Devising an approach to safety management of vessel control through the identification of navigator’s state
DOI:
https://doi.org/10.15587/1729-4061.2023.286156Keywords:
safety management, intelligent system, motivation, identification, p-adic systems, neural networksAbstract
The object of this study is the processes of automated management of maritime safety by analyzing the manifestations of the human factor of sea navigators.
The task solved is justified by the need for formal and logical analysis and intelligent identification of mental motivational states (MMS) of marine navigators whose actions can cause dangerous situations during the control of the ship’s movement. High accident rates due to the fault of the navigators, in the absence of automated means of monitoring their condition, cause a contradiction between the existing means of safety control in controlling the movement of the vessel and the modern requirements of navigation, which needs to be resolved.
A safety management approach was devised that takes into account the specificity of navigational tasks and the p-adic classification of dangerous MMS for navigators. This has made it possible to create three security modes that are activated depending on the detected state of the navigator’s MMS.
Features of the results are the combination of analysis by means of p-adic systems and intelligent methods of data processing. As a result, sufficient identification accuracy was obtained for more than 75 % of MMS through neural network training.
Experimental data collected during the navigation watch, as well as on the Navi Trainer 5000 navigation simulator (Wärtsilä Corporation, Finland), became the basis for simulation by means of neural networks. In turn, the training of neural networks made it possible to obtain sufficient identification accuracy by performing up to 3000 iterations. Overall, the learning rate of the neural network was 0.98, which indicates a high level of identification.
From a practical point of view, the results could be used for the automated management of shipping safety, as well as for evaluating the level of adaptation of the navigator to dynamically changing conditions. The proposed approach provides opportunities for the application of modern intelligent technologies in the field of maritime transport safety, namely artificial neural network tools that determine notification modes or activation of automatic ship traffic control modules.
The specified contradiction requires the design of specialized systems for automated safety management of ship traffic control based on the identified states of navigators
References
- Antão, P., Soares, C. G. (2019). Analysis of the influence of human errors on the occurrence of coastal ship accidents in different wave conditions using Bayesian Belief Networks. Accident Analysis & Prevention, 133, 105262. doi: https://doi.org/10.1016/j.aap.2019.105262
- Nosov, P., Zinchenko, S., Popovych, I., Safonov, M., Palamarchuk, I., Blakh, V. (2020). Decision support during the vessel control at the time of negative manifestation of human factor. Computer Modeling and Intelligent Systems, 2608, 12–26. doi: https://doi.org/10.32782/cmis/2608-2
- Plokhikh V. V., Yanovska S. G. (2022). Sex differentiation in the organization of emergency sensorimotor action. Insight: The Psychological Dimensions of Society, 7, 24–39. doi: https://doi.org/10.32999/2663-970x/2022-7-3
