Identifying the influence of land logistic driver cognitive energy impact on supply chain performance through agent-based simulation
DOI:
https://doi.org/10.15587/1729-4061.2024.302286Keywords:
cognitive energy expenditure (CEE), supply chain network (SCN), agent-based simulation, electroencephalography (EEG), logistic transportAbstract
Logistic transports link demand generators, distributors, and producers in a supply chain network (SCN). The existence of logistic transports is critical to ensure whole nodes’ economic sustainability. This study explores the impact of human factors on SCN performance through cognitive energy expenditure (CEE) tracking. Agent-based model (ABM) simulation was used to analyze the impact of CEE from truck driver’s electroencephalography (EEG) data to obtain the postsynaptic potential values, which were then transformed to calorific energy. The fleet agents, retailers and distributor models were built based on the East Java, Indonesia, logistic transport route around Karanglo, Gempol, Bungurasih, and Gubeng. The frequency and the peak value of the EEG data, postsynaptic potential, and energy data indicate the same information. All data indicate that more challenging routes have higher frequency and higher peak values. The ABM simulation of the fleet agents shows balanced CEE throughout entire routes due to the precise rest period and eat scheduling. The average delivery success rate was 8 out of 30 or 26.7 % in each simulation time step. Hence, most goods delivery tasks can be completed by fleet agents in a balanced system. As a consequence, the SCN performance is also balanced due to the fluid inventory shift without overstock and stockouts. The rest and eat periods of a fleet agent were scheduled after the CEE has been peaked. The time lag between rest periods and transport operations has to be maintained to overcome fleet agents task buildup. Task buildup has a potential to decay both transport safety and inventory shift rates. Therefore, the upgrade in SCN performance is possible through proper fleet agents scheduling
Supporting Agency
- The authors would like to acknowledge Riset Kolaborasi Indonesia (RKI) program for the funding, Brawijaya University, Sepuluh Nopember Institute of Technology and State University of Malang for their encouragements and support.
References
- Essakly, A., Wichmann, M., Spengler, T. S. (2019). A reference framework for the holistic evaluation of Industry 4.0 solutions for small-and medium-sized enterprises. IFAC-PapersOnLine, 52 (13), 427–432. https://doi.org/10.1016/j.ifacol.2019.11.093
- Wang, S., Jiang, Z., Noland, R. B., Mondschein, A. S. (2020). Attitudes towards privately-owned and shared autonomous vehicles. Transportation Research Part F: Traffic Psychology and Behaviour, 72, 297–306. https://doi.org/10.1016/j.trf.2020.05.014
- Walker, G., Strathie, A. (2016). Big data and ergonomics methods: A new paradigm for tackling strategic transport safety risks. Applied Ergonomics, 53, 298–311. https://doi.org/10.1016/j.apergo.2015.09.008
- Dunn, N., Williamson, A. (2012). Mitigating the effects of monotony. Rail Human Factors around the World, 774–782. https://doi.org/10.1201/b12742-87
- Gerstner, W., Kistler, W. M., Naud, R., Paninski, L. (2014). Neuronal Dynamics. Cambridge University Press. https://doi.org/10.1017/cbo9781107447615
