Assessment of plant disease detection by deep learning
DOI:
https://doi.org/10.15587/1729-4061.2023.274483Keywords:
image processing, Inception v3, deep learning, classification, plant diseases, clusteringAbstract
Plant disease and pest detection machines were originally used in agriculture and have, to some extent, replaced traditional visual identification. Plant diseases and pests are important determinants of plant productivity and quality. Plant diseases and pests can be identified using digital image processing. According to the difference in the structure of the network, this study presents research on the detection of plant diseases and pests based on three aspects of the classification network, detection network, and segmentation network in recent years, and summarizes the advantages and disadvantages of each method. A common data set is introduced and the results of existing studies are compared. This study discusses possible problems in the practical application of plant disease and pest detection based on deep learning.
Conventional image processing algorithms or manual descriptive design and classifiers are often used for traditional computer vision-based plant disease and pest detection. This method usually uses various characteristics of plant diseases and pests to create an image layout and selects a useful light source and shooting angle to produce evenly lit images.
The purpose of this work is to identify a group of pests and diseases of domestic and garden plants using a mobile application and display the final result on the screen of a mobile device. In this work, data from 38 different classes were used, including diseased and healthy leaf images of 13 plants from plantVillage. In the experiment, Inception v3 tends to consistently improve accuracy with an increasing number of epochs with no sign of overfitting and performance degradation. Keras with Theano backend used to teach architectures
Supporting Agency
- For providing data on agricultural crops of Northern Kazakhstan in the preparation of this article, the author expresses gratitude to the Scientific and Production Center of Grain Farming named after A. I. Barayev.
References
- Mathew, A., Amudha, P., Sivakumari, S. (2020). Deep Learning Techniques: An Overview. Advanced Machine Learning Technologies and Applications, 599–608. doi: https://doi.org/10.1007/978-981-15-3383-9_54
- Ahmad, J., Farman, H., Jan, Z. (2018). Deep Learning Methods and Applications. SpringerBriefs in Computer Science, 31–42. doi: https://doi.org/10.1007/978-981-13-3459-7_3
- Golenko, Y., Ismailova, A., Shaushenova, A., Mutalova, Z., Dossalyanov, D., Ainagulova, A., Naizagarayeva, A. (2022). Implementation of machine learning models to determine the appropriate model for protein function prediction. Eastern-European Journal of Enterprise Technologies, 5 (4 (119)), 42–49. doi: https://doi.org/10.15587/1729-4061.2022.263270
