Design of a decision support system to form optimal technological processes for parts machining based on artificial intelligence methods
DOI:
https://doi.org/10.15587/1729-4061.2024.306611Keywords:
automation, technological process, machining, artificial intelligence, multi-criteria statement, Pareto-optimal solutionAbstract
The object of this study is the process of designing a decision support system for automating the formation of technological processes (TPs) for machining parts of high-precision equipment for the aviation industry.
The task of improving the efficiency of the optimization of the process of mechanical processing of parts through the use of a decision support system (DSS) and artificial intelligence methods, which, unlike known analytical approaches, allow describing processes and phenomena that do not have strict formalization, has been solved. DSS consists of three subsystems. The first is an information subsystem for the automated formation of the structure in the technological process of machining parts of high-precision equipment. The second is an information subsystem for optimizing parameters of TP operations by cutting, taking into account the accumulation of tool wear. The third is a subsystem of control and adjustment of operating parameters.
In the process of conducting research, an approach was devised for designing optimal technological processes to machine parts of high-precision equipment. The task of designing the structure of technological processes was solved using production rules. The task of determining the optimal parameters of turning and milling operations was solved in a multi-criteria statement. The following objective functions were used: cost of the operation, specific energy consumption for the operation, and productivity of the operation. At the same time, the wear of the tool accumulated over time was taken into account. The solution was obtained by searching for the Pareto-optimal solution using genetic algorithms and artificial neural networks.
As a result of the work of DSS, an optimal technological process for machining parts of high-precision equipment for the aviation industry was formed, which made it possible to reduce the production time of one part by 5 % and reduce the total cost of production of the part by 14 %
References
- Kozlova, E. V. (2011). Integrated decision support system for managing the structures of production processes. Eastern-European Journal of Enterprise Technologies, 3 (12 (51)), 45–50. Available at: https://journals.uran.ua/eejet/article/view/2469
- Chandrasekaran, M., Muralidhar, M., Krishna, C. M., Dixit, U. S. (2009). Application of soft computing techniques in machining performance prediction and optimization: a literature review. The International Journal of Advanced Manufacturing Technology, 46 (5-8), 445–464. https://doi.org/10.1007/s00170-009-2104-x
- Stupnytskyy, V. (2014). Structural-parametric optimization of the technological processes for the assurance of part’s service properties. Eastern-European Journal of Enterprise Technologies, 2 (3 (68)), 9–16. https://doi.org/10.15587/1729-4061.2014.23378
- Grzesik, W. (2017). Advanced Machining Processes of Metallic Materials. Theory, Modelling, and Applications. Elsevier.
- Krasyukova, N. L., Boyko, A. N., Plotitsyna, L. A., Zudenkova, S. A., Danilkevich, M. A. (2020). Intelligent Decision-Making Technology for Choice MVC Models using Neural Networks. International Journal of Advanced Trends in Computer Science and Engineering, 9 (3), 3184–3187. https://doi.org/10.30534/ijatcse/2020/107932020
- Mamtiyev, K., Rzayeva, U. (2024). Finding and implementing the numerical solution of an optimal control problem for oscillations in a coupled objects system. Eastern-European Journal of Enterprise Technologies, 2 (2 (128)), 64–74. https://doi.org/10.15587/1729-4061.2024.301714
