Development a set of mathematical models for anomaly detection in high-load complex computer systems
DOI:
https://doi.org/10.15587/1729-4061.2024.316779Keywords:
high-load complex computer systems, anomaly detection, mathematical models, real-timeAbstract
The subject of this study is the process of anomaly detection in high-load complex computer systems (HLCCSs). The task addressed in the paper is the lack of real-time anomaly detection models in HLCCS with a specified accuracy. A set of mathematical models for real-time anomaly detection has been built and investigated. This set includes a mathematical model for detecting anomalous connections between components of computer system (DACCCSs) and a mathematical model for assessing current state of computer system (CSACS).
The results of models tests showed the following efficiency metrics. For a DACCCS model: accuracy – 84 %, positive predictive value – 87 %, recall – 74 %, and weighted average accuracy (WAA) – 78 %. For a CSACS model: accuracy – 91 %, positive predictive value – 82 %, recall – 68 %, and WAA – 67 %.
The positive results of the study can be attributed to the following factors. A DACCCS model uses projection matrices and orthogonal vector functions to analyze anomalies. This enables the creation of spatial decompositions that reveal complex interrelationships between system components using only eigenvalues and eigenvectors. A CSACS model applies the singular value decomposition method, which implies solving a system of scalar equations to determine the current state of the system. This approach minimizes computational costs compared to methods requiring the solution of complex matrix equations. Thus, the model could be applied for real-time data analysis and anomaly detection under conditions of limited resources and high system load.
The practical application scope includes HLCCS, such as banking transaction servers and cloud platforms, in which it is essential to enable stable operation under high request amount and to minimize the risk of data loss or service failure
References
- Yu, S., Jiang, H., Huang, S., Peng, X., Lu, A. (2021). Compute-in-Memory Chips for Deep Learning: Recent Trends and Prospects. IEEE Circuits and Systems Magazine, 21 (3), 31–56. https://doi.org/10.1109/mcas.2021.3092533
- Kumar, S., Gupta, S., Arora, S. (2021). Research Trends in Network-Based Intrusion Detection Systems: A Review. IEEE Access, 9, 157761–157779. https://doi.org/10.1109/access.2021.3129775
- Lu, P.-J., Lai, M.-C., Chang, J.-S. (2022). A Survey of High-Performance Interconnection Networks in High-Performance Computer Systems. Electronics, 11 (9), 1369. https://doi.org/10.3390/electronics11091369
- Semenov, S., Mozhaiev, O., Kuchuk, N., Mozhaiev, M., Tiulieniev, S., Gnusov, Y. et al. (2022). Devising a procedure for defining the general criteria of abnormal behavior of a computer system based on the improved criterion of uniformity of input data samples. Eastern-European Journal of Enterprise Technologies, 6 (4 (120)), 40–49. https://doi.org/10.15587/1729-4061.2022.269128
- Meleshko, Y., Raskin, L., Semenov, S., Sira, O. (2019). Methodology of probabilistic analysis of state dynamics of multidimensional semiMarkov dynamic systems. Eastern-European Journal of Enterprise Technologies, 6 (4 (102)), 6–13. https://doi.org/10.15587/1729-4061.2019.184637
- Semenov, S., Zhang, L., Cao, W., Bulba, S., Babenko, V., Davydov, V. (2021). Development of a fuzzy GERT-model for investigating common software vulnerabilities. Eastern-European Journal of Enterprise Technologies, 6 (2 (114)), 6–18. https://doi.org/10.15587/1729-4061.2021.243715
- Meleshko, Y., Yakymenko, M., Semenov, S. (2021). A Method of Detecting Bot Networks Based on Graph Clustering in the Recommendation System of Social Network. International Conference on Computational Linguistics and Intelligent Systems. Available at: https://ceur-ws.org/Vol-2870/paper92.pdf
- Semеnov, S., Gavrylenko, S., Chelak, V. (2016). Developing parametrical criterion for registering abnormal behavior in computer and telecommunication systems on the basis of economic tests. Actual problems of economics, 4 (178), 451–459.
