Development of the solution search method using the population algorithm of global search optimization
DOI:
https://doi.org/10.15587/1729-4061.2023.281007Keywords:
hybrid actions, artificial neural networks, bio-inspired algorithms, use of forces (troops) of the Naval ForcesAbstract
The research objects are decision making support systems. subject research is a decision making process in management tasks using bio-inspired algorithms. A method of finding solutions using the population algorithm of global search optimization is proposed. Joint use of invasive weed algorithm is proposed, genetic algorithm and evolving artificial neural networks are improved. The method has the following sequence of actions:
‒ an input of initial data;
‒ processing of initial data taking into account the degree of uncertainty;
‒ formation of the optimization vector;
‒ creation of descendant vectors;
‒ ordering of vectors in descending order;
‒ reducing the dimensionality of the feature space;
‒ teaching knowledge bases.
The peculiarity of the proposed method lies in the placement of agents-weeds, taking into account the uncertainty of the initial data, improved procedures for reducing the space of signs about the analysis object state.
Training of synaptic weights of an artificial neural network, type and parameters of the membership function and the architecture of individual elements, and the architecture of an artificial neural network as a whole is carried out. The proposed method was simulated in the MathСad 14 software environment. The task to be solved during the simulation was to determine the route of the ships in the operational zones of the Black and Azov seas in the conditions of hybrid actions of the enemy. The use of the method makes it possible to increase the efficiency of data processing at the level of 21–27 % due to the use of additional improved procedures. The proposed method should be used to solve the problems of evaluating complex and dynamic processes in the interests of solving national security problems
References
- Bashkyrov, O. M., Kostyna, O. M., Shyshatskyi, A. V. (2015). Rozvytok intehrovanykh system zviazku ta peredachi danykh dlia potreb Zbroinykh Syl. Ozbroiennia ta viyskova tekhnika, 1, 35–39. Available at: http://nbuv.gov.ua/UJRN/ovt_2015_1_7
- Dudnyk, V., Sinenko, Y., Matsyk, M., Demchenko, Y., Zhyvotovskyi, R., Repilo, I. et al. (2020). Development of a method for training artificial neural networks for intelligent decision support systems. Eastern-European Journal of Enterprise Technologies, 3 (2 (105)), 37–47. doi: https://doi.org/10.15587/1729-4061.2020.203301
- Sova, O., Shyshatskyi, A., Salnikova, O., Zhuk, O., Trotsko, O., Hrokholskyi, Y. (2021). Development of a method for assessment and forecasting of the radio electronic environment. EUREKA: Physics and Engineering, 4, 30–40. doi: https://doi.org/10.21303/2461-4262.2021.001940
- Pievtsov, H., Turinskyi, O., Zhyvotovskyi, R., Sova, O., Zvieriev, O., Lanetskii, B., Shyshatskyi, A. (2020). Development of an advanced method of finding solutions for neuro-fuzzy expert systems of analysis of the radioelectronic situation. EUREKA: Physics and Engineering, 4, 78–89. doi: https://doi.org/10.21303/2461-4262.2020.001353
- Zuiev, P., Zhyvotovskyi, R., Zvieriev, O., Hatsenko, S., Kuprii, V., Nakonechnyi, O. et al. (2020). Development of complex methodology of processing heterogeneous data in intelligent decision support systems. Eastern-European Journal of Enterprise Technologies, 4 (9 (106)), 14–23. doi: https://doi.org/10.15587/1729-4061.2020.208554
- Shyshatskyi, A., Zvieriev, O., Salnikova, O., Demchenko, Ye., Trotsko, O., Neroznak, Ye. (2020). Complex Methods of Processing Different Data in Intellectual Systems for Decision Support System. International Journal of Advanced Trends in Computer Science and Engineering, 9 (4), 5583‒5590. doi: https://doi.org/10.30534/ijatcse/2020/206942020
- Yeromina, N., Kurban, V., Mykus, S., Peredrii, O., Voloshchenko, O., Kosenko, V. et al. (2021). The Creation of the Database for Mobile Robots Navigation under the Conditions of Flexible Change of Flight Assignment. International Journal of Emerging Technology and Advanced Engineering, 11 (05), 37‒44. doi: https://doi.org/10.46338/ijetae0521_05
- Rotshteyn, A. P. (1999). Intellektual'nye tekhnologii identifikatsii: nechetkie mnozhestva, geneticheskie algoritmy, neyronnye seti. Vinnitsa: “UNIVERSUM”, 320.