- Tussupov, J., La, L., Mukhanova, A. (2014). A model of fuzzy synthetic evaluation method realized by a neural network. International Journal of Mathematical Models and Methods in Applied Sciences, 8, 103–106. Available at: https://www.naun.org/main/NAUN/ijmmas/2014/a142001-069.pdf
- Orazayeva, A., Wójcik, W., Pavlov, S., Tymchenko, L., Kokriatska, N., Tverdomed, V. et al. (2022). Biomedical image segmentation method based on contour preparation. Photonics Applications in Astronomy, Communications, Industry, and High Energy Physics Experiments 2022. doi: https://doi.org/10.1117/12.2657929
- Burger, W., Burge, M. J. (2022). Scale-Invariant Feature Transform (SIFT). Digital Image Processing, 709–763. doi: https://doi.org/10.1007/978-3-031-05744-1_25
- Yang, J., Huang, J., Jiang, Z., Dong, S., Tang, L., Liu, Y. et al. (2020). SIFT-aided path-independent digital image correlation accelerated by parallel computing. Optics and Lasers in Engineering, 127, 105964. doi: https://doi.org/10.1016/j.optlaseng.2019.105964
- Awaludin, M., Yasin, V. (2020). Application of Oriented Fast and Rotated Brief (Orb) and Bruteforce Hamming in Library Opencv for Classification of Plants. JISAMAR (Journal of Information System, Applied, Management, Accounting and Research), 4 (3), 51–59. Available at: https://journal.stmikjayakarta.ac.id/index.php/jisamar/article/view/247
- Rahmad, C., Asmara, R. A., Putra, D. R. H., Dharma, I., Darmono, H., Muhiqqin, I. (2020). Comparison of Viola-Jones Haar Cascade Classifier and Histogram of Oriented Gradients (HOG) for face detection. IOP Conference Series: Materials Science and Engineering, 732 (1), 012038. doi: https://doi.org/10.1088/1757-899x/732/1/012038
- Mohanty, A., Nam, A., Pozhitkov, A., Yang, L., Srivastava, S., Nathan, A. et al. (2020). A Non-genetic Mechanism Involving the Integrin β4/Paxillin Axis Contributes to Chemoresistance in Lung Cancer. IScience, 23 (9), 101496. doi: https://doi.org/10.1016/j.isci.2020.101496
- Charbuty, B., Abdulazeez, A. (2021). Classification Based on Decision Tree Algorithm for Machine Learning. Journal of Applied Science and Technology Trends, 2 (01), 20–28. doi: https://doi.org/10.38094/jastt20165
- Yessenova, M., Abdikerimova, G., Adilova, A., Yerzhanova, A., Kakabayev, N., Ayazbaev, T. et al. (2022). Identification of factors that negatively affect the growth of agricultural crops by methods of orthogonal transformations. Eastern-European Journal of Enterprise Technologies, 3 (2 (117)), 39–47. doi: https://doi.org/10.15587/1729-4061.2022.257431
- Chen, S., Webb, G. I., Liu, L., Ma, X. (2020). A novel selective naïve Bayes algorithm. Knowledge-Based Systems, 192, 105361. doi: https://doi.org/10.1016/j.knosys.2019.105361
- Hameed, Z., Zahia, S., Garcia-Zapirain, B., Javier Aguirre, J., María Vanegas, A. (2020). Breast Cancer Histopathology Image Classification Using an Ensemble of Deep Learning Models. Sensors, 20 (16), 4373. doi: https://doi.org/10.3390/s20164373
