Improving information theory of context-aware phrase embeddings in HR domain
DOI:
https://doi.org/10.15587/1729-4061.2024.313970Keywords:
natural language processing, large language models, text embeddings, information retrievalAbstract
The object of the study is context-aware phrase representations. The growing need to automate candidate recruitment and job recommendation processes has paved the way for the utilization of text embeddings. These embeddings involve translating the semantic essence of text into a continuous, high-dimensional vector space. By learning context-aware rich and meaningful representations of phrases within the human resource domain, the efficacy of similarity searches and matching procedures is enhanced, which contributes to a more streamlined and effective recruitment process. However, existing approaches do not take into account the context when modeling phrases. This necessitates the improvement of information technology analysis in this area. In this paper, it is proposed to mark the beginning and end of phrases in the text using special tokens. This made it possible to reduce the requirements for computing power by calculating all phrase representations present in the text simultaneously. The effectiveness of the improvement was tested on a new dataset to compare and evaluate the models in the task of modeling phrases in the field of human resources management. The proposed approach to modeling phrase representations with regard to context in the field of human resources management leads to an improvement in computational efficiency by up to 50 % and an increase in accuracy by up to 10 %. The architecture of the machine learning model for creating context-aware phrase representations is developed, which is characterized by the presence of blocks for taking into account phrase boundaries. Experiments and comparisons with existing approaches have confirmed the effectiveness of the proposed solution. In practice, the proposed information analysis technology can be used to automate the process of identifying and normalizing candidates' skills in online recruiting
References
- Green, T., Maynard, D., Lin, C. (2022). Development of a benchmark corpus to support entity recognition in job descriptions. Proceedings of the Thirteenth Language Resources and Evaluation Conference. Available at: https://aclanthology.org/2022.lrec-1.128/
- Zhang, M., Jensen, K., Sonniks, S., Plank, B. (2022). SkillSpan: Hard and Soft Skill Extraction from English Job Postings. Proceedings of the 2022 Conference of the North American Chapter of the Association for Computational Linguistics: Human Language Technologies. https://doi.org/10.18653/v1/2022.naacl-main.366
- O*NET OnLine. Available at: https://www.onetonline.org/
- European Skills/Competences, Qualifications and Occupations (ESCO). Available at: https://ec.europa.eu/social/main.jsp?catId=1326&langId=en
- Malakhov, E., Shchelkonogov, D., Mezhuyev, V. (2019). Algorithms of Classification of Mass Problems of Production Subject Domains. Proceedings of the 2019 8th International Conference on Software and Computer Applications, 149–153. https://doi.org/10.1145/3316615.3316676
- Prykhodko, S., Prykhodko, N. (2022). A Technique for Detecting Software Quality Based on the Confidence and Prediction Intervals of Nonlinear Regression for RFC Metric. 2022 IEEE 17th International Conference on Computer Sciences and Information Technologies (CSIT), 499–502. https://doi.org/10.1109/csit56902.2022.10000532
