Determining the effectiveness of using three-dimensional printing to train computer vision systems for landmine detection
DOI:
https://doi.org/10.15587/1729-4061.2024.311602Keywords:
landmine detection, humanitarian demining, demining, unexploded ordnance, explosive remnants of war, landmine clearanceAbstract
The object of this study is the effectiveness of using three-dimensional printing to train computer vision models for landmine detection. The ongoing war in Ukraine has resulted in significant landmine contamination, particularly after russia’s full-scale invasion in 2022. Given the enormous amount of potentially landmine-contaminated land, fast and efficient demining techniques are required, as human probing and metal detectors are labor-intensive and slow-moving. Machine learning offers promising solutions to speed up the landmine detection process by deploying recognition models on robots and unmanned aerial vehicles. However, training such systems faces certain challenges. Firstly, the number of annotated data available for training is limited, which can hinder the model’s ability to generalize to real-world scenarios. Secondly, the use of real or even defused landmines is dangerous due to the potential for accidental detonation.
This study aims to overcome the problem of limited data and the risk of using real landmines. Three-dimensional printing makes it possible to create safe and diverse training data, which is essential for model performance. The model trained on replicas, achieved 98 % and 91 % precision on printed and actual landmines, respectively. This high precision is attributed to the realism of copies and the use of advanced machine learning algorithms. This approach successfully addressed the research problem due to the safety, accessibility and diversity of copies. The models trained on copies of landmines could be used in humanitarian demining operations. These operations often employ unmanned aerial vehicles or robots to identify landmines that are thrown remotely, exposed on the surface, or partially hidden
References
- Landmine Monitor 2023. Available at: https://backend.icblcmc.org/assets/reports/Landmine-Monitors/LMM2023/Downloads/Landmine-Monitor-2023_web.pdf
- Two SES cadets killed in an explosion in Kharkiv region: what is known. Available at: https://suspilne.media/kharkiv/493801-dvoe-kursantiv-dsns-zaginuli-pid-cas-vibuhu-na-harkivsini-so-vidomo
- Barnawi, A., Kumar, K., Kumar, N., Alzahrani, B., Almansour, A. (2024). A Deep Learning Approach for Landmines Detection Based on Airborne Magnetometry Imaging and Edge Computing. Computer Modeling in Engineering & Sciences, 139 (2), 2117–2137. https://doi.org/10.32604/cmes.2023.044184
- Bestagini, P., Lombardi, F., Lualdi, M., Picetti, F., Tubaro, S. (2021). Landmine Detection Using Autoencoders on Multipolarization GPR Volumetric Data. IEEE Transactions on Geoscience and Remote Sensing, 59 (1), 182–195. https://doi.org/10.1109/tgrs.2020.2984951