- Angel, N. A., Ravindran, D., Vincent, P. M. D. R., Srinivasan, K., Hu, Y.-C. (2021). Recent Advances in Evolving Computing Paradigms: Cloud, Edge, and Fog Technologies. Sensors, 22 (1), 196. https://doi.org/10.3390/s22010196
- Khan, A. R. (2024). Dynamic Load Balancing in Cloud Computing: Optimized RL-Based Clustering with Multi-Objective Optimized Task Scheduling. Processes, 12 (3), 519. https://doi.org/10.3390/pr12030519
- Zhao, L., Gao, W., Fang, J. (2024). Optimizing Large Language Models on Multi-Core CPUs: A Case Study of the BERT Model. Applied Sciences, 14 (6), 2364. https://doi.org/10.3390/app14062364
- Dakić, V., Kovač, M., Slovinac, J. (2024). Evolving High-Performance Computing Data Centers with Kubernetes, Performance Analysis, and Dynamic Workload Placement Based on Machine Learning Scheduling. Electronics, 13 (13), 2651. https://doi.org/10.3390/electronics13132651
- Savi, M. A. (2023). Chaos Theory. Lectures on Nonlinear Dynamics, 283–299. https://doi.org/10.1007/978-3-031-45101-0_10
- Devaney, R. L. (2021). An Introduction to Chaotic Dynamical Systems. Chapman and Hall/CRC. https://doi.org/10.1201/9780429280801
- Göcs, L., Johanyák, Z. C. (2024). Identifying relevant features of CSE-CIC-IDS2018 dataset for the development of an intrusion detection system. Intelligent Data Analysis, 28 (6), 1527–1553. https://doi.org/10.3233/ida-230264
- CSE-CIC-IDS2018 on AWS. Available at: https://www.unb.ca/cic/datasets/ids-2018.html
- Almansoori, M., Telek, M. (2023). Anomaly Detection using combination of Autoencoder and Isolation Forest. 1st Workshop on Intelligent Infocommunication Networks, Systems and Services, 25–30. https://doi.org/10.3311/wins2023-005
- Ribeiro, D., Matos, L. M., Moreira, G., Pilastri, A., Cortez, P. (2022). Isolation Forests and Deep Autoencoders for Industrial Screw Tightening Anomaly Detection. Computers, 11 (4), 54. https://doi.org/10.3390/computers11040054
- Gavrylenko, S. Y., Sheverdin, I. V. (2021). Development of method to identify the computer system state based on the «isolation forest» algorithm. Radio Electronics, Computer Science, Control, 1 (1), 105–116. https://doi.org/10.15588/1607-3274-2021-1-11
