Evaluating the effectiveness of precision farming technologies in the activities of agricultural enterprises
DOI:
https://doi.org/10.15587/1729-4061.2024.298478Keywords:
efficiency of agricultural enterprises, yield of agricultural crops, smart agribusiness, precision farming technologiesAbstract
This paper describes approaches to improving the efficiency of agricultural enterprises. It has been established that the use of technology transfer, in particular, precision farming technologies, makes it possible to enhance the efficiency of agricultural enterprises, in particular, to increase the yield of agricultural crops and, accordingly, to improve the profitability of enterprises. As an example, the activities of agricultural enterprises in the Republic of Kazakhstan were analyzed. The share of agricultural enterprises in the Republic of Kazakhstan that use elements of precision farming technology in their activities was determined. It was established that since 2019, the use of precision farming technologies in the activities of agricultural enterprises in the Republic of Kazakhstan has intensified. At the same time, from 60 to 75 % of enterprises already use elements of precision farming technologies in their activities. Using crop yield data for the past 32 years, estimates of the effectiveness of using precision farming technologies by agro-enterprises in the Republic of Kazakhstan were constructed based on forecasting yield indicators using the linear-weighted moving average method. The efficiency of using precision farming technologies in the activities of agricultural enterprises in the Republic of Kazakhstan in 2022 reached 8.46 %, and the average efficiency for the period 2019–2022 was 4.21 %. Therefore, the use of precision farming technologies makes it possible to improve the validity of decision-making in the management of an agricultural enterprise and to obtain a higher profit from the sale of produced agricultural products for any agricultural enterprise in the world. On average, the results allow us to estimate the possible profit of an agro-enterprise when growing agricultural crops in the case of using precision farming technology
References
- Schauberger, B., Jägermeyr, J., Gornott, C. (2020). A systematic review of local to regional yield forecasting approaches and frequently used data resources. European Journal of Agronomy, 120, 126153. https://doi.org/10.1016/j.eja.2020.126153
- Saiz-Rubio, V., Rovira-Más, F. (2020). From Smart Farming towards Agriculture 5.0: A Review on Crop Data Management. Agronomy, 10 (2), 207. https://doi.org/10.3390/agronomy10020207
- Romanovska, P., Schauberger, B., Gornott, C. (2023). Wheat yields in Kazakhstan can successfully be forecasted using a statistical crop model. European Journal of Agronomy, 147, 126843. https://doi.org/10.1016/j.eja.2023.126843
- Sadenova, M. A., Beisekenov, N. A., Rakhymberdina, M. Y., Varbanov, P. S., Klemeš, J. J. (2021). Mathematical modelling in crop production to predict crop yields. Chemical Engineering Transactions, 88, 1225–1230. https://doi.org/10.3303/CET2188204
- Beisekenov, N. A., Sadenova, M. A., Varbanov, P. S. (2021). Mathematical Optimization as A Tool for the Development of "Smart" Agriculture in Kazakhstan. Chemical Engineering Transactions, 88, 1219–1224. https://doi.org/10.3303/CET2188203
- Cai, Y., Guan, K., Lobell, D., Potgieter, A. B., Wang, S., Peng, J. et al. (2019). Integrating satellite and climate data to predict wheat yield in Australia using machine learning approaches. Agricultural and Forest Meteorology, 274, 144–159. https://doi.org/10.1016/j.agrformet.2019.03.010
- Mimenbayeva, A., Yessen, A., Nurbekova, A., Suleimenova, R., Ospanova, T., Kasymova, A., Niyazova, R. (2023). Development of a linear regression model based on vegetation indices of agricultural crops. Scientific Journal of Astana IT University, 15 (15), 101–110. https://doi.org/10.37943/15emub4283
- Ji, C., Andrashko, Y., Biloshchytska, S., Tsiutsiura, S. (2021). Conceptual Research Model of Developing the Decision Support System for Agriculture Under Uncertainty. 2021 IEEE International Conference on Smart Information Systems and Technologies (SIST). https://doi.org/10.1109/sist50301.2021.9465888
- Chunmei, J. (2022). Risk management for the functionality component of an agricultural enterprise’s decision-making support information system. Management of Development of Complex Systems, 52, 35–38. https://doi.org/10.32347/2412-9933.2022.52.35-38
- Xue, J., Su, B. (2017). Significant Remote Sensing Vegetation Indices: A Review of Developments and Applications. Journal of Sensors, 2017, 1–17. https://doi.org/10.1155/2017/1353691
