Identification of influence of digital twin technologies on production systems: a return on investment-based approach
DOI:
https://doi.org/10.15587/1729-4061.2023.283876Keywords:
digital twin, barcode technology, radiofrequency identification, agent-based simulation, financial evaluationAbstract
The object of this study is the impact of different digital twin solutions on the performance of job-shop manufacturing systems, while economic aspects are also taken into consideration. This paper proposes an approach to analyze the impact of different identification systems on the efficiency and ROI of digital twin deployment in production systems. In order to achieve this aim, let’s analyze the investment and operation cost of different Internet of Things technologies. The next phase of the research work was the definition of performance parameters, which makes it possible to analyze the impact of different digital twin solutions on the productivity of the job-shop manufacturing system. It is possible to choose four financial indicators to analyze the economic impact of digital twin solution on job-shop manufacturing: return on investment, compound annual growth rate, internal rate of return and net present value. Our approach is based on a novel agent-based simulation model using AnyLogic simulation tool. From the results of this productivity analyses, the model computes the financial indicators, which describe the expected financial impact of the investment and operation cost. It is compared the impact of barcodes and radiofrequency identification technologies on the financial and technological impact of the job-shop manufacturing environment. The numerical analysis of a job-shop manufacturing system shows, that the radiofrequency identification-based digital twin solution has 9.2 % higher return on investment, 53 % higher net present value and 1.6 % higher compound annual growth rate. The model can be easily converted to analyze other types of manufacturing systems, which can lead to increased efficiency of digital twin solutions
References
- Digital twin market by enterprise, application, industry and geography - global forecast to 2027. MarketsandMarkets. Available at: https://www.reportlinker.com/p05092748/Digital-Twin-Market-by-End-User-And-Geography-Forecast-to.html
- Fuller, A., Fan, Z., Day, C., Barlow, C. (2020). Digital Twin: Enabling Technologies, Challenges and Open Research. IEEE Access, 8, 108952–108971. doi: https://doi.org/10.1109/access.2020.2998358
- Madni, A., Madni, C., Lucero, S. (2019). Leveraging Digital Twin Technology in Model-Based Systems Engineering. Systems, 7 (1), 7. doi: https://doi.org/10.3390/systems7010007
- Kritzinger, W., Karner, M., Traar, G., Henjes, J., Sihn, W. (2018). Digital Twin in manufacturing: A categorical literature review and classification. IFAC-PapersOnLine, 51 (11), 1016–1022. doi: https://doi.org/10.1016/j.ifacol.2018.08.474
- Urbas, U., Hrga, T., Povh, J., Vukašinović, N. (2022). Novel alignment method for optical 3D gear metrology of spur gears with a plain borehole. Measurement, 192, 110839. doi: https://doi.org/10.1016/j.measurement.2022.110839
- Schuh, G., Bergweiler, G., Chougule, M. V., Fiedler, F. (2021). Effects of Digital Twin Simulation Modelling on a Flexible and Fixtureless Production Concept in Automotive Body Shops. Procedia CIRP, 104, 768–773. doi: https://doi.org/10.1016/j.procir.2021.11.129
- Udugama, I., Kelton, W., Bayer, C. (2023). Digital twins in food processing: A conceptual approach to developing multi-layer digital models. Digital Chemical Engineering, 7, 100087. doi: https://doi.org/10.1016/j.dche.2023.100087
