Removing cloudiness on optical space images by a generative adversarial network model using SAR images
DOI:
https://doi.org/10.15587/1729-4061.2024.313690Keywords:
remote probing, image reconstruction, cloud removal, generative adversarial networkAbstract
The object of this study is the process of removing cloudiness on optical space images. Solving the cloudiness removal task is an important stage in processing data from the Earth remote probing (ERP) aimed at reconstructing the information hidden by these atmospheric disturbances. The analyzed shortcomings in the fusion of purely optical data led to the conclusion that the best solution to the cloudiness removal problem is a combination of optical and radar data. Compared to conventional methods of image processing, neural networks could provide more efficient and better performance indicators due to the ability to adapt to different conditions and types of images. As a result, a generative adversarial network (GAN) model with cyclic-sequential 7-ResNeXt block architecture was constructed for cloud removal in optical space imagery using synthetic aperture radar (SAR) imagery. The model built generates fewer artifacts when transforming the image compared to other models that process multi-temporal images.
The experimental results on the SEN12MS-CR data set demonstrate the ability of the constructed model to remove dense clouds from simultaneous Sentinel-2 space images. This is confirmed by the pixel reconstruction of all multispectral channels with an average RMSE value of 2.4 %. To increase the informativeness of the neural network during model training, a SAR image with a C-band signal is used, which has a longer wavelength and thereby provides medium-resolution data about the geometric structure of the Earth's surface. Applying this model could make it possible to improve the situational awareness at all levels of control over the Armed Forces (AF) of Ukraine through the use of current space observations of the Earth from various ERP systems
References
- Rees, W. G. (2012). Physical Principles of Remote Sensing. Cambridge University Press https://doi.org/10.1017/cbo9781139017411
- Shen, H., Li, X., Cheng, Q., Zeng, C., Yang, G., Li, H., Zhang, L. (2015). Missing Information Reconstruction of Remote Sensing Data: A Technical Review. IEEE Geoscience and Remote Sensing Magazine, 3 (3), 61–85. https://doi.org/10.1109/mgrs.2015.2441912
- Xu, M., Jia, X., Pickering, M., Jia, S. (2019). Thin cloud removal from optical remote sensing images using the noise-adjusted principal components transform. ISPRS Journal of Photogrammetry and Remote Sensing, 149, 215–225. https://doi.org/10.1016/j.isprsjprs.2019.01.025
- Ji, T.-Y., Yokoya, N., Zhu, X. X., Huang, T.-Z. (2018). Nonlocal Tensor Completion for Multitemporal Remotely Sensed Images’ Inpainting. IEEE Transactions on Geoscience and Remote Sensing, 56 (6), 3047–3061. https://doi.org/10.1109/tgrs.2018.2790262
- Li, X., Wang, L., Cheng, Q., Wu, P., Gan, W., Fang, L. (2019). Cloud removal in remote sensing images using nonnegative matrix factorization and error correction. ISPRS Journal of Photogrammetry and Remote Sensing, 148, 103–113. https://doi.org/10.1016/j.isprsjprs.2018.12.013
- Meng, F., Yang, X., Zhou, C., Li, Z. (2017). A Sparse Dictionary Learning-Based Adaptive Patch Inpainting Method for Thick Clouds Removal from High-Spatial Resolution Remote Sensing Imagery. Sensors, 17 (9), 2130. https://doi.org/10.3390/s17092130
- Cheng, Q., Shen, H., Zhang, L., Yuan, Q., Zeng, C. (2014). Cloud removal for remotely sensed images by similar pixel replacement guided with a spatio-temporal MRF model. ISPRS Journal of Photogrammetry and Remote Sensing, 92, 54–68. https://doi.org/10.1016/j.isprsjprs.2014.02.015
- Eckardt, R., Berger, C., Thiel, C., Schmullius, C. (2013). Removal of Optically Thick Clouds from Multi-Spectral Satellite Images Using Multi-Frequency SAR Data. Remote Sensing, 5 (6), 2973–3006. https://doi.org/10.3390/rs5062973
- Zhang, Q., Yuan, Q., Zeng, C., Li, X., Wei, Y. (2018). Missing Data Reconstruction in Remote Sensing Image With a Unified Spatial–Temporal–Spectral Deep Convolutional Neural Network. IEEE Transactions on Geoscience and Remote Sensing, 56 (8), 4274–4288. https://doi.org/10.1109/tgrs.2018.2810208
