Профільний моніторинг контрольних карт залишків за моделю гамма-регресії
DOI:
https://doi.org/10.15587/1729-4061.2022.264904Ключові слова:
СДЦ, контрольні карти, ЕЗКС, УЛМ, гамма-регресія, залишкиАнотація
Статистична контрольна карта вважається одним із найкращих інструментів контролю якості. Нині контрольні карти знаходять широке застосування у різних галузях, зокрема у виробничих процесах. Вони є важливими інструментами, які можуть дати контролерам якості важливу інформацію для підтримки продуктивності. Якість продукту чи процесу можна охарактеризувати взаємозв’язком між двома чи більше змінними, яку зазвичай називають профілем. Також ще одним важливим напрямком, у якому широко використовуються контрольні картки, вважається нагляд за здоров’ям населення. У зв’язку з цим вони є дуже корисними та надійними інструментами для виявлення спалахів інфекційних захворювань. З іншого боку, модель гамма-регресії (МГР) є популярною моделлю, яка використовується в медицині та інших галузях. Вона застосовується, коли змінна відгуку є безперервною, з позитивною асиметрією і добре відповідає гамма-розподілу. У цій роботі представлена схема моніторингу профілю. На основі узагальненої лінійної моделі (УЛМ) у разі двох функцій зв’язку: тотожної та логарифмічної функції зв’язку. Пропонуються контрольні карти експоненційно зваженого ковзного середнього (ЕЗКС) з використанням залишків відхилення та залишків Пірсона для виявлення будь-якого порушення контрольної змінної моделі гамма-регресії. Детальне дослідження моделювання призначене для ретельного вивчення та оцінки ефективності контрольних карт на етапі аналізу I та на етапі II при параметричній оцінці максимальної правдоподібності (ОМП) з використанням показника середньої довжини циклу (СДЦ). Виявляється, використання залишків відхилення функції зв’язку тотожності здається більш придатним, ніж залишки Пірсона. Крім того, зі збільшенням розміру вибірки відсоток неконтрольованих зразків збільшувався, що теоретично прийнятно
Посилання
- Mahmoud, M. A. (2011). Simple Linear Profiles. Statistical Analysis of Profile Monitoring, 21–92. doi: https://doi.org/10.1002/9781118071984.ch2
- Mahmoud, M. A. (2012). The Performance of Phase II Simple Linear Profile Approaches when Parameters Are Estimated. Communications in Statistics - Simulation and Computation, 41 (10), 1816–1833. doi: https://doi.org/10.1080/03610918.2011.621570
- Amiri, A., Koosha, M., Azhdari, A., Wang, G. (2014). Phase I monitoring of generalized linear model-based regression profiles. Journal of Statistical Computation and Simulation, 85 (14), 2839–2859. doi: https://doi.org/10.1080/00949655.2014.942864
- Noorossana, R., Eyvazian, M., Vaghefi, A. (2010). Phase II monitoring of multivariate simple linear profiles. Computers & Industrial Engineering, 58 (4), 563–570. doi: https://doi.org/10.1016/j.cie.2009.12.003
- Mahmoud, M. A., Saad, A. E. N., El Shaer, R. (2014). Phase II Multiple Linear Regression Profile with Small Sample Sizes. Quality and Reliability Engineering International, 31 (5), 851–861. doi: https://doi.org/10.1002/qre.1644
- Roberts, S. W. (1959). Control Chart Tests Based on Geometric Moving Averages. Technometrics, 1 (3), 239–250. doi: https://doi.org/10.1080/00401706.1959.10489860
- Crowder, S. V. (1987). A Program for the Computation of ARL for Combined Individual Measurement and Moving Range Charts. Journal of Quality Technology, 19 (2), 103–106. doi: https://doi.org/10.1080/00224065.1987.11979046
- Lucas, J. M., Saccucci, M. S. (1990). Exponentially Weighted Moving Average Control Schemes: Properties and Enhancements. Technometrics, 32 (1), 1–12. doi: https://doi.org/10.1080/00401706.1990.10484583
- Woodall, W. H., Spitzner, D. J., Montgomery, D. C., Gupta, S. (2004). Using Control Charts to Monitor Process and Product Quality Profiles. Journal of Quality Technology, 36 (3), 309–320. doi: https://doi.org/10.1080/00224065.2004.11980276
- Knoth, S. (2007). Accurate ARL Calculation for EWMA Control Charts Monitoring Normal Mean and Variance Simultaneously. Sequential Analysis, 26 (3), 251–263. doi: https://doi.org/10.1080/07474940701404823
