Formation of a typical form of an object image in a series of digital frames
DOI:
https://doi.org/10.15587/1729-4061.2022.266988Keywords:
transfer function, OLS-evaluation of parameters, linear correlation coefficients, typical image formAbstract
A computational method for the automated formation of a typical form of a digital image of the investigated objects on a series of digital frames has been developed. Due to the imperfection of the mounting of digital cameras, as well as their automated mounts, their immobility at shooting during exposure time can be disturbed, which leads to the formation of "blurred" images of objects of various forms.
Due to such inaccuracies in the tracking of objects on digital frames, even in one series, the typical form of the image of objects can vary from frame to frame. This fact of the difference in the standard form significantly complicates the execution of various image processing tasks.
In order to simplify the evaluation of the image parameters of objects in a series of digital frames, it has been proposed to use a typical image on a digital frame corresponding to the average image of objects as a model of object images. In this case, the appearance of the image of the object, its form, the distribution of brightness in the image will be determined only by the typical image.
This paper proposes a computational method for the automated formation and evaluation of the typical form of the image of an object in a digital frame based on the initial data – the actual given digital frame. This computational method is based on the selection of single images of objects and the formation of their rectangular area. Next, the offset is evaluated, and the selected single images of objects are normalized to calculate the typical form of the object image.
Using the method makes it possible to highlight objects against the background of noise and reduce the number of false detections. It is recommended to apply the method only in the case when the frames have defects and "blurs" during the shooting, otherwise there will be unreasonable additional computational costs.
The developed computational method was successfully tested in practice within the framework of the CoLiTec project and implemented in the intraframe processing unit of the Lemur software.
References
- Smith, G. E. (2010). Nobel Lecture: The invention and early history of the CCD. Reviews of Modern Physics, 82 (3), 2307–2312. doi: https://doi.org/10.1103/revmodphys.82.2307
- Khlamov, S., Vlasenko, V., Savanevych, V., Briukhovetskyi, O., Trunova, T., Chelombitko, V., Tabakova, I. (2022). Development of computational method for matched filtration with analytical profile of the blurred digital image. Eastern-European Journal of Enterprise Technologies, 5 (4 (119)), 24–32. doi: https://doi.org/10.15587/1729-4061.2022.265309
- Steger, C., Ulrich, M., Wiedemann, C. (2018). Machine vision algorithms and applications. John Wiley & Sons, 516.
- Cavuoti, S., Brescia, M., Longo, G. (2012). Data mining and knowledge discovery resources for astronomy in the web 2.0 age. Software and Cyberinfrastructure for Astronomy II. doi: https://doi.org/10.1117/12.925321
- Zhang, Y., Zhao, Y., Cui, C. (2002). Data mining and knowledge discovery in database of astronomy. Progress in Astronomy, 20 (4), 312–323.
- Mykhailova, L., Savanevych, V., Sokovikova, N., Bezkrovniy, M., Khlamov, S., Pogorelov, A. (2014). Method of maximum likelihood estimation of compact group objects location on CCD-frame. Eastern-European Journal of Enterprise Technologies, 5 (4 (71)), 16–21. doi: https://doi.org/10.15587/1729-4061.2014.28028
- Kuz'min, S. Z. (2000). Tsifrovaya radiolokatsiya. Vvedenie v teoriyu. Kyiv: Izdatel'stvo KvіTS, 428.