- Balfe, N. (2020). Human factors applications of on-train-data-recorder (OTDR) data: an exploratory study. Cognition, Technology & Work, 23 (2), 239–253. https://doi.org/10.1007/s10111-019-00622-y
- Basacik, D., Waters, S., Reed, N. (2015). Detecting Cognitive Underload in Train Driving: A Physiological Approach. The Fifth International Rail Human Factors Conference. Available at: https://programme.exordo.com/rhf2015/delegates/presentation/42/
- Zhang, X., Li, J., Liu, Y., Zhang, Z., Wang, Z., Luo, D. et al. (2017). Design of a Fatigue Detection System for High-Speed Trains Based on Driver Vigilance Using a Wireless Wearable EEG. Sensors, 17 (3), 486. https://doi.org/10.3390/s17030486
- Kazil, J., Masad, D. Agent Based Modeling in Python with Mesa. SciPy 2015. Available at: https://www.youtube.com/watch?v=lcySLoprPMc
- Zaitsev, D. A. (2017). A generalized neighborhood for cellular automata. Theoretical Computer Science, 666, 21–35. https://doi.org/10.1016/j.tcs.2016.11.002
- Meneghan, L. L. (1976). “Life,” a fascinating game. The Arithmetic Teacher, 23 (1), 56–60. https://doi.org/10.5951/at.23.1.0056
- Joque, J. (2016). The Invention of the Object: Object Orientation and the Philosophical Development of Programming Languages. Philosophy & Technology, 29 (4), 335–356. https://doi.org/10.1007/s13347-016-0223-5
- Trigueiro de Sousa Junior, W., Barra Montevechi, J. A., de Carvalho Miranda, R., Teberga Campos, A. (2019). Discrete simulation-based optimization methods for industrial engineering problems: A systematic literature review. Computers & Industrial Engineering, 128, 526–540. https://doi.org/10.1016/j.cie.2018.12.073
- Iannino, V., Mocci, C., Vannocci, M., Colla, V., Caputo, A., Ferraris, F. (2020). An Event-Driven Agent-Based Simulation Model for Industrial Processes. Applied Sciences, 10 (12), 4343. https://doi.org/10.3390/app10124343
- Sarraj, R., Ballot, E., Pan, S., Hakimi, D., Montreuil, B. (2013). Interconnected logistic networks and protocols: simulation-based efficiency assessment. International Journal of Production Research, 52 (11), 3185–3208. https://doi.org/10.1080/00207543.2013.865853
- Xu, X., Liu, J., Li, H., Hu, J.-Q. (2014). Analysis of subway station capacity with the use of queueing theory. Transportation Research Part C: Emerging Technologies, 38, 28–43. https://doi.org/10.1016/j.trc.2013.10.010
- Jlassi, S., Tamayo, S., Gaudron, A. (2018). Simulation Applied to Urban Logistics: A State of the Art. City Logistics 3, 65–87. https://doi.org/10.1002/9781119425472.ch4
- Zhao, E., Yan, R., Li, K., Li, L., Xing, J. (2021). Learning to Play Hard Exploration Games Using Graph-Guided Self-Navigation. 2021 International Joint Conference on Neural Networks (IJCNN). https://doi.org/10.1109/ijcnn52387.2021.9534251
- Sert, E., Bar-Yam, Y., Morales, A. J. (2020). Segregation dynamics with reinforcement learning and agent based modeling. Scientific Reports, 10 (1). https://doi.org/10.1038/s41598-020-68447-8
- Li, J., Rombaut, E., Vanhaverbeke, L. (2021). A systematic review of agent-based models for autonomous vehicles in urban mobility and logistics: Possibilities for integrated simulation models. Computers, Environment and Urban Systems, 89, 101686. https://doi.org/10.1016/j.compenvurbsys.2021.101686
