Method for early ignition detection based on the sampling dispersion of dangerous parameter
DOI:
https://doi.org/10.15587/1729-4061.2024.299001Keywords:
early detection of fire, ignition materials, selective dispersion, hazardous parameters, gas environmentAbstract
The object of the study is the selective dispersion of dangerous parameters of the gas environment during material fires. The practical importance of research consists in using the difference of sample dispersions of dangerous parameters of the gas environment on the intervals of absence and presence of ignition of materials for detection of ignition. The theoretical substantiation of the method of detecting fires in premises based on sample dispersions of current measurements of an arbitrary dangerous parameter of the gas environment, corresponding to the general populations of reliable absence and presence of fire, has been carried out. The method, at a given level of significance, determines the unbiased uniformly most powerful fire detection rule. This makes it possible to determine how much differences in sample variances are significant with a given level of significance and are caused by ignition or are random factors. Laboratory experiments were conducted to verify the proposed method. It was established that the influence of ignition on the value of the difference in the sample dispersion at the corresponding intervals of monitoring the carbon monoxide concentration, smoke density, and temperature of the gaseous environment of the laboratory chamber is different and depends on the type of ignition material. At the same time, the minimum difference of the sample dispersions is characteristic for observing the smoke density for all the studied materials. However, early detection of ignition of alcohol, paper, wood, and textiles when observing the smoke density is carried out when the threshold is exceeded by 9.01, 5.31, 2.13 and 2.55 times, respectively. It is shown that the method of early detection of fires, which is based on the detection of significant differences in sample dispersions of data from the relevant general populations
References
- Sadkovyi, V., Andronov, V., Semkiv, O., Kovalov, A., Rybka, E., Otrosh, Yu. et. al.; Sadkovyi, V., Rybka, E., Otrosh, Yu. (Eds.) (2021). Fire resistance of reinforced concrete and steel structures. Kharkiv: РС ТЕСHNOLOGY СЕNTЕR, 180. https://doi.org/10.15587/978-617-7319-43-5
- Vambol, S., Vambol, V., Sobyna, V., Koloskov, V., Poberezhna, L. (2019). Investigation of the energy efficiency of waste utilization technology, with considering the use of low-temperature separation of the resulting gas mixtures. Energetika, 64 (4). https://doi.org/10.6001/energetika.v64i4.3893
- Popov, O., Ivaschenko, T., Markina, L., Yatsyshyn, T., Iatsyshyn, A., Lytvynenko, O. (2023). Peculiarities of Specialized Software Tools Used for Consequences Assessment of Accidents at Chemically Hazardous Facilities. Systems, Decision and Control in Energy V, 779–798. https://doi.org/10.1007/978-3-031-35088-7_45
- Popov, O., Iatsyshyn, A., Kovach, V., Iatsyshyn, A., Neklonskyi, I., Zakora, A. (2023). Is There a Future for Small Modular Reactors in Ukraine? Comparative Analysis with Large Capacity Reactors. Systems, Decision and Control in Energy V, 453–469. https://doi.org/10.1007/978-3-031-35088-7_24
- Semko, A., Rusanova, O., Kazak, O., Beskrovnaya, M., Vinogradov, S., Gricina, I. (2015). The use of pulsed high-speed liquid jet for putting out gas blow-out. The International Journal of Multiphysics, 9 (1), 9–20. https://doi.org/10.1260/1750-9548.9.1.9
- Barannik, V., Babenko, Y., Kulitsa, O., Barannik, V., Khimenko, A., Matviichuk-Yudina, O. (2020). Significant Microsegment Transformants Encoding Method to Increase the Availability of Video Information Resource. 2020 IEEE 2nd International Conference on Advanced Trends in Information Theory (ATIT). https://doi.org/10.1109/atit50783.2020.9349256
- Ragimov, S., Sobyna, V., Vambol, S., Vambol, V., Feshchenko, A., Zakora, A. et al. (2018). Physical modelling of changes in the energy impact on a worker taking into account hightemperature radiation. Journal of Achievements in Materials and Manufacturing Engineering, 1 (91), 27–33. https://doi.org/10.5604/01.3001.0012.9654
- Kovalov, A., Otrosh, Y., Rybka, E., Kovalevska, T., Togobytska, V., Rolin, I. (2020). Treatment of Determination Method for Strength Characteristics of Reinforcing Steel by Using Thread Cutting Method after Temperature Influence. Materials Science Forum, 1006, 179–184. https://doi.org/10.4028/www.scientific.net/msf.1006.179
- Vasyukov, A., Loboichenko, V., Bushtec, S. (2016). Identification of bottled natural waters by using direct conductometry. Ecology, Environment and Conservation, 22 (3), 1171–1176.