- Mutanga, O., Masenyama, A., Sibanda, M. (2023). Spectral saturation in the remote sensing of high-density vegetation traits: A systematic review of progress, challenges, and prospects. ISPRS Journal of Photogrammetry and Remote Sensing, 198, 297–309. https://doi.org/10.1016/j.isprsjprs.2023.03.010
- Aharon‐Rotman, Y., McEvoy, J., Zhaoju, Z., Yu, H., Wang, X., Si, Y. et al. (2017). Water level affects availability of optimal feeding habitats for threatened migratory waterbirds. Ecology and Evolution, 7 (23), 10440–10450. https://doi.org/10.1002/ece3.3566
- Boyko, R., Shumyhai, D., Gladka, M. (2016). Сoncept, Definition and Use of an Agent in the Multi-agent Information Management Systems at the Objects of Various Nature. Advances in Intelligent Systems and Computing, 59–63. https://doi.org/10.1007/978-3-319-48923-0_8
- Kuchansky, A., Biloshchytskyi, A., Andrashko, Y., Biloshchytska, S., Honcharenko, T., Nikolenko, V. (2019). Fractal Time Series Analysis in Non-Stationary Environment. 2019 IEEE International Scientific-Practical Conference Problems of Infocommunications, Science and Technology (PIC S&T). https://doi.org/10.1109/picst47496.2019.9061554
- Kuchansky, A., Biloshchytskyi, A., Andrashko, Y., Biloshchytska, S., Shabala, Y., Myronov, O. (2018). Development of adaptive combined models for predicting time series based on similarity identification. Eastern-European Journal of Enterprise Technologies, 1 (4 (91)), 32–42. https://doi.org/10.15587/1729-4061.2018.121620
- Aubakirova, G., Ivel, V., Gerassimova, Y., Moldakhmetov, S., Petrov, P. (2022). Application of artificial neural network for wheat yield forecasting. Eastern-European Journal of Enterprise Technologies, 3 (4 (117)), 31–39. https://doi.org/10.15587/1729-4061.2022.259653
- Bureau of National Statistics of the Agency for Strategic Planning and Reforms of the Republic of Kazakhstan. Available at: https://www.gov.kz/memleket/entities/stat?lang=en
- Aerospace committee of the Ministry of Digital Development, Innovations and Aerospace Industry of the Republic of Kazakhstan. Available at: https://www.gov.kz/memleket/entities/kazcosmos/press/article/details/1502?lang=ru
- Transfer and adaptation of precision farming technologies in crop production on the principle of "demonstration farms (polygons)" in Akmola region. Report on research work. LLP "A.I. Baraev Scientific and Production Center of Grain Farming" (2020). Shortandy, 240.
- Pilot farm of oil plants. Available at: https://eldala.kz/dannye/kompanii/346-opytnoe-hozyajstvo-maslichnyh-kultur
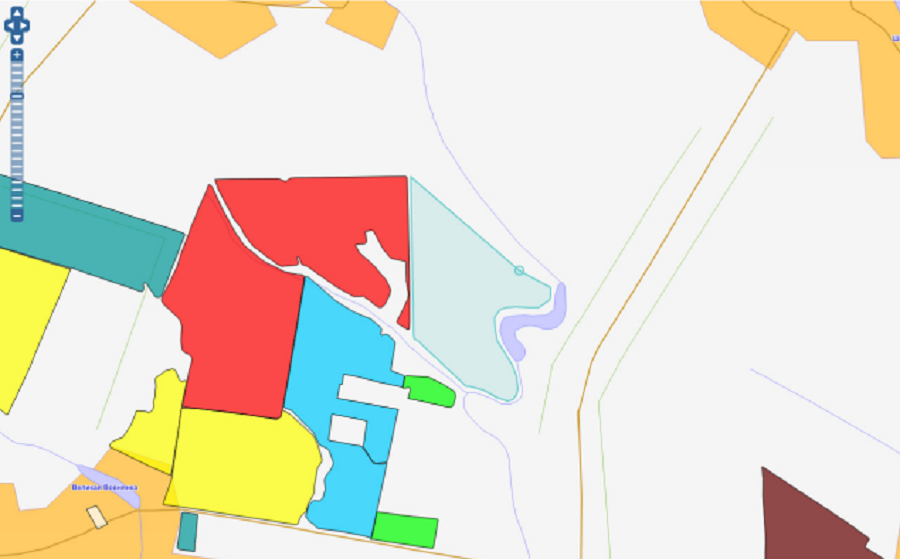
Downloads
Published
How to Cite
Issue
Section
License
Copyright (c) 2024 Alexandr Neftissov, Andrii Biloshchytskyi, Yurii Andrashko, Oleksandr Kuchanskyi, Volodymyr Vatskel, Sapar Toxanov, Myroslava Gladka

This work is licensed under a Creative Commons Attribution 4.0 International License.
The consolidation and conditions for the transfer of copyright (identification of authorship) is carried out in the License Agreement. In particular, the authors reserve the right to the authorship of their manuscript and transfer the first publication of this work to the journal under the terms of the Creative Commons CC BY license. At the same time, they have the right to conclude on their own additional agreements concerning the non-exclusive distribution of the work in the form in which it was published by this journal, but provided that the link to the first publication of the article in this journal is preserved.
A license agreement is a document in which the author warrants that he/she owns all copyright for the work (manuscript, article, etc.).
The authors, signing the License Agreement with TECHNOLOGY CENTER PC, have all rights to the further use of their work, provided that they link to our edition in which the work was published.
According to the terms of the License Agreement, the Publisher TECHNOLOGY CENTER PC does not take away your copyrights and receives permission from the authors to use and dissemination of the publication through the world's scientific resources (own electronic resources, scientometric databases, repositories, libraries, etc.).
In the absence of a signed License Agreement or in the absence of this agreement of identifiers allowing to identify the identity of the author, the editors have no right to work with the manuscript.
It is important to remember that there is another type of agreement between authors and publishers – when copyright is transferred from the authors to the publisher. In this case, the authors lose ownership of their work and may not use it in any way.