- de Bok, M., Tavasszy, L. (2018). An empirical agent-based simulation system for urban goods transport (MASS-GT). Procedia Computer Science, 130, 126–133. https://doi.org/10.1016/j.procs.2018.04.021
- de Bok, M., Tavasszy, L., Sebastiaan Thoen. (2022). Application of an empirical multi-agent model for urban goods transport to analyze impacts of zero emission zones in The Netherlands. Transport Policy, 124, 119–127. https://doi.org/10.1016/j.tranpol.2020.07.010
- Mnih, V., Kavukcuoglu, K., Silver, D., Rusu, A. A., Veness, J., Bellemare, M. G. et al. (2015). Human-level control through deep reinforcement learning. Nature, 518 (7540), 529–533. https://doi.org/10.1038/nature14236
- Knief, U., Forstmeier, W. (2021). Violating the normality assumption may be the lesser of two evils. Behavior Research Methods, 53 (6), 2576–2590. https://doi.org/10.3758/s13428-021-01587-5
- Sanz-Leon, P., Knock, S. A., Spiegler, A., Jirsa, V. K. (2015). Mathematical framework for large-scale brain network modeling in The Virtual Brain. NeuroImage, 111, 385–430. https://doi.org/10.1016/j.neuroimage.2015.01.002
- Hong, S. B., Jung, K.-Y. (2003). Basic Electrophysiology of the Electroencephalography. Journal of the Korean Neurological Association, 21 (3), 225–238. Available at: https://www.jkna.org/upload/pdf/200303001.pdf
- Jap, B. T., Lal, S., Fischer, P. (2011). Comparing combinations of EEG activity in train drivers during monotonous driving. Expert Systems with Applications, 38 (1), 996–1003. https://doi.org/10.1016/j.eswa.2010.07.109
- Shepherd, J. (2021). Flow and the dynamics of conscious thought. Phenomenology and the Cognitive Sciences, 21 (4), 969–988. https://doi.org/10.1007/s11097-021-09762-x
- Street, S. (2016). Neurobiology as Information Physics. Frontiers in Systems Neuroscience, 10. https://doi.org/10.3389/fnsys.2016.00090
- Schelski, M., Bradke, F. (2017). Neuronal polarization: From spatiotemporal signaling to cytoskeletal dynamics. Molecular and Cellular Neuroscience, 84, 11–28. https://doi.org/10.1016/j.mcn.2017.03.008
- Vergara, R. C., Jaramillo-Riveri, S., Luarte, A., Moënne-Loccoz, C., Fuentes, R., Couve, A., Maldonado, P. E. (2019). The Energy Homeostasis Principle: Neuronal Energy Regulation Drives Local Network Dynamics Generating Behavior. Frontiers in Computational Neuroscience, 13. https://doi.org/10.3389/fncom.2019.00049
- Shi, Z., Genack, A. Z. (2018). Diffusion in translucent media. Nature Communications, 9 (1). https://doi.org/10.1038/s41467-018-04242-4
- Fan, C., Peng, Y., Peng, S., Zhang, H., Wu, Y., Kwong, S. (2022). Detection of Train Driver Fatigue and Distraction Based on Forehead EEG: A Time-Series Ensemble Learning Method. IEEE Transactions on Intelligent Transportation Systems, 23 (8), 13559–13569. https://doi.org/10.1109/tits.2021.3125737
- Melnicuk, V., Thompson, S., Jennings, P., Birrell, S. (2021). Effect of cognitive load on drivers’ State and task performance during automated driving: Introducing a novel method for determining stabilisation time following take-over of control. Accident Analysis & Prevention, 151, 105967. https://doi.org/10.1016/j.aap.2020.105967
- Lieberman, H. R. (2007). Cognitive methods for assessing mental energy. Nutritional Neuroscience, 10 (5-6), 229–242. https://doi.org/10.1080/10284150701722273