- Purcell, W., Neubauer, T. (2023). Digital Twins in Agriculture: A State-of-the-art review. Smart Agricultural Technology, 3, 100094. doi: https://doi.org/10.1016/j.atech.2022.100094
- Manocha, A., Afaq, Y., Bhatia, M. (2023). Digital Twin-assisted Blockchain-inspired irregular event analysis for eldercare. Knowledge-Based Systems, 260, 110138. doi: https://doi.org/10.1016/j.knosys.2022.110138
- Zuhr, P., Rissmann, L., Meißner, S. (2022). Framework for planning and implementation of Digital Process Twins in the field of internal logistics. IFAC-PapersOnLine, 55 (10), 2221–2227. doi: https://doi.org/10.1016/j.ifacol.2022.10.038
- Xie, X., Lu, Q., Parlikad, A. K., Schooling, J. M. (2020). Digital Twin Enabled Asset Anomaly Detection for Building Facility Management. IFAC-PapersOnLine, 53 (3), 380–385. doi: https://doi.org/10.1016/j.ifacol.2020.11.061
- Wong, J., Hoong, P., Teo, E., Lin, A. (2022). Digital Twin: A Conceptualization of the Task-Technology Fit for Individual Users in the Building Maintenance Sector. IOP Conference Series: Earth and Environmental Science, 1101 (9), 092041. doi: https://doi.org/10.1088/1755-1315/1101/9/092041
- Kumar, S. M., Al Mahmoud, M. A. H., Al Yahyaee, N. (2022). Gap to Potential Identification through An Online Process Digital Twin. Day 3 Wed, November 02, 2022. doi: https://doi.org/10.2118/211130-ms
- Lai, W., Zhang, H., Jiang, D., Wang, Y., Wang, R., Zhu, J. et al. (2022). Digital Twin and Big Data Technologies Benefit Oilfield Management. Day 3 Wed, November 02, 2022. doi: https://doi.org/10.2118/211116-ms
- Aslanyan, A., Popov, A., Zhdanov, I., Pakhomov, E., Gulyaev, D., Farakhova, R. et al. (2022). Multiscenario Development Planning by Means of the Digital Twin of the Petroleum Field. Day 1 Wed, March 16, 2022. doi: https://doi.org/10.2118/208970-ms
- Westcott, B. J., Hag-Elsafi, O., Mosaferchi, G., Alampalli, S. (2021). Lifting load restrictions on the NYS Fort Plain Bridge: A case study in SHM and the internet of things. 10th International Conference on Structural Health Monitoring of Intelligent Infrastructure: Transferring Research into Practice. Porto, 1135–1139.
- Skobelev, P., Tabachinskiy, A., Simonova, E., Lee, T.-R., Zhilyaev, A., Laryukhin, V. (2021). Digital twin of rice as a decision-making service for precise farming, based on environmental datasets from the fields. 2021 International Conference on Information Technology and Nanotechnology (ITNT). doi: https://doi.org/10.1109/itnt52450.2021.9649038
- Eppinger, T., Longwell, G., Mas, P., Goodheart, K., Badiali, U., Aglave, R. (2021). Increase food production efficiency using the executable Digital Twin (xDT). Chemical Engineering Transactions, 87, 37–42. doi: https://doi.org/10.3303/CET2187007
- Behnke, J. (2020). Digital Transformation's Impact on Smart Manufacturing. 2020 International Symposium on Semiconductor Manufacturing (ISSM). doi: https://doi.org/10.1109/issm51728.2020.9377506
- Venkateswaran, N. (2020). Industry 4.0 solutions - A pathway to use smart technologies / build smart factories. International Journal of Management (IJM), 11 (2), 132–140.
- Park, S., Lee, S., Park, S., Park, S. (2019). AI-Based Physical and Virtual Platform with 5-Layered Architecture for Sustainable Smart Energy City Development. Sustainability, 11 (16), 4479. doi: https://doi.org/10.3390/su11164479
- Caldarelli, G., Arcaute, E., Barthelemy, M., Batty, M., Gershenson, C., Helbing, D. et al. (2023). The role of complexity for digital twins of cities. Nature Computational Science, 3 (5), 374–381. doi: https://doi.org/10.1038/s43588-023-00431-4
- Bányai, K., Kovács, L. (2023). Impact of digital twin technology on production systems. In Hungarian: Digitális iker technológia hatása a gyártórendszerekre. Production Systems and Information Engineering, 11 (2), 13–32.