- Khlamov, S., Savanevych, V. (2020). Big Astronomical Datasets and Discovery of New Celestial Bodies in the Solar System in Automated Mode by the CoLiTec Software. Knowledge Discovery in Big Data from Astronomy and Earth Observation, 331–345. doi: https://doi.org/10.1016/b978-0-12-819154-5.00030-8
- Akhmetov, V., Khlamov, S., Dmytrenko, A. (2018). Fast Coordinate Cross-Match Tool for Large Astronomical Catalogue. Advances in Intelligent Systems and Computing III, 3–16. doi: https://doi.org/10.1007/978-3-030-01069-0_1
- Vavilova, I. B., Shatokhina, S. V., Pakuliak, L. K., Yizhakevych, O. M., Eglitis, I., Andruk, V. M., Protsyuk, Yu. I. (2019). Astrometry and photometry of asteroids from the UkrVO database of astroplates. Proceedings of the International Astronomical Union, 15 (S364), 239–245. doi: https://doi.org/10.1017/s1743921322000047
- Dearborn, D. P. S., Miller, P. L. (2014). Defending Against Asteroids and Comets. Handbook of Cosmic Hazards and Planetary Defense, 1–18. doi: https://doi.org/10.1007/978-3-319-02847-7_59-1
- Klette, R. (2014). Concise computer vision. An Introduction into Theory and Algorithms. Springer, 429. doi: https://doi.org/10.1007/978-1-4471-6320-6
- Savanevych, V. E., Khlamov, S. V., Akhmetov, V. S., Briukhovetskyi, A. B., Vlasenko, V. P., Dikov, E. N. et al. (2022). CoLiTecVS software for the automated reduction of photometric observations in CCD-frames. Astronomy and Computing, 40, 100605. doi: https://doi.org/10.1016/j.ascom.2022.100605
- Khlamov, S., Savanevych, V., Briukhovetskyi, O., Oryshych, S. (2016). Development of computational method for detection of the object’s near-zero apparent motion on the series of ccd-frames. Eastern-European Journal of Enterprise Technologies, 2 (9 (80)), 41–48. doi: https://doi.org/10.15587/1729-4061.2016.65999
- Vavilova, I., Pakuliak, L., Babyk, I., Elyiv, A., Dobrycheva, D., Melnyk, O. (2020). Surveys, Catalogues, Databases, and Archives of Astronomical Data. Knowledge Discovery in Big Data from Astronomy and Earth Observation, 57–102. doi: https://doi.org/10.1016/b978-0-12-819154-5.00015-1
- Minaee, S., Boykov, Y. Y., Porikli, F., Plaza, A. J., Kehtarnavaz, N., Terzopoulos, D. (2021). Image Segmentation Using Deep Learning: A Survey. IEEE Transactions on Pattern Analysis and Machine Intelligence, 44 (7). doi: https://doi.org/10.1109/tpami.2021.3059968
- Kobzar', A. I. (2006). Prikladnaya matematicheskaya statistika. Dlya inzhenerov i nauchnykh rabotnikov. Moscow: FIZMATLI, 816.
- Khlamov, S., Savanevych, V., Tabakova, I., Trunova, T. (2022). The astronomical object recognition and its near-zero motion detection in series of images by in situ modeling. 2022 29th International Conference on Systems, Signals and Image Processing (IWSSIP). doi: https://doi.org/10.1109/iwssip55020.2022.9854475
- Akhmetov, V., Khlamov, S., Tabakova, I., Hernandez, W., Nieto Hipolito, J. I., Fedorov, P. (2019). New approach for pixelization of big astronomical data for machine vision purpose. 2019 IEEE 28th International Symposium on Industrial Electronics (ISIE). doi: https://doi.org/10.1109/isie.2019.8781270
- Khlamov, S., Tabakova, I., Trunova, T. (2022). Recognition of the astronomical images using the Sobel filter. 2022 29th International Conference on Systems, Signals and Image Processing (IWSSIP). doi: https://doi.org/10.1109/iwssip55020.2022.9854425
- Bishop, C. M. (2013). Model-based machine learning. Philosophical Transactions of the Royal Society A: Mathematical, Physical and Engineering Sciences, 371 (1984), 20120222. doi: https://doi.org/10.1098/rsta.2012.0222
- Burger, W., Burge, M. (2010). Principles of digital image processing: core algorithms. Springer, 332. doi: https://doi.org/10.1007/978-1-84800-195-4
- Gonzalez, R., Woods, R. (2018). Digital image processing. New York, NY: Pearson, 1168.
- Rubin, B. (2015). Introduction to Radon transforms. With Elements of Fractional Calculus and Harmonic Analysis. Encyclopedia of Mathematics and its Applications. Cambridge University Press, 596.
