Design of an intelligent data analysis platform for pharmaceutical forecasts
DOI:
https://doi.org/10.15587/1729-4061.2024.313490Keywords:
deep learning, artificial neural networks, pharmaceutical market, data analysis, predictive tasksAbstract
This study considers the task to design a data analysis platform with predictive capabilities of neural networks. The object of research is intelligent decision-making systems built on deep learning methods. The proposed intelligent platform takes into account the specificity of working with data in the dynamic and uncertain environment of the pharmaceutical market. Its main purpose is the processing of various data formats, such as time series, regression, classification data sets to create forecasts based on various indicators. At the core of the platform architecture, along with technologies for backend and frontend development (HTML, JS, CSS, C#, .NET), MSSQL Server and TSQL for database management, are AI Microservices (Python, Flask); they are responsible for artificial intelligence services, in particular neural networks.
In order to identify the optimal model, which is able to effectively solve regression problems based on the selected indicators, the study analyzed several configurations of neural networks on End-to-end machine learning platforms. Distinctive features of the architecture of the designed data analysis platform include its ability to dynamically switch between different machine learning models based on predefined indicators and criteria such as prediction accuracy and model selection.
Improved interpretation of forecasts through the use of Explainable AI enables effective decision-making in the pharmaceutical industry. The functioning of the proposed instrumental decision-making base is demonstrated on the examples of forecasting trends in the consumption of pharmaceuticals by different groups in the pharmaceutical markets of different countries. Automating the model selection and prediction loss minimization process in a comprehensive data analysis platform (CDAP) improves forecast accuracy by approximately 15 % compared to traditional manually selected models.
References
- PROXIMA RESEARCH. Available at: https://proximaresearch.com/ua/en/
- Rathipriya, R., Abdul Rahman, A. A., Dhamodharavadhani, S., Meero, A., Yoganandan, G. (2022). Demand forecasting model for time-series pharmaceutical data using shallow and deep neural network model. Neural Computing and Applications, 35 (2), 1945–1957. https://doi.org/10.1007/s00521-022-07889-9
- Ikegwu, A. C., Nweke, H. F., Anikwe, C. V., Alo, U. R., Okonkwo, O. R. (2022). Big data analytics for data-driven industry: a review of data sources, tools, challenges, solutions, and research directions. Cluster Computing, 25 (5), 3343–3387. https://doi.org/10.1007/s10586-022-03568-5
- Taherdoost, H. (2023). Deep Learning and Neural Networks: Decision-Making Implications. Symmetry, 15 (9), 1723. https://doi.org/10.3390/sym15091723
- Lundberg, S. M., Lee, S.-I. (2017). A unified approach to interpreting model predictions. Advances in neural information processing systems. arXiv. https://doi.org/10.48550/arXiv.1705.07874
- Jemmali, M., Alharbi, M., Melhim, L. K. B. (2018). Intelligent Decision-Making Algorithm for Supplier Evaluation Based on Multi-criteria Preferences. 2018 1st International Conference on Computer Applications & Information Security (ICCAIS), 184, 1–5. https://doi.org/10.1109/cais.2018.8441992
- Sarker, I. H. (2021). Deep Learning: A Comprehensive Overview on Techniques, Taxonomy, Applications and Research Directions. SN Computer Science, 2 (6). https://doi.org/10.1007/s42979-021-00815-1