- Maravelakis, P. E., Castagliola, P., Khoo, M. B. C. (2017). Run length properties of run rules EWMA chart using integral equations. Quality Technology & Quantitative Management, 16 (2), 129–139. doi: https://doi.org/10.1080/16843703.2017.1372853
- Khan, N., Yasmin, T., Aslam, M., Jun, C.-H. (2018). On the performance of modified EWMA charts using resampling schemes. Operations Research and Decisions, 3, 29–43. doi: https://doi.org/10.5277/ord180303
- Akram, M. N., Amin, M., Qasim, M. (2021). A new biased estimator for the gamma regression model: Some applications in medical sciences. Communications in Statistics - Theory and Methods, 50 (23), 1–21. doi: https://doi.org/10.1080/03610926.2021.1977958
- Krishnamoorthy, K. (2006). Handbook of statistical distributions with applications. Chapman and Hall/CRC, 376. doi: https://doi.org/10.1201/9781420011371
- Jearkpaporn, D., Montgomery, D. C., Runger, G. C., Borror, C. M. (2003). Process monitoring for correlated gamma-distributed data using generalized-linear-model-based control charts. Quality and Reliability Engineering International, 19 (6), 477–491. doi: https://doi.org/10.1002/qre.521
- Braimah, O. J., Osanaiye, P. A., Omaku, P. E., Saheed, Y. K., Eshimokhai, S. A. (2014). On the Use of Exponentially Weighted Moving Average (Ewma) Contrl Chart in Monitoring Road Traffic Crashes. International Journal of Mathematics and Statistics Invention (IJMSI), 2 (5), 01–09. Available at: https://ijmsi.org/Papers/Volume.2.Issue.5/A0250109.pdf
- Cepeda-Cuervo, E., Corrales, M., Cifuentes, V., Zarate, H. (2016). On Gamma Regression Residuals. Journal of The Iranian Statistical Society, 15 (1), 29–44. Available at: http://jirss.irstat.ir/browse.php?a_code=A-11-304-2&slc_lang=en&sid=1
- Lu, C.-W., Reynolds, M. R. (1999). EWMA Control Charts for Monitoring the Mean of Autocorrelated Processes. Journal of Quality Technology, 31 (2), 166–188. doi: https://doi.org/10.1080/00224065.1999.11979913
- Wang, H. (2016). Application of Residual-Based EWMA Control Charts for Detecting Faults in Variable-Air-Volume Air Handling Unit System. Journal of Control Science and Engineering, 2016, 1–7. doi: https://doi.org/10.1155/2016/1467823
- Kang, L., Albin, S. L. (2000). On-Line Monitoring When the Process Yields a Linear Profile. Journal of Quality Technology, 32 (4), 418–426. doi: https://doi.org/10.1080/00224065.2000.11980027
- Messaoud, A., Theis, W., Hering, F., Weihs, C. (2008). Monitoring a Drilling Process Using Residual Control Charts. Quality Engineering, 21 (1), 1–9. doi: https://doi.org/10.1080/08982110802355869
- Areepong, Y. (2013). A comparison of performance of residual control charts for trend stationary AR(p) processes. International Journal of Pure and Apllied Mathematics, 85 (3). doi: https://doi.org/10.12732/ijpam.v85i3.13
- García-Bustos, S., Cárdenas-Escobar, N., Debón, A., Pincay, C. (2021). A control chart based on Pearson residuals for a negative binomial regression: application to infant mortality data. International Journal of Quality & Reliability Management, 39 (10), 2378–2399. doi: https://doi.org/10.1108/ijqrm-03-2021-0062
- Jamal, A., Mahmood, T., Riaz, M., Al-Ahmadi, H. M. (2021). GLM-Based Flexible Monitoring Methods: An Application to Real-Time Highway Safety Surveillance. Symmetry, 13 (2), 362. doi: https://doi.org/10.3390/sym13020362
- Kinat, S., Amin, M., Mahmood, T. (2019). GLM‐based control charts for the inverse Gaussian distributed response variable. Quality and Reliability Engineering International, 36 (2), 765–783. doi: https://doi.org/10.1002/qre.2603
- Nelder, J. A., Wedderburn, R. W. M. (1972). Generalized Linear Models. Journal of the Royal Statistical Society. Series A (General), 135 (3), 370. doi: https://doi.org/10.2307/2344614
- McCullagh, P., Nelder, J. A. (1989). Generalized Linear Models. Chapman and Hall/CRC. doi: https://doi.org/10.1007/978-1-4899-3242-6
- Bossio, M. C., Cuervo, E. C. (2015). Gamma regression models with the Gammareg R package. Comunicaciones En Estadística, 8 (2), 211. doi: https://doi.org/10.15332/s2027-3355.2015.0002.05
- Cuervo, E. C. (2001). Modelagem da Variabilidade em Modelos Lineares Generalizados. Instituto de Matemáticas, Universidade Federal do Río do Janeiro.