- Brandt, A. (2011). Noise and vibration analysis: signal analysis and experimental procedures. John Wiley & Sons, 464. doi: https://doi.org/10.1002/9780470978160
- Dadkhah, M., Lyashenko, V. V., Deineko, Z. V., Shamshirband, S., Jazi, M. D. (2019). Methodology of wavelet analysis in research of dynamics of phishing attacks. International Journal of Advanced Intelligence Paradigms, 12 (3/4), 220. doi: https://doi.org/10.1504/ijaip.2019.098561
- Jorgensen, B. (2012). Statistical properties of the generalized inverse Gaussian distribution. Springer, 188. doi: https://doi.org/10.1007/978-1-4612-5698-4
- Lemur software. CoLiTec. Available at: https://colitec.space/
- Khlamov, S., Savanevych, V., Briukhovetskyi, O., Pohorelov, A., Vlasenko, V., Dikov, E. (2018). CoLiTec Software for the Astronomical Data Sets Processing. 2018 IEEE Second International Conference on Data Stream Mining & Processing (DSMP). doi: https://doi.org/10.1109/dsmp.2018.8478504
- Kashuba, S., Tsvetkov, M., Bazyey, N., Isaeva, E., Golovnia, V. (2018). The Simeiz plate collection of the ODESSA astronomical observatory. Proceedings of the XI Bulgarian-Serbian Astronomical Conference, 207–216.
- Molotov, I. et al. (2009). ISON worldwide scientific optical network. Fifth European Conference on Space Debris, ESA.
- Mingmuang, Y., Tummuangpak, P., Asanok, K., Jaroenjittichai, P. (2019). The mass distribution and the rotation curve of the Milky Way Galaxy using NARIT 4.5 m small radio telescope and the 2.3 m Onsala radio telescope. Journal of Physics: Conference Series, 1380 (1), 012028. doi: https://doi.org/10.1088/1742-6596/1380/1/012028
- Soyfer, V. A. (Ed.) (2003). Metody komp'yuternoy obrabotki izobrazheniy. Moscow: Fizmatlit, 784.
- Sergienko, A. B. (2011). Tsifrovaya obrabotka signalov. Sankt-Peterburg: BKhV-Peterburg, 768.
- Le, D.-H., Pham, C.-K., Nguyen, T. T. T., Bui, T. T. (2012). Parameter extraction and optimization using Levenberg-Marquardt algorithm. 2012 Fourth International Conference on Communications and Electronics (ICCE). doi: https://doi.org/10.1109/cce.2012.6315945
- Khlamov, S., Savanevych, V., Briukhovetskyi, O., Tabakova, I., Trunova, T. (2022). Data Mining of the Astronomical Images by the CoLiTec Software. CEUR Workshop Proceedings, 3171, 1043–1055.
