Implementation of knowledge distillation in developing a prediction model to know the performance of air transportation vocational education using machine learning
DOI:
https://doi.org/10.15587/1729-4061.2024.318533Keywords:
strategic change management, knowledge management, organizational performance, machine learning, knowledge distillationAbstract
The object of this research is the performance of air transportation vocational education. The problem in this research that must be solved is the complexity of the model in machine learning which requires a long processing time and requires high resources, so the knowledge transfer process in knowledge distillation must be carried out carefully so that the student model can capture and reproduce knowledge from the teacher's model. without loss of accuracy and problems such as Good Corporate Governance, Organizational Flexibility, and Strategic Change Management variables, which are interrelated and difficult to model accurately. The results obtained are in the form of a model that can predict vocational education performance by utilizing machine learning and knowledge distillation. The interpretation of this research is to apply the XGBoost machine learning algorithm and knowledge distillation. The characteristics and characteristics obtained are that the teacher model has the best performance in terms of loss, while the student model with distillation shows a significant reduction in loss compared to training without distillation. Thus, the distillation process is proven to help student models capture knowledge from teacher models, producing prediction accuracy of up to 90 % and being an efficient alternative in predicting the influence of main factors on the performance of air transportation vocational education. These findings are expected to provide a significant contribution to the development of more efficient and effective prediction models in the context of vocational education, especially in the field of air transportation
References
- Gryshova, I., Kyzym, M., Khaustova, V., Korneev, V., Kramarev, H. (2020). Assessment of the Industrial Structure and its Influence on Sustainable Economic Development and Quality of Life of the Population of Different World Countries. Sustainability, 12 (5), 2072. https://doi.org/10.3390/su12052072
- Malik, S., Muhammad, K., Waheed, Y. (2023). Nanotechnology: A Revolution in Modern Industry. Molecules, 28 (2), 661. https://doi.org/10.3390/molecules28020661
- Angelopoulos, A., Michailidis, E. T., Nomikos, N., Trakadas, P., Hatziefremidis, A., Voliotis, S., Zahariadis, T. (2019). Tackling Faults in the Industry 4.0 Era – A Survey of Machine-Learning Solutions and Key Aspects. Sensors, 20 (1), 109. https://doi.org/10.3390/s20010109
- Tarihoran, A. D. B., Hubeis, M., Jahroh, S., Zulbainarni, N. (2023). Developing an Integrated Conceptual Model of Dynamic Capabilities for MSME in Agribusiness Sector: A Systematic Review. Proceedings of the Business Innovation and Engineering Conference (BIEC 2022), 61–79. https://doi.org/10.2991/978-94-6463-144-9_7
- Serin, F., Alisan, Y., Erturkler, M. (2022). Predicting bus travel time using machine learning methods with three-layer architecture. Measurement, 198, 111403. https://doi.org/10.1016/j.measurement.2022.111403
