Developing a risk management approach based on reinforcement training in the formation of an investment portfolio
DOI:
https://doi.org/10.15587/1729-4061.2023.277997Keywords:
investment portfolio, risk management, machine learning, actor-critic, learning without a trainerAbstract
Investments play a significant role in the functioning and development of the economy. Risk management is an integral part of the formation of the investment portfolio. This means that an investor must be willing to take on a certain level of risk in order to receive a certain level of return. However, when forming an investment portfolio, an investor faces such problems as market unpredictability, asset correlation, incorrect asset allocation. Therefore, when forming an investment portfolio, an investor should carefully study all possible risks and try to minimize them. The object of research is an approach to risk management in the formation of an investment portfolio using the method of reinforcement training. The basic principles of formation of the investment portfolio and determination of risks are described. The application of the method of reinforcement training for building a model of risk management of investment portfolio is considered. The process of selecting optimal investment assets based on alternative data sources that minimize risks and maximize profits is also considered. A functional model of the process of risk optimization in the formation of an investment portfolio based on machine learning methods has been developed. The functional model constructed makes it possible to build a process of risk optimization, including asset selection, risk comparison and assessment, to form an investment portfolio and monitor its risks. The study results showed that the proposed approach to the formation of the investment portfolio increased the total growth of the investment portfolio by 0.4363 compared to the base model. Also, the volatility indicator improved compared to the market, as evidenced by the percentage difference between the initial and final cash amount, which increased from 128.98 to 295.57
Supporting Agencies
- інвестиційний портфель
- управління ризиками
- машинне навчання
- актор-критик
- навчання без учителя
References
- Kaftia, M. A. (2019). The Formation of Modern Portfolio Theories: the Main Problems and Tendencies of Development. Business Inform, 2 (493), 414–419. doi: https://doi.org/10.32983/2222-4459-2019-2-414-419
- Romanenkov, Y., Vartanian, V. (2016). Formation of prognostic software support for strategic decision-making in an organization. Eastern-European Journal of Enterprise Technologies, 2 (9 (80)), 25–34. doi: https://doi.org/10.15587/1729-4061.2016.66306
- Zadoia, A. O. (2019). Portfolio investments in Ukraine: chance or challenges? Academic Review, 2, 81–92. doi: https://doi.org/10.32342/2074-5354-2019-2-51-8
- Markowitz, H. (1952). Portfolio selection. The Journal of Finance, 7 (1), 77–91. doi: https://doi.org/10.1111/j.1540-6261.1952.tb01525.x
- Roy, A. D. (1952). Safety First and the Holding of Assets. Econometrica, 20 (3), 431. doi: https://doi.org/10.2307/1907413
- Sullivan, E. J. (2011). A.D. Roy: The Forgotten Father of Portfolio Theory. Research in the History of Economic Thought and Methodology, 73–82. doi: https://doi.org/10.1108/s0743-4154(2011)000029a008
- Jagannathan, R., Ma, T. (2003). Risk Reduction in Large Portfolios: Why Imposing the Wrong Constraints Helps. The Journal of Finance, 58 (4), 1651–1683. doi: https://doi.org/10.1111/1540-6261.00580
- Ratushna, Yu. S. (2019). Foreign financial investment development factors. Naukovyi visnyk Uzhhorodskoho natsionalnoho universytetu. Seriya: Mizhnarodni ekonomichni vidnosyny ta svitove hospodarstvo, 24 (3), 59–66. Available at: http://nbuv.gov.ua/UJRN/Nvuumevcg_2019_24%283%29__13
- Lopez, J. A. (1999). Methods for evaluating value-at-risk estimates. Economic Review, Federal Reserve Bank of San Francisco.
- Sunchalin, A. M. et al. (2019). Methods of risk management in portfolio theory. Available at: https://www.revistaespacios.com/a19v40n16/a19v40n16p25.pdf
- Ye, Y., Pei, H., Wang, B., Chen, P.-Y., Zhu, Y., Xiao, J., Li, B. (2020). Reinforcement-Learning Based Portfolio Management with Augmented Asset Movement Prediction States. Proceedings of the AAAI Conference on Artificial Intelligence, 34 (01), 1112–1119. doi: https://doi.org/10.1609/aaai.v34i01.5462
- Reinders, H. J., Schoenmaker, D., van Dijk, M. (2023). A finance approach to climate stress testing. Journal of International Money and Finance, 131, 102797. doi: https://doi.org/10.1016/j.jimonfin.2022.102797
- Emamat, M. S. M. M., Mota, C. M. de M., Mehregan, M. R., Sadeghi Moghadam, M. R., Nemery, P. (2022). Using ELECTRE-TRI and FlowSort methods in a stock portfolio selection context. Financial Innovation, 8 (1). doi: https://doi.org/10.1186/s40854-021-00318-1
- Lukina, N. P., Nurgaleeva, L. V. (2005). Valuegical and ideological status of a network community in information social space: statement of a problem. Gumanitarnaya informatika.
