The optimization of cargo delivery processes with dynamic route updates in smart logistics
DOI:
https://doi.org/10.15587/1729-4061.2023.277583Keywords:
dynamic routing, intelligent methods, smart logistics, Internet of Things, big dataAbstract
The object of research is the processes of transport logistics management under the influence of non-stationary factors of different nature on the functioning of street-road networks (SRN) in cities. The task of dynamic routing at large and variable loading of SRN sections is solved by managing the processes of cargo delivery in real time within the framework of the implementation of the Smart Logistics concept.
Simulation studies of cargo delivery routing with dynamic real-time route updating using a modified ant colony algorithm and data on the dynamics of traffic flow (TF) were conducted using an SRN in the city of Kyiv as an example. Here, experimental data were obtained using motion sensors of intelligent transport systems. During the optimization, current data were used acquired online within the framework of the Internet of Things technology, as well as historical data obtained over past periods of time and averaged using Big Data (BD) technology. Route optimization at each stage of real-time updates was achieved using a modified ant colony algorithm. This method has a sufficiently high optimization performance and makes it possible, unlike many other intelligent methods, to directly take into account the non-stationary dynamics of TF within SRN. It is shown that the use of properly averaged BD historical data allows for more efficient planning of transport routes.
The simulation studies indicate the possibility of using the proposed approach by transport companies and authorities to solve the problems of managing logistics flows in an automated mode under conditions of complex, unpredictable traffic
References
- A handbook on sustainable urban mobility and spatial planning: promoting active mobility (2020). United Nations Economic Commission for Europe. doi: https://doi.org/10.18356/8d742f54-en
- Schroten, A., Van Grinsven, A., Tol, E., Leestemaker, L., Schackmann, P. P., Vonk-Noordegraaf, D. et al. (2020). Research for TRAN Committee – The impact of emerging technologies on the transport system. European Parliament, Policy Department for Structural and Cohesion Policies, Brussels. Available at: https://www.europarl.europa.eu/RegData/etudes/STUD/2020/652226/IPOL_STU(2020)652226_EN.pdf
- Larsen, A. (2000). The dynamic vehicle routing problem. Technical University of Denmark. IMM-PHD. Available at: https://backend.orbit.dtu.dk/ws/portalfiles/portal/5261816/imm143.pdf
- Erdoğan, G. (2017). An open source Spreadsheet Solver for Vehicle Routing Problems. Computers & Operations Research, 84, 62–72. doi: https://doi.org/10.1016/j.cor.2017.02.022
- Thompson, R. G., Zhang, L. (2018). Optimising courier routes in central city areas. Transportation Research Part C: Emerging Technologies, 93, 1–12. doi: https://doi.org/10.1016/j.trc.2018.05.016
- Jamil, A., Abdallah, B. N., Leksono, V. A. (2021). Firefly Algorithm for Multi-type Vehicle Routing Problem. Journal of Physics: Conference Series, 1726 (1), 012006. doi: https://doi.org/10.1088/1742-6596/1726/1/012006
- Wu, H., Gao, Y., Wang, W., Zhang, Z. (2021). A hybrid ant colony algorithm based on multiple strategies for the vehicle routing problem with time windows. Complex & Intelligent Systems. doi: https://doi.org/10.1007/s40747-021-00401-1
- Giuffrida, N., Fajardo-Calderin, J., Masegosa, A. D., Werner, F., Steudter, M., Pilla, F. (2022). Optimization and Machine Learning Applied to Last-Mile Logistics: A Review. Sustainability, 14 (9), 5329. doi: https://doi.org/10.3390/su14095329
- Zajkani, M. A., Baghdorani, R. R., Haeri, M. (2021). Model predictive based approach to solve DVRP with traffic congestion. IFAC-PapersOnLine, 54 (21), 163–167. doi: https://doi.org/10.1016/j.ifacol.2021.12.028
- Zhang, H., Zhang, Q., Ma, L., Zhang, Z., Liu, Y. (2019). A hybrid ant colony optimization algorithm for a multi-objective vehicle routing problem with flexible time windows. Information Sciences, 490, 166–190. doi: https://doi.org/10.1016/j.ins.2019.03.070
