Smart charging process development based on ant colony optimization machine learning for controlling lead-acid battery charging capacity
DOI:
https://doi.org/10.15587/1729-4061.2024.299582Keywords:
smart charging, ant colony optimization, machine learning, lead acid batteryAbstract
The Indonesian government has targeted 2.1 million two-wheeled electric vehicles and 2,200 four-wheeled electric vehicles (EV) by 2025. This is hampered by limited electricity supply and EV charging, which takes long time. Multi device interleaved DC-DC bidirectional converter has been applied and assessed as the most suitable method for battery EV and plug-in hybrid EV because it produces high power >10 kW. For power below 10 kW, it is recommended to use a sinusoidal, Z-Source, and boost amplifier type converter. The smart charging (SC) system will be applied to electric vehicles, which only require a minimum charging power of around 169 W for four lead acid batteries. This paper focuses on an SC system that is capable of charging the battery quickly while still paying attention to the state of health (SoH) of the battery. The SC developed uses a DC-DC boost converter to increase the voltage produced by the switch mode power supply (SMPS). Estimated charging time is less than 30 minutes and still pay attention to the battery SoH. SC will also use pulse width modulation (PWM) as a duty power cycle regulator. This research applies a multi-layer perceptron (MLP) classifier to a neural network (NN). The results of the research show that smart charging can charge up to 600 W with an estimated charging time of around 11 minutes. The charging condition is above 60 % and the power duty cycle setting is 100 %. The power estimation results processed using the ant colony optimization (ACO) based neural network method show a root mean square deviation value of 0.010013430 for charging four lead acid batteries. These results are useful to help solve the problem of capacity requirements and battery charging speed for EVs, with good SoH
References
- Sun, X., Li, Z., Wang, X., Li, C. (2019). Technology Development of Electric Vehicles: A Review. Energies, 13 (1), 90. https://doi.org/10.3390/en13010090
- Li, Z., Khajepour, A., Song, J. (2019). A comprehensive review of the key technologies for pure electric vehicles. Energy, 182, 824–839. https://doi.org/10.1016/j.energy.2019.06.077
- Utami, M. W. D., Yuniaristanto, Y., Sutopo, W. (2020). Adoption Intention Model of Electric Vehicle in Indonesia. Jurnal Optimasi Sistem Industri, 19 (1), 70–81. https://doi.org/10.25077/josi.v19.n1.p70-81.2020
- Bozhkov, S. (2021). Structure of the Model of Hybrid Electric Vehicle Energy Efficiency. Trans Motauto World, 6 (3), 76–79. https://stumejournals.com/journals/tm/2021/3/76
- Ehsani, M., Singh, K. V., Bansal, H. O., Mehrjardi, R. T. (2021). State of the Art and Trends in Electric and Hybrid Electric Vehicles. Proceedings of the IEEE, 109 (6), 967–984. https://doi.org/10.1109/jproc.2021.3072788
- Arif, S. M., Lie, T. T., Seet, B. C., Ayyadi, S., Jensen, K. (2021). Review of Electric Vehicle Technologies, Charging Methods, Standards and Optimization Techniques. Electronics, 10 (16), 1910. https://doi.org/10.3390/electronics10161910
- Mohamed, N., Aymen, F., Ali, Z. M., Zobaa, A. F., Abdel Aleem, S. H. E. (2021). Efficient Power Management Strategy of Electric Vehicles Based Hybrid Renewable Energy. Sustainability, 13 (13), 7351. https://doi.org/10.3390/su13137351
- Nieuwenhuis, P., Cipcigan, L., Berkem Sonder, H. (2020). The Electric Vehicle Revolution. Future Energy, 227–243. https://doi.org/10.1016/b978-0-08-102886-5.00011-6
- Muslimin, S., Maulidda, R., Nawawi, M., Rahman, A., Kurnia, P. M. (2023). The Method of Constant Current - Constant Voltage (CC – CV) for SECA Electric Car Battery Charging with Fuzzy Logic Controller. Atlantis Highlights in Engineering, 14–23. https://doi.org/10.2991/978-94-6463-118-0_3
- Carter, R., Cruden, A., Hall, P. J., Zaher, A. S. (2012). An Improved Lead–Acid Battery Pack Model for Use in Power Simulations of Electric Vehicles. IEEE Transactions on Energy Conversion, 27 (1), 21–28. https://doi.org/10.1109/tec.2011.2170574
- B (2015). Dictionary of Energy, 41–81. https://doi.org/10.1016/b978-0-08-096811-7.50002-0
- Bergveld, H. J., Kruijt, W. S., Notten, P. H. L. (2002). Battery Management Systems. Springer Netherlands. https://doi.org/10.1007/978-94-017-0843-2
- Adityawan, A. P., Cahya, D. D., Sulistijono, L., Madyono (2013). Sistem Pengisian Batteray Lead Acid Secara Adaptive. PENS-ITS Sukolilo, 5 (1).
