Investigation of the relationship between the dynamics of GDP and economic sentiment index
DOI:
https://doi.org/10.15587/1729-4061.2022.265656Keywords:
Economic Sentiment Index, confidence indices, short-term cyclicality, technology industry, critical points, econometric modelsAbstract
The paper develops and presents an appropriate model toolkit that allows assessing the relationship between the calculated indices of economic sentiment and confidence for the main types of economic activity. The aim of the study is to experimentally substantiate the relevance of data on the opinions of technological economic agents and assess the value of this information for the statistical description and analysis of macroeconomic trends, including economic cycles and unforeseen and prolonged crises. The main hypothesis about the cyclical sensitivity of composite indices, in particular the index of economic sentiment in relation to the dynamics of the physical volume of GDP, is tested. The authors calculate a composite indicator of aggregate economic sentiment and, based on a consistent analysis of the relationship between the index of physical volume of GDP and the indicator of economic sentiment, identify aggregate empirical patterns and features of the cyclical development of technological enterprises. Accordingly, the turning points of the business cycle are discussed and the leading nature of the proposed index of economic sentiment is affirmed. The importance of the composite indicators in the economic analysis of entrepreneurial behavior in the implementation of technological innovations is shown.
The nature of the calculated Economic Sentiment Index was established, and its predictive capabilities for monthly and annual real GDP growth rates using autoregression and error correction models were investigated. The stages of calculating and setting indices with the application of the DEMETRA+ statistical package were implemented.
References
- J Rubio-Romero, J. C., Pardo-Ferreira, M. del C., De la Varga-Salto, J., Galindo-Reyes, F. (2018). Composite leading indicator to assess the resilience engineering in occupational health & safety in municipal solid waste management companies. Safety Science, 108, 161–172. doi: https://doi.org/10.1016/j.ssci.2018.04.014
- Romer, P. M. (1990). Endogenous Technological Change. Journal of Political Economy, 98 (5, Part 2), S71–S102. doi: https://doi.org/10.1086/261725
- Aghion, P., Howitt, P. (1992). A Model of Growth Through Creative Destruction. Econometrica, 60 (2), 323. doi: https://doi.org/10.2307/2951599
- Arif, S., Lee, C. M. C. (2014). Aggregate Investment and Investor Sentiment. Review of Financial Studies, 27 (11), 3241–3279. doi: https://doi.org/10.1093/rfs/hhu054
- Mclean, R. D., Zhao, M. (2014). The Business Cycle, Investor Sentiment, and Costly External Finance. The Journal of Finance, 69 (3), 1377–1409. doi: https://doi.org/10.1111/jofi.12047
- Romer, C. D. (1999). Changes in Business Cycles: Evidence and Explanations. Journal of Economic Perspectives, 13 (2), 23–44. doi: https://doi.org/10.1257/jep.13.2.23
- Zwetsloot, G., Leka, S., Kines, P., Jain, A. (2020). Vision zero: Developing proactive leading indicators for safety, health and wellbeing at work. Safety Science, 130, 104890. doi: https://doi.org/10.1016/j.ssci.2020.104890
- Nilsson, R. Country Specific or Harmonised Confidence Indicators: EC And OECD Practices. Available at: https://www.oecd.org/sdd/leading-indicators/33654955.pdf