- Kondratenko, O., Vambol, S., Strokov, O., Avramenko, A. (2015). Mathematical model of the efficiency of diesel particulate matter filter. Naukovyi Visnyk Natsionalnoho Hirnychoho Universytetu, 6, 55–61.
- Pospelov, B., Kovrehin, V., Rybka, E., Krainiukov, O., Petukhova, O., Butenko, T. et al. (2020). Development of a method for detecting dangerous states of polluted atmospheric air based on the current recurrence of the combined risk. Eastern-European Journal of Enterprise Technologies, 5 (9 (107)), 49–56. https://doi.org/10.15587/1729-4061.2020.213892
- World Fire Statistics (2022). Center for Fire Statistics of CTIF, 27, 65. Available at: https://ctif.org/sites/default/files/2022-08/CTIF_Report27_ESG.pdf
- Chernukha, A., Teslenko, A., Kovalov, P., Bezuglov, O. (2020). Mathematical Modeling of Fire-Proof Efficiency of Coatings Based on Silicate Composition. Materials Science Forum, 1006, 70–75. https://doi.org/10.4028/www.scientific.net/msf.1006.70
- Pospelov, B., Rybka, E., Meleshchenko, R., Krainiukov, O., Biryukov, I., Butenko, T. et al. (2021). Short-term fire forecast based on air state gain recurrence and zero-order brown model. Eastern-European Journal of Enterprise Technologies, 3 (10 (111)), 27–33. https://doi.org/10.15587/1729-4061.2021.233606
- Davie County, NC: Fire Marshal’s Office, revision date. Standard operating guidelines. 6/5/2018. Stages of fire growth. Available at: https://www.daviecountync.gov/DocumentCenter/View/5942/500-006-Stages-of-Fire-Growth-PDF
- Gaur, A., Singh, A., Kumar, A., Kulkarni, K. S., Lala, S., Kapoor, K. et al. (2019). Fire Sensing Technologies: A Review. IEEE Sensors Journal, 19 (9), 3191–3202. https://doi.org/10.1109/jsen.2019.2894665
- Zhang, G., Li, H., Zhu, G., Li, J. (2020). Temperature fields for fire resistance analysis of structures exposed to natural fires in large space buildings. The Structural Design of Tall and Special Buildings, 29 (4). https://doi.org/10.1002/tal.1708
- Oswald, D. (2021). Homeowner vulnerability in residential buildings with flammable cladding. Safety Science, 136, 105185. https://doi.org/10.1016/j.ssci.2021.105185
- Snoonian, D. (2003). Smart buildings. IEEE Spectrum, 40 (8), 18–23. https://doi.org/10.1109/mspec.2003.1222043
- Xu, Z., Guo, Y., Saleh, J. H. (2021). Advances Toward the Next Generation Fire Detection: Deep LSTM Variational Autoencoder for Improved Sensitivity and Reliability. IEEE Access, 9, 30636–30653. https://doi.org/10.1109/access.2021.3060338
- Liu, Z. (2003). Review of Recent Developments in Fire Detection Technologies. Journal of Fire Protection Engineering, 13 (2), 129–151. https://doi.org/10.1177/1042391503013002003
