Розробка методу виявлення атак «злий двійник» на мережі стандарту IEEE 802.11 (Wi-Fi) за допомогою моделі класифікації KNN
DOI:
https://doi.org/10.15587/1729-4061.2023.282131Ключові слова:
IEEE 802.11, Wi-Fi, evil twin, машинне навчання, класифікація, тріангуляція, кібербезпекаАнотація
Обʼєктом дослідження виступають IEEE 802.11 (Wi-Fi) мережі, які часто є цілями групи атак під назвою «злий двійник». Дослідження даної теми є надзвичайно важливим, оскільки технологія Wi-Fi є дуже розповсюдженим методом підключення до мережі і зазвичай є першою ціллю кіберзлочинців в атаках на підприємства. За допомогою систематичного аналізу літератури зосередженої на протидію атакам типу «злий двійник», дана робота визначає основні переваги застосування систем штучного інтелекту, у аналізі мережевих даних та ідентифікації вторгнення в мережі Wi-Fi. Для оцінки ефективності виявлення вторгнень та аналізу кіберзлочинів проведено ряд експериментів максимально наближених до реальних атак на мережі Wi-Fi.
В рамках дослідження, що описано в даній статті, запропоновано метод виявлення кіберзлочинів у бездротових мережах стандарту IEEE 802.11 (Wi-Fi) за допомогою штучного інтелекту, а саме моделі побудованої на базі методу k-найближчих сусідів. Даний метод заснований на класифікації попередньо зібраних даних, а саме потужності сигналу від точки доступу, а потім безперервному порівнянню новозібраних даних із натренованою моделлю.
Розроблено компактний та енергоефективний прототип апаратно-програмного комплексу для реалізації моніторингу, аналізу мережевих пакетів ефірі та збереження даних на основі часових рядів. Задля зменшення навантаження на комп’ютерну мережу, і, зважаючи на обмежену обчислювальну здатність комплексу, було запропоновано метод агрегації даних, який забезпечує швидку передачу інформації.
Отримані результати, а саме 100 % тестових випадків (більше 7 тисяч), було класифіковано правильно, що вказує, на те, що обраний метод аналізу даних дозволить значно підвищити безпеку інформаційно-комунікаційних системах державного та приватного рівнів
Посилання
- Nagpal, J., Patil, R., Jain, V., Pokhriyal, R., Rajawat, R. (2018). Evil Twin Attack and Its Detection. International Journal of Emerging Technologies and Innovative Research, 5 (12), 169–171. doi: https://www.jetir.org/view?paper=JETIR1812326
- Bednarczyk, M., Piotrowski, Z. (2019). Will WPA3 really provide Wi-Fi security at a higher level? XII Conference on Reconnaissance and Electronic Warfare Systems. doi: https://doi.org/10.1117/12.2525020
- Vanhoef, M., Ronen, E. (2020). Dragonblood: Analyzing the Dragonfly Handshake of WPA3 and EAP-pwd. 2020 IEEE Symposium on Security and Privacy (SP). doi: https://doi.org/10.1109/sp40000.2020.00031
- Value of Wi-Fi. Wi-Fi Alliance. Available at: https://www.wi-fi.org/discover-wi-fi/value-of-wi-fi
- Forbes, G., Massie, S., Craw, S. (2020). WiFi-based Human Activity Recognition using Raspberry Pi. 2020 IEEE 32nd International Conference on Tools with Artificial Intelligence (ICTAI). doi: https://doi.org/10.1109/ictai50040.2020.00115
- Banakh, R., Piskozub, A. (2018). Attackers' Wi-Fi Devices Metadata Interception for their Location Identification. 2018 IEEE 4th International Symposium on Wireless Systems within the International Conferences on Intelligent Data Acquisition and Advanced Computing Systems (IDAACS-SWS). doi: https://doi.org/10.1109/idaacs-sws.2018.8525538
- Lu, Q., Qu, H., Zhuang, Y., Lin, X.-J., Ouyang, Y. (2018). Client-Side Evil Twin Attacks Detection Using Statistical Characteristics of 802.11 Data Frames. IEICE Transactions on Information and Systems, E101.D (10), 2465–2473. doi: https://doi.org/10.1587/transinf.2018edp7030
- Modi, V., Parekh, C. (2017). Detection of Rogue Access Point to Prevent Evil Twin Attack in Wireless Network. International Journal of Engineering Research And, V6 (04). doi: https://doi.org/10.17577/ijertv6is040102
- Kuo, E.-C., Chang, M.-S., Kao, D.-Y. (2018). User-side evil twin attack detection using time-delay statistics of TCP connection termination. 2018 20th International Conference on Advanced Communication Technology (ICACT). doi: https://doi.org/10.23919/icact.2018.8323699
- Agarwal, M., Biswas, S., Nandi, S. (2018). An Efficient Scheme to Detect Evil Twin Rogue Access Point Attack in 802.11 Wi-Fi Networks. International Journal of Wireless Information Networks, 25 (2), 130–145. doi: https://doi.org/10.1007/s10776-018-0396-1
- Banakh, R., Piskozub, A., Opirskyy, I. (2018). Detection of MAC Spoofing Attacks in IEEE 802.11 Networks Using Signal Strength from Attackers’ Devices. Advances in Computer Science for Engineering and Education, 468–477. doi: https://doi.org/10.1007/978-3-319-91008-6_47
- Harsha, S. et al. (2019). Improving Wi-Fi security against evil twin attack using light weight machine learning application. COMPUSOFT, 8 (3). Available at: https://www.researchgate.net/publication/332344245_Improving_Wi-Fi_security_against_evil_twin_attack_using_light_weight_machine_learning_application
- Dong, Y., Zampella, F., Alsehly, F. (2023). Beyond KNN: Deep Neighborhood Learning for WiFi-based Indoor Positioning Systems. 2023 IEEE Wireless Communications and Networking Conference (WCNC). doi: https://doi.org/10.1109/wcnc55385.2023.10118752
- Yang, C., Song, Y., Gu, G. (2012). Active User-Side Evil Twin Access Point Detection Using Statistical Techniques. IEEE Transactions on Information Forensics and Security, 7 (5), 1638–1651. doi: https://doi.org/10.1109/tifs.2012.2207383
- Scapy. Available at: https://scapy.net/
- InfluxDB. Available at: https://www.influxdata.com/
- NumPy. Available at: https://numpy.org/
- Pandas. Available at: https://pandas.pydata.org/
- Matplotlib. Available at: https://matplotlib.org/
- Seaborn. Available at: https://seaborn.pydata.org/
- Salkind, N. J., Frey, B. B. (2019). Statistics for people who (think they) hate statistics. SAGE Publications, 76–102.
