Determining the influence of data on working with video materials on the accuracy of student success prediction models
DOI:
https://doi.org/10.15587/1729-4061.2024.313333Keywords:
success prediction, random forest, logistic regression, neural networks, naive BayesAbstract
The object of this study is models for predicting students’ success, constructed on the basis of machine learning methods. The paper reports results of research into the problem of improving their accuracy by expanding the data set for training the specified models. The most available are data on student actions, which are automatically collected by learning management systems. Entering additional information about students’ work increases time and resources but allows the improvement of the accuracy of the models. In the study, information about students’ work with video materials, particularly the number and duration of views, was entered into the original data set. To automate the collection of this data, the plugin for the Moodle system has been developed, which stores information about user’s actions with the video player and the duration of watching video materials in the database. Model training was carried out using Naive Bayes (NB), logistic regression (LR), random forest (RF), and neural networks (NN) algorithms with and without video data. For the models using video viewing data, accuracy increased by 10 %, balanced accuracy by 15 %, and overall performance, expressed as area under the curve (AUC), increased by 14 %. The highest prediction accuracy, with a difference of 1.8 %, was obtained by models built using RF algorithms – 87.1 % and NN – 85.3 %. At the same time, the accuracy of the models obtained by the NB and LR algorithms was 70.7 % and 76.5 %. The increase in accuracy for them was 2.3 % and 8.1 %, respectively. Analysis of calculations confirms the assumption that students’ work with educational video materials is correlated with their success. The results make it possible to find a reasonable compromise between model development costs and its accuracy at the stage of data preparation for model training.
References
- Liu, M., Yu, D. (2022). Towards intelligent E-learning systems. Education and Information Technologies, 28 (7), 7845–7876. https://doi.org/10.1007/s10639-022-11479-6
- Soloshych, I., Grynova, M., Kononets, N., Shvedchykova, I., Bunetska, I. (2021). Competence and Resource-Oriented Approaches to the Development of Digital Educational Resources. 2021 IEEE International Conference on Modern Electrical and Energy Systems (MEES), 2, 1–5. https://doi.org/10.1109/mees52427.2021.9598603
- Panasiuk, O., Akimova, L., Kuznietsova, O., Panasiuk, I. (2021). Virtual Laboratories for Engineering Education. 2021 11th International Conference on Advanced Computer Information Technologies (ACIT), 1, 637–641. https://doi.org/10.1109/acit52158.2021.9548567
- Bradley, V. M. (2020). Learning Management System (LMS) Use with Online Instruction. International Journal of Technology in Education, 4 (1), 68. https://doi.org/10.46328/ijte.36