- The use of short-term indicators and survey data for predicting turning points in economic activity (2016). OECD Statistics Working Papers. doi: https://doi.org/10.1787/5jlz4gs2pkhf-en
- Sauer, S., Wohlrabe, K. (2018). The New İFO Business Climate Index for Germany. CESifo Forum, 19 (2), 59–64. Available at: https://www.econstor.eu/bitstream/10419/181211/1/cesifo-forum-v19-y2018-i2-p59-64.pdf
- Zeng, Q., Beelaerts van Blokland, W. W. A., Santema, S. C., Lodewijks, G. (2020). Composite Indicators of Company Performance: A Literature Survey. Performance Improvement Quarterly, 33 (4), 385–418. doi: https://doi.org/10.1002/piq.21328
- Abberger, K., Graff, M., Siliverstovs, B., Sturm, J.-E. (2014). The KOF Economic Barometer, Version 2014: A Composite Leading Indicator for the Swiss Business Cycle. SSRN Electronic Journal. doi: https://doi.org/10.2139/ssrn.2408944
- Kitrar, L., Lipkind, T. (2021). The relationship of economic sentiment and GDP growth in Russia in light of the Covid-19 crisis. Entrepreneurial Business and Economics Review, 9 (1), 7–29. doi: https://doi.org/10.15678/eber.2021.090101
- Mourougane, A., Roma, M. (2003). Can confidence indicators be useful to predict short term real GDP growth? Applied Economics Letters, 10 (8), 519–522. doi: https://doi.org/10.1080/1350485032000100305
- Čižmešija, M., Škrinjarić, T. (2021). Economic sentiment and business cycles: A spillover methodology approach. Economic Systems, 45 (3), 100770. doi: https://doi.org/10.1016/j.ecosys.2020.100770
- Christiansen, C., Eriksen, J. N., Møller, S. V. (2014). Forecasting US recessions: The role of sentiment. Journal of Banking & Finance, 49, 459–468. doi: https://doi.org/10.1016/j.jbankfin.2014.06.017
- de Bondt, G. J. (2018). A PMI-Based Real GDP Tracker for the Euro Area. Journal of Business Cycle Research, 15 (2), 147–170. doi: https://doi.org/10.1007/s41549-018-0032-2
- Biau, O., D’Elia, A. (2011). Is There a Decoupling Between Soft and Hard Data? The Relationship Between GDP Growth and the ESI. Fifth Joint EU/OECD Workshop on on business and consumer surveys. Brussels. Available at: https://www.oecd.org/sdd/leading-indicators/49016412.pdf
- van Aarle, B., Moons, C. (2017). Sentiment and Uncertainty Fluctuations and Their Effects on the Euro Area Business Cycle. Journal of Business Cycle Research, 13 (2), 225–251. doi: https://doi.org/10.1007/s41549-017-0020-y
- Handbook on Constructing Composite Indicators: Methodology and User Guide (2008). OECD. Available at: https://www.oecd.org/sdd/42495745.pdf
- OECD System of Composite Leading Indicators (2012). OECD. Available at: http://www.oecd.org/std/leading-indicators/41629509.pdf
- Cieślik, A., Ghodsi, M. (2021). Economic sentiment indicators and foreign direct investment: Empirical evidence from European Union countries. International Economics, 168, 56–75. doi: https://doi.org/10.1016/j.inteco.2021.07.001
- Business Sentiment Index. Business Environment Profiles - United States. Available at: https://www.ibisworld.com/us/bed/business-sentiment-index/88232/
- De Jongh, J., Mncayi, P. (2018). An econometric analysis on the impact of business confidence and investment on economic growth in post-apartheid South Africa. International Journal of Economics and Finance Studies, 10 (01), 115–133.
- Annual Report (2013). European Central Bank. Available at: https://www.ecb.europa.eu/pub/pdf/annrep/ar2013en.pdf
