Виявлення бур'янів на полях на основі технології комп'ютерного зору
DOI:
https://doi.org/10.15587/1729-4061.2023.284600Ключові слова:
комп'ютерний зір, сегментація зображень, модель нейронної мережі, алгоритми розпізнавання образівАнотація
Проблема множинності зон у комп'ютерному зорі, зокрема розпізнавання образів в агросекторі займає особливе місце в області штучного інтелекту у сучасному аспекті.
Об'єктом дослідження є розпізнавання бур'янових рослин на основі глибокого навчання і комп'ютерного зору. Предметом дослідження є ефективне використання моделей нейронних мереж у навчанні, що включає в себе класифікацію та обробку з використанням дата сетів рослин та бур'янів. Актуальність дослідження полягає у вимозі сучасного світу щодо застосування нових інформаційних технологій в агропромисловій сфері, що сприяє підвищенню ефективності роботи агропромислових комплексів. Інтерес приватних сільгосппідприємств та держави викликаний збільшенням зростання врожайності сільгосппродуктів. Для розпізнавання бур'янів використовувалися методи машинного навчання, зокрема нейронні мережі. За допомогою моделі Марка описаний процес розпізнавання бур'янів, в результаті обробки 1562 картин отримані сегментовані зображення. У зв'язку зі щорічним посиленням бур'янів на території Казахстану і в ході вирішення цих проблем розроблений новий код для розпізнавання рослин та прописаний в програмний модуль сканера. Сканер, в свою чергу, забезпечує автоматичне виявлення бур'янів. За результатами навченої нейронної мережі на основі моделі нейромережі MaskRCNN, прописаної в програмному модулі сканера, що відповідає новим стандартам часу, покращена автоматизована система сканування та розпізнавання рослин. Бур'янову рослину було розпізнано в середньому за 0,2 секунди з показником точності 89 %, при цьому додатковий людський фактор був повністю видалений. Застосування нових технологій допомагає боротися з бур'янами та сприяє вирішенню проблеми боротьби з ними
Посилання
- Patrício, D. I., Rieder, R. (2018). Computer vision and artificial intelligence in precision agriculture for grain crops: A systematic review. Computers and Electronics in Agriculture, 153, 69–81. doi: https://doi.org/10.1016/j.compag.2018.08.001
- Gomes, J. F. S., Leta, F. R. (2012). Applications of computer vision techniques in the agriculture and food industry: a review. European Food Research and Technology, 235 (6), 989–1000. doi: https://doi.org/10.1007/s00217-012-1844-2
- Urmashev, B., Buribayev, Z., Amirgaliyeva, Z., Ataniyazova, A., Zhassuzak, M., Turegali, A. (2021). Development of a weed detection system using machine learning and neural network algorithms. Eastern-European Journal of Enterprise Technologies, 6 (2 (114)), 70–85. doi: https://doi.org/10.15587/1729-4061.2021.246706
- Sunil, G. C., Zhang, Y., Koparan, C., Ahmed, M. R., Howatt, K., Sun, X. (2022). Weed and crop species classification using computer vision and deep learning technologies in greenhouse conditions. Journal of Agriculture and Food Research, 9, 100325. doi: https://doi.org/10.1016/j.jafr.2022.100325
- Tian, H., Wang, T., Liu, Y., Qiao, X., Li, Y. (2020). Computer vision technology in agricultural automation – A review. Information Processing in Agriculture, 7 (1), 1–19. doi: https://doi.org/10.1016/j.inpa.2019.09.006
- Ahmad, Z., Shahid Khan, A., Wai Shiang, C., Abdullah, J., Ahmad, F. (2020). Network intrusion detection system: A systematic study of machine learning and deep learning approaches. Transactions on Emerging Telecommunications Technologies, 32 (1). doi: https://doi.org/10.1002/ett.4150
- Li, Y., Randall, C. J., Woesik, R. van, Ribeiro, E. (2019). Underwater video mosaicing using topology and superpixel-based pairwise stitching. Expert Systems with Applications, 119, 171–183. doi: https://doi.org/10.1016/j.eswa.2018.10.041
- Sivarajan, S., Maharlooei, M., Bajwa, S. G., Nowatzki, J. (2018). Impact of soil compaction due to wheel traffic on corn and soybean growth, development and yield. Soil and Tillage Research, 175, 234–243. doi: https://doi.org/10.1016/j.still.2017.09.001
- Liu, H., Lee, S.-H., Chahl, J. S. (2016). A review of recent sensing technologies to detect invertebrates on crops. Precision Agriculture, 18 (4), 635–666. doi: https://doi.org/10.1007/s11119-016-9473-6
- Sabzi, S., Abbaspour-Gilandeh, Y., García-Mateos, G. (2018). A fast and accurate expert system for weed identification in potato crops using metaheuristic algorithms. Computers in Industry, 98, 80–89. doi: https://doi.org/10.1016/j.compind.2018.03.001
- Toseef, M., Khan, M. J. (2018). An intelligent mobile application for diagnosis of crop diseases in Pakistan using fuzzy inference system. Computers and Electronics in Agriculture, 153, 1–11. doi: https://doi.org/10.1016/j.compag.2018.07.034
- Magic, M., Magic, J. (2019). Image Classification Using Python and Techniques of Computer Vision and Machine Learning. Independently published, 114.