- Sun, X., Wandelt, S., Zhang, A. (2021). Technological and educational challenges towards pandemic-resilient aviation. Transport Policy, 114, 104–115. https://doi.org/10.1016/j.tranpol.2021.09.010
- de la Torre, R., Corlu, C. G., Faulin, J., Onggo, B. S., Juan, A. A. (2021). Simulation, Optimization, and Machine Learning in Sustainable Transportation Systems: Models and Applications. Sustainability, 13 (3), 1551. https://doi.org/10.3390/su13031551
- Nogueira, R. P. R., Melicio, R., Valério, D., Santos, L. F. F. M. (2023). Learning Methods and Predictive Modeling to Identify Failure by Human Factors in the Aviation Industry. Applied Sciences, 13 (6), 4069. https://doi.org/10.3390/app13064069
- Lin, X., Zhong, W., Lin, X., Zhou, Y., Jiang, L., Du-Ikonen, L., Huang, L. (2024). Component modeling and updating method of integrated energy systems based on knowledge distillation. Energy and AI, 16, 100350. https://doi.org/10.1016/j.egyai.2024.100350
- Zhang, Y., Shen, J., Li, J., Yao, X., Chen, X., Liu, D. (2024). A new lightweight framework based on knowledge distillation for reducing the complexity of multi-modal solar irradiance prediction model. Journal of Cleaner Production, 475, 143663. https://doi.org/10.1016/j.jclepro.2024.143663
- Li, Y., Li, P., Yan, D., Liu, Y., Liu, Z. (2024). Deep knowledge distillation: A self-mutual learning framework for traffic prediction. Expert Systems with Applications, 252, 124138. https://doi.org/10.1016/j.eswa.2024.124138
- Zhang, L., Liu, Y., Zeng, Z., Cao, Y., Wu, X., Xu, Y. et al. (2024). Package Arrival Time Prediction via Knowledge Distillation Graph Neural Network. ACM Transactions on Knowledge Discovery from Data, 18 (5), 1–19. https://doi.org/10.1145/3643033
- Alruwais, N., Zakariah, M. (2023). Evaluating Student Knowledge Assessment Using Machine Learning Techniques. Sustainability, 15 (7), 6229. https://doi.org/10.3390/su15076229
- Li, J., Li, Y., Xiang, X., Xia, S.-T., Dong, S., Cai, Y. (2020). TNT: An Interpretable Tree-Network-Tree Learning Framework using Knowledge Distillation. Entropy, 22 (11), 1203. https://doi.org/10.3390/e22111203
- Li, X., Dong, D., Liu, K., Zhao, Y., Li, M. (2022). Identification of Mine Mixed Water Inrush Source Based on Genetic Algorithm and XGBoost Algorithm: A Case Study of Huangyuchuan Mine. Water, 14 (14), 2150. https://doi.org/10.3390/w14142150
- Tseng, Y.-S., Yang, M.-H. (2023). Using Kernel Density Estimation in Knowledge Distillation to Construct the Prediction Model for Bipolar Disorder Patients. Applied Sciences, 13 (18), 10280. https://doi.org/10.3390/app131810280
- Phillips, P. A., Wright, C. (2009). E-business’s impact on organizational flexibility. Journal of Business Research, 62 (11), 1071–1080. https://doi.org/10.1016/j.jbusres.2008.09.014
- Arnold, V., Benford, T., Canada, J., Sutton, S. G. (2011). The role of strategic enterprise risk management and organizational flexibility in easing new regulatory compliance. International Journal of Accounting Information Systems, 12 (3), 171–188. https://doi.org/10.1016/j.accinf.2011.02.002
- Okorie, O., Subramoniam, R., Charnley, F., Patsavellas, J., Widdifield, D., Salonitis, K. (2020). Manufacturing in the Time of COVID-19: An Assessment of Barriers and Enablers. IEEE Engineering Management Review, 48 (3), 167–175. https://doi.org/10.1109/emr.2020.3012112