- Gotthardt, M., Mezhuyev, V. (2022). Measuring the Success of Recommender Systems: A PLS-SEM Approach. IEEE Access, 10, 30610–30623. https://doi.org/10.1109/access.2022.3159652
- Pro zastosuvannia anhliyskoi movy v Ukraini. Dokument 3760-IX. Pryiniattia vid 04.06.2024. Available at: https://zakon.rada.gov.ua/laws/show/3760-20#Text
- Vaswani, A., Shazeer, N., Parmar, N., Uszkoreit, J., Jones, L., Gomez, A. N. et al. (2017). Attention is all you need. arXiv. https://doi.org/10.48550/arXiv.1706.03762
- Devlin, J., Chang, M.-W., Lee, K., Toutanova, K. (2019). BERT: Pre-training of Deep Bidirectional Transformers for Language Understanding. Proceedings of the 2019 Conference of the North. https://doi.org/10.18653/v1/n19-1423
- Reimers, N., Gurevych, I. (2019). Sentence-BERT: Sentence Embeddings using Siamese BERT-Networks. Proceedings of the 2019 Conference on Empirical Methods in Natural Language Processing and the 9th International Joint Conference on Natural Language Processing (EMNLP-IJCNLP). https://doi.org/10.18653/v1/d19-1410
- Wang, S., Thompson, L., Iyyer, M. (2021). Phrase-BERT: Improved Phrase Embeddings from BERT with an Application to Corpus Exploration. Proceedings of the 2021 Conference on Empirical Methods in Natural Language Processing. https://doi.org/10.18653/v1/2021.emnlp-main.846
- Cer, D., Diab, M., Agirre, E., Lopez-Gazpio, I., Specia, L. (2017). SemEval-2017 Task 1: Semantic Textual Similarity Multilingual and Crosslingual Focused Evaluation. Proceedings of the 11th International Workshop on Semantic Evaluation (SemEval-2017). https://doi.org/10.18653/v1/s17-2001
- Cohen, A., Gonen, H., Shapira, O., Levy, R., Goldberg, Y. (2022). McPhraSy: Multi-Context Phrase Similarity and Clustering. Findings of the Association for Computational Linguistics: EMNLP 2022, 3538–3550. https://doi.org/10.18653/v1/2022.findings-emnlp.259
- Decorte, J.-J., Van Hautte, J., Demeester, T., Develder, C. (2021). JobBERT: Understanding job titles through skill. International workshop on Fair, Effective And Sustainable Talent management using data science (FEAST) as part of ECML-PKDD 2021. arXiv. https://doi.org/10.48550/arXiv.2109.09605
- Decorte, J.-J., Van Hautte, J., Deleu, J., Develder, C., Demeester, T. (2022). Design of negative sampling strategies for distantly supervised skill extraction. 2nd Workshop on Recommender Systems for Human Resources (RecSys in HR 2022) as part of RecSys 2022. arXiv. https://doi.org/10.48550/arXiv.2209.05987
- Bhola, A., Halder, K., Prasad, A., Kan, M.-Y. (2020). Retrieving Skills from Job Descriptions: A Language Model Based Extreme Multi-label Classification Framework. Proceedings of the 28th International Conference on Computational Linguistics. https://doi.org/10.18653/v1/2020.coling-main.513
- Djumalieva, J., Sleeman, C. (2018). An Open and Data-driven Taxonomy of Skills Extracted from Online Job Adverts. Developing Skills in a Changing World of Work, 425–454. https://doi.org/10.5771/9783957103154-425
- Decorte, J.-J., Verlinden, S., Van Hautte, J., Deleu, J., Develder, C., Demeester, T. (2020). Extreme Multi-Label Skill Extraction Training using Large Language Models. International workshop on AI for Human Resources and Public Employment Services (AI4HR&PES) as part of ECML-PKDD 2023. arXiv. https://doi.org/10.48550/arXiv.2307.10778