- Huang, Y., Jiang, L., Han, T., Xu, S., Liu, Y., Fu, J. (2022). High-Accuracy Insulator Defect Detection for Overhead Transmission Lines Based on Improved YOLOv5. Applied Sciences, 12 (24), 12682. doi: https://doi.org/10.3390/app122412682
- Kubo, S., Yamane, T., Chun, P. (2022). Study on Accuracy Improvement of Slope Failure Region Detection Using Mask R-CNN with Augmentation Method. Sensors, 22 (17), 6412. doi: https://doi.org/10.3390/s22176412
- Osorio, K., Puerto, A., Pedraza, C., Jamaica, D., Rodríguez, L. (2020). A Deep Learning Approach for Weed Detection in Lettuce Crops Using Multispectral Images. AgriEngineering, 2 (3), 471–488. doi: https://doi.org/10.3390/agriengineering2030032
- Almodaresi, S. A., Mohammadrezaei, M., Dolatabadi, M., Nateghi, M. R. (2019). Qualitative Analysis of Groundwater Quality Indicators Based on Schuler and Wilcox Diagrams: IDW and Kriging Models. Journal of Environmental Health and Sustainable Development. doi: https://doi.org/10.18502/jehsd.v4i4.2023
- Tseng, H.-H., Yang, M.-D., Saminathan, R., Hsu, Y.-C., Yang, C.-Y., Wu, D.-H. (2022). Rice Seedling Detection in UAV Images Using Transfer Learning and Machine Learning. Remote Sensing, 14 (12), 2837. doi: https://doi.org/10.3390/rs14122837
- Chilukuri, D. M., Yi, S., Seong, Y. (2022). A robust object detection system with occlusion handling for mobile devices. Computational Intelligence, 38 (4), 1338–1364. doi: https://doi.org/10.1111/coin.12511
- Yu, Y., Zhang, K., Yang, L., Zhang, D. (2019). Fruit detection for strawberry harvesting robot in non-structural environment based on Mask-RCNN. Computers and Electronics in Agriculture, 163, 104846. doi: https://doi.org/10.1016/j.compag.2019.06.001
- Su, W.-H., Zhang, J., Yang, C., Page, R., Szinyei, T., Hirsch, C. D., Steffenson, B. J. (2020). Automatic Evaluation of Wheat Resistance to Fusarium Head Blight Using Dual Mask-RCNN Deep Learning Frameworks in Computer Vision. Remote Sensing, 13 (1), 26. doi: https://doi.org/10.3390/rs13010026
- Valladares, S., Toscano, M., Tufiño, R., Morillo, P., Vallejo-Huanga, D. (2021). Performance Evaluation of the Nvidia Jetson Nano Through a Real-Time Machine Learning Application. Intelligent Human Systems Integration 2021, 343–349. doi: https://doi.org/10.1007/978-3-030-68017-6_51
- Jain, N., Gupta, V., Shubham, S., Madan, A., Chaudhary, A., Santosh, K. C. (2021). Understanding cartoon emotion using integrated deep neural network on large dataset. Neural Computing and Applications, 34 (24), 21481–21501. doi: https://doi.org/10.1007/s00521-021-06003-9
- Liu, W., Chen, S., Guo, L., Zhu, X., Liu, J. (2021). CPTR: Full transformer network for image captioning. arXiv. doi: https://doi.org/10.48550/arXiv.2101.10804
- Rashid, K. M Louis, J. (2019). Times-series data augmentation and deep learning for construction equipment activity recognition. Advanced Engineering Informatics, 42, 100944. doi: https://doi.org/10.1016/j.aei.2019.100944