- Katona, G. (1975). Psychological Economics. Elsevier.
- Malgarini, M., Margani, P. (2007). Psychology, consumer sentiment and household expenditures. Applied Economics, 39 (13), 1719–1729. doi: https://doi.org/10.1080/00036840600606351
- Fuhrer, J. C. (1993). What role does consumer sentiment play in the U.S. macroeconomy? New England Economic Review. Available at: https://www.bostonfed.org/publications/new-england-economic-review/1993-issues/issue-january-february-1993/what-role-does-consumer-sentiment-play-in-the-us-macroeconomy.aspx
- Batchelor, R., Dua, P. (1998). Improving macro-economic forecasts: The role of consumer confidence. International Journal of Forecasting, 14 (1), 71–81. doi: https://doi.org/10.1016/s0169-2070(97)00052-6
- Confidence indicators and economic developments (2013). ECB Monthly Bulletin. Available at: https://www.ecb.europa.eu/pub/pdf/other/art1_mb201301en_pp45-58en.pdf
- Kheyfets, B., Chernova, V. (2021). Comparative assessment of the influence of a technological factor on economic growth. Eastern-European Journal of Enterprise Technologies, 1 (13 (109)), 6–15. doi: https://doi.org/10.15587/1729-4061.2021.225526
- Simkins, S. (1995). Forecasting with vector autoregressive (VAR) models subject to business cycle restrictions. International Journal of Forecasting, 11 (4), 569–583. doi: https://doi.org/10.1016/0169-2070(95)00616-8
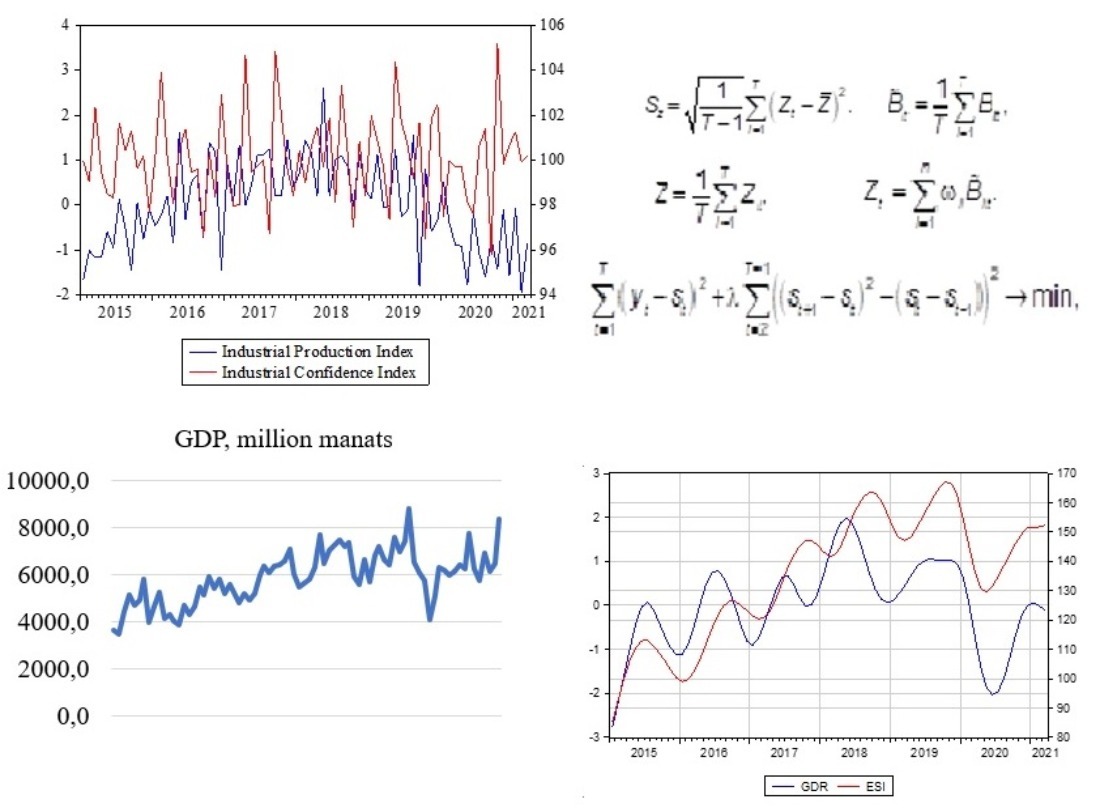
Downloads
Published
How to Cite
Issue
Section
License
Copyright (c) 2022 Arzu Huseynova, Tarana Aliyeva, Ulviyya Rzayeva

This work is licensed under a Creative Commons Attribution 4.0 International License.
The consolidation and conditions for the transfer of copyright (identification of authorship) is carried out in the License Agreement. In particular, the authors reserve the right to the authorship of their manuscript and transfer the first publication of this work to the journal under the terms of the Creative Commons CC BY license. At the same time, they have the right to conclude on their own additional agreements concerning the non-exclusive distribution of the work in the form in which it was published by this journal, but provided that the link to the first publication of the article in this journal is preserved.
A license agreement is a document in which the author warrants that he/she owns all copyright for the work (manuscript, article, etc.).
The authors, signing the License Agreement with TECHNOLOGY CENTER PC, have all rights to the further use of their work, provided that they link to our edition in which the work was published.
According to the terms of the License Agreement, the Publisher TECHNOLOGY CENTER PC does not take away your copyrights and receives permission from the authors to use and dissemination of the publication through the world's scientific resources (own electronic resources, scientometric databases, repositories, libraries, etc.).
In the absence of a signed License Agreement or in the absence of this agreement of identifiers allowing to identify the identity of the author, the editors have no right to work with the manuscript.
It is important to remember that there is another type of agreement between authors and publishers – when copyright is transferred from the authors to the publisher. In this case, the authors lose ownership of their work and may not use it in any way.