- Jadon, A., Omama, M., Varshney, A., Ansari, M. S., Sharma, R. (2019). FireNet: a specialized lightweight fire & smoke detection model for real-time IoT applications. arXiv. https://doi.org/10.48550/arXiv.1905.11922
- Fire and rescue incident statistics, England, year ending December 2020. Available at: https://assets.publishing.service.gov.uk/media/609a533fe90e07357a9e250c/fire-and-rescue-incident-dec20-hosb1021.pdf
- Pospelov, B., Rybka, E., Savchenko, A., Dashkovska, O., Harbuz, S., Naden, E. et al. (2022). Peculiarities of amplitude spectra of the third order for the early detection of indoor fires. Eastern-European Journal of Enterprise Technologies, 5 (10 (119)), 49–56. https://doi.org/10.15587/1729-4061.2022.265781
- Pospelov, B., Andronov, V., Rybka, E., Skliarov, S. (2017). Research into dynamics of setting the threshold and a probability of ignition detection by selfadjusting fire detectors. Eastern-European Journal of Enterprise Technologies, 5 (9 (89)), 43–48. https://doi.org/10.15587/1729-4061.2017.110092
- Çetin, A. E., Dimitropoulos, K., Gouverneur, B., Grammalidis, N., Günay, O., Habiboǧlu, Y. H. et al. (2013). Video fire detection – Review. Digital Signal Processing, 23 (6), 1827–1843. https://doi.org/10.1016/j.dsp.2013.07.003
- Li, P., Zhao, W. (2020). Image fire detection algorithms based on convolutional neural networks. Case Studies in Thermal Engineering, 19, 100625. https://doi.org/10.1016/j.csite.2020.100625
- Ayyubi, S., Miao, Y., Shi, H. (2014). Automating standalone smoke alarms for early remote notifications. 2014 13th International Conference on Control Automation Robotics & Vision (ICARCV). https://doi.org/10.1109/icarcv.2014.7064385
- Mao, W., Wang, W., Dou, Z., Li, Y. (2018). Fire Recognition Based On Multi-Channel Convolutional Neural Network. Fire Technology, 54 (2), 531–554. https://doi.org/10.1007/s10694-017-0695-6
- Wen, Z., Xie, L., Feng, H., Tan, Y. (2019). Robust fusion algorithm based on RBF neural network with TS fuzzy model and its application to infrared flame detection problem. Applied Soft Computing, 76, 251–264. https://doi.org/10.1016/j.asoc.2018.12.019
- Geetha, S., Abhishek, C. S., Akshayanat, C. S. (2020). Machine Vision Based Fire Detection Techniques: A Survey. Fire Technology, 57 (2), 591–623. https://doi.org/10.1007/s10694-020-01064-z
- Wu, Y., Harada, T. (2004). Study on the Burning Behaviour of Plantation Wood. Scientia Silvae Sinicae, 40, 131.
- Ji, J., Yang, L., Fan, W. (2003). Experimental Study on Effects of Burning Behaviours of Materials Caused by External Heat Radiation. Journal of Combustion Science and Technology, 9, 139.
- Peng, X., Liu, S., Lu, G. (2005). Experimental Analysis on Heat Release Rate of Materials. Journal of Chongqing University, 28, 122.