- Gamage, S. H. P. W., Ayres, J. R., Behrend, M. B. (2022). A systematic review on trends in using Moodle for teaching and learning. International Journal of STEM Education, 9 (1). https://doi.org/10.1186/s40594-021-00323-x
- Bognár, L., Fauszt, T. (2022). Factors and conditions that affect the goodness of machine learning models for predicting the success of learning. Computers and Education: Artificial Intelligence, 3, 100100. https://doi.org/10.1016/j.caeai.2022.100100
- Rastrollo-Guerrero, J. L., Gómez-Pulido, J. A., Durán-Domínguez, A. (2020). Analyzing and Predicting Students’ Performance by Means of Machine Learning: A Review. Applied Sciences, 10 (3), 1042. https://doi.org/10.3390/app10031042
- Sáiz-Manzanares, M. C., Marticorena-Sánchez, R., García-Osorio, C. I. (2020). Monitoring Students at the University: Design and Application of a Moodle Plugin. Applied Sciences, 10 (10), 3469. https://doi.org/10.3390/app10103469
- Gaftandzhieva, S., Talukder, A., Gohain, N., Hussain, S., Theodorou, P., Salal, Y. K., Doneva, R. (2022). Exploring Online Activities to Predict the Final Grade of Student. Mathematics, 10 (20), 3758. https://doi.org/10.3390/math10203758
- Yağcı, M. (2022). Educational data mining: prediction of students’ academic performance using machine learning algorithms. Smart Learning Environments, 9 (1). https://doi.org/10.1186/s40561-022-00192-z
- Pylypenko, V., Statsenko, V. (2024). Assessment of the efficiency of the success prediction model using machine learning methods. Herald of Khmelnytskyi National University. Technical Sciences, 1 (3 (335)), 349–356. https://doi.org/10.31891/2307-5732-2024-335-3-47
- Ljubobratović, D., Matetić, M. (2020). Using LMS activity logs to predict student failure with random forest algorithm. INFuture2019: Knowledge in the Digital Age. https://doi.org/10.17234/infuture.2019.14
- Aleksandrova, Y. (2019). Predicting students performance in moodle platforms using machine learning algorithms. Conferences of the department Informatics, 1, 177–187. Available at: https://informatics.ue-varna.bg/conference19/Conf.proceedings_Informatics-50.years%20177-187.pdf
- Zacharis, N. (2016). Predicting Student Academic Performance in Blended Learning Using Artificial Neural Networks. International Journal of Artificial Intelligence & Applications, 7 (5), 17–29. https://doi.org/10.5121/ijaia.2016.7502
- Tamada, M. M., Giusti, R., Netto, J. F. de M. (2022). Predicting Students at Risk of Dropout in Technical Course Using LMS Logs. Electronics, 11 (3), 468. https://doi.org/10.3390/electronics11030468
- Althibyani, H. A. (2024). Predicting student success in MOOCs: a comprehensive analysis using machine learning models. PeerJ Computer Science, 10, e2221. https://doi.org/10.7717/peerj-cs.2221
- Jabbar, H. K., Khan, R. Z. (2014). Methods to Avoid Over-Fitting and Under-Fitting in Supervised Machine Learning (Comparative Study). Computer Science, Communication and Instrumentation Devices, 163–172. https://doi.org/10.3850/978-981-09-5247-1_017
- Géron, A. (2022). Hands-on machine learning with Scikit-Learn, Keras, and TensorFlow. O'Reilly Media, Inc.