- Srivastava, S., Divekar, A. V., Anilkumar, C., Naik, I., Kulkarni, V., Pattabiraman, V. (2021). Comparative analysis of deep learning image detection algorithms. Journal of Big Data, 8 (1). doi: https://doi.org/10.1186/s40537-021-00434-w
- Ghayour, L., Neshat, A., Paryani, S., Shahabi, H., Shirzadi, A., Chen, W. et al. (2021). Performance Evaluation of Sentinel-2 and Landsat 8 OLI Data for Land Cover/Use Classification Using a Comparison between Machine Learning Algorithms. Remote Sensing, 13 (7), 1349. doi: https://doi.org/10.3390/rs13071349
- Liu, Y.-C., Ma, C.-Y., He, Z., Kuo, C.-W., Chen, K., Zhang, P. et al. (2021). Unbiased teacher for semi-supervised object detection. arXiv. doi: https://doi.org/10.48550/arXiv.2102.09480
- Yeshmukhametov, A. N., Koganezawa, K., Buribayev, Z., Amirgaliyev, Y., Yamamoto, Y. (2020). Study on multi-section continuum robot wire-tension feedback control and load manipulability. Industrial Robot: The International Journal of Robotics Research and Application, 47 (6), 837–845. doi: https://doi.org/10.1108/ir-03-2020-0054
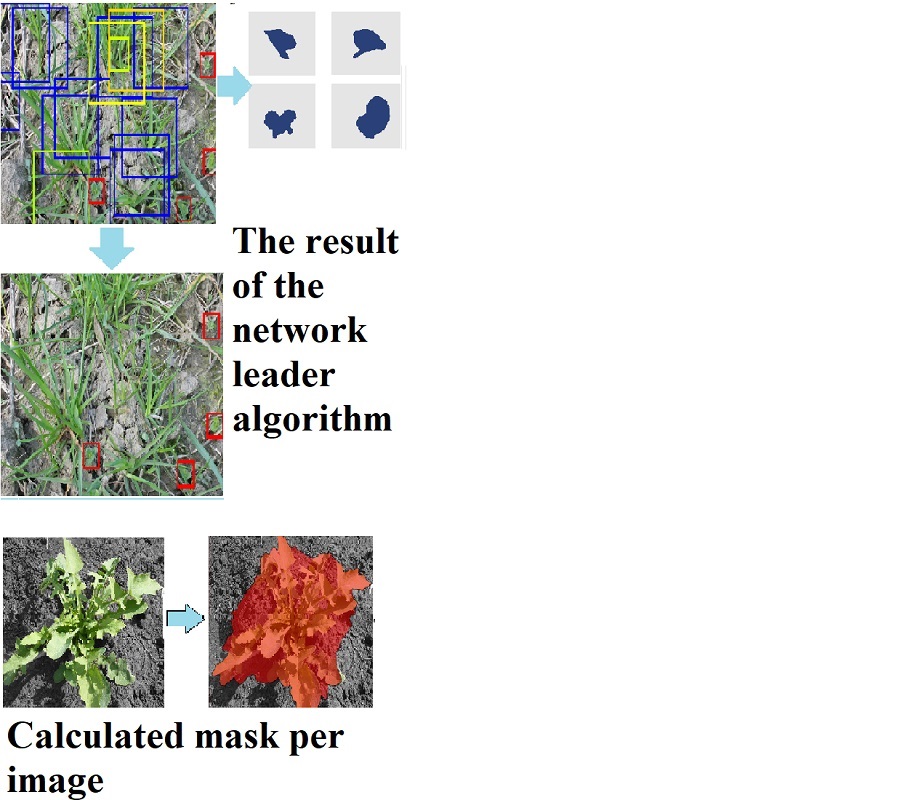
##submission.downloads##
Опубліковано
Як цитувати
Номер
Розділ
Ліцензія
Авторське право (c) 2023 Mira Kaldarova, Akerke Аkanova, Aizhan Nazyrova, Assel Mukanova, Assemgul Tynykulova

Ця робота ліцензується відповідно до Creative Commons Attribution 4.0 International License.
Закріплення та умови передачі авторських прав (ідентифікація авторства) здійснюється у Ліцензійному договорі. Зокрема, автори залишають за собою право на авторство свого рукопису та передають журналу право першої публікації цієї роботи на умовах ліцензії Creative Commons CC BY. При цьому вони мають право укладати самостійно додаткові угоди, що стосуються неексклюзивного поширення роботи у тому вигляді, в якому вона була опублікована цим журналом, але за умови збереження посилання на першу публікацію статті в цьому журналі.
Ліцензійний договір – це документ, в якому автор гарантує, що володіє усіма авторськими правами на твір (рукопис, статтю, тощо).
Автори, підписуючи Ліцензійний договір з ПП «ТЕХНОЛОГІЧНИЙ ЦЕНТР», мають усі права на подальше використання свого твору за умови посилання на наше видання, в якому твір опублікований. Відповідно до умов Ліцензійного договору, Видавець ПП «ТЕХНОЛОГІЧНИЙ ЦЕНТР» не забирає ваші авторські права та отримує від авторів дозвіл на використання та розповсюдження публікації через світові наукові ресурси (власні електронні ресурси, наукометричні бази даних, репозитарії, бібліотеки тощо).
За відсутності підписаного Ліцензійного договору або за відсутністю вказаних в цьому договорі ідентифікаторів, що дають змогу ідентифікувати особу автора, редакція не має права працювати з рукописом.
Важливо пам’ятати, що існує і інший тип угоди між авторами та видавцями – коли авторські права передаються від авторів до видавця. В такому разі автори втрачають права власності на свій твір та не можуть його використовувати в будь-який спосіб.