Developing an early detection model for skin diseases using a hybrid deep neural network to enhance health independence in coastal communities
DOI:
https://doi.org/10.15587/1729-4061.2024.313983Keywords:
hybrid deep neural network (HDNN), EfficientNetB7, YOLOv8, skin diseases, coastal communities, healthAbstract
The object of study is a solution for early skin disease diagnosis by integrating hybrid deep neural networks – EfficientNetB7 for Classification and YOLOv8 for detection. The system is designed to classify five skin conditions: Melanoma, Basal Cell Carcinoma (BCC), melanoma is a type of skin cancer that originates from melanocytes, the cells that produce skin pigment, Melanocytic Nevi (NV) Melanocytic nevus is a mole or dark spot on the skin formed due to the accumulation of melanocytes, Benign Keratosis-like Lesions (BKL) is a term for a group of skin changes that resemble keratosis but are non-cancerous, and Seborrheic Keratoses and other benign tumors to enhance the health diagnostics. The problem to be solved in this study revolves around improving early and accurate skin disease diagnosis, particularly in resource-limited or underserved areas and the lack of Accessible Diagnostic Tools and Low Efficiency of Current Diagnostic Methods. The study highlights EfficientNetB7's classification accuracy at 94 % and YOLOv8's means average precision (mAP) of 0.812 for detection. This hybrid system processes skin images efficiently, providing classification and detection outcomes with consistent performance in multiple tests. The results demonstrate that the EfficientNetB7 model achieved an accuracy of 94 % on test data, while YOLOv8 delivered a detection performance with a mean average precision (mAP) of 0.812. The web-based system efficiently processed skin images and provided classification and detection outcomes.
Furthermore, integrating EfficientNetB7 and YOLOv8 allowed the skin disease detection system to classify five different diseases and assess malignancy risk. The systems are portable and can be used with minimal setup, making them practical for real-world diagnostic use. The Scope and Practical applications are designed for accessibility in resource-limited settings. The website-based skin disease detection tool provides a user-friendly platform accessible to the public and healthcare providers, especially in underserved areas. Each application's high accuracy and ease of use make them viable aids in early diagnosis, potentially improving healthcare access
References
- Liopyris, K., Gregoriou, S., Dias, J., Stratigos, A. J. (2022). Artificial Intelligence in Dermatology: Challenges and Perspectives. Dermatology and Therapy, 12 (12), 2637–2651. https://doi.org/10.1007/s13555-022-00833-8
- He, Z., Marrone, G., Ou, A., Liu, H., Ma, L., Huang, Y. et al. (2020). Factors affecting health-related quality of life in patients with skin disease: cross-sectional results from 8,789 patients with 16 skin diseases. Health and Quality of Life Outcomes, 18 (1). https://doi.org/10.1186/s12955-020-01542-6
