Application of a data stratification approach in computer medical monitoring systems
DOI:
https://doi.org/10.15587/1729-4061.2024.298805Keywords:
data stratification, anomaly detection, fuzzy clustering, neural network, sensitivity analysisAbstract
The research object is the processes occurring in the data stratification subsystem in the medical monitoring computer system, which is part of the decision support system. Such a subsystem aims to solve data analysis and processing problems in the medical monitoring system. Among them, the problems of anomaly detection, data marking, state determination, selection of the most informative variables, and justification of decision-making are selected for solving.
The paper proposes the structure and implementation of the data stratification subsystem in the decision support system. The subsystem contains modules for anomaly detection and an autoencoder, a clustering module using an advanced multi-agent clustering method, and a state detection module with a modified neural network training procedure.
Modules of the stratification subsystem have been tested using diabetes monitoring data. The results showed that the clustering module provides 25.7 % lower accuracy than the achieved neural network. The accuracy difference is explained by the complexity of the data and the lack of adaptability of the proposed method to solving such problems. It is shown that the method of determining the overall informativeness of variables covers 90 % informativeness with 10 variables, comparable to the variability data. In general, the flexible nature of the proposed stratification subsystem allows for solving the problems.
The proposed stratification subsystem offers a robust solution for improving treatment strategies and decision-making in computer medical monitoring systems. Its versatility allows it to be used in any system where decision support is needed, providing valuable information about informative variables and decision-making features for clinicians and researchers
References
- Logeshwaran, J., Malik, J. A., Adhikari, N., Joshi, S. S., Bishnoi, P. (2022). IoT-TPMS: An innovation development of triangular patient monitoring system using medical internet of things. International Journal of Health Sciences, 6 (S5), 9070–9084. https://doi.org/10.53730/ijhs.v6ns5.10765
- Humayun, M., Jhanjhi, N. Z., Almotilag, A., Almufareh, M. F. (2022). Agent-Based Medical Health Monitoring System. Sensors, 22 (8), 2820. https://doi.org/10.3390/s22082820
- Yu, M., Li, G., Jiang, D., Jiang, G., Tao, B., Chen, D. (2019). Hand medical monitoring system based on machine learning and optimal EMG feature set. Personal and Ubiquitous Computing, 27 (6), 1991–2007. https://doi.org/10.1007/s00779-019-01285-2
- Singh, V. J., Sharma, P., Mehta, D. A. (2023). Big Data Analytics in Healthcare: Opportunities and Challenges. International Journal of Advanced Research in Science, Communication and Technology, 275–282. https://doi.org/10.48175/ijarsct-9414
- Yang, J., Soltan, A. A. S., Yang, Y., Clifton, D. A. (2022). Algorithmic Fairness and Bias Mitigation for Clinical Machine Learning: Insights from Rapid COVID-19 Diagnosis by Adversarial Learning. https://doi.org/10.1101/2022.01.13.22268948
- Ferrario, A., Gloeckler, S., Biller-Andorno, N. (2023). AI knows best? Avoiding the traps of paternalism and other pitfalls of AI-based patient preference prediction. Journal of Medical Ethics, 49 (3), 185–186. https://doi.org/10.1136/jme-2023-108945
- Goel, K. (2023). Price Discrimination and Product Variety: The Case of Implantable Medical Devices. SSRN Electronic Journal. https://doi.org/10.2139/ssrn.4467475