- Alpeeva, E. A., Volkova, I. I. (2019). The use of fuzzy cognitive maps in the development of an experimental model of automation of production accounting of material flows. Russian Journal of Industrial Economics, 12 (1), 97–106. doi: https://doi.org/10.17073/2072-1633-2019-1-97-106
- Zagranovskaya, A. V., Eyssner, Yu. N. (2017). Modelirovanie stsenariev razvitiya ekonomicheskoy situatsii na osnove nechetkikh kognitivnykh kart. Sovremennaya Ekonomika: Problemy i Resheniya, 10, 33–47. doi: https://doi.org/10.17308/meps.2017.10/1754
- Simankov, V. S., Putyato, M. M. (2013). Issledovanie metodov kognitivnogo analiza. Sistemnyy analiz, upravlenie i obrabotka informatsii, 13, 31‒35.
- Ko, Y.-C., Fujita, H. (2019). An evidential analytics for buried information in big data samples: Case study of semiconductor manufacturing. Information Sciences, 486, 190–203. doi: https://doi.org/10.1016/j.ins.2019.01.079
- Ramaji, I. J., Memari, A. M. (2018). Interpretation of structural analytical models from the coordination view in building information models. Automation in Construction, 90, 117–133. doi: https://doi.org/10.1016/j.autcon.2018.02.025
- Pérez-González, C. J., Colebrook, M., Roda-García, J. L., Rosa-Remedios, C. B. (2019). Developing a data analytics platform to support decision making in emergency and security management. Expert Systems with Applications, 120, 167–184. doi: https://doi.org/10.1016/j.eswa.2018.11.023
- Chen, H. (2018). Evaluation of Personalized Service Level for Library Information Management Based on Fuzzy Analytic Hierarchy Process. Procedia Computer Science, 131, 952–958. doi: https://doi.org/10.1016/j.procs.2018.04.233
- Chan, H. K., Sun, X., Chung, S.-H. (2019). When should fuzzy analytic hierarchy process be used instead of analytic hierarchy process? Decision Support Systems, 125, 113114. doi: https://doi.org/10.1016/j.dss.2019.113114
- A Osman, A. M. S. (2019). A novel big data analytics framework for smart cities. Future Generation Computer Systems, 91, 620–633. doi: https://doi.org/10.1016/j.future.2018.06.046
- Gödri, I., Kardos, C., Pfeiffer, A., Váncza, J. (2019). Data analytics-based decision support workflow for high-mix low-volume production systems. CIRP Annals, 68 (1), 471–474. doi: https://doi.org/10.1016/j.cirp.2019.04.001
- Harding, J. L. (2013). Data quality in the integration and analysis of data from multiple sources: some research challenges. International Archives of the Photogrammetry, Remote Sensing and Spatial Information Sciences, XL-2/W1, 59–63. doi: https://doi.org/10.5194/isprsarchives-XL-2-W1-59-2013
- Kosko, B. (1986). Fuzzy cognitive maps. International Journal of Man-Machine Studies, 24 (1), 65–75. doi: https://doi.org/10.1016/S0020-7373(86)80040-2
- Gorelova, G. V. (2013). Kognitivnyy podkhod k imitatsionnomu modelirovaniyu slozhnykh sistem. Izvestiya YuFU. Tekhnicheskie nauki, 3, 239–250.
- Koval, M., Sova, O., Shyshatskyi, A., Artabaiev, Y., Garashchuk, N., Yivzhenko, Y. et al. (2022). Improving the method for increasing the efficiency of decision-making based on bio-inspired algorithms. Eastern-European Journal of Enterprise Technologies, 6 (4 (120)), 6–13. doi: https://doi.org/10.15587/1729-4061.2022.268621
- Rad, H. S., Lucas, C. (2007). A recommender system based on invasive weed optimization algorithm. 2007 IEEE Congress on Evolutionary Computation. doi: https://doi.org/10.1109/cec.2007.4425032
- Koshlan, A., Salnikova, O., Chekhovska, M., Zhyvotovskyi, R., Prokopenko, Y., Hurskyi, T. et al. (2019). Development of an algorithm for complex processing of geospatial data in the special-purpose geoinformation system in conditions of diversity and uncertainty of data. Eastern-European Journal of Enterprise Technologies, 5 (9 (101)), 35–45. doi: https://doi.org/10.15587/1729-4061.2019.180197
- Mahdi, Q. A., Shyshatskyi, A., Prokopenko, Y., Ivakhnenko, T., Kupriyenko, D., Golian, V. et al. (2021). Development of estimation and forecasting method in intelligent decision support systems. Eastern-European Journal of Enterprise Technologies, 3 (9 (111)), 51–62. doi: https://doi.org/10.15587/1729-4061.2021.232718
- Emel'yanov, V. V., Kureychik, V. V., Kureychik, V. M., Emel'yanov, V. V. (2003). Teoriya i praktika evolyutsionnogo modelirovaniya. Moscow: Fizmatlit, 432.
