Ontology modeling for automation of questionnaire data processing
DOI:
https://doi.org/10.15587/1729-4061.2024.314129Keywords:
ontological modeling, questionnaire data, data integration, automation of decision-making systems, questionnaire data analysis, preschool educationAbstract
The object of this study is the analysis of questionnaire data using ontological modeling. The task relates to the fact that conventional methods for processing questionnaire data are often insufficiently effective when working with large volumes of information and do not make it possible to automate many analysis processes.
As a result of the study, an ontology was designed that structures and analyzes questionnaire data, which allows for a more accurate identification of hidden relationships between variables. Using these theoretical provisions, an information system for assessing the quality of assimilation of preschool children's competencies was built. 150 children from various preschool organizations were involved in the study as respondents. The data integration method proposed in this paper significantly facilitated the process of data analysis both for a group and for an individual respondent.
The key difference of the proposed methodology is the automation of routine data analysis operations based on the ontological structure, which significantly simplifies the processing of large volumes of information. This makes it possible to solve the problem of limitations in conventional analysis methods and makes data analysis more scalable and reproducible.
The practical application of the results is possible in marketing for analyzing customer satisfaction, market segmentation, and evaluating the effectiveness of advertising campaigns. In the educational domain, the ontology could be used to evaluate the quality of programs and analyze respondents' opinions, and in sociology – to analyze public opinion and conduct research on social phenomena.
Thus, the proposed ontology provides an effective tool for analyzing large volumes of questionnaire data, allowing organizations to make more informed decisions and improve their efficiency
References
- Hanscom, B., Lurie, J. D., Homa, K., Weinstein, J. N. (2002). Computerized Questionnaires and the Quality of Survey Data. Spine, 27 (16), 1797–1801. https://doi.org/10.1097/00007632-200208150-00020
- Cartwright, A. (1986). Who responds to postal questionnaires? Journal of Epidemiology & Community Health, 40 (3), 267–273. https://doi.org/10.1136/jech.40.3.267
- Martin, E., Polivka, A. E. (1995). Diagnostics for Redesigning Survey Questionnaires: Measuring Work in the Current Population Survey. Public Opinion Quarterly, 59 (4), 547–567. https://doi.org/10.1086/269493
- Chang, R. M., Kauffman, R. J., Kwon, Y. (2014). Understanding the paradigm shift to computational social science in the presence of big data. Decision Support Systems, 63, 67–80. https://doi.org/10.1016/j.dss.2013.08.008
- Feijóo, C., Gómez-Barroso, J. L., Voigt, P. (2014). Exploring the economic value of personal information from firms’ financial statements. International Journal of Information Management, 34 (2), 248–256. https://doi.org/10.1016/j.ijinfomgt.2013.12.005
- Klee, S., Janson, A., Leimeister, J. M. (2021). How Data Analytics Competencies Can Foster Business Value– A Systematic Review and Way Forward. Information Systems Management, 38 (3), 200–217. https://doi.org/10.1080/10580530.2021.1894515
- Jansen, B. J., Jung, S., Salminen, J. (2023). Finetuning Analytics Information Systems for a Better Understanding of Users: Evidence of Personification Bias on Multiple Digital Channels. Information Systems Frontiers, 26(2), 775–798. https://doi.org/10.1007/s10796-023-10395-5
- Tuovinen, L., Smeaton, A. F. (2022). Privacy-aware sharing and collaborative analysis of personal wellness data: Process model, domain ontology, software system and user trial. PLOS ONE, 17 (4), e0265997. https://doi.org/10.1371/journal.pone.0265997
- Alshammari, M., Simpson, A. (2018). Personal Data Management: An Abstract Personal Data Lifecycle Model. Business Process Management Workshops, 685–697. https://doi.org/10.1007/978-3-319-74030-0_55
- Moore, J., Goffin, P., Wiese, J., Meyer, M. (2022). Exploring the Personal Informatics Analysis Gap: “There’s a Lot of Bacon.” IEEE Transactions on Visualization and Computer Graphics, 28 (1), 96–106. https://doi.org/10.1109/tvcg.2021.3114798
- Deutch, D., Milo, T. (2011). A quest for beauty and wealth (or, business processes for database researchers). Proceedings of the thirtieth ACM SIGMOD-SIGACT-SIGART symposium on Principles of database systems (PODS '11). Association for Computing Machinery. New York. https://doi.org/10.1145/1989284.1989286
- Bellomarini, L., Benedetto, D., Gottlob, G., Sallinger, E. (2022). Vadalog: A modern architecture for automated reasoning with large knowledge graphs. Information Systems, 105, 101528. https://doi.org/10.1016/j.is.2020.101528
- Janssen, M., van der Voort, H., Wahyudi, A. (2017). Factors influencing big data decision-making quality. Journal of Business Research, 70, 338–345. https://doi.org/10.1016/j.jbusres.2016.08.007
- Iklassova, K., Aitymova, A., Kopnova, O., Sarzhanova, A., Abildinova, G., Kushumbayev, A., Aitymov, Z. (2024). Construction of a fuzzy model for managing the process of forming IT-competences. Eastern-European Journal of Enterprise Technologies, 3 (3 (129)), 32–43. https://doi.org/10.15587/1729-4061.2024.306183
- Aitymova, A., Shaporeva, A., Kopnova, O., Kushumbayev, A., Aitymov, Z. (2022). Development and modeling of combined components of the information environment. Eastern-European Journal of Enterprise Technologies, 2 (2 (116)), 51–60. https://doi.org/10.15587/1729-4061.2022.255084
- Aitymova, A., Iklassova, K., Abildinova, G., Shaporeva, A., Kopnova, O., Kushumbayev, A. et al. (2023). Development of a model of information process management in the information and educational environment of preschool education organizations. Eastern-European Journal of Enterprise Technologies, 2 (3 (122)), 95–105. https://doi.org/10.15587/1729-4061.2023.276253
- Kulikov, V., Iklassova, K., Kazanbayeva, A. (2020). Entropy based decision making method in managing the development of a socioinformational system. Journal of Theoretical and Applied Information Technology, 98 (1), 92–102.