- Prasitpuriprecha, N., Santaweesuk, S., Boonkert, P., Chamnan, P. (2022). Prevalence and DALYs of skin diseases in Ubonratchathani based on real-world national healthcare service data. Scientific Reports, 12 (1). https://doi.org/10.1038/s41598-022-20237-0
- Paithankar, V., Uprit, I., Bairagi, A., Zoting, H., Katarnavare, A. (2023). Skin Disease Identification using Image Processing. International Journal of Advanced Research in Science, Communication and Technology, 3 (2), 225–227. https://doi.org/10.48175/ijarsct-8687
- Ramadan, R., Aly, S. (2022). CU-Net: A New Improved Multi-Input Color U-Net Model for Skin Lesion Semantic Segmentation. IEEE Access, 10, 15539–15564. https://doi.org/10.1109/access.2022.3148402
- Abdelhafeez, A., Mohamed, H. K., Maher, A., Khalil, N. A. (2023). A novel approach toward skin cancer classification through fused deep features and neutrosophic environment. Frontiers in Public Health, 11. https://doi.org/10.3389/fpubh.2023.1123581
- Salma, W., Eltrass, A. S. (2022). Automated deep learning approach for classification of malignant melanoma and benign skin lesions. Multimedia Tools and Applications, 81 (22), 32643–32660. https://doi.org/10.1007/s11042-022-13081-x
- Gound, R. S., Gadre, P. S., Gaikwad, J. B., Wagh, P. K. (2018). Skin Disease Diagnosis System using Image Processing and Data Mining. International Journal of Computer Applications, 179 (16), 38–40. https://doi.org/10.5120/ijca2018916253
- Rao, T. K., Chamanthi, P., Kumar, N. T., Amulya, R. L., Sagar, M. U. (2023). Skin Disease Detection Using Machine Learning. International Journal of Food and Nutritional Sciences, 11 (12). https://doi.org/10.48047/ijfans/v11/i12/171
- Nguyen, V. D., Bui, N. D., Do, H. K. (2022). Skin Lesion Classification on Imbalanced Data Using Deep Learning with Soft Attention. Sensors, 22 (19), 7530. https://doi.org/10.3390/s22197530
- Alsahafi, Y. S., Kassem, M. A., Hosny, K. M. (2023). Skin-Net: a novel deep residual network for skin lesions classification using multilevel feature extraction and cross-channel correlation with detection of outlier. Journal of Big Data, 10 (1). https://doi.org/10.1186/s40537-023-00769-6
- Hosseinzadeh, M., Hussain, D., Zeki Mahmood, F. M., Alenizi, F., Varzeghani, A. N., Asghari, P. et al. (2024). A model for skin cancer using combination of ensemble learning and deep learning. PLOS ONE, 19 (5), e0301275. https://doi.org/10.1371/journal.pone.0301275
- Sharma, A. K., Tiwari, S., Aggarwal, G., Goenka, N., Kumar, A., Chakrabarti, P. et al. (2022). Dermatologist-Level Classification of Skin Cancer Using Cascaded Ensembling of Convolutional Neural Network and Handcrafted Features Based Deep Neural Network. IEEE Access, 10, 17920–17932. https://doi.org/10.1109/access.2022.3149824
- Zhang, X., Wang, S., Liu, J., Tao, C. (2018). Towards improving diagnosis of skin diseases by combining deep neural network and human knowledge. BMC Medical Informatics and Decision Making, 18 (S2). https://doi.org/10.1186/s12911-018-0631-9