- Semenov, S., Sira, O., Gavrylenko, S., Kuchuk, N. (2019). Identification of the state of an object under conditions of fuzzy input data. Eastern-European Journal of Enterprise Technologies, 1 (4 (97)), 22–30. https://doi.org/10.15587/1729-4061.2019.157085
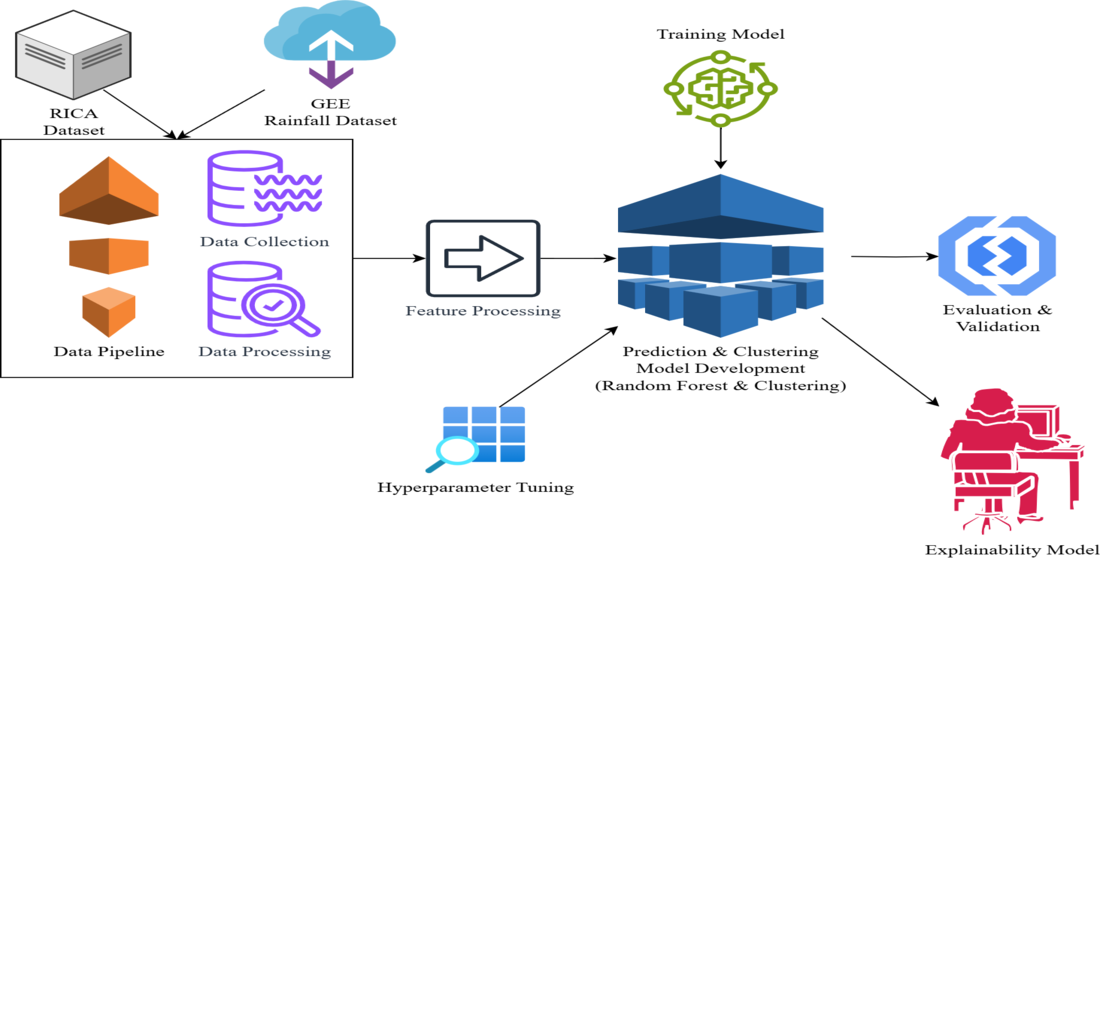
Downloads
Published
How to Cite
Issue
Section
License
Copyright (c) 2024 Yelyzaveta Meleshko, Mykola Yakymenko, Volodymyr Mikhav, Yaroslav Shulika, Viacheslav Davydov

This work is licensed under a Creative Commons Attribution 4.0 International License.
The consolidation and conditions for the transfer of copyright (identification of authorship) is carried out in the License Agreement. In particular, the authors reserve the right to the authorship of their manuscript and transfer the first publication of this work to the journal under the terms of the Creative Commons CC BY license. At the same time, they have the right to conclude on their own additional agreements concerning the non-exclusive distribution of the work in the form in which it was published by this journal, but provided that the link to the first publication of the article in this journal is preserved.
A license agreement is a document in which the author warrants that he/she owns all copyright for the work (manuscript, article, etc.).
The authors, signing the License Agreement with TECHNOLOGY CENTER PC, have all rights to the further use of their work, provided that they link to our edition in which the work was published.
According to the terms of the License Agreement, the Publisher TECHNOLOGY CENTER PC does not take away your copyrights and receives permission from the authors to use and dissemination of the publication through the world's scientific resources (own electronic resources, scientometric databases, repositories, libraries, etc.).
In the absence of a signed License Agreement or in the absence of this agreement of identifiers allowing to identify the identity of the author, the editors have no right to work with the manuscript.
It is important to remember that there is another type of agreement between authors and publishers – when copyright is transferred from the authors to the publisher. In this case, the authors lose ownership of their work and may not use it in any way.