- Gipe, G. W. (1976). Using residual analysis to search for specification errors. Decision Sciences, 7 (1), 40–56. doi: https://doi.org/10.1111/j.1540-5915.1976.tb00656.x
- Shao, Y. E., Lin, Y. (2013). Applying Residual Control Charts to Identify the False Alarms in a TFT-LCD Manufacturing Process. Applied Mathematics & Information Sciences, 7 (4), 1459–1464. doi: https://doi.org/10.12785/amis/070426
- Pregibon, D. (1981). Logistic Regression Diagnostics. The Annals of Statistics, 9 (4). https://doi.org/10.1214/aos/1176345513
- Cordeiro, G. M., Simas, A. B. (2009). The distribution of Pearson residuals in generalized linear models. Computational Statistics & Data Analysis, 53 (9), 3397–3411. doi: https://doi.org/10.1016/j.csda.2009.02.025
- Pierce, D. A., Schafer, D. W. (1986). Residuals in Generalized Linear Models. Journal of the American Statistical Association, 81 (396), 977–986. doi: https://doi.org/10.1080/01621459.1986.10478361
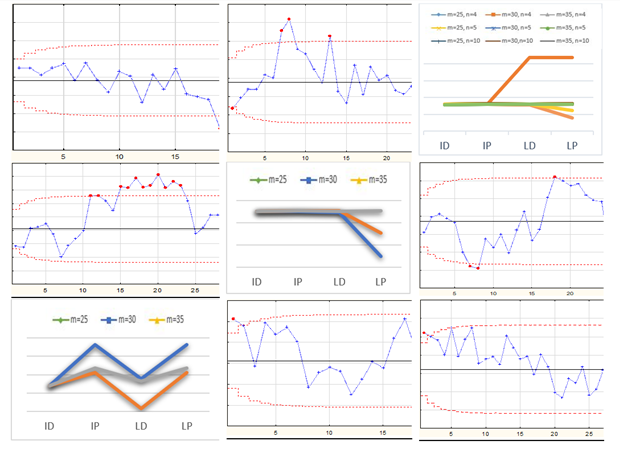
##submission.downloads##
Опубліковано
Як цитувати
Номер
Розділ
Ліцензія
Авторське право (c) 2022 Salah Mohamed, Engy Mohamed, Shereen Abdel Latif

Ця робота ліцензується відповідно до Creative Commons Attribution 4.0 International License.
Закріплення та умови передачі авторських прав (ідентифікація авторства) здійснюється у Ліцензійному договорі. Зокрема, автори залишають за собою право на авторство свого рукопису та передають журналу право першої публікації цієї роботи на умовах ліцензії Creative Commons CC BY. При цьому вони мають право укладати самостійно додаткові угоди, що стосуються неексклюзивного поширення роботи у тому вигляді, в якому вона була опублікована цим журналом, але за умови збереження посилання на першу публікацію статті в цьому журналі.
Ліцензійний договір – це документ, в якому автор гарантує, що володіє усіма авторськими правами на твір (рукопис, статтю, тощо).
Автори, підписуючи Ліцензійний договір з ПП «ТЕХНОЛОГІЧНИЙ ЦЕНТР», мають усі права на подальше використання свого твору за умови посилання на наше видання, в якому твір опублікований. Відповідно до умов Ліцензійного договору, Видавець ПП «ТЕХНОЛОГІЧНИЙ ЦЕНТР» не забирає ваші авторські права та отримує від авторів дозвіл на використання та розповсюдження публікації через світові наукові ресурси (власні електронні ресурси, наукометричні бази даних, репозитарії, бібліотеки тощо).
За відсутності підписаного Ліцензійного договору або за відсутністю вказаних в цьому договорі ідентифікаторів, що дають змогу ідентифікувати особу автора, редакція не має права працювати з рукописом.
Важливо пам’ятати, що існує і інший тип угоди між авторами та видавцями – коли авторські права передаються від авторів до видавця. В такому разі автори втрачають права власності на свій твір та не можуть його використовувати в будь-який спосіб.