- Cheng, C., Sun, F., Zhou, X. (2011). One fire detection method using neural networks. Tsinghua Science and Technology, 16 (1), 31–35. https://doi.org/10.1016/s1007-0214(11)70005-0
- Ding, Q., Peng, Z., Liu, T., Tong, Q. (2014). Multi-Sensor Building Fire Alarm System with Information Fusion Technology Based on D-S Evidence Theory. Algorithms, 7 (4), 523–537. https://doi.org/10.3390/a7040523
- Sadkovyi, V., Pospelov, B., Rybka, E., Kreminskyi, B., Yashchenko, O., Bezuhla, Y. et al. (2022). Development of a method for assessing the reliability of fire detection in premises. Eastern-European Journal of Enterprise Technologies, 3 (10 (117)), 56–62. https://doi.org/10.15587/1729-4061.2022.259493
- Pospelov, B., Rybka, E., Togobytska, V., Meleshchenko, R., Danchenko, Y., Butenko, T. et al. (2019). Construction of the method for semi-adaptive threshold scaling transformation when computing recurrent plots. Eastern-European Journal of Enterprise Technologies, 4 (10 (100)), 22–29. https://doi.org/10.15587/1729-4061.2019.176579
- Pospelov, B., Andronov, V., Rybka, E., Popov, V., Semkiv, O. (2018). Development of the method of frequencytemporal representation of fluctuations of gaseous medium parameters at fire. Eastern-European Journal of Enterprise Technologies, 2 (10 (92)), 44–49. https://doi.org/10.15587/1729-4061.2018.125926
- Pospelov, B., Andronov, V., Rybka, E., Krainiukov, O., Karpets, K., Pirohov, O. et al. (2019). Development of the correlation method for operative detection of recurrent states. Eastern-European Journal of Enterprise Technologies, 6 (4 (102)), 39–46. https://doi.org/10.15587/1729-4061.2019.187252
- Pospelov, B., Rybka, E., Meleshchenko, R., Krainiukov, O., Harbuz, S., Bezuhla, Y. et al. (2020). Use of uncertainty function for identification of hazardous states of atmospheric pollution vector. Eastern-European Journal of Enterprise Technologies, 2 (10 (104)), 6–12. https://doi.org/10.15587/1729-4061.2020.200140
- Sadkovyi, V., Pospelov, B., Andronov, V., Rybka, E., Krainiukov, O., Rud, A. et al. (2020). Construction of a method for detecting arbitrary hazard pollutants in the atmospheric air based on the structural function of the current pollutant concentrations. Eastern-European Journal of Enterprise Technologies, 6 (10 (108)), 14–22. https://doi.org/10.15587/1729-4061.2020.218714
- Pospelov, B., Andronov, V., Rybka, E., Chubko, L., Bezuhla, Y., Gordiichuk, S. et al. (2023). Revealing the peculiarities of average bicoherence of frequencies in the spectra of dangerous parameters of the gas environment during fire. Eastern-European Journal of Enterprise Technologies, 1 (10 (121)), 46–54. https://doi.org/10.15587/1729-4061.2023.272949
- Pospelov, B., Andronov, V., Rybka, E., Bezuhla, Y., Liashevska, O., Butenko, T. et al. (2022). Empirical cumulative distribution function of the characteristic sign of the gas environment during fire. Eastern-European Journal of Enterprise Technologies, 4 (10 (118)), 60–66. https://doi.org/10.15587/1729-4061.2022.263194
- Pospelov, B., Andronov, V., Rybka, E., Samoilov, M., Krainiukov, O., Biryukov, I. et al. (2021). Development of the method of operational forecasting of fire in the premises of objects under real conditions. Eastern-European Journal of Enterprise Technologies, 2 (10 (110)), 43–50. https://doi.org/10.15587/1729-4061.2021.226692
- Dubinin, D., Cherkashyn, O., Maksymov, A., Beliuchenko, D., Hovalenkov, S., Shevchenko, S., Avetisyan, V. (2020). Investigation of the effect of carbon monoxide on people in case of fire in a building. Sigurnost, 62 (4), 347–357. https://doi.org/10.31306/s.62.4.2
- Pasport. Spovishchuvach pozhezhnyi teplovyi tochkovyi. Arton. Available at: https://ua.arton.com.ua/files/passports/%D0%A2%D0%9F%D0%A2-4_UA.pdf
- Pasport. Spovishchuvach pozhezhnyi dymovyi tochkovyi optychnyi. Arton. Available at: https://ua.arton.com.ua/files/passports/spd-32_new_pas_ua.pdf
- Optical/Heat Multisensor Detector (2019). Discovery. Available at: https://www.nsc-hellas.gr/pdf/APOLLO/discovery/B02704-00%20Discovery%20Multisensor%20Heat-%20Optical.pdf
- Pospelov, B., Rybka, E., Samoilov, M., Morozov, I., Bezuhla, Y., Butenko, T. et al. (2022). Defining the features of amplitude and phase spectra of dangerous factors of gas medium during the ignition of materials in the premises. Eastern-European Journal of Enterprise Technologies, 2 (10 (116)), 57–65. https://doi.org/10.15587/1729-4061.2022.254500
- McGrattan, K., Hostikka, S., McDermott, R., Floyd, J., Weinschenk, C., Overholt, K. (2016). Fire Dynamics Simulator Technical Reference Guide. Vol. 3. National Institute of Standards and Technology.
