Eastern-European Journal of Enterprise Technologies, 2 (2 (116)), 51–60. doi: https://doi.org/10.15587/1729-4061.2022.255084
- Sarma, A. D. N. (2021). The five key components for building an operational business intelligence ecosystem. International Journal of Business Intelligence and Data Mining, 19 (3), 343. doi: https://doi.org/10.1504/ijbidm.2021.118191
- Duarte, R., Guimarães, T., Santos, M. F. (2022). A Business Intelligence Platform for Portuguese Misericórdias. Procedia Computer Science, 198, 341–346. doi: https://doi.org/10.1016/j.procs.2021.12.251
- Jantakoon, T., Wannapiroon, P. (2017). System Architecture of Business Intelligence to AUN-QA Framework for Higher Education Institution. Turkish Online Journal of Educational Technology, 2. Available at: https://www.researchgate.net/publication/321796638_System_Architecture_of_Business_Intelligence_to_AUN-QA_Framework_for_Higher_Education_Institution
- Halaweh, M., El Massry, A. (2018). A Synergetic Model for Implementing Big Data in Organizations. Operations and Service Management, 281–298. doi: https://doi.org/10.4018/978-1-5225-3909-4.ch014
- Shokin, Yu. I., Yurchenko, A. V. (2019). Models of organizing research data storage and usage: basic principles, processes and implementation mechanisms. Information and Control Systems, 3, 45–54. doi: https://doi.org/10.31799/1684-8853-2019-3-45-54
- Maisel, L., Cokins, G. (2015). Why Analytics Will Be the Next Competitive Edge. Journal of Corporate Accounting & Finance, 26 (4), 91–100. doi: https://doi.org/10.1002/jcaf.22054
- Sooriamurthi, R. (2018). Introducing big data analytics in high school and college. Proceedings of the 23rd Annual ACM Conference on Innovation and Technology in Computer Science Education. doi: https://doi.org/10.1145/3197091.3205834
- Ramos, J., Alturas, B., Moro, S. (2017). Business intelligence in a public institution – Evaluation of a financial data mart. 2017 12th Iberian Conference on Information Systems and Technologies (CISTI). doi: https://doi.org/10.23919/cisti.2017.7975672
- Wang, Y., Kung, L., Byrd, T. A. (2018). Big data analytics: Understanding its capabilities and potential benefits for healthcare organizations. Technological Forecasting and Social Change, 126, 3–13. doi: https://doi.org/10.1016/j.techfore.2015.12.019
- Adnan, K., Akbar, R. (2019). An analytical study of information extraction from unstructured and multidimensional big data. Journal of Big Data, 6 (1). doi: https://doi.org/10.1186/s40537-019-0254-8
- Yuan, Y., Li, H., Wang, Q. (2019). Spatiotemporal Modeling for Video Summarization Using Convolutional Recurrent Neural Network. IEEE Access, 7, 64676–64685. doi: https://doi.org/10.1109/access.2019.2916989
- Maddumala, V. R., R, A. (2020). A Weight Based Feature Extraction Model on Multifaceted Multimedia Bigdata Using Convolutional Neural Network. Ingénierie Des Systèmes d Information, 25 (6), 729–735. doi: https://doi.org/10.18280/isi.250603
- Cui, Z., Xu, C., Zheng, W., Yang, J. (2018). Context-Dependent Diffusion Network for Visual Relationship Detection. Proceedings of the 26th ACM International Conference on Multimedia. doi: https://doi.org/10.1145/3240508.3240668
- Ruvinskaya, V., Troynina, A. (2017). Development of information technology for the generation and maintenance of knowledge–oriented control systems. Eastern-European Journal of Enterprise Technologies, 2 (2 (86)), 41–49. doi: https://doi.org/10.15587/1729-4061.2017.98727
- Sharma, S., Chen, K., Sheth, A. (2018). Toward Practical Privacy-Preserving Analytics for IoT and Cloud-Based Healthcare Systems. IEEE Internet Computing, 22 (2), 42–51. doi: https://doi.org/10.1109/mic.2018.112102519
- Goutham, V., Ramamurthy, A. (2018). Cloud based building confidential and efficient query services. Journal of Advanced Research in Dynamical and Control Systems, 10 (7), 744–749.
- Xu, H., Guo, S., Chen, K. (2014). Building Confidential and Efficient Query Services in the Cloud with RASP Data Perturbation. IEEE Transactions on Knowledge and Data Engineering, 26 (2), 322–335. doi: https://doi.org/10.1109/tkde.2012.251
- Ramamurthy, A., Goutham, V. (2019). Efficient System Performance for Data Replication in Cloud Computing. International Journal of Engineering and Advanced Technology, 8 (3), 540–544. Available at: https://www.ijeat.org/wp-content/uploads/papers/v8i3/C5979028319.pdf
- Krishna Kishore, S., Murali, G., Chandra Mouli, A. (2018). Building Confidential and Efficient Query Services in the Cloud with RASP Data Perturbation. International Journal of Engineering & Technology, 7 (3.27), 466. doi: https://doi.org/10.14419/ijet.v7i3.27.17998
- Qureshi, T. M. (2020). HR Analytics, Fad or Fashion for Organizational Sustainability. Advances in Science, Technology & Innovation, 103–107. doi: https://doi.org/10.1007/978-3-030-32922-8_9
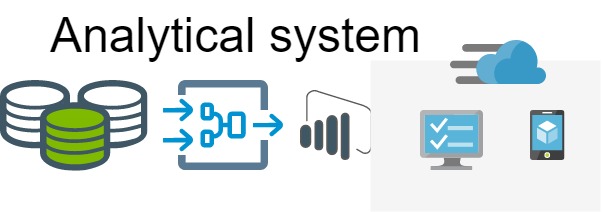
Downloads
Published
How to Cite
Issue
Section
License
Copyright (c) 2022 Oxana Kopnova, Anna Shaporeva, Kainizhamal Iklassova, Agibay Kushumbayev, Askar Tadzhigitov, Aliya Aitymova

This work is licensed under a Creative Commons Attribution 4.0 International License.
The consolidation and conditions for the transfer of copyright (identification of authorship) is carried out in the License Agreement. In particular, the authors reserve the right to the authorship of their manuscript and transfer the first publication of this work to the journal under the terms of the Creative Commons CC BY license. At the same time, they have the right to conclude on their own additional agreements concerning the non-exclusive distribution of the work in the form in which it was published by this journal, but provided that the link to the first publication of the article in this journal is preserved.
A license agreement is a document in which the author warrants that he/she owns all copyright for the work (manuscript, article, etc.).
The authors, signing the License Agreement with TECHNOLOGY CENTER PC, have all rights to the further use of their work, provided that they link to our edition in which the work was published.
According to the terms of the License Agreement, the Publisher TECHNOLOGY CENTER PC does not take away your copyrights and receives permission from the authors to use and dissemination of the publication through the world's scientific resources (own electronic resources, scientometric databases, repositories, libraries, etc.).
In the absence of a signed License Agreement or in the absence of this agreement of identifiers allowing to identify the identity of the author, the editors have no right to work with the manuscript.
It is important to remember that there is another type of agreement between authors and publishers – when copyright is transferred from the authors to the publisher. In this case, the authors lose ownership of their work and may not use it in any way.