Information-analytical support to business processes for making investment decisions
DOI:
https://doi.org/10.15587/1729-4061.2024.304688Keywords:
business process algorithm, investment attractiveness, information-analytical supportAbstract
The object of this study is the business processes of making an investment decision based on determining the state of the investment attractiveness of the enterprise.
To support the adoption of investment decisions under the conditions of a fast-moving and dynamic environment, information-analytical support to the algorithm using intelligent information systems has been developed. The relevance of the study is justified by the continuous development of digitization processes, in particular in the financial realm. The traditional approach to the reproduction of management decision-making technology is complemented by the tools and methods of intelligent information systems. In particular, the modeling of the target subject area using UML made it possible to determine the main requirements for the projected information-analytical support (user roles, available options, types of connections and the logic of interaction between them). SQL queries to the information database speed up the process of processing and obtaining the necessary data samples. Business intelligence (BI) tools are used to create interactive reports that provide access to operational financial data. At the stage of making investment decisions, these tools make it possible to study a wide range of analytical data based on the results of the assessment of the investment attractiveness of the enterprise obtained at the previous stage of the developed algorithm. Monitoring of the main indicators of the enterprise's investment attractiveness is carried out on the basis of a dashboard, an information panel (display) with graphs, tables, and figures that clearly reflect the dynamics and rates of change of the investigated indicators. The results of the use of algorithmic information-analytical support make it possible to quickly prepare and make investment decisions. A visual description of the projected information-analytical support, visual content of the results of investment analysis, the validity of decisions due to the use of reliable retrospective information from an aggregated database
References
- Antoniuk, B. P. (2022). Osnovy alhorytmizatsii ta prohramuvannia. Ch. 1. Lutsk: Vezha-druk, 36. Available at: https://evnuir.vnu.edu.ua/bitstream/123456789/21329/1/ОАтП_ВСЕ02.pdf
- Number of fintechs worldwide from 2018 to 2024, by region. Available at: https://www.statista.com/statistics/893954/number-fintech-startups-by-region/
- Lei, X., Mohamad, U. H., Sarlan, A., Shutaywi, M., Daradkeh, Y. I., Mohammed, H. O. (2022). Development of an intelligent information system for financial analysis depend on supervised machine learning algorithms. Information Processing & Management, 59 (5), 103036. https://doi.org/10.1016/j.ipm.2022.103036
- Shiralkar, K., Bongale, A., Kumar, S., Bongale, A. M. (2023). An intelligent method for supply chain finance selection using supplier segmentation: A payment risk portfolio approach. Cleaner Logistics and Supply Chain, 8, 100115. https://doi.org/10.1016/j.clscn.2023.100115
- Hlibko, S., Vnukova, N., Davydenko, D., Pyvovarov, V., Avanesian, V. (2023). The Use of Linguistic Methods of Text Processing for the Individualization of the Bank’s Financial Servise. Proceedings of the 7th International Conference on Computational Linguistics and Intelligent Systems. Volume III: Intelligent Systems Workshop, 157–167. Available at: https://ceur-ws.org/Vol-3403/paper13.pdf
- Königstorfer, F., Thalmann, S. (2020). Applications of Artificial Intelligence in commercial banks – A research agenda for behavioral finance. Journal of Behavioral and Experimental Finance, 27, 100352. https://doi.org/10.1016/j.jbef.2020.100352
- Nalyvaichenko, K. (2013). Vplyv informatsiynykh system na efektyvnist investytsiynykh protsesiv na pidpryiemstvakh. Visnyk ekonomichnoi nauky Ukrainy, 2, 105–108. Available at: http://nbuv.gov.ua/UJRN/Venu_2013_2_28