- Floyd, J., Forney, G., Hostikka, S., Korhonen, T., McDermott, R., McGrattan, K. (2013). Fire Dynamics Simulator (Version 6) User’s Guide. Vol. 1. National Institute of Standard and Technology.
- Hogg, R. V., McKean, J. W., Craig, A. T. (2019). Introduction to mathematical statistics. Pearson, 746. Available at: https://minerva.it.manchester.ac.uk/~saralees/statbook2.pdf
- Van de Geer, S. (2010). Mathematical Statistics. Available at: https://people.math.ethz.ch/~geer/mathstat.pdf
- Ling, S. (2020). Lecture Notes on Mathematical Statistics. Available at: https://cims.nyu.edu/~sling/Math_Stats_Lecture_2020F.pdf
- Lehmann, E. L. (Ed.) (1999). Elements of Large-Sample Theory. Springer, 632. https://doi.org/10.1007/b98855
- Levin, B. R. (1966). Teoreticheskie osnovy statisticheskoy radiotehniki. Moscow: Sovetskoe radio.
- Cramér, H. (1999). Mathematical methods of statistics. Vol. 26. Princeton University Press.
- Otrosh, Y., Semkiv, O., Rybka, E., Kovalov, A. (2019). About need of calculations for the steel framework building in temperature influences conditions. IOP Conference Series: Materials Science and Engineering, 708 (1), 012065. https://doi.org/10.1088/1757-899x/708/1/012065
- Otrosh, Y., Rybka, Y., Danilin, O., Zhuravskyi, M. (2019). Assessment of the technical state and the possibility of its control for the further safe operation of building structures of mining facilities. E3S Web of Conferences, 123, 01012. https://doi.org/10.1051/e3sconf/201912301012
- Loboichenko, V. M., Vasyukov, A. E., Tishakova, T. S. (2017). Investigations of Mineralization of Water Bodies on the Example of River Waters of Ukraine. Asian Journal of Water, Environment and Pollution, 14 (4), 37–41. https://doi.org/10.3233/ajw-170035
- Vambol, S., Vambol, V., Kondratenko, O., Koloskov, V., Suchikova, Y. (2018). Substantiation of expedience of application of high-temperature utilization of used tires for liquefied methane production. Journal of Achievements in Materials and Manufacturing Engineering, 2 (87), 77–84. https://doi.org/10.5604/01.3001.0012.2830
- Pospelov, B., Rybka, E., Krainiukov, O., Yashchenko, O., Bezuhla, Y., Bielai, S. et al. (2021). Short-term forecast of fire in the premises based on modification of the Brown’s zero-order model. Eastern-European Journal of Enterprise Technologies, 4 (10 (112)), 52–58. https://doi.org/10.15587/1729-4061.2021.238555