- Mochurad, L., Savchyn, V., Kravchenko, O. (2023). Recognition of Explosive Devices Based on the Detectors Signal Using Machine Learning Methods. Proceedings of the 4th International Workshop on Intelligent Information Technologies & Systems of Information Security. https://ceur-ws.org/Vol-3373/paper14.pdf
- Bai, X., Yang, Y., Wei, S., Chen, G., Li, H., Li, Y. et al. (2023). A Comprehensive Review of Conventional and Deep Learning Approaches for Ground-Penetrating Radar Detection of Raw Data. Applied Sciences, 13 (13), 7992. https://doi.org/10.3390/app13137992
- Lameri, S., Lombardi, F., Bestagini, P., Lualdi, M., Tubaro, S. (2017). Landmine detection from GPR data using convolutional neural networks. 2017 25th European Signal Processing Conference (EUSIPCO). https://doi.org/10.23919/eusipco.2017.8081259
- gprMax. Available at: https://www.gprmax.com
- Srimuk, P., Boonpoonga, A., Kaemarungsi, K., Athikulwongse, K., Dentri, S. (2022). Implementation of and Experimentation with Ground-Penetrating Radar for Real-Time Automatic Detection of Buried Improvised Explosive Devices. Sensors, 22 (22), 8710. https://doi.org/10.3390/s22228710
- Pryshchenko, O. A., Plakhtii, V., Dumin, O. M., Pochanin, G. P., Ruban, V. P., Capineri, L., Crawford, F. (2022). Implementation of an Artificial Intelligence Approach to GPR Systems for Landmine Detection. Remote Sensing, 14 (17), 4421. https://doi.org/10.3390/rs14174421
- Kunichik, O., Tereshchenko, V. (2023). Improving the accuracy of landmine detection using data augmentation: a comprehensive study. Artificial Intelligence, 28 (AI.2023.28 (2))), 42–54. https://doi.org/10.15407/jai2023.02.042
- Baur, J., Steinberg, G., Nikulin, A., Chiu, K., de Smet, T. S. (2020). Applying Deep Learning to Automate UAV-Based Detection of Scatterable Landmines. Remote Sensing, 12 (5), 859. https://doi.org/10.3390/rs12050859
- Barnawi, A., Kumar, krishan, Kumar, N., al zahrani, B., Almansour, A. (2023). A Graph Learning Framework for Prediction of Missing Landmines Using Airborne Magnetometry in Iot Environment. https://doi.org/10.2139/ssrn.4526746
- Kunichik, O., Tereshchenko, V. (2022). Analysis of modern methods of search and classification of explosive objects. Artificial Intelligence, 27 (AI.2022.27 (2)), 52–59. https://doi.org/10.15407/jai2022.02.052
- Pahadia, H., Lu, D., Chakravarthi, B., Yang, Y. (2023). SKoPe3D: A Synthetic Dataset for Vehicle Keypoint Perception in 3D from Traffic Monitoring Cameras. 2023 IEEE 26th International Conference on Intelligent Transportation Systems (ITSC), 28, 4367–4372. https://doi.org/10.1109/itsc57777.2023.10422667
- Didur, O. L., Shevchenko, M. S. (2023). MINY: yaki vykorystovuiutsia abo mozhut vykorystovuvatysia viyskamy rosiiskykh zaharbnykiv na sukhoputnomu teatri boiovykh diy. Konsultant: Hlokoza V.H. Aktualizovano – Lisnyk. Available at: https://shron1.chtyvo.org.ua/Didur_Oleksandr/Miny_iaki_vykorystovuiutsia_abo_mozhut_vykorystovuvatysia_viiskamy_rosiiskykh_zaharbnykiv_na_sukhopu.pdf?PHPSESSID=7r7ecak3135fa9kap9uoqltok6
- Anti-Personnel Landmines Convention. Available at: https://disarmament.unoda.org/anti-personnel-landmines-convention