- Wan, L., Ai, Z., Chen, J., Jiang, Q., Chen, H., Li, Q. et al. (2022). Detection algorithm for pigmented skin disease based on classifier-level and feature-level fusion. Frontiers in Public Health, 10. https://doi.org/10.3389/fpubh.2022.1034772
- Foahom Gouabou, A. C., Collenne, J., Monnier, J., Iguernaissi, R., Damoiseaux, J.-L., Moudafi, A., Merad, D. (2022). Computer Aided Diagnosis of Melanoma Using Deep Neural Networks and Game Theory: Application on Dermoscopic Images of Skin Lesions. International Journal of Molecular Sciences, 23 (22), 13838. https://doi.org/10.3390/ijms232213838
- Tahir, M., Naeem, A., Malik, H., Tanveer, J., Naqvi, R. A., Lee, S.-W. (2023). DSCC_Net: Multi-Classification Deep Learning Models for Diagnosing of Skin Cancer Using Dermoscopic Images. Cancers, 15 (7), 2179. https://doi.org/10.3390/cancers15072179
- Efimenko, M., Ignatev, A., Koshechkin, K. (2020). Review of medical image recognition technologies to detect melanomas using neural networks. BMC Bioinformatics, 21 (S11). https://doi.org/10.1186/s12859-020-03615-1
- Lyakhov, P. A., Lyakhova, U. A., Nagornov, N. N. (2022). System for the Recognizing of Pigmented Skin Lesions with Fusion and Analysis of Heterogeneous Data Based on a Multimodal Neural Network. Cancers, 14 (7), 1819. https://doi.org/10.3390/cancers14071819
- Han, S. S., Moon, I. J., Lim, W., Suh, I. S., Lee, S. Y., Na, J.-I. et al. (2020). Keratinocytic Skin Cancer Detection on the Face Using Region-Based Convolutional Neural Network. JAMA Dermatology, 156 (1), 29. https://doi.org/10.1001/jamadermatol.2019.3807
- Rasel, M. A., Obaidellah, U. H., Kareem, S. A. (2022). Convolutional Neural Network-Based Skin Lesion Classification With Variable Nonlinear Activation Functions. IEEE Access, 10, 83398–83414. https://doi.org/10.1109/access.2022.3196911
- Ding, J., Song, J., Li, J., Tang, J., Guo, F. (2022). Two-Stage Deep Neural Network via Ensemble Learning for Melanoma Classification. Frontiers in Bioengineering and Biotechnology, 9. https://doi.org/10.3389/fbioe.2021.758495
- Hoefler, T., Alistarh, D., Ben-Nun, T., Dryden, N., Peste, A. (2021). Sparsity in deep learning: Pruning and growth for efficient inference and training in neural networks. Journal of Machine Learning Research, 22. Available at: https://jmlr.org/papers/volume22/21-0366/21-0366.pdf
- Saleem Abdullah, S. M., Abdulazeez, A. M. (2021). Facial Expression Recognition Based on Deep Learning Convolution Neural Network: A Review. Journal of Soft Computing and Data Mining, 02 (01). https://doi.org/10.30880/jscdm.2021.02.01.006
- Bhanumathi, M., Dhanya, S., Gugan, R., Kirthika, K. G. (2022). Marine Plastic Detection Using Deep Learning. Advances in Parallel Computing Algorithms, Tools and Paradigms, 406–413. https://doi.org/10.3233/apc220057
- Harumy, T., Ginting, D. S. Br. (2021). Neural Network Enhancement Forecast of Dengue Fever Outbreaks in Coastal Region. Journal of Physics: Conference Series, 1898 (1), 012027. https://doi.org/10.1088/1742-6596/1898/1/012027