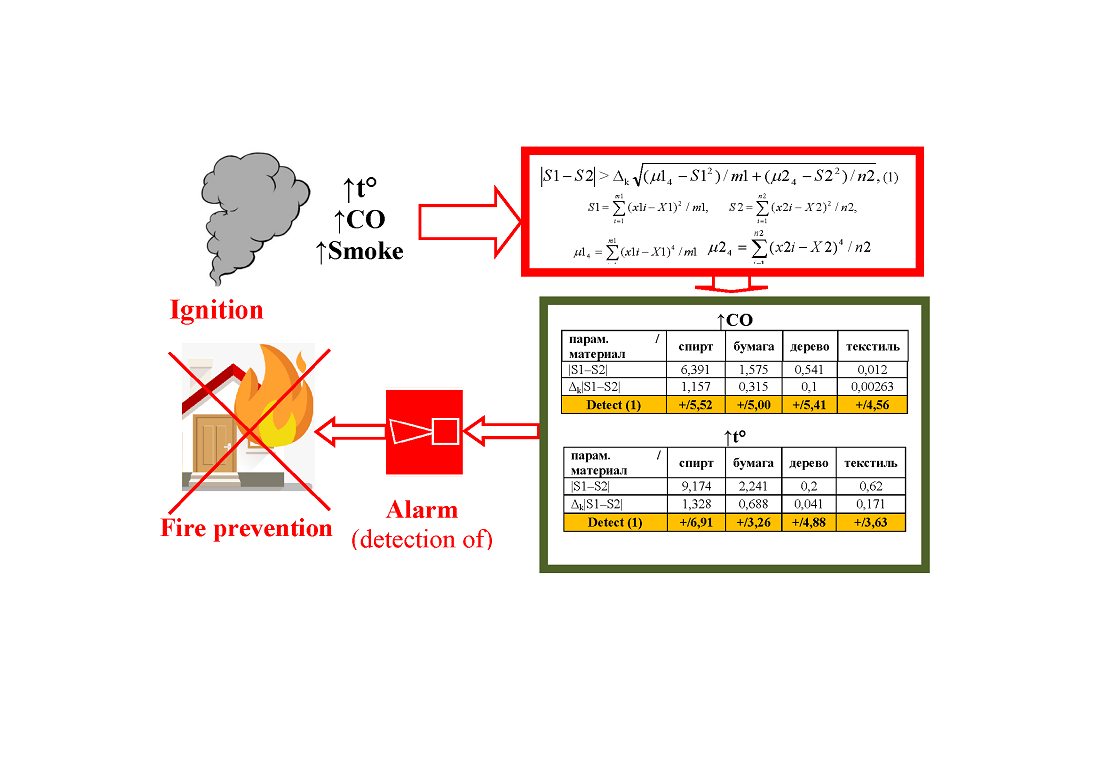
Downloads
Published
How to Cite
Issue
Section
License
Copyright (c) 2024 Boris Pospelov, Evgenіy Rybka, Olekcii Krainiukov, Vasyl Fedyna, Yuliia Bezuhla, Andrii Melnychenko, Pavlo Borodych, Svitlana Hryshko, Svyatoslav Manzhura, Olha Yesipova

This work is licensed under a Creative Commons Attribution 4.0 International License.
The consolidation and conditions for the transfer of copyright (identification of authorship) is carried out in the License Agreement. In particular, the authors reserve the right to the authorship of their manuscript and transfer the first publication of this work to the journal under the terms of the Creative Commons CC BY license. At the same time, they have the right to conclude on their own additional agreements concerning the non-exclusive distribution of the work in the form in which it was published by this journal, but provided that the link to the first publication of the article in this journal is preserved.
A license agreement is a document in which the author warrants that he/she owns all copyright for the work (manuscript, article, etc.).
The authors, signing the License Agreement with TECHNOLOGY CENTER PC, have all rights to the further use of their work, provided that they link to our edition in which the work was published.
According to the terms of the License Agreement, the Publisher TECHNOLOGY CENTER PC does not take away your copyrights and receives permission from the authors to use and dissemination of the publication through the world's scientific resources (own electronic resources, scientometric databases, repositories, libraries, etc.).
In the absence of a signed License Agreement or in the absence of this agreement of identifiers allowing to identify the identity of the author, the editors have no right to work with the manuscript.
It is important to remember that there is another type of agreement between authors and publishers – when copyright is transferred from the authors to the publisher. In this case, the authors lose ownership of their work and may not use it in any way.