- Achaibou, N., Haddadi, M., Malek, A. (2008). Lead acid batteries simulation including experimental validation. Journal of Power Sources, 185 (2), 1484–1491. https://doi.org/10.1016/j.jpowsour.2008.06.059
- Setiaji, B., Dwiono, W., Tamam, M. T. (2020). Rancang Bangun Pengisi Baterai Lead Acid Dan Li-Ion Secara Otomatis Menggunakan Mikrokontroler PIC 16F877A Bersumber Energi Matahari Dengan Pengendali PI. Jurnal Riset Rekayasa Elektro, 1 (2). https://doi.org/10.30595/jrre.v1i2.5187
- Shahriari, M., Farrokhi, M. (2013). Online State-of-Health Estimation of VRLA Batteries Using State of Charge. IEEE Transactions on Industrial Electronics, 60 (1), 191–202. https://doi.org/10.1109/tie.2012.2186771
- Baterai Asam-Timbal. Dede Hendriono. Available at: https://henduino.github.io/library/ototronik/leadacid/
- Thowil Afif, M., Ayu Putri Pratiwi, I. (2015). Analisis Perbandingan Baterai Lithium-Ion, Lithium-Polymer, Lead Acid dan Nickel-Metal Hydride pada Penggunaan Mobil Listrik - Review. Jurnal Rekayasa Mesin, 6 (2), 95–99. https://doi.org/10.21776/ub.jrm.2015.006.02.1
- Wang, L., Qin, Z., Slangen, T., Bauer, P., van Wijk, T. (2021). Grid Impact of Electric Vehicle Fast Charging Stations: Trends, Standards, Issues and Mitigation Measures - An Overview. IEEE Open Journal of Power Electronics, 2, 56–74. https://doi.org/10.1109/ojpel.2021.3054601
- Town, G., Taghizadeh, S., Deilami, S. (2022). Review of Fast Charging for Electrified Transport: Demand, Technology, Systems, and Planning. Energies, 15 (4), 1276. https://doi.org/10.3390/en15041276
- Sidiq, R. K. (2015). Rancang Bangun Sistem Pengisi Baterai Mobil Listrik Berbasis Mikrokontroller Atmega16. Universitas Jember. Available at: https://repository.unej.ac.id/bitstream/handle/123456789/73490/111910201039--ROHMAT%20KHOIRUL%20SIDIQ-1-56.pdf?sequence=1&isAllowed=y
- Yusuf Margowadi, R. A. N., Samodra, B., Dawam, M. D. N. (2021). Pengembangan Pengisi Baterai Cepat 72 Volt Untuk Charging Station Kendaraan Listrik Roda Dua. Seminar Teknologi Bahan Dan Barang Teknik 2020, 49–54.
- Prianto, E., Yuniarti, N., Nugroho, D. C. (2020). Boost-converter sebagai alat pengisian baterai pada sepeda listrik secara otomatis. Jurnal Edukasi Elektro, 4 (1). https://doi.org/10.21831/jee.v4i1.32632
- Camacho, O. M. F., Mihet-Popa, L. (2016). Tests de recharge rapide et intelligente de batteries pour voitures électriques utilisant des énergies renouvelables. Oil and Gas Science and Technology, 71 (1).