- Mahdi, Q. A., Shyshatskyi, A., Babenko, V., Bieliakov, R., Odarushchenko, E., Protas, N. et al. (2024). Development of a solution search method using artificial intelligence. Eastern-European Journal of Enterprise Technologies, 2 (4 (128)), 38–47. https://doi.org/10.15587/1729-4061.2024.300261
- Owaid, S. R., Zhuravskyi, Y., Lytvynenko, O., Veretnov, A., Sokolovskyi, D., Plekhova, G. et al. (2024). Development of a method of increasing the efficiency of decision-making in organizational and technical systems. Eastern-European Journal of Enterprise Technologies, 1 (4 (127)), 14–22. https://doi.org/10.15587/1729-4061.2024.298568
- Sova, O., Turinskyi, O., Shyshatskyi, A., Dudnyk, V., Zhyvotovskyi, R., Prokopenko, Y. et al. (2020). Development of an algorithm to train artificial neural networks for intelligent decision support systems. Eastern-European Journal of Enterprise Technologies, 1 (9 (103)), 46–55. https://doi.org/10.15587/1729-4061.2020.192711
- Galchonkov, O., Nevrev, A., Glava, M., Babych, M. (2020). Exploring the efficiency of the combined application of connection pruning and source data preprocessing when training a multilayer perceptron. Eastern-European Journal of Enterprise Technologies, 2 (9 (104)), 6–13. https://doi.org/10.15587/1729-4061.2020.200819
- Rao, R. V. (2011). Advanced Modeling and Optimization of Manufacturing Processes. In Springer Series in Advanced Manufacturing. Springer London. https://doi.org/10.1007/978-0-85729-015-1
- Gorbiychuk, M., Kropyvnytskyi, D., Kropyvnytska, V. (2023). Improving empirical models of complex technological objects under conditions of uncertainty. Eastern-European Journal of Enterprise Technologies, 2 (2 (122)), 53–63. https://doi.org/10.15587/1729-4061.2023.276586
- von Lücken, C., Barán, B., Brizuela, C. (2014). A survey on multi-objective evolutionary algorithms for many-objective problems. Computational Optimization and Applications. https://doi.org/10.1007/s10589-014-9644-1
- Machining Power (Full). Іscar. Available at: https://mpwr.iscar.com/MachiningPower
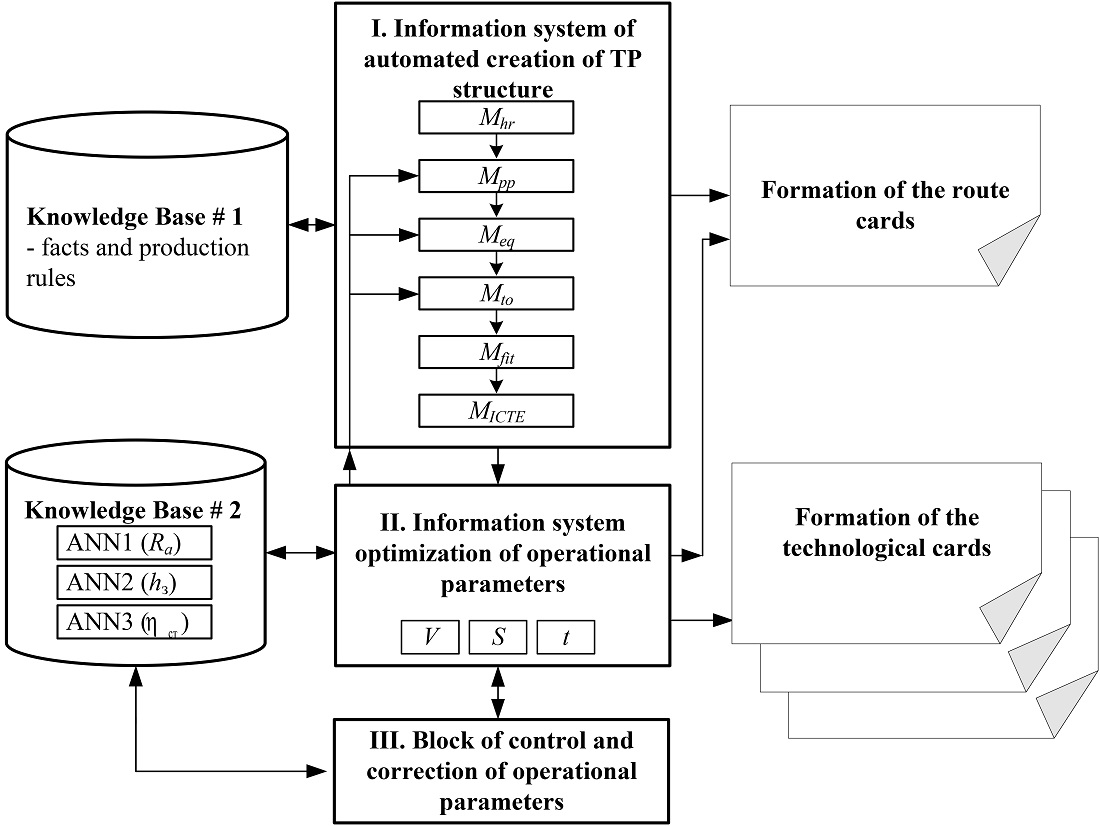
Downloads
Published
How to Cite
Issue
Section
License
Copyright (c) 2024 Viacheslav Lymarenko, Oleksandr Mozhaiev, Inna Khavina, Serhii Tiulieniev, Mykhailo Mozhaiev, Yurii Onishchenko, Yurii Gnusov, Mikhail Tsuranov, Volodymyr Homon

This work is licensed under a Creative Commons Attribution 4.0 International License.
The consolidation and conditions for the transfer of copyright (identification of authorship) is carried out in the License Agreement. In particular, the authors reserve the right to the authorship of their manuscript and transfer the first publication of this work to the journal under the terms of the Creative Commons CC BY license. At the same time, they have the right to conclude on their own additional agreements concerning the non-exclusive distribution of the work in the form in which it was published by this journal, but provided that the link to the first publication of the article in this journal is preserved.
A license agreement is a document in which the author warrants that he/she owns all copyright for the work (manuscript, article, etc.).
The authors, signing the License Agreement with TECHNOLOGY CENTER PC, have all rights to the further use of their work, provided that they link to our edition in which the work was published.
According to the terms of the License Agreement, the Publisher TECHNOLOGY CENTER PC does not take away your copyrights and receives permission from the authors to use and dissemination of the publication through the world's scientific resources (own electronic resources, scientometric databases, repositories, libraries, etc.).
In the absence of a signed License Agreement or in the absence of this agreement of identifiers allowing to identify the identity of the author, the editors have no right to work with the manuscript.
It is important to remember that there is another type of agreement between authors and publishers – when copyright is transferred from the authors to the publisher. In this case, the authors lose ownership of their work and may not use it in any way.