- Popovych, I. S., Blynova, O. Ye., Aleksieieva, M. I., Nosov, P. S., Zavatska, N. Ye., Smyrnova, O. O. (2019). Research of Relationship between the Social Expectations and Professional Training of Lyceum Students studying in the Field of Shipbuilding. Revista ESPACIOS, 40 (33). Available at: http://ekhsuir.kspu.edu/bitstream/handle/123456789/9358/5.pdf?sequence=1&isAllowed=y
- Solovey, O., Ben, A., Dudchenko, S., Nosov, P. (2020). Development of control model for loading operations on heavy lift vessels based on inverse algorithm. Eastern-European Journal of Enterprise Technologies, 5 (2 (107)), 48–56. doi: https://doi.org/10.15587/1729-4061.2020.214856
- Dinis, D., Teixeira, A. P., Guedes Soares, C. (2020). Probabilistic approach for characterising the static risk of ships using Bayesian networks. Reliability Engineering & System Safety, 203, 107073. doi: https://doi.org/10.1016/j.ress.2020.107073
- Makarowski, R., Plopa, M., Piotrowski, A., Plopa, W. (2020). The Human Factor in Maritime Transport: Personality and Aggression Levels of Master Mariners and Navigation Students. Advances in Cognitive Psychology, 16 (4), 363–369. doi: https://doi.org/10.5709/acp-0310-5
- Nosov, P., Ben, A., Zinchenko, S., Popovych, I., Mateichuk, V., Nosova, H. (2020). Formal approaches to identify cadet fatigue factors by means of marine navigation simulators. In: CEUR Workshop Proceedings, 2732, 823–838. Available at: https://ksma.ks.ua/wp-content/uploads/2021/03/2-LW.pdf
- Li, W., Zhong, L., Xu, Y., Shi, G. (2022). Collision Risk Index Calculation Based on an Improved Ship Domain Model. Journal of Marine Science and Engineering, 10 (12), 2016. doi: https://doi.org/10.3390/jmse10122016
- Hoem, Å. S., Fjortoft, K., Rødseth, Ø. J. (2019). Addressing the Accidental Risks of Maritime Transportation: Could Autonomous Shipping Technology Improve the Statistics? TransNav, the International Journal on Marine Navigation and Safety of Sea Transportation, 13 (3), 487–494. doi: https://doi.org/10.12716/1001.13.03.01
- Man, Y., Lundh, M., Porathe, T., MacKinnon, S. (2015). From Desk to Field - Human Factor Issues in Remote Monitoring and Controlling of Autonomous Unmanned Vessels. Procedia Manufacturing, 3, 2674–2681. doi: https://doi.org/10.1016/j.promfg.2015.07.635
- Zhang, X., Chen, W., Xi, Y., Hu, S., Tang, L. (2020). Dynamics Simulation of the Risk Coupling Effect between Maritime Pilotage Human Factors under the HFACS Framework. Journal of Marine Science and Engineering, 8 (2), 144. doi: https://doi.org/10.3390/jmse8020144
- Wróbel, K., Gil, M., Chae, C.-J. (2021). On the Influence of Human Factors on Safety of Remotely-Controlled Merchant Vessels. Applied Sciences, 11 (3), 1145. doi: https://doi.org/10.3390/app11031145
- Wang, X., Zhang, B., Zhao, X., Wang, L., Tong, R. (2020). Exploring the Underlying Causes of Chinese Eastern Star, Korean Sewol, and Thai Phoenix Ferry Accidents by Employing the HFACS-MA. International Journal of Environmental Research and Public Health, 17 (11), 4114. doi: https://doi.org/10.3390/ijerph17114114
- Hasanspahić, N., Vujičić, S., Frančić, V., Čampara, L. (2021). The Role of the Human Factor in Marine Accidents. Journal of Marine Science and Engineering, 9 (3), 261. doi: https://doi.org/10.3390/jmse9030261