- Isola, P., Zhu, J.-Y., Zhou, T., Efros, A. A. (2017). Image-to-Image Translation with Conditional Adversarial Networks. 2017 IEEE Conference on Computer Vision and Pattern Recognition (CVPR). https://doi.org/10.1109/cvpr.2017.632
- Zhang, X., Zhang, T., Wang, G., Zhu, P., Tang, X., Jia, X., Jiao, L. (2023). Remote Sensing Object Detection Meets Deep Learning: A metareview of challenges and advances. IEEE Geoscience and Remote Sensing Magazine, 11 (4), 8–44. https://doi.org/10.1109/mgrs.2023.3312347
- He, K., Zhang, X., Ren, S., Sun, J. (2016). Deep Residual Learning for Image Recognition. 2016 IEEE Conference on Computer Vision and Pattern Recognition (CVPR). https://doi.org/10.1109/cvpr.2016.90
- Meng, Q., Borders, B. E., Cieszewski, C. J., Madden, M. (2009). Closest Spectral Fit for Removing Clouds and Cloud Shadows. Photogrammetric Engineering & Remote Sensing, 75 (5), 569–576. https://doi.org/10.14358/pers.75.5.569
- Schmitt, M., Zhu, X. X. (2016). Data Fusion and Remote Sensing: An ever-growing relationship. IEEE Geoscience and Remote Sensing Magazine, 4 (4), 6–23. https://doi.org/10.1109/mgrs.2016.2561021
- Wang, L., Xu, X., Yu, Y., Yang, R., Gui, R., Xu, Z., Pu, F. (2019). SAR-to-Optical Image Translation Using Supervised Cycle-Consistent Adversarial Networks. IEEE Access, 7, 129136–129149. https://doi.org/10.1109/access.2019.2939649
- Zhu, J.-Y., Park, T., Isola, P., Efros, A. A. (2017). Unpaired Image-to-Image Translation Using Cycle-Consistent Adversarial Networks. 2017 IEEE International Conference on Computer Vision (ICCV). https://doi.org/10.1109/iccv.2017.244
- Mao, X., Li, Q., Xie, H., Lau, R. Y. K., Wang, Z., Smolley, S. P. (2017). Least Squares Generative Adversarial Networks. 2017 IEEE International Conference on Computer Vision (ICCV). https://doi.org/10.1109/iccv.2017.304
- Goodfellow, I., Bengio, Y., Courville, A. (2016). Deep learning. MIT Press. Available at: https://www.deeplearningbook.org/
- Ebel, P., Meraner, A., Schmitt, M., Zhu, X. X. (2021). Multisensor Data Fusion for Cloud Removal in Global and All-Season Sentinel-2 Imagery. IEEE Transactions on Geoscience and Remote Sensing, 59 (7), 5866–5878. https://doi.org/10.1109/tgrs.2020.3024744
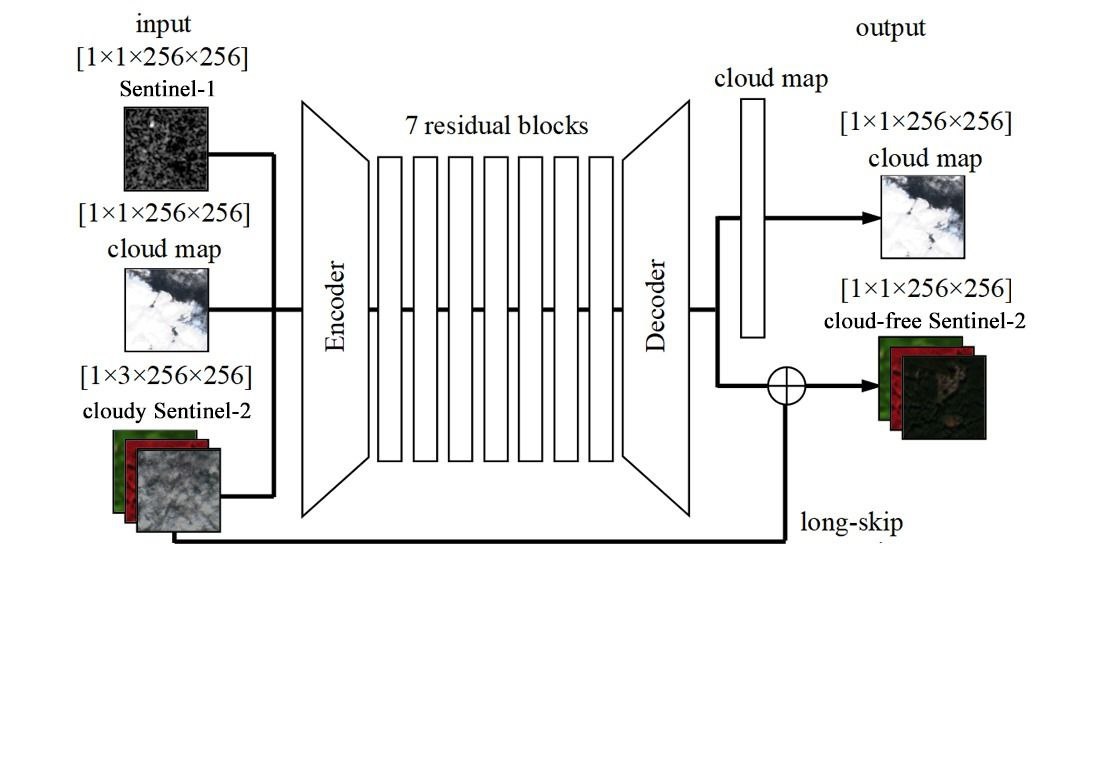
Downloads
Published
How to Cite
Issue
Section
License
Copyright (c) 2024 Mykola Romanchuk, Andrii Zavada, Olena Naumchak, Leonid Naumchak, Iryna Kosheva

This work is licensed under a Creative Commons Attribution 4.0 International License.
The consolidation and conditions for the transfer of copyright (identification of authorship) is carried out in the License Agreement. In particular, the authors reserve the right to the authorship of their manuscript and transfer the first publication of this work to the journal under the terms of the Creative Commons CC BY license. At the same time, they have the right to conclude on their own additional agreements concerning the non-exclusive distribution of the work in the form in which it was published by this journal, but provided that the link to the first publication of the article in this journal is preserved.
A license agreement is a document in which the author warrants that he/she owns all copyright for the work (manuscript, article, etc.).
The authors, signing the License Agreement with TECHNOLOGY CENTER PC, have all rights to the further use of their work, provided that they link to our edition in which the work was published.
According to the terms of the License Agreement, the Publisher TECHNOLOGY CENTER PC does not take away your copyrights and receives permission from the authors to use and dissemination of the publication through the world's scientific resources (own electronic resources, scientometric databases, repositories, libraries, etc.).
In the absence of a signed License Agreement or in the absence of this agreement of identifiers allowing to identify the identity of the author, the editors have no right to work with the manuscript.
It is important to remember that there is another type of agreement between authors and publishers – when copyright is transferred from the authors to the publisher. In this case, the authors lose ownership of their work and may not use it in any way.