- Sarker, I. H. (2022). AI-Based Modeling: Techniques, Applications and Research Issues Towards Automation, Intelligent and Smart Systems. SN Computer Science, 3 (2). https://doi.org/10.1007/s42979-022-01043-x
- Pedregosa, F., Varoquaux, G., Gramfort, A., Michel, V., Thirion, B., Grisel, O. et al. (2012). Scikit-learn: machine learning in python. arXiv. https://doi.org/10.48550/arXiv.1201.0490
- Sarker, I. H. (2021). Machine Learning: Algorithms, Real-World Applications and Research Directions. SN Computer Science, 2 (3). https://doi.org/10.1007/s42979-021-00592-x
- Alzubaidi, L., Zhang, J., Humaidi, A. J., Al-Dujaili, A., Duan, Y., Al-Shamma, O. et al. (2021). Review of deep learning: concepts, CNN architectures, challenges, applications, future directions. Journal of Big Data, 8 (1). https://doi.org/10.1186/s40537-021-00444-8
- Janiesch, C., Zschech, P., Heinrich, K. (2021). Machine learning and deep learning. Electronic Markets, 31 (3), 685–695. https://doi.org/10.1007/s12525-021-00475-2
- Roberts, D. A., Yaida, S., Hanin, B. (2022). The Principles of Deep Learning Theory. Cambridge University Press. https://doi.org/10.1017/9781009023405
- Candan, G., Taşkin, M. F., Yazgan, H. R. (2014). Demand Forecasting in Pharmaceutical Industry Using Artificial Intelligence: Neuro-Fuzzy Approach. Journal of Military and Information Science, 2 (2), 41–49. Available at: https://citeseerx.ist.psu.edu/document?repid=rep1&type=pdf&doi=443a425bee280e0a0795f0193a5b82e1aa5c04be
- Mousa, B. A., Al-Khateeb, B. (2023). Predicting medicine demand using deep learning techniques: A review. Journal of Intelligent Systems, 32 (1). https://doi.org/10.1515/jisys-2022-0297
- Kumari, A., Bohra, N. (2021). Prediction of Drug Sales by Using Neural Network Algorithm. Proceedings of the 2nd International Conference on ICT for Digital, Smart, and Sustainable Development, ICIDSSD 2020, 27-28 February 2020, Jamia Hamdard, New Delhi, India. https://doi.org/10.4108/eai.27-2-2020.2303124
- Apteka.ua. Available at: https://www.apteka.ua/
- E-commerce. Available at: https://e-commerce.com.ua/
- FARMAK. Available at: https://farmak.ua/regularna-informacia/
- DARNITSA. Available at: https://darnytsia.ua/reports
- Machine Learning Playground. Available at: https://ml-playground.com/
- An end-to-end platform for machine learning. TensorFlow. Available at: https://www.tensorflow.org/
- Karra, J. (2020). Deep learning using Keras.Net with C#. Available at: https://jagathprasad0.medium.com/deep-learning-using-keras-net-with-c-1d1fceac4531
- Brownlee, J. (2017). Deep Learning With Python. Available at: https://machinelearningmastery.com/deep-learning-with-python/
- Sarker, I. H. (2021). Data Science and Analytics: An Overview from Data-Driven Smart Computing, Decision-Making and Applications Perspective. SN Computer Science, 2 (5). https://doi.org/10.1007/s42979-021-00765-8
- Khalil Zadeh, N., Sepehri, M. M., Farvaresh, H. (2014). Intelligent Sales Prediction for Pharmaceutical Distribution Companies: A Data Mining Based Approach. Mathematical Problems in Engineering, 2014, 1–15. https://doi.org/10.1155/2014/420310
- Davenport, T. H., Harris, J. G. (2007). The dark side of customer analytics. Harvard Business Review, 85 (5). Available at: https://www.researchgate.net/publication/228386781_The_dark_side_of_customer_analytics
- Landset, S., Khoshgoftaar, T. M., Richter, A. N., Hasanin, T. (2015). A survey of open source tools for machine learning with big data in the Hadoop ecosystem. Journal of Big Data, 2 (1). https://doi.org/10.1186/s40537-015-0032-1
- Geron, A. (2019). Hands-On Machine Learning with Scikit-Learn, Keras, and TensorFlow: Concepts, Tools, and Techniques to Build Intelligent Systems. O’Reilly Media, 856.