- Belhaiza, S., M’Hallah, R., Ben Brahim, G., Laporte, G. (2019). Three multi-start data-driven evolutionary heuristics for the vehicle routing problem with multiple time windows. Journal of Heuristics, 25 (3), 485–515. doi: https://doi.org/10.1007/s10732-019-09412-1
- Hoogeboom, M., Dullaert, W. (2019). Vehicle routing with arrival time diversification. European Journal of Operational Research, 275 (1), 93–107. doi: https://doi.org/10.1016/j.ejor.2018.11.020
- Yu, X. (2022). Logistics Distribution for Path Optimization Using Artificial Neural Network and Decision Support System. Research Square. doi: https://doi.org/10.21203/rs.3.rs-1249887/v1
- Zhang, N. (2018). Smart Logistics Path for Cyber-Physical Systems With Internet of Things. IEEE Access, 6, 70808–70819. doi: https://doi.org/10.1109/access.2018.2879966
- Sánchez-Díaz, I., Holguín-Veras, J., Ban, X. (2015). A time-dependent freight tour synthesis model. Transportation Research Part B: Methodological, 78, 144–168. doi: https://doi.org/10.1016/j.trb.2015.04.007
- Zhang, L., Thompson, R. G. (2019). Understanding the benefits and limitations of occupancy information systems for couriers. Transportation Research Part C: Emerging Technologies, 105, 520–535. doi: https://doi.org/10.1016/j.trc.2019.06.013
- Russo, F., Comi, A. (2021). Sustainable Urban Delivery: The Learning Process of Path Costs Enhanced by Information and Communication Technologies. Sustainability, 13 (23), 13103. doi: https://doi.org/10.3390/su132313103
- Danchuk, V., Bakulich, O., Svatko, V. (2019). Building Optimal Routes for Cargo Delivery in Megacities. Transport and Telecommunication Journal, 20 (2), 142–152. doi: https://doi.org/10.2478/ttj-2019-0013
- Danchuk, V., Weiß, C., Svatko, V. (2022). Smart logistics within the framework of the concept of cyber-physical systems. Intelligent Transport Systems: Ecology, Safety, Quality, Comfort. doi: https://doi.org/10.33744/978-966-632-318-0-2022-3-14-19
- Zenchenko, V. A., Rementsov, A. N., Pavlov, A. V., Sotskov, A. V. (2012). Assessment of parameters of environment and the main transport streams defining a situation on a street road network. Modern High Technologies, 2, 52–59. Available at: https://s.top-technologies.ru/pdf/2012/2/9.pdf
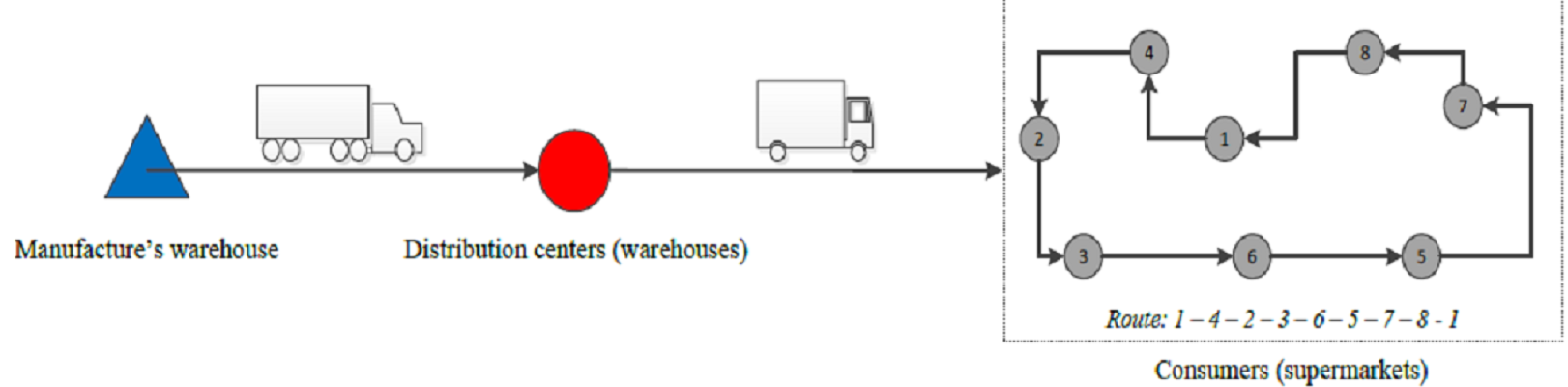
Downloads
Published
How to Cite
Issue
Section
License
Copyright (c) 2023 Viktor Danchuk, Antonio Comi, Christian Weiß, Vitalii Svatko

This work is licensed under a Creative Commons Attribution 4.0 International License.
The consolidation and conditions for the transfer of copyright (identification of authorship) is carried out in the License Agreement. In particular, the authors reserve the right to the authorship of their manuscript and transfer the first publication of this work to the journal under the terms of the Creative Commons CC BY license. At the same time, they have the right to conclude on their own additional agreements concerning the non-exclusive distribution of the work in the form in which it was published by this journal, but provided that the link to the first publication of the article in this journal is preserved.
A license agreement is a document in which the author warrants that he/she owns all copyright for the work (manuscript, article, etc.).
The authors, signing the License Agreement with TECHNOLOGY CENTER PC, have all rights to the further use of their work, provided that they link to our edition in which the work was published.
According to the terms of the License Agreement, the Publisher TECHNOLOGY CENTER PC does not take away your copyrights and receives permission from the authors to use and dissemination of the publication through the world's scientific resources (own electronic resources, scientometric databases, repositories, libraries, etc.).
In the absence of a signed License Agreement or in the absence of this agreement of identifiers allowing to identify the identity of the author, the editors have no right to work with the manuscript.
It is important to remember that there is another type of agreement between authors and publishers – when copyright is transferred from the authors to the publisher. In this case, the authors lose ownership of their work and may not use it in any way.