- Pietrzykowski, Z., Wielgosz, M., Breitsprecher, M. (2020). Navigators’ Behavior Analysis Using Data Mining. Journal of Marine Science and Engineering, 8 (1), 50. doi: https://doi.org/10.3390/jmse8010050
- Lin, W.-C., Cheng, H.-H. (2021). Enhancing marine administrative management based on human factor through safety criteria. Journal of Marine Science and Technology, 29 (3), 268–279. doi: https://doi.org/10.51400/2709-6998.1432
- Progoulakis, I., Rohmeyer, P., Nikitakos, N. (2021). Cyber Physical Systems Security for Maritime Assets. Journal of Marine Science and Engineering, 9 (12), 1384. doi: https://doi.org/10.3390/jmse9121384
- Zhuravlova L. P., Lytvynchuk A. I., Grechukha I. A., Bedny I. S. (2023). Subclinical personal correlates of psychological safety. Insight: The Psychological Dimensions of Society, 9, 94–111. doi: https://doi.org/10.32999/ksu2663-970x/2023-9-6
- Zinchenko, S., Tovstokoryi, O., Ben, A., Nosov, P., Popovych, I., Nahrybelnyi, Y. (2021). Automatic Optimal Control of a Vessel with Redundant Structure of Executive Devices. Lecture Notes on Data Engineering and Communications Technologies, 266–281. doi: https://doi.org/10.1007/978-3-030-82014-5_18
- Zinchenko, S., Tovstokoryi, O., Nosov, P., Popovych, I., Kobets, V., Abramov, G. (2020). Mathematical support of the vessel information and risk control systems. In: CEUR Workshop Proceedings, 2805, 335–354. Available at: http://ceur-ws.org/Vol-2805/paper25.pdf
- Rakić, T., Živković, S., Veljković, M. (2017). Hierarchy of work motives and motivators with the aim of forming more efficient working environment. Facta Universitatis, Series: Working and Living Environmental Protection, 087. doi: https://doi.org/10.22190/fuwlep1701087r
- Nosov, P., Zinchenko, S., Plokhikh, V., Popovych, I., Prokopchuk, Y., Makarchuk, D. et al. (2021). Development and experimental study of analyzer to enhance maritime safety. Eastern-European Journal of Enterprise Technologies, 4 (3 (112)), 27–35. doi: https://doi.org/10.15587/1729-4061.2021.239093
- Baksh, A.-A., Khan, F., Gadag, V., Ferdous, R. (2015). Network based approach for predictive accident modelling. Safety Science, 80, 274–287. doi: https://doi.org/10.1016/j.ssci.2015.08.003
- Khraban, T. E., Silko, O. V. (2022). Combat and military-professional stress: the influence of emotions and emotional states on the choice of coping strategies. Insight: The Psychological Dimensions of Society, 8, 71–87. doi: https://doi.org/10.32999/2663-970x/2022-8-6
- Mamenko, P., Zinchenko, S., Kobets, V., Nosov, P., Popovych, I. (2021). Solution of the Problem of Optimizing Route with Using the Risk Criterion. Lecture Notes on Data Engineering and Communications Technologies, 252–265. doi: https://doi.org/10.1007/978-3-030-82014-5_17
- Khrennikov, A. (2004). Modeling thinking processes in p-adic coordinate systems. Moscow: Fizmatlit, 296.
- Popovych, I., Blynova, O. (2019). The Structure, Variables and Interdependence of the Factors of Mental States of Expectations in Students’ Academic and Professional Activities. The New Educational Review, 55 (1), 293–306. doi: https://doi.org/10.15804/tner.2019.55.1.24