- Shukla, S. K., Sushil, Sharma, M. K. (2019). Managerial Paradox Toward Flexibility: Emergent Views Using Thematic Analysis of Literature. Global Journal of Flexible Systems Management, 20 (4), 349–370. https://doi.org/10.1007/s40171-019-00220-x
- Lee, J., Jang, D., Park, S. (2017). Deep Learning-Based Corporate Performance Prediction Model Considering Technical Capability. Sustainability, 9 (6), 899. https://doi.org/10.3390/su9060899
- Tutcu, B., Kayakuş, M., Terzioğlu, M., Ünal Uyar, G. F., Talaş, H., Yetiz, F. (2024). Predicting Financial Performance in the IT Industry with Machine Learning: ROA and ROE Analysis. Applied Sciences, 14 (17), 7459. https://doi.org/10.3390/app14177459
- Vukovic, D. B., Spitsina, L., Gribanova, E., Spitsin, V., Lyzin, I. (2023). Predicting the Performance of Retail Market Firms: Regression and Machine Learning Methods. Mathematics, 11 (8), 1916. https://doi.org/10.3390/math11081916
- Rithanasophon, T., Thitisiriwech, K., Kantavat, P., Kijsirikul, B., Iwahori, Y., Fukui, S. et al. (2023). Quality of Life Prediction on Walking Scenes Using Deep Neural Networks and Performance Improvement Using Knowledge Distillation. Electronics, 12 (13), 2907. https://doi.org/10.3390/electronics12132907
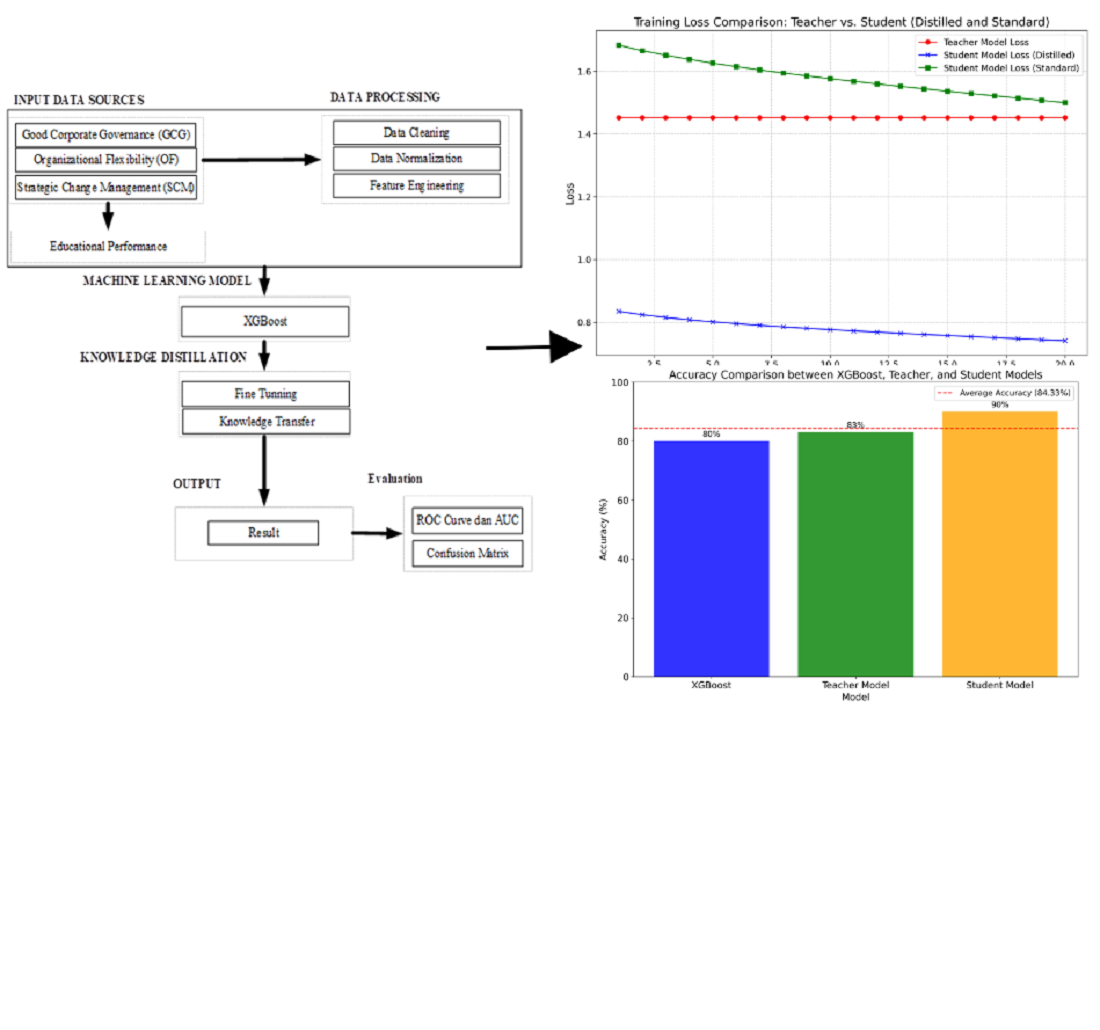
Downloads
Published
How to Cite
Issue
Section
License
Copyright (c) 2024 Daniel D Romani, Darmeli Nasution, Okvi Nugroho

This work is licensed under a Creative Commons Attribution 4.0 International License.
The consolidation and conditions for the transfer of copyright (identification of authorship) is carried out in the License Agreement. In particular, the authors reserve the right to the authorship of their manuscript and transfer the first publication of this work to the journal under the terms of the Creative Commons CC BY license. At the same time, they have the right to conclude on their own additional agreements concerning the non-exclusive distribution of the work in the form in which it was published by this journal, but provided that the link to the first publication of the article in this journal is preserved.
A license agreement is a document in which the author warrants that he/she owns all copyright for the work (manuscript, article, etc.).
The authors, signing the License Agreement with TECHNOLOGY CENTER PC, have all rights to the further use of their work, provided that they link to our edition in which the work was published.
According to the terms of the License Agreement, the Publisher TECHNOLOGY CENTER PC does not take away your copyrights and receives permission from the authors to use and dissemination of the publication through the world's scientific resources (own electronic resources, scientometric databases, repositories, libraries, etc.).
In the absence of a signed License Agreement or in the absence of this agreement of identifiers allowing to identify the identity of the author, the editors have no right to work with the manuscript.
It is important to remember that there is another type of agreement between authors and publishers – when copyright is transferred from the authors to the publisher. In this case, the authors lose ownership of their work and may not use it in any way.