- Ginting, D. S. B., Harumy, T. H. F., Nasution, F. A. F., Siregar, M. E. S. (2021). Analysis Skin Health Patterns in Highlands Area with Apriori and Bayes Contributions. 2021 International Conference on Data Science, Artificial Intelligence, and Business Analytics (DATABIA), 83–87. https://doi.org/10.1109/databia53375.2021.9650158
- Harumy, T. H. F., Chan, H. Y., Sodhy, G. C. (2020). Prediction for Dengue Fever in Indonesia Using Neural Network and Regression Method. Journal of Physics: Conference Series, 1566 (1), 012019. https://doi.org/10.1088/1742-6596/1566/1/012019
- Harumy, T. H. F., Yustika Manik, F., Altaha (2021). Optimization Classification of Diseases Which is Dominant Suffered by Coastal Areas Using Neural Network. 2021 International Conference on Data Science, Artificial Intelligence, and Business Analytics (DATABIA), 136–141. https://doi.org/10.1109/databia53375.2021.9650223
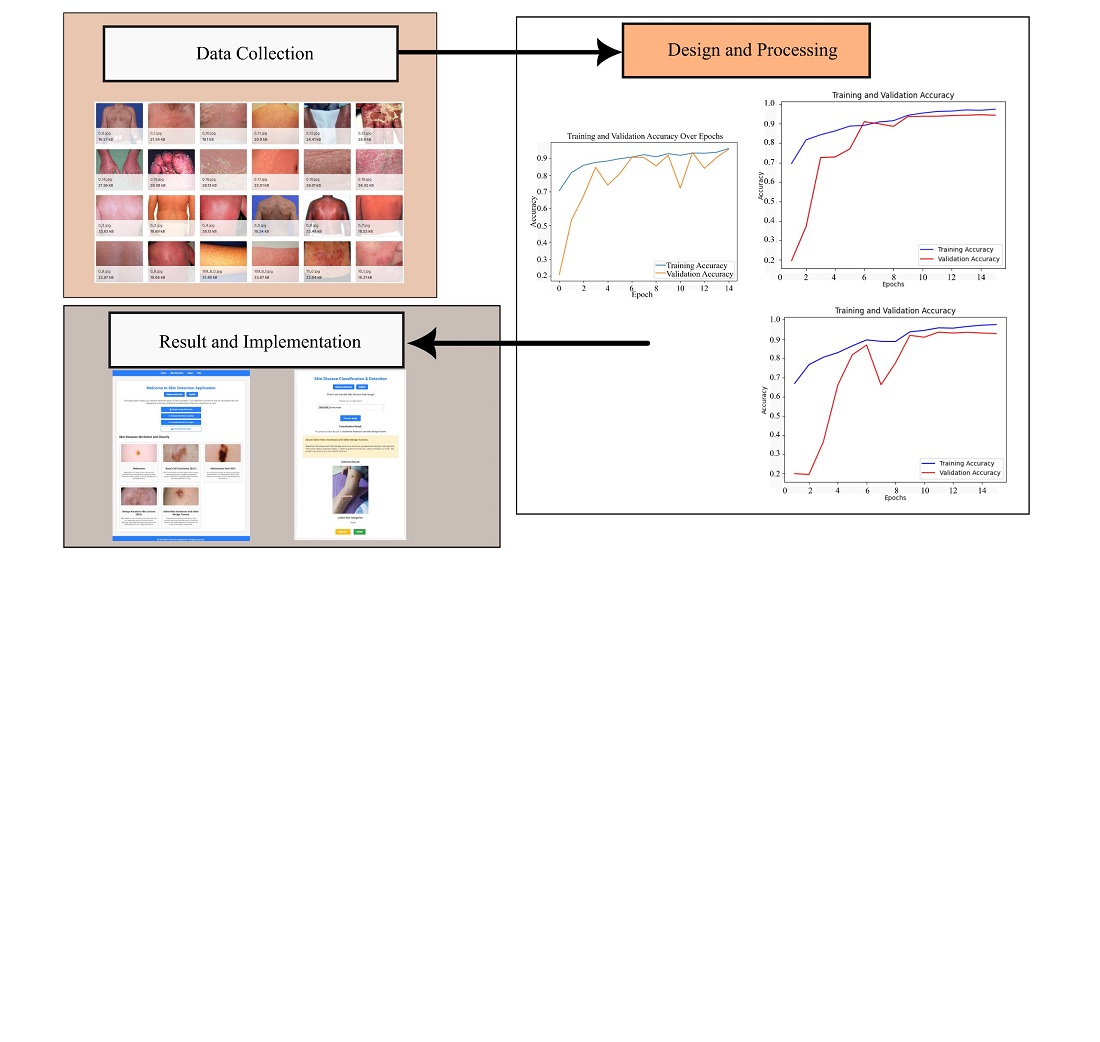
Downloads
Published
How to Cite
Issue
Section
License
Copyright (c) 2024 Tengku Henny Febriana Harumy, Dewi Sartika Br Ginting, Fuzy Yustika Manik, Alkhowarizmi Alkhowarizmi

This work is licensed under a Creative Commons Attribution 4.0 International License.
The consolidation and conditions for the transfer of copyright (identification of authorship) is carried out in the License Agreement. In particular, the authors reserve the right to the authorship of their manuscript and transfer the first publication of this work to the journal under the terms of the Creative Commons CC BY license. At the same time, they have the right to conclude on their own additional agreements concerning the non-exclusive distribution of the work in the form in which it was published by this journal, but provided that the link to the first publication of the article in this journal is preserved.
A license agreement is a document in which the author warrants that he/she owns all copyright for the work (manuscript, article, etc.).
The authors, signing the License Agreement with TECHNOLOGY CENTER PC, have all rights to the further use of their work, provided that they link to our edition in which the work was published.
According to the terms of the License Agreement, the Publisher TECHNOLOGY CENTER PC does not take away your copyrights and receives permission from the authors to use and dissemination of the publication through the world's scientific resources (own electronic resources, scientometric databases, repositories, libraries, etc.).
In the absence of a signed License Agreement or in the absence of this agreement of identifiers allowing to identify the identity of the author, the editors have no right to work with the manuscript.
It is important to remember that there is another type of agreement between authors and publishers – when copyright is transferred from the authors to the publisher. In this case, the authors lose ownership of their work and may not use it in any way.