Applying a neural network method to search for optimal air ionization conditions
DOI:
https://doi.org/10.15587/1729-4061.2022.270315Keywords:
air ionization, air quality, neural network, production facilities, air indicatorsAbstract
This paper reports measuring, modeling, and determining the optimized air ionic composition of the air at industrial premises to ensure safe living and working conditions for workers.
The possibility of using saline solutions with different degrees of concentration to increase the number of negative ions in the airspace, as well as the variability of the air flow rate for the process of ionization of the air of industrial premises, has been investigated. Analysis of experimental data revealed that an increase in the concentration of saline solutions leads to a decrease in the release of the number of air ions into the vapor-air space of the room.
It is proved that in order to improve air quality, it is advisable to enable air ionization using an ultrasonic air ion generator and the use of demineralized water. The optimal input parameters established for the ultrasonic installation are: s –distance to the ultrasonic installation, 40 cm; v ‒ airflow rate, 6.00 m/s; and c ‒ concentration of salt water solution, 3.3 %.
The result reported here could be used in the design and development of a control system for an ultrasonic generator of air ions of ventilation systems and microclimate systems in order to create the most comfortable high-quality ionized air at industrial premises.
To find the optimal mode of operation of the ionization process, a representation procedure for a neural network was applied, which was most accurate to determine the optimal parameters for ionizing the airspace of the working room.
Optimization was performed using a Feed Forward Bottle Neck Neural Network (FFBN NN) representation. This approach allows one to determine several optimal conditions for the process under study on the basis of a compromise solution.
References
- Standard of Building Biology Testing Methods: SBM-2015. BAUBIOLOGIE MAES / Institut für Baubiologie + Nachhaltigkeit IBN. Available at: https://buildingbiology.com/site/wp-content/uploads/standard-2015-englisch.pdf
- Wang, H., Wang, B., Niu, X., Song, Q., Li, M., Luo, Y. et al. (2020). Study on the change of negative air ion concentration and its influencing factors at different spatio-temporal scales. Global Ecology and Conservation, 23, e01008. doi: https://doi.org/10.1016/j.gecco.2020.e01008
- Yue, C., Yuxin, Z., Nan, Z., Dongyou, Z., Jiangning, Y. (2020). An inversion model for estimating the negative air ion concentration using MODIS images of the Daxing’anling region. PLOS ONE, 15 (11), e0242554. doi: https://doi.org/10.1371/journal.pone.0242554
- Kaiser, F.-J., Bassler, N., Tölli, H., Jäkel, O. (2011). Initial recombination in the track of heavy charged particles: Numerical solution for air filled ionization chambers. Acta Oncologica, 51 (3), 368–375. doi: https://doi.org/10.3109/0284186x.2011.626452
- Rubenstone, J. (2021). Cleaning the Air, Fighting The Virus. ENR: Engineering News-Record, 286 (8), 8–11.
- Wei, W., Ramalho, O., Malingre, L., Sivanantham, S., Little, J. C., Mandin, C. (2019). Machine learning and statistical models for predicting indoor air quality. Indoor Air, 29 (5), 704–726. doi: https://doi.org/10.1111/ina.12580
- Sukach, S., Kozlovs’ka, T., Serhiienko, I., Khodakovskyy, O., Liashok, I., Kipko, O. (2018). Studying and substantiation of the method for normalization of airionic regime at industrial premises at the ultrasonic ionization of air. Eastern-European Journal of Enterprise Technologies, 4 (10 (94)), 36–45. doi: https://doi.org/10.15587/1729-4061.2018.141060
- Noakes, C. J., Sleigh, P. A., Beggs, C. B. (2007). Modelling the air cleaning performance of negative air ionisers in ventilated rooms. Proceeding of the 10 th Int. Conference on Air Distribution in Rooms (Roomvent 2007). Helsinki.