- Scikit-Learn. Available at: https://scikit-learn.org/stable/
- Mladenova, T., Valova, I. (2021). Analysis of the KNN Classifier Distance Metrics for Bulgarian Fake News Detection. 2021 3rd International Congress on Human-Computer Interaction, Optimization and Robotic Applications (HORA). doi: https://doi.org/10.1109/hora52670.2021.9461333
- Taunk, K., De, S., Verma, S., Swetapadma, A. (2019). A Brief Review of Nearest Neighbor Algorithm for Learning and Classification. 2019 International Conference on Intelligent Computing and Control Systems (ICCS). doi: https://doi.org/10.1109/iccs45141.2019.9065747
- sklearn.metrics.classification_report. Available at: https://scikit-learn.org/stable/modules/generated/sklearn.metrics.classification_report.html
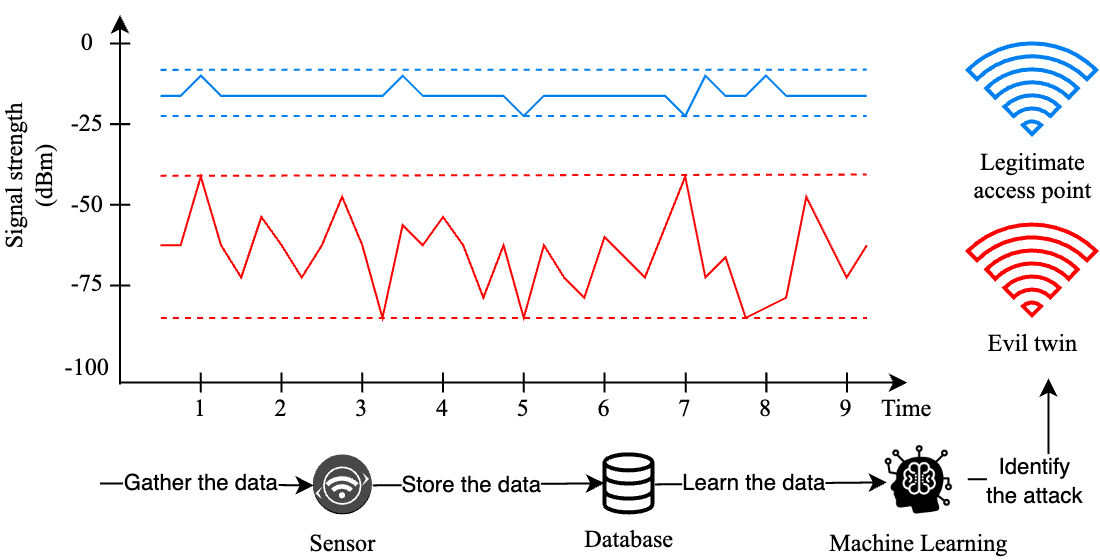
##submission.downloads##
Опубліковано
Як цитувати
Номер
Розділ
Ліцензія
Авторське право (c) 2023 Roman Banakh, Andrian Piskozub, Ivan Opirskyy

Ця робота ліцензується відповідно до Creative Commons Attribution 4.0 International License.
Закріплення та умови передачі авторських прав (ідентифікація авторства) здійснюється у Ліцензійному договорі. Зокрема, автори залишають за собою право на авторство свого рукопису та передають журналу право першої публікації цієї роботи на умовах ліцензії Creative Commons CC BY. При цьому вони мають право укладати самостійно додаткові угоди, що стосуються неексклюзивного поширення роботи у тому вигляді, в якому вона була опублікована цим журналом, але за умови збереження посилання на першу публікацію статті в цьому журналі.
Ліцензійний договір – це документ, в якому автор гарантує, що володіє усіма авторськими правами на твір (рукопис, статтю, тощо).
Автори, підписуючи Ліцензійний договір з ПП «ТЕХНОЛОГІЧНИЙ ЦЕНТР», мають усі права на подальше використання свого твору за умови посилання на наше видання, в якому твір опублікований. Відповідно до умов Ліцензійного договору, Видавець ПП «ТЕХНОЛОГІЧНИЙ ЦЕНТР» не забирає ваші авторські права та отримує від авторів дозвіл на використання та розповсюдження публікації через світові наукові ресурси (власні електронні ресурси, наукометричні бази даних, репозитарії, бібліотеки тощо).
За відсутності підписаного Ліцензійного договору або за відсутністю вказаних в цьому договорі ідентифікаторів, що дають змогу ідентифікувати особу автора, редакція не має права працювати з рукописом.
Важливо пам’ятати, що існує і інший тип угоди між авторами та видавцями – коли авторські права передаються від авторів до видавця. В такому разі автори втрачають права власності на свій твір та не можуть його використовувати в будь-який спосіб.