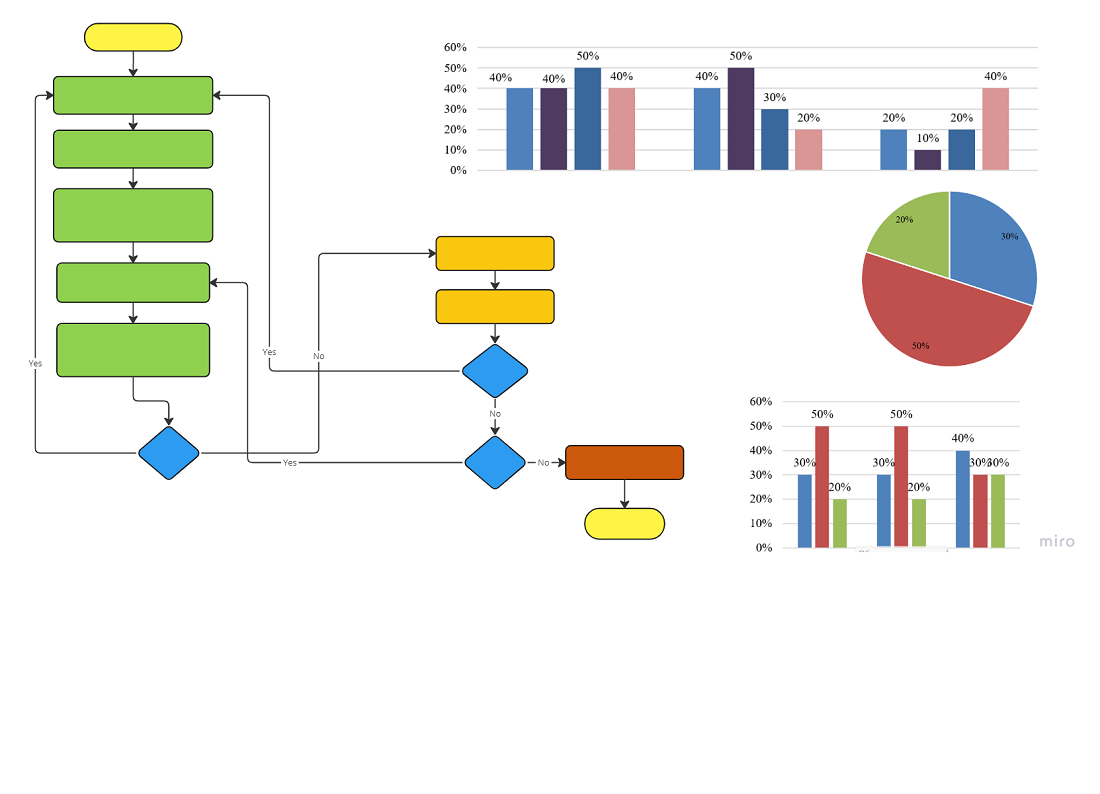
Downloads
Published
How to Cite
Issue
Section
License
Copyright (c) 2024 Kainizhamal Iklassova, Aliya Aitymova, Oxana Kopnova, Anna Shaporeva, Gulmira Abildinova, Zhanat Nurbekova, Leila Almagambetova, Alexey Gorokhov, Zhanat Aitymov

This work is licensed under a Creative Commons Attribution 4.0 International License.
The consolidation and conditions for the transfer of copyright (identification of authorship) is carried out in the License Agreement. In particular, the authors reserve the right to the authorship of their manuscript and transfer the first publication of this work to the journal under the terms of the Creative Commons CC BY license. At the same time, they have the right to conclude on their own additional agreements concerning the non-exclusive distribution of the work in the form in which it was published by this journal, but provided that the link to the first publication of the article in this journal is preserved.
A license agreement is a document in which the author warrants that he/she owns all copyright for the work (manuscript, article, etc.).
The authors, signing the License Agreement with TECHNOLOGY CENTER PC, have all rights to the further use of their work, provided that they link to our edition in which the work was published.
According to the terms of the License Agreement, the Publisher TECHNOLOGY CENTER PC does not take away your copyrights and receives permission from the authors to use and dissemination of the publication through the world's scientific resources (own electronic resources, scientometric databases, repositories, libraries, etc.).
In the absence of a signed License Agreement or in the absence of this agreement of identifiers allowing to identify the identity of the author, the editors have no right to work with the manuscript.
It is important to remember that there is another type of agreement between authors and publishers – when copyright is transferred from the authors to the publisher. In this case, the authors lose ownership of their work and may not use it in any way.