- Syafii, Mayura, Y., El Gazaly, A. (2019). Characteristics of Lead-Acid Battery Charging and Discharging Against Residential Load in Tropical Area. 2019 16th International Conference on Quality in Research (QIR): International Symposium on Electrical and Computer Engineering. https://doi.org/10.1109/qir.2019.8898277
- Catherino, H. A., Burgel, J. F., Rusek, A., Feres, F. (1999). Modelling and simulation of lead-acid battery charging. Journal of Power Sources, 80 (1-2), 17–20. https://doi.org/10.1016/s0378-7753(98)00248-1
- Sadeghian, O., Oshnoei, A., Mohammadi-ivatloo, B., Vahidinasab, V., Anvari-Moghaddam, A. (2022). A comprehensive review on electric vehicles smart charging: Solutions, strategies, technologies, and challenges. Journal of Energy Storage, 54, 105241. https://doi.org/10.1016/j.est.2022.105241
- Xing, Y., Li, F., Sun, K., Wang, D., Chen, T., Zhang, Z. (2022). Multi-type electric vehicle load prediction based on Monte Carlo simulation. Energy Reports, 8, 966–972. https://doi.org/10.1016/j.egyr.2022.05.264
- Mololoth, V. K., Saguna, S., Åhlund, C. (2023). Blockchain and Machine Learning for Future Smart Grids: A Review. Energies, 16 (1), 528. https://doi.org/10.3390/en16010528
- Esmalifalak, M., Liu, L., Nguyen, N., Zheng, R., Han, Z. (2017). Detecting Stealthy False Data Injection Using Machine Learning in Smart Grid. IEEE Systems Journal, 11 (3), 1644–1652. https://doi.org/10.1109/jsyst.2014.2341597
- Teichgraeber, H., Brandt, A. R. (2019). Clustering methods to find representative periods for the optimization of energy systems: An initial framework and comparison. Applied Energy, 239, 1283–1293. https://doi.org/10.1016/j.apenergy.2019.02.012
- Sakhnini, J., Karimipour, H., Dehghantanha, A., Parizi, R. M. (2021). Physical layer attack identification and localization in cyber–physical grid: An ensemble deep learning based approach. Physical Communication, 47, 101394. https://doi.org/10.1016/j.phycom.2021.101394
- Javaid, N., Jan, N., Javed, M. U. (2021). An adaptive synthesis to handle imbalanced big data with deep siamese network for electricity theft detection in smart grids. Journal of Parallel and Distributed Computing, 153, 44–52. https://doi.org/10.1016/j.jpdc.2021.03.002
- Pallonetto, F., De Rosa, M., Milano, F., Finn, D. P. (2019). Demand response algorithms for smart-grid ready residential buildings using machine learning models. Applied Energy, 239, 1265–1282. https://doi.org/10.1016/j.apenergy.2019.02.020
- Srdic, S., Lukic, S. (2019). Toward Extreme Fast Charging: Challenges and Opportunities in Directly Connecting to Medium-Voltage Line. IEEE Electrification Magazine, 7 (1), 22–31. https://doi.org/10.1109/mele.2018.2889547
- Ronanki, D., Kelkar, A., Williamson, S. S. (2019). Extreme Fast Charging Technology—Prospects to Enhance Sustainable Electric Transportation. Energies, 12 (19), 3721. https://doi.org/10.3390/en12193721