- Jia, W., Wang, W., Zhang, Z. (2022). From simple digital twin to complex digital twin Part I: A novel modeling method for multi-scale and multi-scenario digital twin. Advanced Engineering Informatics, 53, 101706. doi: https://doi.org/10.1016/j.aei.2022.101706
- Types of databases. Available at: https://www.javatpoint.com/types-of-databases
- What is Application Server? Available at: https://www.educba.com/what-is-application-server/
- What Are Application Controls? Definition, Examples & Best Practices. Diligent. Available at: https://www.diligent.com/insights/grc/application-controls/
- The Cost of IoT Sensors Is Dropping Fast. Available at: https://www.iofficecorp.com/blog/cost-of-iot-sensors
- How much does IoT cost? URL: https://itrexgroup.com/blog/how-much-iot-cost-factors-challenges/
- How to calculate your true database costs. Available at: https://www.cockroachlabs.com/blog/true-cost-cloud-database/
- How Much Does Cyber Security Cost? Common Cyber Security Expenses & Fees. Available at: https://www.provendata.com/blog/cyber-security-cost-expenses-fees/
- Net Present Value (NPV): What It Means and Steps to Calculate It. Investopedia. Available at: https://www.investopedia.com/terms/n/npv.asp
- Roser, C., Ballach, D., Langer, B., Wuttke, C.-C. (2021). A Simulation-Based Performance Comparison Between Flow Shops and Job Shops. IFIP Advances in Information and Communication Technology, 326–332. doi: https://doi.org/10.1007/978-3-030-92934-3_33
- Shukla, O. J., Soni, G., Kumar, R. (2021). SimEvents-based discrete-event simulation modelling and performance analysis for dynamic job-shop manufacturing system. International Journal of Advanced Operations Management, 13 (2), 167. doi: https://doi.org/10.1504/ijaom.2021.116137
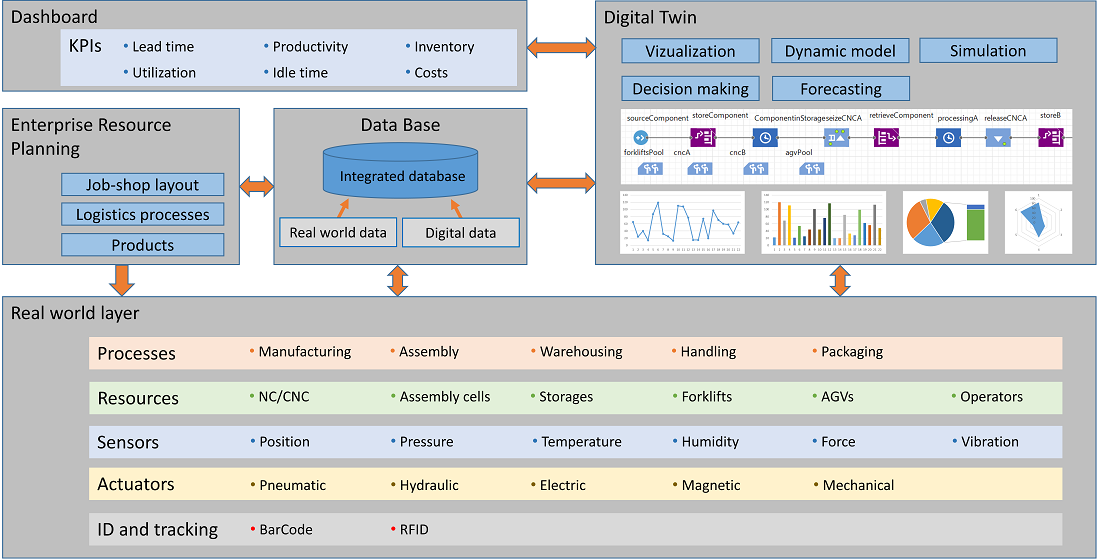
Downloads
Published
How to Cite
Issue
Section
License
Copyright (c) 2023 Kristof Banyai, Laszlo Kovacs

This work is licensed under a Creative Commons Attribution 4.0 International License.
The consolidation and conditions for the transfer of copyright (identification of authorship) is carried out in the License Agreement. In particular, the authors reserve the right to the authorship of their manuscript and transfer the first publication of this work to the journal under the terms of the Creative Commons CC BY license. At the same time, they have the right to conclude on their own additional agreements concerning the non-exclusive distribution of the work in the form in which it was published by this journal, but provided that the link to the first publication of the article in this journal is preserved.
A license agreement is a document in which the author warrants that he/she owns all copyright for the work (manuscript, article, etc.).
The authors, signing the License Agreement with TECHNOLOGY CENTER PC, have all rights to the further use of their work, provided that they link to our edition in which the work was published.
According to the terms of the License Agreement, the Publisher TECHNOLOGY CENTER PC does not take away your copyrights and receives permission from the authors to use and dissemination of the publication through the world's scientific resources (own electronic resources, scientometric databases, repositories, libraries, etc.).
In the absence of a signed License Agreement or in the absence of this agreement of identifiers allowing to identify the identity of the author, the editors have no right to work with the manuscript.
It is important to remember that there is another type of agreement between authors and publishers – when copyright is transferred from the authors to the publisher. In this case, the authors lose ownership of their work and may not use it in any way.