- Gorokhovatsky, V., Stiahlyk, N., Tsarevska, V. (2021). Combination method of accelerated metric data search in image classification problems. Advanced Information Systems, 5 (3), 5–12. doi: https://doi.org/10.20998/2522-9052.2021.3.01
- Levashenko, V., Liashenko, O., Kuchuk, H. (2020). Building Decision Support Systems based on Fuzzy Data. Advanced Information Systems, 4 (4), 48–56. doi: https://doi.org/10.20998/2522-9052.2020.4.07
- Meleshko, Y., Drieiev, O., Drieieva, H. (2020). Method of identification bot profiles based on neural networks in recommendation systems. Advanced Information Systems, 4 (2), 24–28. doi: https://doi.org/10.20998/2522-9052.2020.2.05
- Kuchuk, N., Merlak, V., Skorodelov, V. (2020). A method of reducing access time to poorly structured data. Advanced Information Systems, 4 (1), 97–102. doi: https://doi.org/10.20998/2522-9052.2020.1.14
- Shyshatskyi, A., Tiurnikov, M., Suhak, S., Bondar, O., Melnyk, A., Bokhno, T., Lyashenko, A. (2020). Method of assessment of the efficiency of the communication of operational troop grouping system. Advanced Information Systems, 4 (1), 107–112. doi: https://doi.org/10.20998/2522-9052.2020.1.16
- Raskin, L., Sira, O. (2016). Method of solving fuzzy problems of mathematical programming. Eastern-European Journal of Enterprise Technologies, 5 (4 (83)), 23–28. doi: https://doi.org/10.15587/1729-4061.2016.81292
- Lytvyn, V., Vysotska, V., Pukach, P., Brodyak, O., Ugryn, D. (2017). Development of a method for determining the keywords in the slavic language texts based on the technology of web mining. Eastern-European Journal of Enterprise Technologies, 2 (2 (86)), 14–23. doi: https://doi.org/10.15587/1729-4061.2017.98750
- Stepanenko, A., Oliinyk, A., Deineha, L., Zaiko, T. (2018). Development of the method for decomposition of superpositions of unknown pulsed signals using the secondorder adaptive spectral analysis. Eastern-European Journal of Enterprise Technologies, 2 (9 (92)), 48–54. doi: https://doi.org/10.15587/1729-4061.2018.126578
- Gorbenko, I., Ponomar, V. (2017). Examining a possibility to use and the benefits of post-quantum algorithms dependent on the conditions of their application. Eastern-European Journal of Enterprise Technologies, 2 (9 (86)), 21–32. doi: https://doi.org/10.15587/1729-4061.2017.96321
- Lovska, A. A. (2015). Peculiarities of computer modeling of strength of body bearing construction of gondola car during transportation by ferry-bridge. Metallurgical and Mining Industry, 1, 49–54. Available at: https://www.metaljournal.com.ua/assets/Journal/english-edition/MMI_2015_1/10%20Lovska.pdf
- Lovska, A., Fomin, O. (2020). A new fastener to ensure the reliability of a passenger car body on a train ferry. Acta Polytechnica, 60 (6). doi: https://doi.org/10.14311/ap.2020.60.0478
- Koval, M., Sova, O., Orlov, O., Shyshatskyi, A., Artabaiev, Y., Shknai, O. et al. (2022). Improvement of complex resource management of special-purpose communication systems. Eastern-European Journal of Enterprise Technologies, 5 (9 (119)), 34–44. doi: https://doi.org/10.15587/1729-4061.2022.266009
- Syrotenko, A. M. (Red.) (2020). Voienni aspekty protydiyi “hibrydniy ahresiyi”: dosvid Ukrainy. Kyiv: NUOU imeni Ivana Cherniakhovskoho, 176. Available at: https://nuou.org.ua/assets/monography/mono_gibr_viin.pdf
- Yakymiak, S. (2021). Hybrid warfare in the Black Sea: lessons learned and training improvement. Current issues of military specialists training in the security and defence sector under conditions of hybrid threats. Warszawa: Wydawnictwo Instytutu Bezpieczeństwa I Rozwoju Międzynarodowego, 396–405. Available at: https://www.academia.edu/47706282/CURRENT_ISSUES_OF_MILITARY_SPECIALISTS_TRAINING_IN_THE_SECURITY_AND_DEFENCE_SECTOR_UNDER_CONDITIONS_OF_HYBRID_THREATS
- Yakimyak, S., Vdovitsky, E. (2021). Analysis of factors that may affect on the effectiveness of use the Navy duties during the protection of the economic activities of the state at sea in the context of hybrid enemy actions. Збірник Наукових Prats Tsentru Voienno-Stratehichnykh Doslidzhen NUOU Imeni Ivana Cherniakhovskoho, 1 (71), 75–81.doi: https://doi.org/10.33099/2304-2745/2021-1-71/75-81
- Vdovytskyi, Ye. A. (2022). Analiz isnuiuchoho naukovo-metodychnoho aparatu otsiniuvannia efektyvnosti zastosuvannia syl (viysk) VMS pid chas vykonannia zavdan zakhystu ekonomichnoi diyalnosti derzhavy na mori v umovakh hibrydnykh diy protyvnyka. Trudy universytetu, 3 (172), 202–208.