- Tu, H., Feng, H., Srdic, S., Lukic, S. (2019). Extreme Fast Charging of Electric Vehicles: A Technology Overview. IEEE Transactions on Transportation Electrification, 5 (4), 861–878. https://doi.org/10.1109/tte.2019.2958709
- Ant colony optimization (2007). Handbook of Approximation Algorithms and Metaheuristics. Available at: https://www.taylorfrancis.com/chapters/mono/10.1201/9781420010749-33/ant-colony-optimization-teofilo-gonzalez?context=ubx&refId=21abeb5d-2476-4646-acdf-25c398f93e16
- Kao, Y., Cheng, K. (2006). An ACO-Based Clustering Algorithm. Lecture Notes in Computer Science, 340–347. https://doi.org/10.1007/11839088_31
- Dorigo, M., Gambardella, L. M. (1997). Ant colony system: a cooperative learning approach to the traveling salesman problem. IEEE Transactions on Evolutionary Computation, 1 (1), 53–66. https://doi.org/10.1109/4235.585892
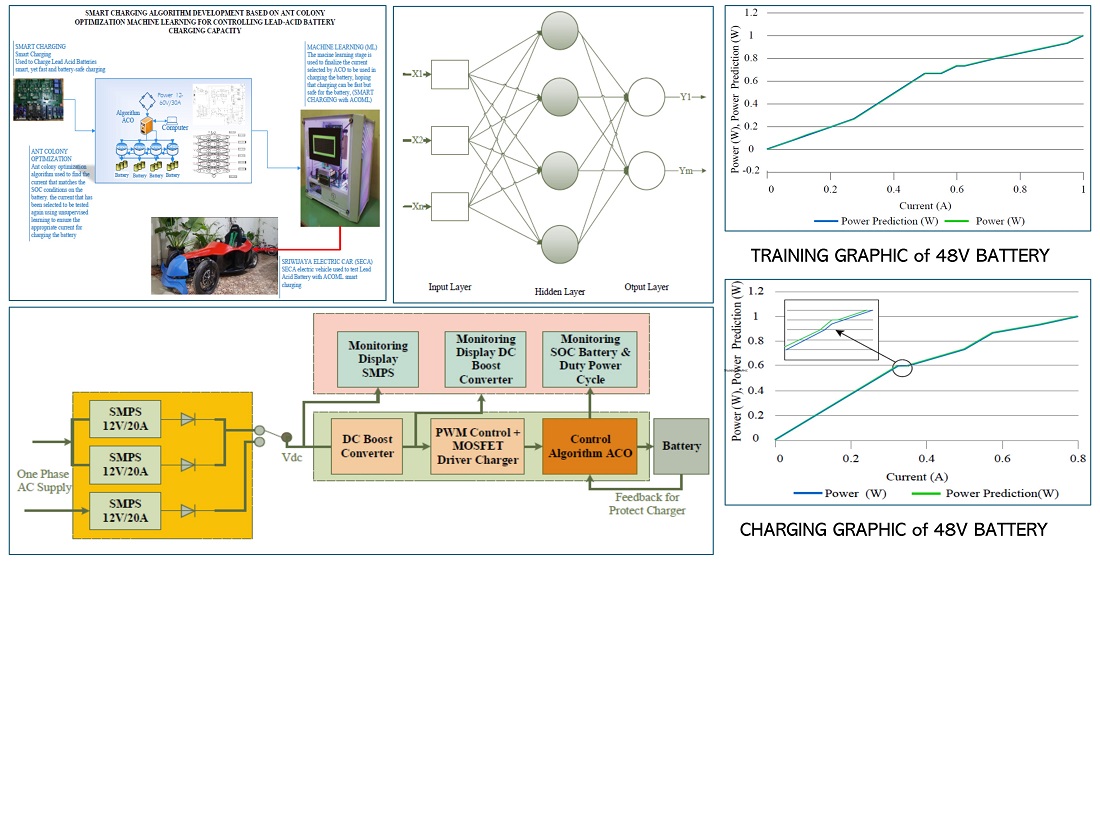
Downloads
Published
How to Cite
Issue
Section
License
Copyright (c) 2024 Selamat Muslimin, Zainuddin Nawawi, Bhakti Yudho Suprapto, Tresna Dewi

This work is licensed under a Creative Commons Attribution 4.0 International License.
The consolidation and conditions for the transfer of copyright (identification of authorship) is carried out in the License Agreement. In particular, the authors reserve the right to the authorship of their manuscript and transfer the first publication of this work to the journal under the terms of the Creative Commons CC BY license. At the same time, they have the right to conclude on their own additional agreements concerning the non-exclusive distribution of the work in the form in which it was published by this journal, but provided that the link to the first publication of the article in this journal is preserved.
A license agreement is a document in which the author warrants that he/she owns all copyright for the work (manuscript, article, etc.).
The authors, signing the License Agreement with TECHNOLOGY CENTER PC, have all rights to the further use of their work, provided that they link to our edition in which the work was published.
According to the terms of the License Agreement, the Publisher TECHNOLOGY CENTER PC does not take away your copyrights and receives permission from the authors to use and dissemination of the publication through the world's scientific resources (own electronic resources, scientometric databases, repositories, libraries, etc.).
In the absence of a signed License Agreement or in the absence of this agreement of identifiers allowing to identify the identity of the author, the editors have no right to work with the manuscript.
It is important to remember that there is another type of agreement between authors and publishers – when copyright is transferred from the authors to the publisher. In this case, the authors lose ownership of their work and may not use it in any way.