- Tamura, M., Nakano, S., Sugahara, T. (2018). Reimbursement pricing for new medical devices in Japan: Is the evaluation of innovation appropriate? The International Journal of Health Planning and Management, 34 (2), 583–593. https://doi.org/10.1002/hpm.2719
- Strilets, V., Donets, V., Ugryumov, M., Artiuch, S., Zelenskyi, R., Goncharova, T. (2022). Agent-oriented data clustering for medical monitoring. Radioelectronic and Computer Systems, 1, 103–114. https://doi.org/10.32620/reks.2022.1.08
- Strilets, V., Bakumenko, N., Chernysh, S., Ugryumov, M., Donets, V. (2020). Application of Artificial Neural Networks in the Problems of the Patient’s Condition Diagnosis in Medical Monitoring Systems. Advances in Intelligent Systems and Computing, 173–185. https://doi.org/10.1007/978-3-030-37618-5_16
- Bakumenko, N., Chernysh, S., Bezlyubchenko, A., Goryachaya, V., Donets, V., Strilets, V. et al. (2021). Stratification of Patients in Medical Monitoring Systems based on Machine Learning Methods. https://doi.org/10.13140/RG.2.2.11832.52482
- Donets, V., Strilets, V., Ugryumov, M., Shevchenko, D., Prokopovych, S., Chagovets, L. (2023). Methodology of the countries’ economic development data analysis. System research and information technologies, 4, 21–36. https://doi.org/10.20535/SRIT.2308-8893.2023.4.02
- Lykhach, O., Ugryumov, M., Shevchenko, D., Shmatkov, S. (2022). Anomaly detection methods in sample datasets when managing processes in systems by the state. Bulletin of V.N. Karazin Kharkiv National University, Series «Mathematical Modeling. Information Technology. Automated Control Systems», 53, 21–40. https://doi.org/10.26565/2304-6201-2022-53-03
- Shevchenko, D., Ugryumov, M., Artiukh, S. (2023). Monitoring data aggregation of dynamic systems using information technologies. Innovative Technologies and Scientific Solutions for Industries, 1 (23), 123–131. https://doi.org/10.30837/itssi.2023.23.123
- Prihanditya, H. A., Alamsyah, A. (2020). The Implementation of Z-Score Normalization and Boosting Techniques to Increase Accuracy of C4.5 Algorithm in Diagnosing Chronic Kidney Disease. Journal of Soft Computing Exploration, 1 (1). https://doi.org/10.52465/joscex.v1i1.8
- Liu, F. T., Ting, K. M., Zhou, Z.-H. (2008). Isolation Forest. 2008 Eighth IEEE International Conference on Data Mining. https://doi.org/10.1109/icdm.2008.17
- Hinton, G. E., Srivastava, N., Krizhevsky, A., Sutskever, I., Salakhutdinov, R. R. (2012). Improving neural networks by preventing co-adaptation of feature detectors. arXiv. https://doi.org/10.48550/arXiv.1207.0580
- Ioffe, S., Szegedy, C. (2015). Batch normalization: Accelerating deep network training by reducing internal covariate shift. International conference on machine learning. arXiv. https://doi.org/10.48550/arXiv.1502.03167
- Pal, N. R., Bezdek, J. C. (1995). On cluster validity for the fuzzy c-means model. IEEE Transactions on Fuzzy Systems, 3 (3), 370–379. https://doi.org/10.1109/91.413225
- Bridle, J. S. (1990). Probabilistic Interpretation of Feedforward Classification Network Outputs, with Relationships to Statistical Pattern Recognition. Neurocomputing, 227–236. https://doi.org/10.1007/978-3-642-76153-9_28
- Li, S., Song, Z., Xia, Y., Yu, T., Zhou, T. (2023). The Closeness of In-Context Learning and Weight Shifting for Softmax Regression. arXiv. https://doi.org/10.48550/arXiv.2304.13276
- Zhang, Z., & Sabuncu, M.R. (2018). Generalized Cross Entropy Loss for Training Deep Neural Networks with Noisy Labels. arXiv. https://doi.org/10.48550/arXiv.1805.07836