- Sydorov, O. V., Hlyva, V. A. (2013). Vplyv elektrostatychnykh poliv na kontsentratsiyi lehkykh aeroioniv na robochomu mistsi operatora PEOM. Stroitel'stvo, materialovedenie, mashinostroenie. Seriya: Bezopasnost' zhiznedeyatel'nosti, 71 (2), 176–183. Available at: https://dspace.nau.edu.ua/handle/NAU/29609
- Biliaiev, M. M., Tsygankova, S. G. (2016). Complex of numerical models for computation of air ion concentration in premises. Science and Transport Progress. Bulletin of Dnipropetrovsk National University of Railway Transport, 2 (62), 16–24. doi: https://doi.org/10.15802/stp2016/67281
- Rusakova, T. I. (2019). Method for predicting parameters of the aeroionic mode in open terrain ground areas. Science and Transport Progress. Bulletin of Dnipropetrovsk National University of Railway Transport, 3 (81), 16–26. doi: https://doi.org/10.15802/stp2019/170273
- Podorozhniak, A., Liubchenko, N., Kvochka, M., Suarez, I. (2021). Usage of intelligent methods for multispectral data processing in the field of environmental monitoring. Advanced Information Systems, 5 (3), 97–102. doi: https://doi.org/10.20998/2522-9052.2021.3.13
- Sobchuk, V., Zamrii, I., Olimpiyeva, Y., Laptiev, S. (2021). Functional stability of technological processes based on nonlinear dynamics with the application of neural networks. Advanced Information Systems, 5 (2), 49–57. doi: https://doi.org/10.20998/2522-9052.2021.2.08
- Sukach, S. V., Sydorov, O. V. (2016). Metodolohichni zasady pidvyshchennia yakosti kontroliu aeroionnoho skladu povitria vyrobnychoho seredovyshcha. Problemy okhorony pratsi v Ukraini, 32, 127–133.
- Daszykowski, M. (2003). A journey into low-dimensional spaces with autoassociative neural networks. Talanta, 59 (6), 1095–1105. doi: https://doi.org/10.1016/s0039-9140(03)00018-3
- Eriksson, L., Johansson, E., Kettaneh-Wold, N., Wikström, C., Wold, S. (2008). Design of Experiments: Principles and Applications. Umetrics AB, Umeе.
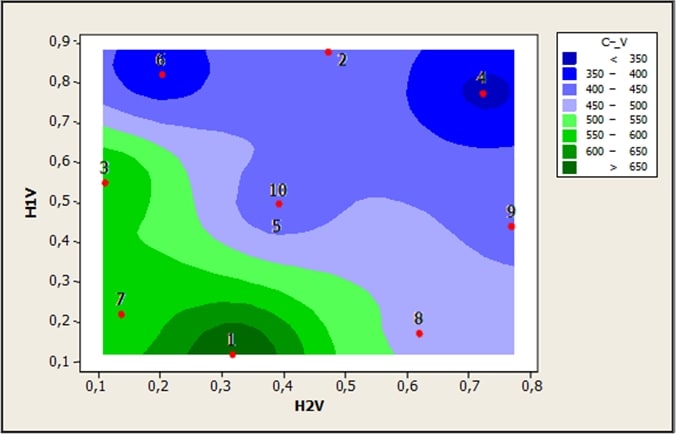
Downloads
Published
How to Cite
Issue
Section
License
Copyright (c) 2022 Serhii Sukach, Volodymyr Chenchevoi, Natalja Fjodorova, Olga Chencheva, Volodymyr Bakharev, Olena Kortsova, Volodymyr Shevchenko, Ivan Petrenko

This work is licensed under a Creative Commons Attribution 4.0 International License.
The consolidation and conditions for the transfer of copyright (identification of authorship) is carried out in the License Agreement. In particular, the authors reserve the right to the authorship of their manuscript and transfer the first publication of this work to the journal under the terms of the Creative Commons CC BY license. At the same time, they have the right to conclude on their own additional agreements concerning the non-exclusive distribution of the work in the form in which it was published by this journal, but provided that the link to the first publication of the article in this journal is preserved.
A license agreement is a document in which the author warrants that he/she owns all copyright for the work (manuscript, article, etc.).
The authors, signing the License Agreement with TECHNOLOGY CENTER PC, have all rights to the further use of their work, provided that they link to our edition in which the work was published.
According to the terms of the License Agreement, the Publisher TECHNOLOGY CENTER PC does not take away your copyrights and receives permission from the authors to use and dissemination of the publication through the world's scientific resources (own electronic resources, scientometric databases, repositories, libraries, etc.).
In the absence of a signed License Agreement or in the absence of this agreement of identifiers allowing to identify the identity of the author, the editors have no right to work with the manuscript.
It is important to remember that there is another type of agreement between authors and publishers – when copyright is transferred from the authors to the publisher. In this case, the authors lose ownership of their work and may not use it in any way.