- Liu, X., Yuan, X., Zhang, R., Ye, N. (2022). Risk Assessment and Regulation Algorithm for Financial Technology Platforms in Smart City. Computational Intelligence and Neuroscience, 2022, 1–13. https://doi.org/10.1155/2022/9903364
- Back, C., Morana, S., Spann, M. (2023). When do robo-advisors make us better investors? The impact of social design elements on investor behavior. Journal of Behavioral and Experimental Economics, 103, 101984. https://doi.org/10.1016/j.socec.2023.101984
- Cioranu, C., Cioca, M., Novac, C. (2015). Database Versioning 2.0, a Transparent SQL Approach Used in Quantitative Management and Decision Making. Procedia Computer Science, 55, 523–528. https://doi.org/10.1016/j.procs.2015.07.030
- Rao, A., Khankhoje, D., Namdev, U., Bhadane, C., Dongre, D. (2022). Insights into NoSQL databases using financial data: A comparative analysis. Procedia Computer Science, 215, 8–23. https://doi.org/10.1016/j.procs.2022.12.002
- Pavaloaia, V.-D., Strimbei, C. (2015). Experiments and Results by Modeling the Financial Domain with UML. Procedia Economics and Finance, 20, 510–517. https://doi.org/10.1016/s2212-5671(15)00103-3
- Karampure, R., Wang, C. Y., Vashi, Y. (2021). UML sequence diagram to axiomatic design matrix conversion: a method for concept improvement for software in integrated systems. Procedia CIRP, 100, 457–462. https://doi.org/10.1016/j.procir.2021.05.104
- Ding, D., Shen, Y., Jiang, J., Yuan, Q., Xiu, T., Ni, K., Liu, C. (2023). Data collection and information security analysis in sports teaching system based on intelligent sensor. Measurement: Sensors, 28, 100854. https://doi.org/10.1016/j.measen.2023.100854
- World investment report 2023. Available at: https://unctad.org/publication/world-investment-report-2023
- Kovalenko, A. G. (2013). Modern aspects of attractive investment analyses of enterprise. Efektyvna ekonomika, 7. Available at: http://www.economy.nayka.com.ua/?op=1&z=2165
- Kolodchak, O. M. (2013). Intelektualnyi analiz danykh. Visnyk Natsionalnoho universytetu "Lvivska politekhnika". Kompiuterni systemy ta merezhi, 773, 49–58. Available at: http://nbuv.gov.ua/UJRN/VNULPKSM_2013_773_11
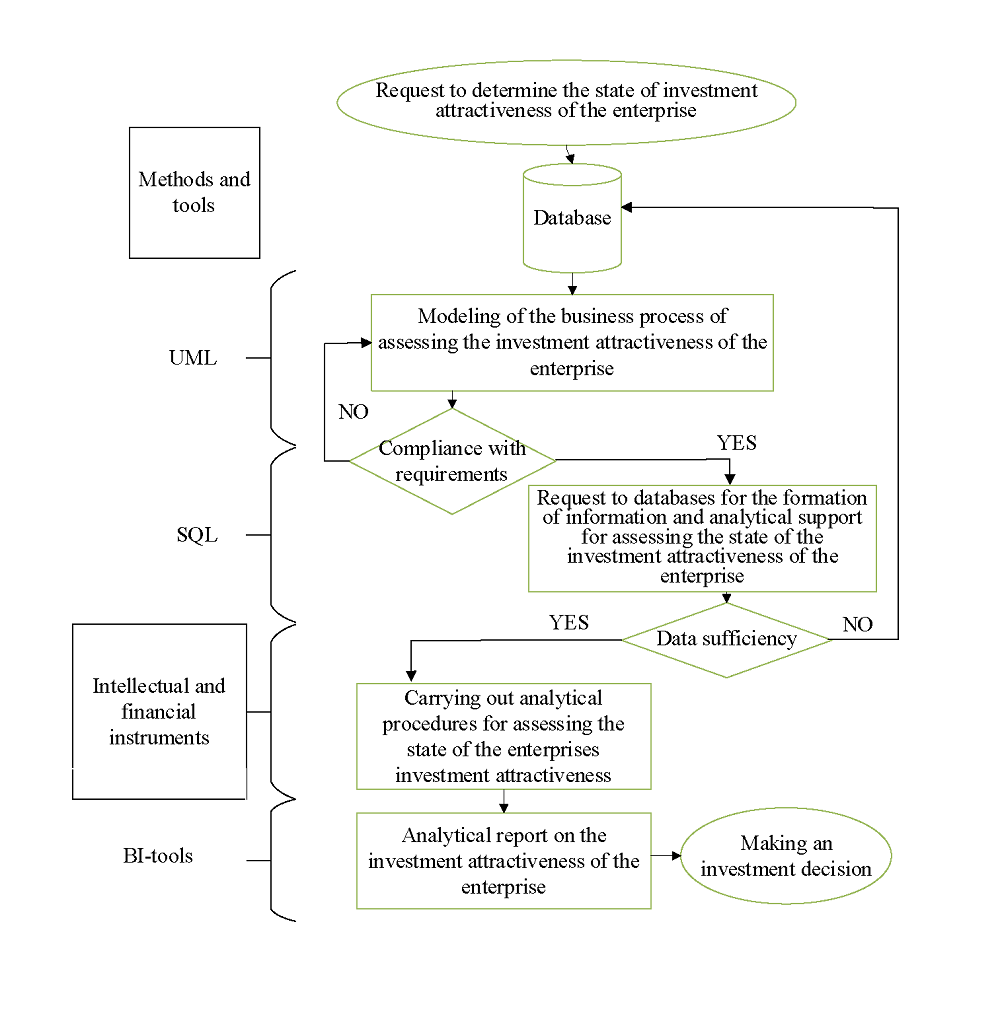
Downloads
Published
How to Cite
Issue
Section
License
Copyright (c) 2024 Nataliya Vnukova, Inna Aleksieienko, Svitlana Leliuk, Yevheniia Malyshko, Volodymyr Chernyshov

This work is licensed under a Creative Commons Attribution 4.0 International License.
The consolidation and conditions for the transfer of copyright (identification of authorship) is carried out in the License Agreement. In particular, the authors reserve the right to the authorship of their manuscript and transfer the first publication of this work to the journal under the terms of the Creative Commons CC BY license. At the same time, they have the right to conclude on their own additional agreements concerning the non-exclusive distribution of the work in the form in which it was published by this journal, but provided that the link to the first publication of the article in this journal is preserved.
A license agreement is a document in which the author warrants that he/she owns all copyright for the work (manuscript, article, etc.).
The authors, signing the License Agreement with TECHNOLOGY CENTER PC, have all rights to the further use of their work, provided that they link to our edition in which the work was published.
According to the terms of the License Agreement, the Publisher TECHNOLOGY CENTER PC does not take away your copyrights and receives permission from the authors to use and dissemination of the publication through the world's scientific resources (own electronic resources, scientometric databases, repositories, libraries, etc.).
In the absence of a signed License Agreement or in the absence of this agreement of identifiers allowing to identify the identity of the author, the editors have no right to work with the manuscript.
It is important to remember that there is another type of agreement between authors and publishers – when copyright is transferred from the authors to the publisher. In this case, the authors lose ownership of their work and may not use it in any way.