- Vangara, R. V. B., Thirupathur, K., Vangara, S. P. (2020). Opinion Mining Classification u sing Naive Bayes Algorithm. International Journal of Innovative Technology and Exploring Engineering, 9 (5), 495–498. doi: https://doi.org/10.35940/ijitee.e2402.039520
- Jignesh Chowdary, G., Punn, N. S., Sonbhadra, S. K., Agarwal, S. (2020). Face Mask Detection Using Transfer Learning of InceptionV3. Lecture Notes in Computer Science, 81–90. doi: https://doi.org/10.1007/978-3-030-66665-1_6
- Degadwala, S., Vyas, D., Biswas, H., Chakraborty, U., Saha, S. (2021). Image Captioning Using Inception V3 Transfer Learning Model. 2021 6th International Conference on Communication and Electronics Systems (ICCES). doi: https://doi.org/10.1109/icces51350.2021.9489111
- Berrar, D. (2019). Bayes’ Theorem and Naive Bayes Classifier. Encyclopedia of Bioinformatics and Computational Biology, 403–412. doi: https://doi.org/10.1016/b978-0-12-809633-8.20473-1
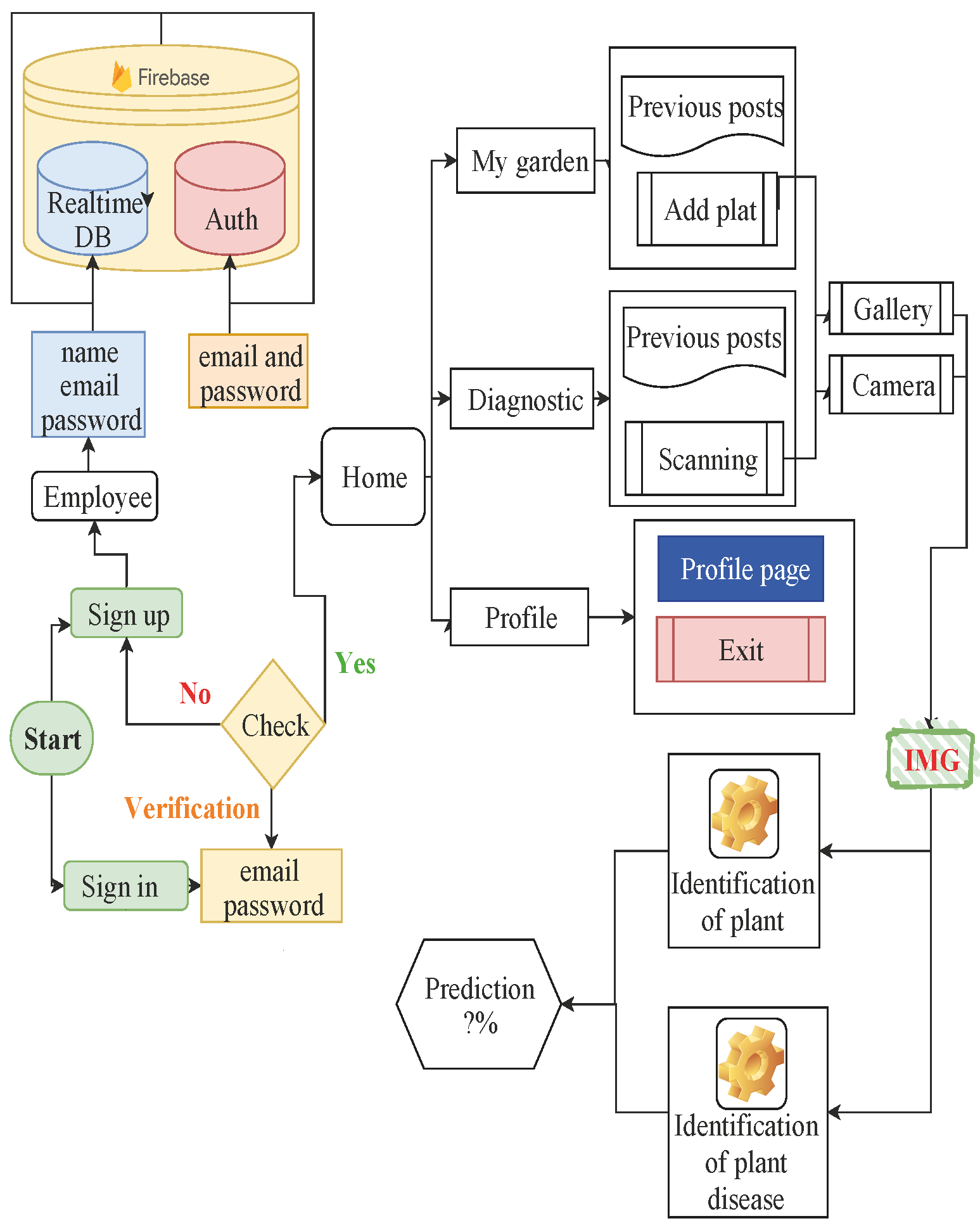
Downloads
Published
How to Cite
Issue
Section
License
Copyright (c) 2023 Akan Alpyssov, Nurgul Uzakkyzy, Ayazbaev Talgatbek, Raushan Moldasheva, Gulmira Bekmagambetova, Mnyaura Yessekeyeva, Dossym Kenzhaliev, Assel Yerzhan, Ailanysh Tolstoy

This work is licensed under a Creative Commons Attribution 4.0 International License.
The consolidation and conditions for the transfer of copyright (identification of authorship) is carried out in the License Agreement. In particular, the authors reserve the right to the authorship of their manuscript and transfer the first publication of this work to the journal under the terms of the Creative Commons CC BY license. At the same time, they have the right to conclude on their own additional agreements concerning the non-exclusive distribution of the work in the form in which it was published by this journal, but provided that the link to the first publication of the article in this journal is preserved.
A license agreement is a document in which the author warrants that he/she owns all copyright for the work (manuscript, article, etc.).
The authors, signing the License Agreement with TECHNOLOGY CENTER PC, have all rights to the further use of their work, provided that they link to our edition in which the work was published.
According to the terms of the License Agreement, the Publisher TECHNOLOGY CENTER PC does not take away your copyrights and receives permission from the authors to use and dissemination of the publication through the world's scientific resources (own electronic resources, scientometric databases, repositories, libraries, etc.).
In the absence of a signed License Agreement or in the absence of this agreement of identifiers allowing to identify the identity of the author, the editors have no right to work with the manuscript.
It is important to remember that there is another type of agreement between authors and publishers – when copyright is transferred from the authors to the publisher. In this case, the authors lose ownership of their work and may not use it in any way.