- Community, B. O. (2018). Blender – a 3D modelling and rendering package, Stichting Blender Foundation, Amsterdam. Available at: http://www.blender.org
- GNU Image Manipulation Program. Available at: https://www.gimp.org/about
- Prusa Research. Available at: https://www.prusa3d.com/page/about-us_77
- PMN AP MINE (Historical Prop) by mussy is licensed under the Creative Commons - Attribution - Non-Commercial - No Derivatives license. Available at: https://www.thingiverse.com/thing:3157971
- PMN-2. by _-HB-_ is licensed under the Creative Commons - Attribution license. Available at: https://www.thingiverse.com/thing:5777217
- PMN-2 Landmine. Available at: https://mmf.io/o/98395
- OZM-72 Anti-Personnel Mine Gameready Lowpoly. Available at: https://sketchfab.com/3d-models/ozm-72-anti-personnel-mine-gameready-lowpoly-9226037a15ab47bd9fb08ed434ea1ae8
- MON-50. МОН-50. Soviet claymore shaped AP-mine. Available at: https://sketchfab.com/3d-models/mon-50-50-soviet-claymore-shaped-ap-mine-5a5da292827f41278df15e4fcee85807
- PFM-1 AP MINE (Historical Prop). Available at: https://www.thingiverse.com/thing:3660586
- Redmon, J., Divvala, S., Girshick, R., Farhadi, A. (2016). You Only Look Once: Unified, Real-Time Object Detection. 2016 IEEE Conference on Computer Vision and Pattern Recognition (CVPR). https://doi.org/10.1109/cvpr.2016.91
- Jocher, G., Qiu, J., Chaurasia, A. (2023). Ultralytics YOLO (Version 8.0.0) [Computer software]. Available at: https://github.com/ultralytics/ultralytics
- Reis, D., Kupec, J., Hong, J., Daoudi, A. (2023). Real-Time Flying Object Detection with YOLOv8. arXiv. Available at: https://doi.org/10.48550/arXiv.2305.09972
- Waite, J. R., Feng, J., Tavassoli, R., Harris, L., Tan, S. Y., Chakraborty, S., Sarkar, S. (2023). Active shooter detection and robust tracking utilizing supplemental synthetic data. arXiv. https://doi.org/10.48550/arXiv.2309.03381
- Lou, H., Liu, H., Bi, L., Liu, L., Guo, J., Gu, J. (2023). Bd-Yolo: Detection Algorithm for High-Resolution Remote Sensing Images. https://doi.org/10.2139/ssrn.4542996
- Kang, M., Ting, C.-M., Ting, F. F., Phan, R. C.-W. (2023). RCS-YOLO: A Fast and High-Accuracy Object Detector for Brain Tumor Detection. Medical Image Computing and Computer Assisted Intervention – MICCAI 2023, 600–610. https://doi.org/10.1007/978-3-031-43901-8_57
- Ang, G. J. N., Goil, A. K., Chan, H., Lew, J. J., Lee, X. C., Mustaff, R. B. A. et al. (2023). A Novel real-time arrhythmia detection model using YOLOv8. arXiv. https://doi.org/10.48550/arXiv.2305.16727
- Agarwal, V., Pichappa, A. G., Ramisetty, M., Murugan, B., Rajagopal, M. K. (2023). Suspicious Vehicle Detection Using Licence Plate Detection And Facial Feature Recognition. arXiv. https://doi.org/10.48550/arXiv.2304.14507
- Zhou, F., Deng, H., Xu, Q., Lan, X. (2023). CNTR-YOLO: Improved YOLOv5 Based on ConvNext and Transformer for Aircraft Detection in Remote Sensing Images. Electronics, 12 (12), 2671. https://doi.org/10.3390/electronics12122671