- Günther, M., Mastrapas, G., Wang, B., Xiao, H., Geuter, J. (2023). Jina Embeddings: A Novel Set of High-Performance Sentence Embedding Models. Proceedings of the 3rd Workshop for Natural Language Processing Open Source Software (NLP-OSS 2023), 8–18. https://doi.org/10.18653/v1/2023.nlposs-1.2
- Mashtalir, S. V., Nikolenko, O. V. (2023). Data preprocessing and tokenization techniques for technical Ukrainian texts. Applied Aspects of Information Technology, 6 (3), 318–326. https://doi.org/10.15276/aait.06.2023.22
- Bojanowski, P., Grave, E., Joulin, A., Mikolov, T. (2017). Enriching Word Vectors with Subword Information. Transactions of the Association for Computational Linguistics, 5, 135–146. https://doi.org/10.1162/tacl_a_00051
- Lee, K., Ippolito, D., Nystrom, A., Zhang, C., Eck, D., Callison-Burch, C., Carlini, N. (2022). Deduplicating Training Data Makes Language Models Better. Proceedings of the 60th Annual Meeting of the Association for Computational Linguistics (Volume 1: Long Papers). https://doi.org/10.18653/v1/2022.acl-long.577
- Wang, L., Yang, N., Huang, X., Jiao, B., Yang, L., Jiang, D. et al. (2022). Text embeddings by weakly-supervised contrastive pre-training. arXiv. https://doi.org/10.48550/arXiv.2212.03533
- Xiao, S., Liu, Z., Shao, Y., Cao, Z. (2022). RetroMAE: Pre-Training Retrieval-oriented Language Models Via Masked Auto-Encoder. Proceedings of the 2022 Conference on Empirical Methods in Natural Language Processing. https://doi.org/10.18653/v1/2022.emnlp-main.35
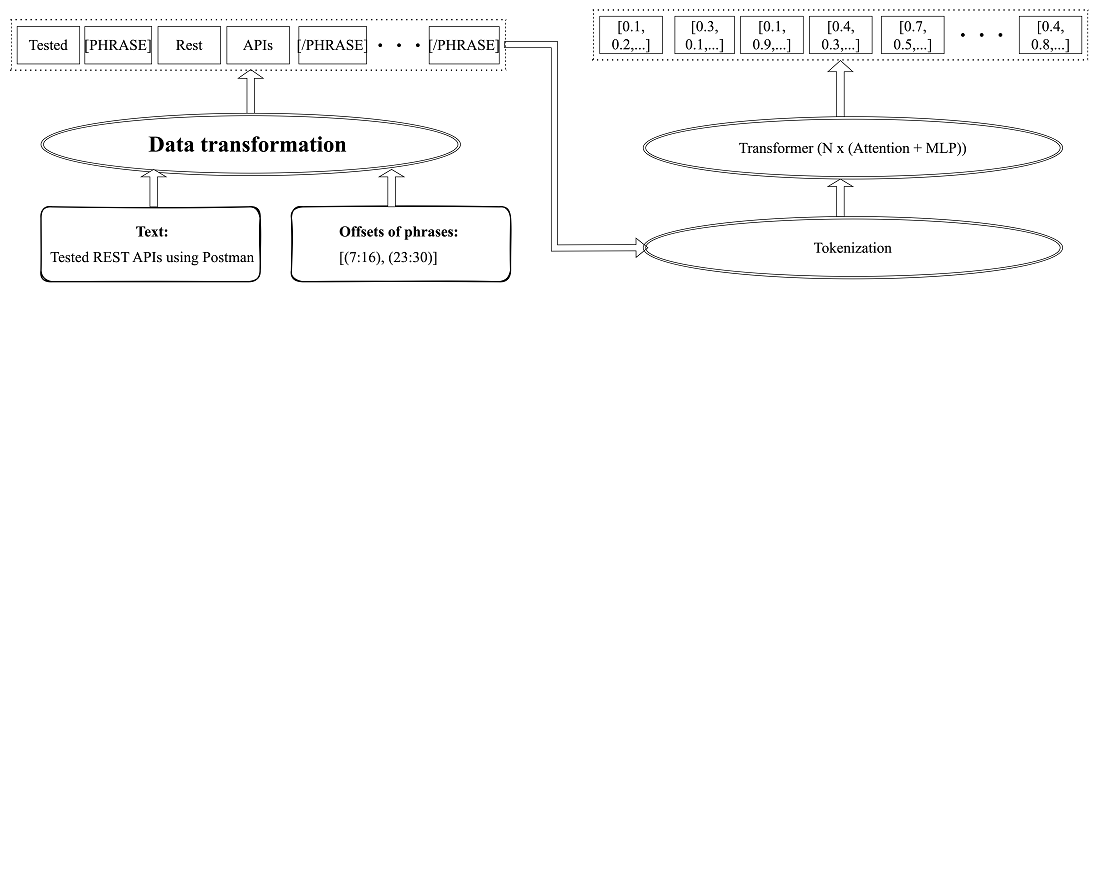
Downloads
Published
How to Cite
Issue
Section
License
Copyright (c) 2024 Maiia Bocharova, Eugene Malakhov

This work is licensed under a Creative Commons Attribution 4.0 International License.
The consolidation and conditions for the transfer of copyright (identification of authorship) is carried out in the License Agreement. In particular, the authors reserve the right to the authorship of their manuscript and transfer the first publication of this work to the journal under the terms of the Creative Commons CC BY license. At the same time, they have the right to conclude on their own additional agreements concerning the non-exclusive distribution of the work in the form in which it was published by this journal, but provided that the link to the first publication of the article in this journal is preserved.
A license agreement is a document in which the author warrants that he/she owns all copyright for the work (manuscript, article, etc.).
The authors, signing the License Agreement with TECHNOLOGY CENTER PC, have all rights to the further use of their work, provided that they link to our edition in which the work was published.
According to the terms of the License Agreement, the Publisher TECHNOLOGY CENTER PC does not take away your copyrights and receives permission from the authors to use and dissemination of the publication through the world's scientific resources (own electronic resources, scientometric databases, repositories, libraries, etc.).
In the absence of a signed License Agreement or in the absence of this agreement of identifiers allowing to identify the identity of the author, the editors have no right to work with the manuscript.
It is important to remember that there is another type of agreement between authors and publishers – when copyright is transferred from the authors to the publisher. In this case, the authors lose ownership of their work and may not use it in any way.