- Romanenkov, Y., Danova, M., Kashcheyeva, V., Bugaienko, O., Volk, M., Karminska-Bielobrova, M., Lobach, O. (2018). Complexification methods of interval forecast estimates in the problems on shortterm prediction. Eastern-European Journal of Enterprise Technologies, 3 (3 (93)), 50–58. doi: https://doi.org/10.15587/1729-4061.2018.131939
- Model velykykh danykh ta mashynnoho navchannia. Available at: https://business.diia.gov.ua/en/handbook/impact-investment/model-velikih-danih-ta-masinnogo-navcanna
- Marbach, P., Tsitsiklis, J. N. (2001). Simulation-based optimization of Markov reward processes. IEEE Transactions on Automatic Control, 46 (2), 191–209. doi: https://doi.org/10.1109/9.905687
- Raskin, L., Sukhomlyn, L., Sagaidachny, D., Korsun, R. (2021). Analysis of multi-threaded markov systems. Advanced Information Systems, 5 (4), 70–78. doi: https://doi.org/10.20998/2522-9052.2021.4.11
- Raskin, L., Sira, O., Sukhomlyn, L., Parfeniuk, Y. (2021). Universal method for solving optimization problems under the conditions of uncertainty in the initial data. Eastern-European Journal of Enterprise Technologies, 1 (4 (109)), 46–53. doi: https://doi.org/10.15587/1729-4061.2021.225515
- Raskin, L., Sira, O. (2016). Method of solving fuzzy problems of mathematical programming. Eastern-European Journal of Enterprise Technologies, 5 (4 (83)), 23–28. doi: https://doi.org/10.15587/1729-4061.2016.81292
- Alibekov, E., Kubalík, J., Babuška, R. (2018). Policy derivation methods for critic-only reinforcement learning in continuous spaces. Engineering Applications of Artificial Intelligence, 69, 178–187. doi: https://doi.org/10.1016/j.engappai.2017.12.004
- Semenov, S., Weilin, C., Zhang, L., Bulba, S. (2021). Automated penetration testing method using deep machine learning technology. Advanced Information Systems, 5 (3), 119–127. doi: https://doi.org/10.20998/2522-9052.2021.3.16
- Zheng, L., Fiez, T., Alumbaugh, Z., Chasnov, B., Ratliff, L. J. (2022). Stackelberg Actor-Critic: Game-Theoretic Reinforcement Learning Algorithms. Proceedings of the AAAI Conference on Artificial Intelligence, 36 (8), 9217–9224. doi: https://doi.org/10.1609/aaai.v36i8.20908
- Mnih, V. et al. (2016). Asynchronous methods for deep reinforcement learning. International conference on machine learning. doi: https://doi.org/10.48550/arXiv.1602.01783
- Grondman, I., Busoniu, L., Lopes, G. A. D., Babuska, R. (2012). A Survey of Actor-Critic Reinforcement Learning: Standard and Natural Policy Gradients. IEEE Transactions on Systems, Man, and Cybernetics, Part C (Applications and Reviews), 42 (6), 1291–1307. doi: https://doi.org/10.1109/tsmcc.2012.2218595
- A faster, simpler approach to parallel Python. Available at: https://www.ray.io/ray-core
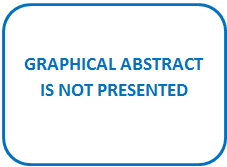
Downloads
Published
How to Cite
Issue
Section
License
Copyright (c) 2023 Vitalii Martovytskyi, Volodymyr Argunov, Igor Ruban, Yuri Romanenkov

This work is licensed under a Creative Commons Attribution 4.0 International License.
The consolidation and conditions for the transfer of copyright (identification of authorship) is carried out in the License Agreement. In particular, the authors reserve the right to the authorship of their manuscript and transfer the first publication of this work to the journal under the terms of the Creative Commons CC BY license. At the same time, they have the right to conclude on their own additional agreements concerning the non-exclusive distribution of the work in the form in which it was published by this journal, but provided that the link to the first publication of the article in this journal is preserved.
A license agreement is a document in which the author warrants that he/she owns all copyright for the work (manuscript, article, etc.).
The authors, signing the License Agreement with TECHNOLOGY CENTER PC, have all rights to the further use of their work, provided that they link to our edition in which the work was published.
According to the terms of the License Agreement, the Publisher TECHNOLOGY CENTER PC does not take away your copyrights and receives permission from the authors to use and dissemination of the publication through the world's scientific resources (own electronic resources, scientometric databases, repositories, libraries, etc.).
In the absence of a signed License Agreement or in the absence of this agreement of identifiers allowing to identify the identity of the author, the editors have no right to work with the manuscript.
It is important to remember that there is another type of agreement between authors and publishers – when copyright is transferred from the authors to the publisher. In this case, the authors lose ownership of their work and may not use it in any way.