- Rublee, E., Rabaud, V., Konolige, K., Bradski, G. (2011). ORB: An efficient alternative to SIFT or SURF. 2011 International Conference on Computer Vision. https://doi.org/10.1109/iccv.2011.6126544
- Alcantarilla, P., Nuevo, J., Bartoli, A. (2013). Fast Explicit Diffusion for Accelerated Features in Nonlinear Scale Spaces. Proceedings of the British Machine Vision Conference 2013, 13.1-13.11. Available at: https://projet.liris.cnrs.fr/imagine/pub/proceedings/BMVC-2013/Papers/paper0013/paper0013.pdf
- Tan, M., Pang, R., Le, Q. V. (2020). EfficientDet: Scalable and Efficient Object Detection. 2020 IEEE/CVF Conference on Computer Vision and Pattern Recognition (CVPR). https://doi.org/10.1109/cvpr42600.2020.01079
- Carion, N., Massa, F., Synnaeve, G., Usunier, N., Kirillov, A., Zagoruyko, S. (2020). End-to-End Object Detection with Transformers. Computer Vision – ECCV 2020, 213–229. https://doi.org/10.1007/978-3-030-58452-8_13
- Zhu, X., Su, W., Lu, L., Li, B., Wang, X., Dai, J. (2020). Deformable DETR: Deformable Transformers for End-to-End Object Detection. arXiv. https://doi.org/10.48550/arXiv.2010.04159
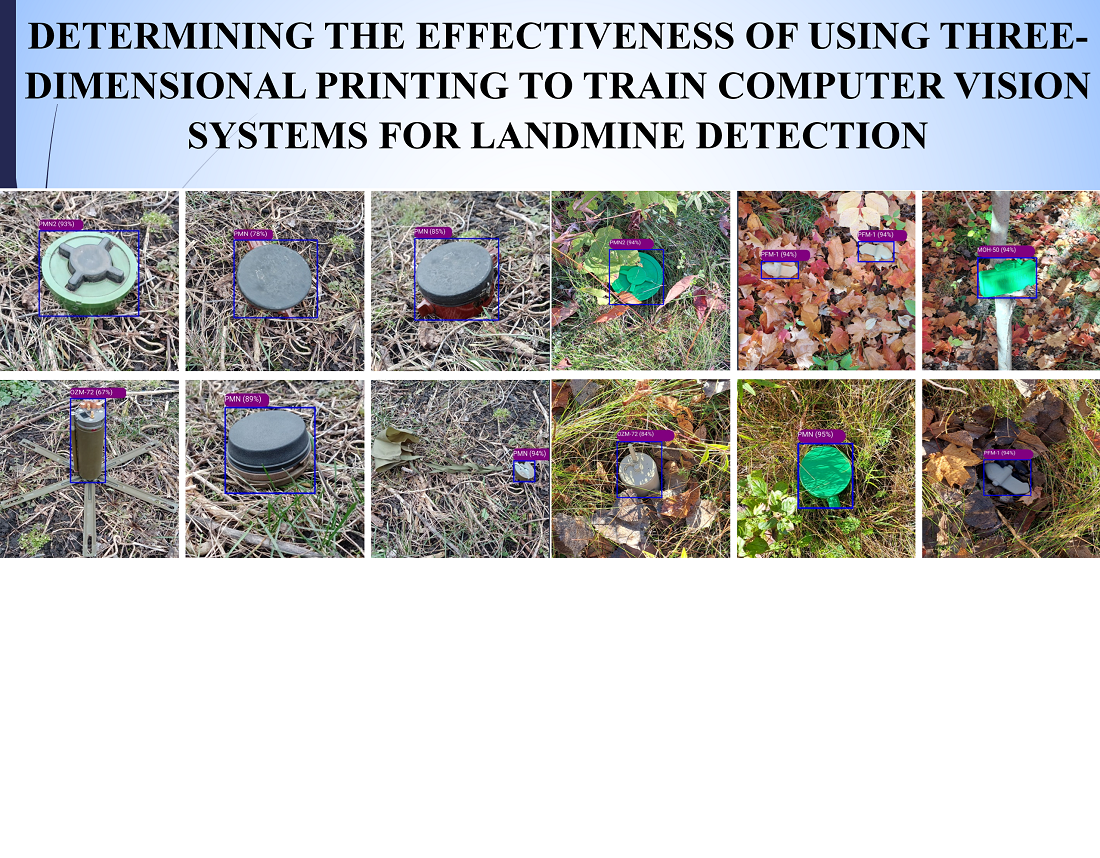
Downloads
Published
How to Cite
Issue
Section
License
Copyright (c) 2024 Oleksandr Kunichik, Vasyl Tereshchenko

This work is licensed under a Creative Commons Attribution 4.0 International License.
The consolidation and conditions for the transfer of copyright (identification of authorship) is carried out in the License Agreement. In particular, the authors reserve the right to the authorship of their manuscript and transfer the first publication of this work to the journal under the terms of the Creative Commons CC BY license. At the same time, they have the right to conclude on their own additional agreements concerning the non-exclusive distribution of the work in the form in which it was published by this journal, but provided that the link to the first publication of the article in this journal is preserved.
A license agreement is a document in which the author warrants that he/she owns all copyright for the work (manuscript, article, etc.).
The authors, signing the License Agreement with TECHNOLOGY CENTER PC, have all rights to the further use of their work, provided that they link to our edition in which the work was published.
According to the terms of the License Agreement, the Publisher TECHNOLOGY CENTER PC does not take away your copyrights and receives permission from the authors to use and dissemination of the publication through the world's scientific resources (own electronic resources, scientometric databases, repositories, libraries, etc.).
In the absence of a signed License Agreement or in the absence of this agreement of identifiers allowing to identify the identity of the author, the editors have no right to work with the manuscript.
It is important to remember that there is another type of agreement between authors and publishers – when copyright is transferred from the authors to the publisher. In this case, the authors lose ownership of their work and may not use it in any way.