- Popovych, I., Hoi, N., Koval, I., Vorobel, M., Semenov, O., Semenova, N., Hrys, A. (2022). Strengthening of student youth’s mental health using play sports. Journal of Physical Education and Sport, 22 (6), 1384–1395. doi: https://doi.org/10.7752/jpes.2022.06174
- Popovych, I., Halian, I., Lialiuk, G., Chopyk, R., Karpenko, Ye., Melnyk, Yu. (2022). Research of young female handball players’ self-actualizing mental states. Journal of Physical Education and Sport, 22 (7), 1599–1607. doi: https://doi.org/10.7752/jpes.2022.07201
- Murtagh, F. (2016). Sparse p-adic data coding for computationally efficient and effective big data analytics. P-Adic Numbers, Ultrametric Analysis, and Applications, 8 (3), 236–247. doi: https://doi.org/10.1134/s2070046616030055
- Amit, D. J. (1990). Attractor neural networks and biological reality: associative memory and learning. Future Generation Computer Systems, 6 (2), 111–119. doi: https://doi.org/10.1016/0167-739x(90)90027-b
- Bradley, P. E. (2009). On p-adic classification. P-Adic Numbers, Ultrametric Analysis, and Applications, 1 (4), 271–285. doi: https://doi.org/10.1134/s2070046609040013
- Serhii, Z., Oleh, T., Pavlo, N., Ihor, P., Kostiantyn, K. (2022). Pivot Point position determination and its use for manoeuvring a vessel. Ships and Offshore Structures, 18 (3), 358–364. doi: https://doi.org/10.1080/17445302.2022.2052480
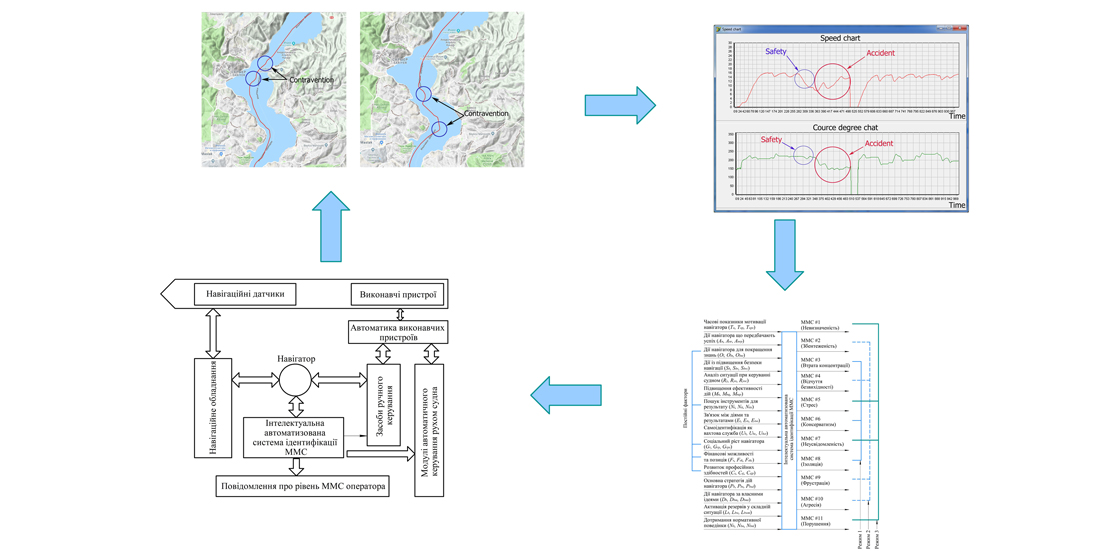
Downloads
Published
How to Cite
Issue
Section
License
Copyright (c) 2023 Pavlo Nosov, Oleksiy Koretsky, Serhii Zinchenko, Yurii Prokopchuk, Igor Gritsuk, Ihor Sokol, Kostiantyn Kyrychenko

This work is licensed under a Creative Commons Attribution 4.0 International License.
The consolidation and conditions for the transfer of copyright (identification of authorship) is carried out in the License Agreement. In particular, the authors reserve the right to the authorship of their manuscript and transfer the first publication of this work to the journal under the terms of the Creative Commons CC BY license. At the same time, they have the right to conclude on their own additional agreements concerning the non-exclusive distribution of the work in the form in which it was published by this journal, but provided that the link to the first publication of the article in this journal is preserved.
A license agreement is a document in which the author warrants that he/she owns all copyright for the work (manuscript, article, etc.).
The authors, signing the License Agreement with TECHNOLOGY CENTER PC, have all rights to the further use of their work, provided that they link to our edition in which the work was published.
According to the terms of the License Agreement, the Publisher TECHNOLOGY CENTER PC does not take away your copyrights and receives permission from the authors to use and dissemination of the publication through the world's scientific resources (own electronic resources, scientometric databases, repositories, libraries, etc.).
In the absence of a signed License Agreement or in the absence of this agreement of identifiers allowing to identify the identity of the author, the editors have no right to work with the manuscript.
It is important to remember that there is another type of agreement between authors and publishers – when copyright is transferred from the authors to the publisher. In this case, the authors lose ownership of their work and may not use it in any way.