- Xin, Y., Kong, L., Liu, Z., Chen, Y., Li, Y., Zhu, H. et al. (2018). Machine Learning and Deep Learning Methods for Cybersecurity. IEEE Access, 6, 35365–35381. https://doi.org/10.1109/access.2018.2836950
- Sarker, I. H., Kayes, A. S. M., Badsha, S., Alqahtani, H., Watters, P., Ng, A. (2020). Cybersecurity data science: an overview from machine learning perspective. Journal of Big Data, 7 (1). https://doi.org/10.1186/s40537-020-00318-5
- Pharmaceutical market (2024). OECD. https://doi.org/10.1787/data-00545-en
- National Health Service of Ukraine. Available at: https://edata.e-health.gov.ua/e-data/open-data
- Information on written electronic prescriptions under the drug reimbursement program ("Available drugs"). Available at: https://data.gov.ua/dataset/9980a894-67c5-4da6-a0fc-1bab6c764cbf
- Medical procurement of Ukraine. Available at: https://mpu.gov.ua/uk
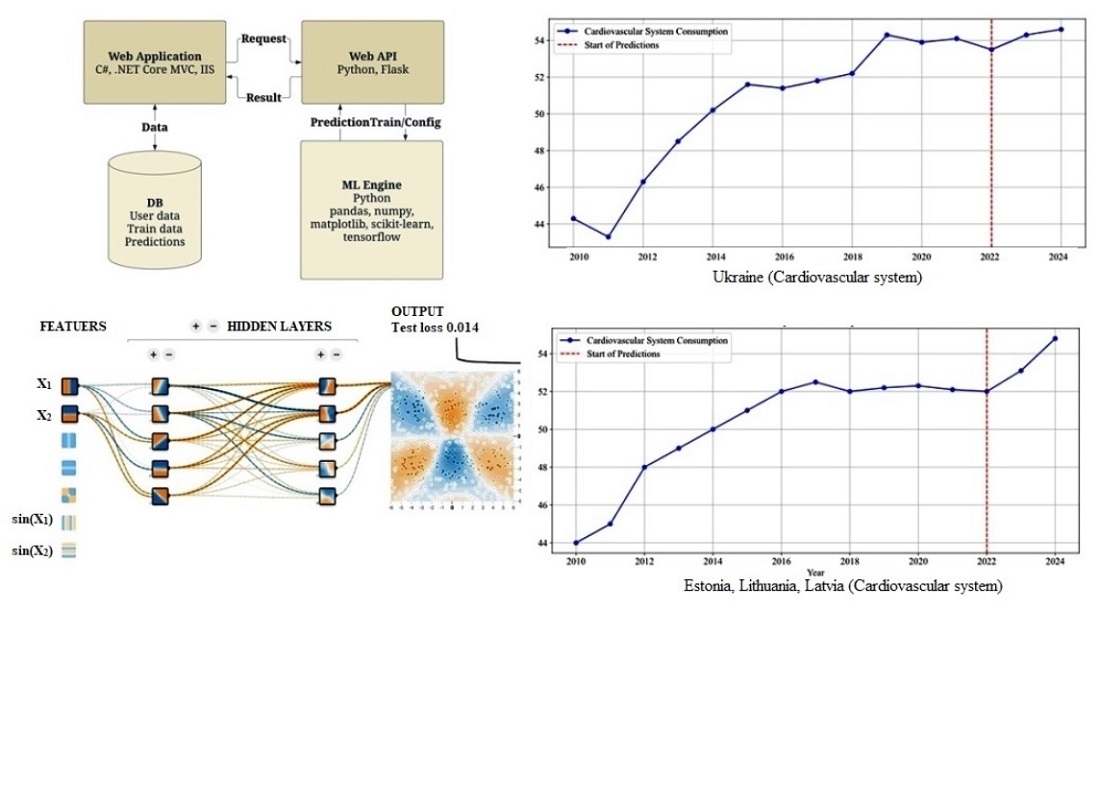
Downloads
Published
How to Cite
Issue
Section
License
Copyright (c) 2024 Zoia Sokolovska, Iryna Ivchenko, Oleg Ivchenko

This work is licensed under a Creative Commons Attribution 4.0 International License.
The consolidation and conditions for the transfer of copyright (identification of authorship) is carried out in the License Agreement. In particular, the authors reserve the right to the authorship of their manuscript and transfer the first publication of this work to the journal under the terms of the Creative Commons CC BY license. At the same time, they have the right to conclude on their own additional agreements concerning the non-exclusive distribution of the work in the form in which it was published by this journal, but provided that the link to the first publication of the article in this journal is preserved.
A license agreement is a document in which the author warrants that he/she owns all copyright for the work (manuscript, article, etc.).
The authors, signing the License Agreement with TECHNOLOGY CENTER PC, have all rights to the further use of their work, provided that they link to our edition in which the work was published.
According to the terms of the License Agreement, the Publisher TECHNOLOGY CENTER PC does not take away your copyrights and receives permission from the authors to use and dissemination of the publication through the world's scientific resources (own electronic resources, scientometric databases, repositories, libraries, etc.).
In the absence of a signed License Agreement or in the absence of this agreement of identifiers allowing to identify the identity of the author, the editors have no right to work with the manuscript.
It is important to remember that there is another type of agreement between authors and publishers – when copyright is transferred from the authors to the publisher. In this case, the authors lose ownership of their work and may not use it in any way.