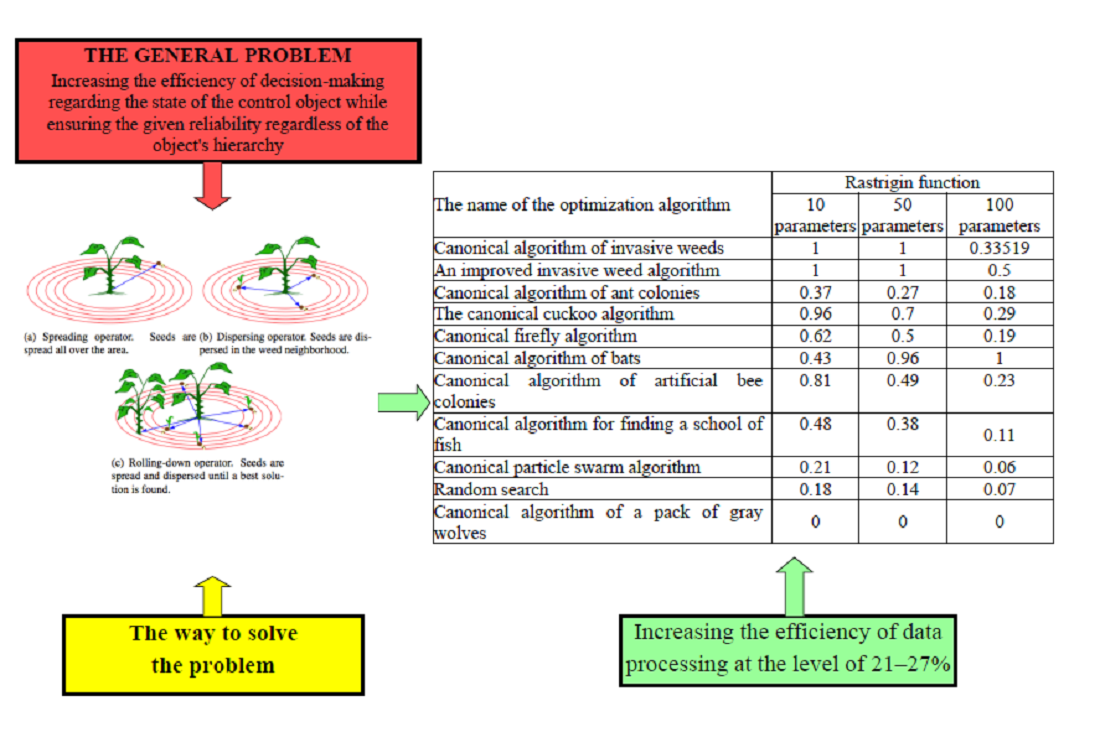
Downloads
Published
How to Cite
Issue
Section
License
Copyright (c) 2023 Stepan Yakymiak, Yevhenii Vdovytskyi, Yurii Artabaiev, Larisa Degtyareva, Yuliia Vakulenko, Serhii Nevhad, Vitalii Andronov, Roman Lazuta, Petro Shapoval, Yevhen Artamonov

This work is licensed under a Creative Commons Attribution 4.0 International License.
The consolidation and conditions for the transfer of copyright (identification of authorship) is carried out in the License Agreement. In particular, the authors reserve the right to the authorship of their manuscript and transfer the first publication of this work to the journal under the terms of the Creative Commons CC BY license. At the same time, they have the right to conclude on their own additional agreements concerning the non-exclusive distribution of the work in the form in which it was published by this journal, but provided that the link to the first publication of the article in this journal is preserved.
A license agreement is a document in which the author warrants that he/she owns all copyright for the work (manuscript, article, etc.).
The authors, signing the License Agreement with TECHNOLOGY CENTER PC, have all rights to the further use of their work, provided that they link to our edition in which the work was published.
According to the terms of the License Agreement, the Publisher TECHNOLOGY CENTER PC does not take away your copyrights and receives permission from the authors to use and dissemination of the publication through the world's scientific resources (own electronic resources, scientometric databases, repositories, libraries, etc.).
In the absence of a signed License Agreement or in the absence of this agreement of identifiers allowing to identify the identity of the author, the editors have no right to work with the manuscript.
It is important to remember that there is another type of agreement between authors and publishers – when copyright is transferred from the authors to the publisher. In this case, the authors lose ownership of their work and may not use it in any way.