- Burrough, P. A. (1992). Are GIS data structures too simple minded? Computers & Geosciences, 18 (4), 395–400. https://doi.org/10.1016/0098-3004(92)90068-3
- Hasan, R. A., Alhayali, R. A. I., Zaki, N. D., Ali, A. H. (2019). An adaptive clustering and classification algorithm for Twitter data streaming in Apache Spark. TELKOMNIKA (Telecommunication Computing Electronics and Control), 17 (6), 3086. https://doi.org/10.12928/telkomnika.v17i6.11711
- Rezqianita, B. L., Ardi, R. (2020). Drivers and Barriers of Industry 4.0 Adoption in Indonesian Manufacturing Industry. Proceedings of the 3rd Asia Pacific Conference on Research in Industrial and Systems Engineering 2020. https://doi.org/10.1145/3400934.3400958
- Khonsary, S. (2017). Guyton and Hall: Textbook of Medical Physiology. Surgical Neurology International, 8 (1), 275. https://doi.org/10.4103/sni.sni_327_17
- Witt, M., Kompaß, K., Wang, L., Kates, R., Mai, M., Prokop, G. (2019). Driver profiling – Data-based identification of driver behavior dimensions and affecting driver characteristics for multi-agent traffic simulation. Transportation Research Part F: Traffic Psychology and Behaviour, 64, 361–376. https://doi.org/10.1016/j.trf.2019.05.007
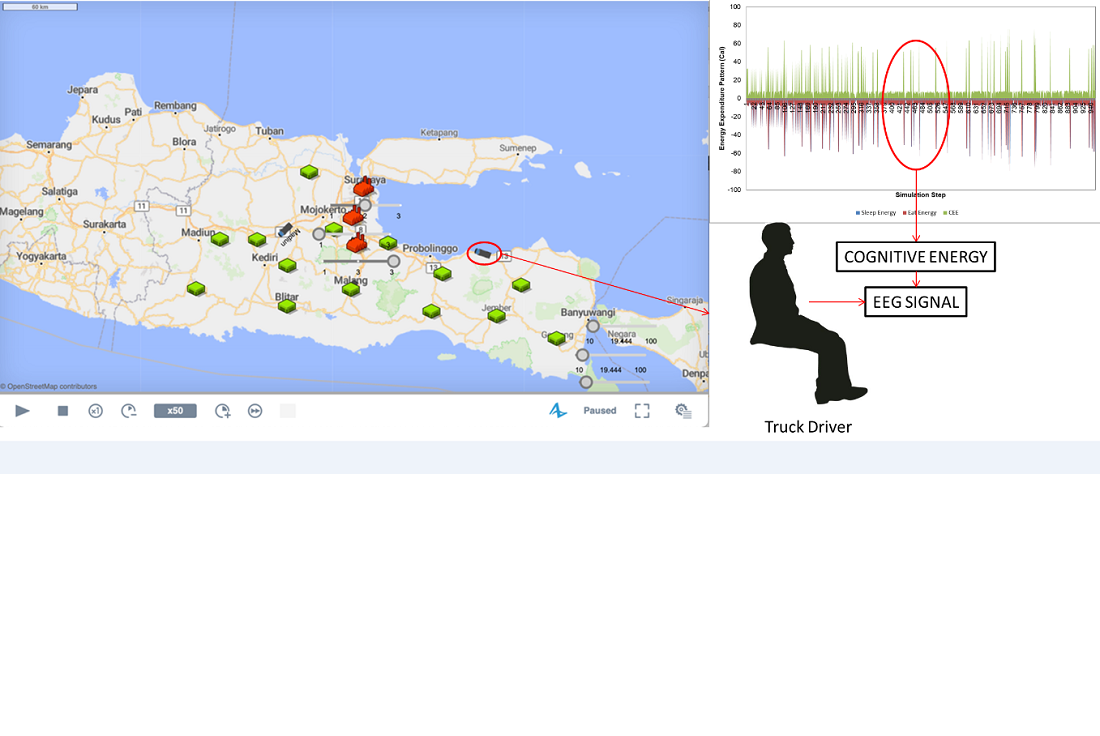
Downloads
Published
How to Cite
Issue
Section
License
Copyright (c) 2024 Ishardita Pambudi Tama, Dewi Hardiningtyas, Adithya Sudiarno, Aisyah Larasati, Willy Satrio Nugroho

This work is licensed under a Creative Commons Attribution 4.0 International License.
The consolidation and conditions for the transfer of copyright (identification of authorship) is carried out in the License Agreement. In particular, the authors reserve the right to the authorship of their manuscript and transfer the first publication of this work to the journal under the terms of the Creative Commons CC BY license. At the same time, they have the right to conclude on their own additional agreements concerning the non-exclusive distribution of the work in the form in which it was published by this journal, but provided that the link to the first publication of the article in this journal is preserved.
A license agreement is a document in which the author warrants that he/she owns all copyright for the work (manuscript, article, etc.).
The authors, signing the License Agreement with TECHNOLOGY CENTER PC, have all rights to the further use of their work, provided that they link to our edition in which the work was published.
According to the terms of the License Agreement, the Publisher TECHNOLOGY CENTER PC does not take away your copyrights and receives permission from the authors to use and dissemination of the publication through the world's scientific resources (own electronic resources, scientometric databases, repositories, libraries, etc.).
In the absence of a signed License Agreement or in the absence of this agreement of identifiers allowing to identify the identity of the author, the editors have no right to work with the manuscript.
It is important to remember that there is another type of agreement between authors and publishers – when copyright is transferred from the authors to the publisher. In this case, the authors lose ownership of their work and may not use it in any way.