- Sial, A. H., Rashdi, S. Y. S., Khan, A. H. (2021). Comparative Analysis of Data Visualization Libraries Matplotlib and Seaborn in Python. International Journal of Advanced Trends in Computer Science and Engineering, 10 (1), 277–281. https://doi.org/10.30534/ijatcse/2021/391012021
- Sapre, A., Vartak, S. (2020). Scientific Computing and Data Analysis using NumPy and Pandas. International Research Journal of Engineering and Technology, 7, 1334–1346.
- Krstinić, D., Braović, M., Šerić, L., Božić-Štulić, D. (2020). Multi-label Classifier Performance Evaluation with Confusion Matrix. Computer Science & Information Technology. https://doi.org/10.5121/csit.2020.100801
- Lavazza, L., Morasca, S., Rotoloni, G. (2023). On the Reliability of the Area Under the ROC Curve in Empirical Software Engineering. Proceedings of the 27th International Conference on Evaluation and Assessment in Software Engineering. https://doi.org/10.1145/3593434.3593456
- Bowers, A. J., Zhou, X. (2019). Receiver Operating Characteristic (ROC) Area Under the Curve (AUC): A Diagnostic Measure for Evaluating the Accuracy of Predictors of Education Outcomes. Journal of Education for Students Placed at Risk (JESPAR), 24 (1), 20–46. https://doi.org/10.1080/10824669.2018.1523734
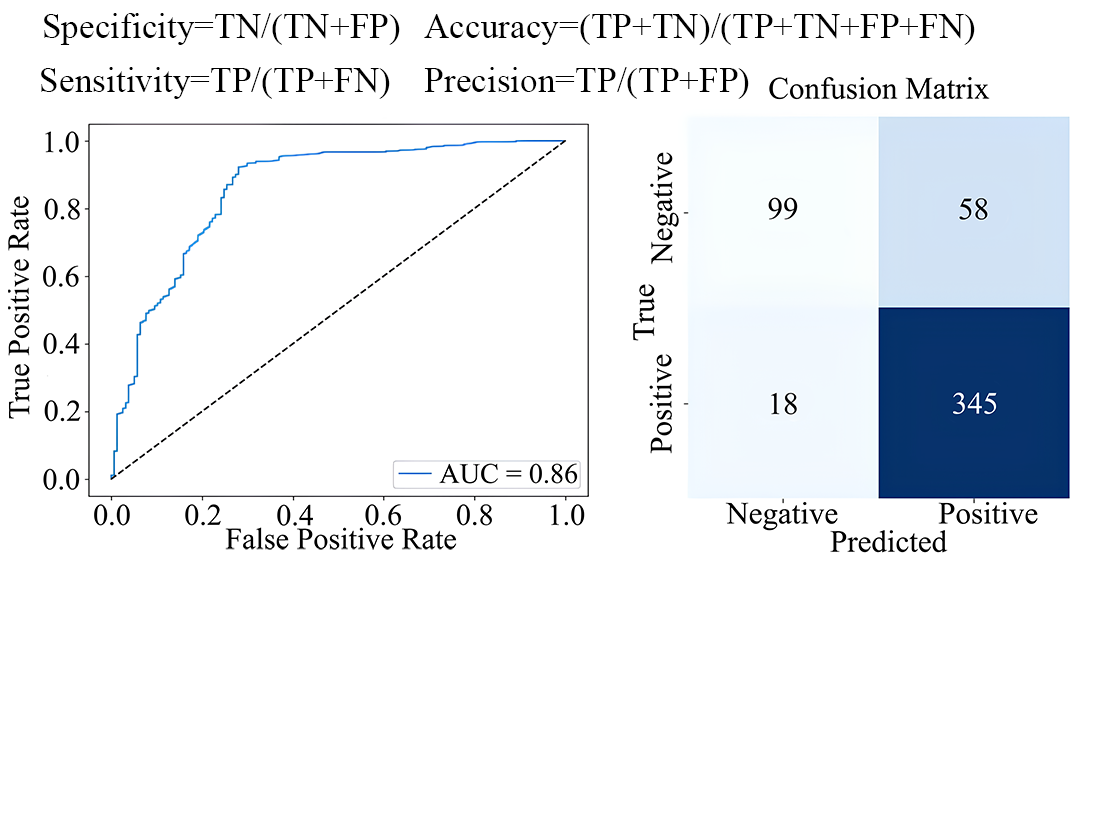
Downloads
Published
How to Cite
Issue
Section
License
Copyright (c) 2024 Vladyslav Pylypenko, Volodymyr Statsenko, Tetiana Bila, Dmytro Statsenko

This work is licensed under a Creative Commons Attribution 4.0 International License.
The consolidation and conditions for the transfer of copyright (identification of authorship) is carried out in the License Agreement. In particular, the authors reserve the right to the authorship of their manuscript and transfer the first publication of this work to the journal under the terms of the Creative Commons CC BY license. At the same time, they have the right to conclude on their own additional agreements concerning the non-exclusive distribution of the work in the form in which it was published by this journal, but provided that the link to the first publication of the article in this journal is preserved.
A license agreement is a document in which the author warrants that he/she owns all copyright for the work (manuscript, article, etc.).
The authors, signing the License Agreement with TECHNOLOGY CENTER PC, have all rights to the further use of their work, provided that they link to our edition in which the work was published.
According to the terms of the License Agreement, the Publisher TECHNOLOGY CENTER PC does not take away your copyrights and receives permission from the authors to use and dissemination of the publication through the world's scientific resources (own electronic resources, scientometric databases, repositories, libraries, etc.).
In the absence of a signed License Agreement or in the absence of this agreement of identifiers allowing to identify the identity of the author, the editors have no right to work with the manuscript.
It is important to remember that there is another type of agreement between authors and publishers – when copyright is transferred from the authors to the publisher. In this case, the authors lose ownership of their work and may not use it in any way.