- Akhmetov, V., Khlamov, S., Khramtsov, V., Dmytrenko, A. (2019). Astrometric Reduction of the Wide-Field Images. Advances in Intelligent Systems and Computing, 896–909. doi: https://doi.org/10.1007/978-3-030-33695-0_58
- Buslov, P., Shvedun, V., Streltsov, V. (2018). Modern Tendencies of Data Protection in the Corporate Systems of Information Consolidation. 2018 International Scientific-Practical Conference Problems of Infocommunications. Science and Technology (PIC S&T). doi: https://doi.org/10.1109/infocommst.2018.8632089
- Baranova, V., Zeleniy, O., Deineko, Z., Bielcheva, G., Lyashenko, V. (2019). Wavelet Coherence as a Tool for Studying of Economic Dynamics in Infocommunication Systems. 2019 IEEE International Scientific-Practical Conference Problems of Infocommunications, Science and Technology (PIC S&T). doi: https://doi.org/10.1109/picst47496.2019.9061301
- Dombrovska, S., Shvedun, V., Streltsov, V., Husarov, K. (2018). The prospects of integration of the advertising market of Ukraine into the global advertising business. Problems and Perspectives in Management, 16 (2), 321–330. doi: https://doi.org/10.21511/ppm.16(2).2018.29
- Wang, J., Cai, D., Wen, Y. (2011). Comparison of matched filter and dechirp processing used in Linear Frequency Modulation. 2011 IEEE 2nd International Conference on Computing, Control and Industrial Engineering. doi: https://doi.org/10.1109/ccieng.2011.6008069
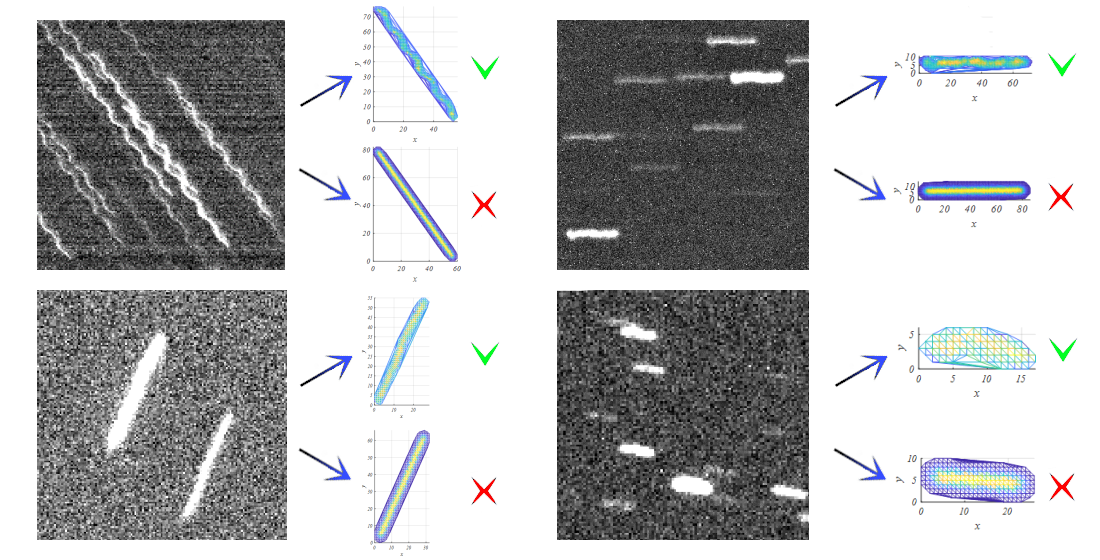
Downloads
Published
How to Cite
Issue
Section
License
Copyright (c) 2022 Vadym Savanevych, Sergii Khlamov, Vladimir Vlasenko, Zhanna Deineko, Oleksandr Briukhovetskyi, Iryna Tabakova, Tetiana Trunova

This work is licensed under a Creative Commons Attribution 4.0 International License.
The consolidation and conditions for the transfer of copyright (identification of authorship) is carried out in the License Agreement. In particular, the authors reserve the right to the authorship of their manuscript and transfer the first publication of this work to the journal under the terms of the Creative Commons CC BY license. At the same time, they have the right to conclude on their own additional agreements concerning the non-exclusive distribution of the work in the form in which it was published by this journal, but provided that the link to the first publication of the article in this journal is preserved.
A license agreement is a document in which the author warrants that he/she owns all copyright for the work (manuscript, article, etc.).
The authors, signing the License Agreement with TECHNOLOGY CENTER PC, have all rights to the further use of their work, provided that they link to our edition in which the work was published.
According to the terms of the License Agreement, the Publisher TECHNOLOGY CENTER PC does not take away your copyrights and receives permission from the authors to use and dissemination of the publication through the world's scientific resources (own electronic resources, scientometric databases, repositories, libraries, etc.).
In the absence of a signed License Agreement or in the absence of this agreement of identifiers allowing to identify the identity of the author, the editors have no right to work with the manuscript.
It is important to remember that there is another type of agreement between authors and publishers – when copyright is transferred from the authors to the publisher. In this case, the authors lose ownership of their work and may not use it in any way.