- Qi, Z., Khorram, S., Fuxin, L. (2020). Visualizing Deep Networks by Optimizing with Integrated Gradients. Proceedings of the AAAI Conference on Artificial Intelligence, 34 (07), 11890–11898. https://doi.org/10.1609/aaai.v34i07.6863
- Enguehard, J. (2023). Sequential Integrated Gradients: a simple but effective method for explaining language models. Findings of the Association for Computational Linguistics: ACL 2023. https://doi.org/10.18653/v1/2023.findings-acl.477
- Lundstrom, D., Huang, T., Razaviyayn, M. (2022). A Rigorous Study of Integrated Gradients Method and Extensions to Internal Neuron Attributions. arXiv. https://doi.org/10.48550/arXiv.2202.11912
- Daher, M., Al Rifai, M., Kherallah, R. Y., Rodriguez, F., Mahtta, D., Michos, E. D. et al. (2021). Gender disparities in difficulty accessing healthcare and cost-related medication non-adherence: The CDC behavioral risk factor surveillance system (BRFSS) survey. Preventive Medicine, 153, 106779. https://doi.org/10.1016/j.ypmed.2021.106779
- Iwendi, C., Bashir, A. K., Peshkar, A., Sujatha, R., Chatterjee, J. M., Pasupuleti, S. et al. (2020). COVID-19 Patient Health Prediction Using Boosted Random Forest Algorithm. Frontiers in Public Health, 8. https://doi.org/10.3389/fpubh.2020.00357
- Pereira, J. P. B., Stroes, E. S. G., Zwinderman, A. H., Levin, E. (2022). Covered Information Disentanglement: Model Transparency via Unbiased Permutation Importance. Proceedings of the AAAI Conference on Artificial Intelligence, 36 (7), 7984–7992. https://doi.org/10.1609/aaai.v36i7.20769
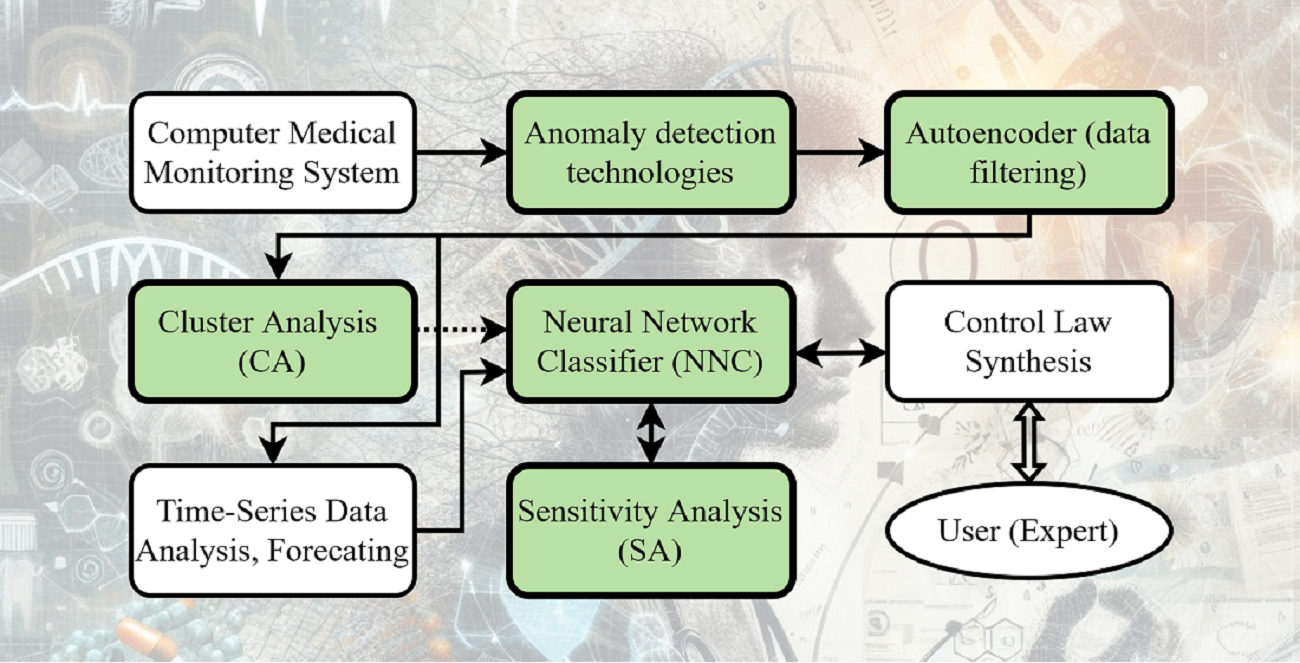
Downloads
Published
How to Cite
Issue
Section
License
Copyright (c) 2024 Volodymyr Donets, Dmytro Shevchenko, Maksym Holikov, Viktoriia Strilets, Serhiy Shmatkov

This work is licensed under a Creative Commons Attribution 4.0 International License.
The consolidation and conditions for the transfer of copyright (identification of authorship) is carried out in the License Agreement. In particular, the authors reserve the right to the authorship of their manuscript and transfer the first publication of this work to the journal under the terms of the Creative Commons CC BY license. At the same time, they have the right to conclude on their own additional agreements concerning the non-exclusive distribution of the work in the form in which it was published by this journal, but provided that the link to the first publication of the article in this journal is preserved.
A license agreement is a document in which the author warrants that he/she owns all copyright for the work (manuscript, article, etc.).
The authors, signing the License Agreement with TECHNOLOGY CENTER PC, have all rights to the further use of their work, provided that they link to our edition in which the work was published.
According to the terms of the License Agreement, the Publisher TECHNOLOGY CENTER PC does not take away your copyrights and receives permission from the authors to use and dissemination of the publication through the world's scientific resources (own electronic resources, scientometric databases, repositories, libraries, etc.).
In the absence of a signed License Agreement or in the absence of this agreement of identifiers allowing to identify the identity of the author, the editors have no right to work with the manuscript.
It is important to remember that there is another type of agreement between authors and publishers – when copyright is transferred from the authors to the publisher. In this case, the authors lose ownership of their work and may not use it in any way.